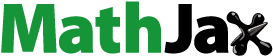
Abstract
The identification of factors affecting longevity in dairy cows is of great importance to improve their length of productive life. Cows can be replaced for poor milk production (i.e. true productive lifespan, TPL) or other reasons than production (functional productive lifespan, FPL). The objective of the present study was to investigate the influence of milk yield, fat and protein contents, insemination status (INS), somatic cell count (SCC) and β-hydroxybutyrate (BHB) concentration in milk on TPL and FPL. Data included 70,748 Italian Holstein-Friesian cows that calved for the first time between 2014 and 2018. Proportional hazards models assuming a piecewise Weibull distribution of the baseline hazard function, with or without adjustment for milk production, were used to compute relative risks of culling associated with FPL and TPL, respectively. Our study revealed that milk yield, protein and fat contents, INS, SCC and concentration of BHB in milk exhibited significant relationships with TPL and FPL. In particular, the huge difference in risk ratios between high- and low-producing cows confirms the central role of milk production in culling decision-making. In addition, farmers appear more prone to cull cows with several events of high SCC and BHB concentration in milk.
Milk yield, fat and protein contents, insemination status, somatic cell count and ketosis affect productive lifespan in Italian Holstein cows.
These factors, therefore, contribute significantly to culling decisions.
The identified risk factors of culling can be exploited to improve the current management practices in Italian Holstein Friesian herds and cows’ longevity.
HIGHLIGHTS
Introduction
Reduced cow longevity is a growing concern for the dairy industry (Schuster et al. Citation2020) because animals with longer productive life are globally more efficient, bringing economic, environmental and animal welfare benefits. Dairy cows’ longevity can be measured as both herd life (time from birth to culling) and productive life (PL; time from first calving to culling) (Ducrocq et al. Citation1988; Schuster et al. Citation2020). A dairy cow is biologically able to have a herd life of up to 20 years but, usually, cows remain in the herd for a much shorter time than their life expectancy (De Vries and Marcondes Citation2020). In developed countries, the average PL of dairy cows ranges between 2.5 and 4 years (De Vries and Marcondes Citation2020). With a specific focus on Italian Holstein-Friesian cows, the National Breeders Association (Associazione Italiana Allevatori Citation2020) reports that the average PL for the year 2020 was 2.3 lactations (corresponding to approximately 2.6 years of life), with a slightly decreasing trend in the last 15 years. The time that cows spend in the herd is mainly determined by management decisions made by individual farmers. Culling is due to a large extent to the voluntary discard of low-producing cows. However, poor fertility, health issues and injuries may force farmers to involuntarily cull also high-producing cows, with detrimental effects on farm profits (Dallago et al. Citation2021). The ability to avoid voluntary culling can be defined as ‘true productive lifespan’ (TPL), whereas the aptitude to delay culling for involuntary reasons is the ‘functional productive lifespan’ (FPL) (Ducrocq et al. Citation1988). Several studies in the literature identified non-genetic factors affecting PL in different dairy cattle breeds. Age at first calving, lactation stage, milk production, variation in herd size, as well as fertility problems, poor udder health and diseases are factors that contribute significantly to culling decisions (Beaudeau et al. Citation2000; Olechnowicz et al. Citation2016).
Productive lifespan in dairy cattle is most often analysed by survival analysis (e.g. Ducrocq Citation2005; Sewalem et al. Citation2006). This methodology allows the inclusion of both alive and dead individuals, it accounts for non-linear characteristics of life data, and it can accommodate the model effects that vary with time (such as herd size and milk yield).
Identification of risk factors for culling is of great importance to better understand culling decision-making and improve PL in a given breed. When talking specifically about TPL and FPL, the scientific literature is lacking in studies that investigate factors affecting them in Italian Holstein-Friesian cows. To our knowledge, the only study of Samoré et al. (Citation2003) was carried out in this breed and it was limited to assessing the relationship between somatic cell count (SCC) and functional longevity.
Hence, the objective of the present study was to assess the influence of production, reproduction and health traits collected by the official test days (TD) on TPL and FPL in Italian Holstein-Friesian cows using survival analysis.
Material and methods
Data editing
Data used in the present study were provided by the Italian Holstein breed association (ANAFIBJ) and included the TD of all the Italian Holstein cows controlled in the Veneto Region (north-eastern Italy) from 2014 to 2018. Specifically, the following information was provided for each cow: cow’s official identification number; date of birth; dates of calvings; dates of artificial inseminations (AI); date of culling or death of the animal; and data collected at each TD performed approximately every 40 days during the lactation and including milk yield; milk fat, protein and lactose content; SCC; and β-hydroxybutyrate (BHB) concentration in milk which is used as an indicator of clinical ketosis (CK).
Herds with a mean of at least 5 calvings per year between July 2014 and March 2018 were selected. Moreover, just cows in the selected herds and with at least 1 TD measurement in the first lactation were considered. After editing, the dataset included 117,003 lactations from 70,748 Italian Holstein-Friesian cows, reared in 1,111 herds. The average herd size was 63.7 ± 86.8 (±SD). The investigated animals calved for the first time at 27.7 ± 4.5 months of age and the average number of lactations of the cows included in the analysis was 1.7 ± 0.8.
Test-day measurements for fat and protein contents, SCC, and BHB were set as missing in the case of abnormal values: fat content lower than 20 g/kg or higher than 90 g/kg; protein content lower than 15 g/kg or higher 70 g/kg; SCC equal to 0; BHB lower than 0.
Data were edited and prepared for survival analysis using R statistical software version 3.5.0 (R Core Team Citation2018) and FORTRAN 90.
Computation and classification of the effects
In the present study, we investigated the influence of several traits related to production, reproduction and health status on productive lifespan in Italian Holstein cows. Milk yield (MILK), fat (FAT) and protein (PROT) contents from 305-day lactation length were classified as reported in Rostellato et al. (Citation2021). Within herd-year deviations for the three traits were computed and transformed into 10 classes of equal size for MILK and 5 classes for FAT and PROT. The first class represented the worst within herd-year deviation, whereas the latest included cows with the best within herd-year deviation for the trait. An extra class for each trait was added to include lactations shorter than 60 days, for which extrapolation to 305 days is not accurate.
The investigated trait related to reproduction was the insemination status (INS). To study how INS influences culling decisions, it is necessary to make assumptions about what information is available to the farmer (Rostellato et al. Citation2021). The exact moment when the farmer knows the pregnancy status of the cows and the moment when the culling decision is taken is lacking in our dataset. In the Italian dairy system, the pregnancy status of the cows is usually assessed by ultrasound or rectal palpation after approximately 40 days from the last AI. We assumed that the farmer does not know the pregnancy status of the animals during the first 2 months after the AI. After this delay and in presence of a positive pregnancy test, the cow is usually considered pregnant. In case of a negative result, the farmer can choose between inseminating her again or not. Hence, INS was classified as follows: class 0 corresponds to the complete absence of information about AI during the ongoing lactation; class 1 to class 4 represent the unknown outcome of the last AI (this last AI being respectively the 1st, 2nd, 3rd or 4th one of the current lactation); class 5 to class 8 represent the cases where the farmer is considered to know the pregnancy status of the cow (either positive or negative), starting 2 months after the latest AI (this last AI is respectively the 1st, 2nd, 3rd, 4th AI after calving).
Somatic cell count and BHB were considered traits related to cows’ health status. The former was classified as the number of TD per lactation with SCC higher than 400,000 cells/ml. Hence, 7 successive classes were defined: from 0 (no TD with SCC >400,000 cells/ml so far during a given lactation) to 6 (6 or more TD with SCC >400,000 cells/ml).
In order to classify BHB, the number of TD per lactation having BHB ≥0.2 mmol/l was counted. As reported in Benedet et al. (Citation2019), this concentration in milk can be used to identify cows affected by CK. The trait was classified into two classes: class 0 represented the absence of TD with BHB concentration ≥0.2 mmol/l during a given lactation, whereas class 1 included cows with at least one TD having BHB ≥0.2 mmol/l.
Survival analysis
Productive lifespan was calculated as the time between the date of the first calving and the date of culling or censoring. Right-censored records included PL of cows still alive at the limit date of 31 August 2017, cows that moved to another herd (censoring at the date of the first change of herd), and PL of animals with lactation length longer than 800 days (censoring at 800 days in milk). Moreover, the PL of cows that calved for the first time before 1 January 2015, and with subsequent calving within the investigated period was left-censored. Productive life for cows that died or left the herd for disposal was considered uncensored. Animals that calved for the first time after the limit date (31 August 2017) were ignored.
The influence of milk production traits, INS, SCC and BHB on TPL (i.e. the ability to avoid voluntary culling) and FPL (i.e. the ability to avoid involuntary culling) were evaluated using a proportional hazard model assuming a piecewise Weibull distribution of the baseline hazard function, implemented in the software Survival Kit v6.12 (Mészàros et al. Citation2013).
To study TPL and FPL, two different base models were used. True productive lifespan was investigated using the following base model:
(1)
(1)
where λijkl(t) is the hazard function of an individual at time t; λ0(t′) is a piecewise Weibull baseline hazard function for the nth lactation stage (1–4, with changes at days 150 and 250 and when the cow was dried, class 4 being the dry period) of the pth parity (1–4) with specific scale parameter λ and shape parameter ρ for each lactation × stage combination and with t′ being the number of days since the beginning of the current lactation (as in Ducrocq Citation2005);
is the time-dependent year-season effect;
is the time-independent fixed effects of age at the first calving classified in 19 monthly classes from month 22 to month 40;
is the time-dependent effect of variation of herd size classified in 14 categories;
is the random effect of herd-year which is assumed to follow a log-gamma distribution with parameter γ. As reported in Ducrocq et al. (Citation1988), FPL can be obtained including a correction for the probability to be culled for low milk production. Hence, the base model for FPL was as EquationEquation (1)
(1)
(1) with the inclusion of MILK, FAT and PROT as follows:
(2)
(2)
Insemination status, SCC and BHB were added to the two base models and tested one at a time. In addition, a model that included all factors at the same time was investigated.
To evaluate the significance of the effects added to the models, log-likelihood ratio test (LRT) statistics were computed. The statistics were calculated as follows:
(3)
(3)
where LogL is the log-likelihood of a model. These statistics are assumed to follow a chi-square distribution with as many degrees of freedom as the number of estimable parameters added to the reduced model.
Results and discussion
Survival analysis
Survival analysis was performed on 57,787 Holstein-Friesian cows. The percentage of right-censored and uncensored records was 73.4% and 26.6%, respectively. In addition, data of 7,034 cows (12.2%) were left-truncated. The average age at culling, calculated as the time between the birth date and the culling date, was 3.4 ± 0.8 years. Among the 15,320 uncensored cows, 59% and 34.8% were culled during the first and second lactation, respectively. The minimum and maximum censoring times within lactation were 1 day and 800 days, respectively. The average within-lactation failure time was 219 days, suggesting that culling occurred in general before the end of the lactation.
Log-likelihood ratio test
To compare the ability of the investigated traits to improve the goodness of fit and, hence, to evaluate whether they had a significant relationship with TPL and FPL, LRT statistics were computed. Results are reported in Table . All the investigated traits had highly statistically significant associations with both TPL and FPL (p < 0.01). As expected, MILK is the trait exhibiting the strongest influence of PL. In addition, the strong relationship of INS with both TPL and FPL confirms that fertility plays a key role in culling decisions.
Table 1. Likelihood ratio test to compare the investigated modelsa.
Estimates of relative risks of culling
The magnitude of risks of being culled from a model including all factors at the same time was very close to those estimated tested each factor distinctly from others (data not shown). Because the study aimed at investigating each of the investigated traits individually, only RR for the factors tested one at a time are presented and discussed hereinafter.
Relative risks (RR) of being culled for AGE are presented in Figure . Cows that calved for the first time after 2 years of age exhibited a higher risk of being culled with respect to animals in the reference class (AGE = 24). The slight decrease in RR between 35 and 38 months of age may be due to a low number of uncensored individuals for those classes. The trends are quite similar between TPL and FLP. Our results are consistent with other studies (e.g. Ducrocq Citation2005; Zavadilová and Štípková Citation2013).
Figure 1. Relative risk of culling for age at the first calving associated to true productive lifespan (TPL) and functional productive lifespan (FPL). Significance of the χ2 statistic, testing the difference between risk ratios associated to each level and that one of the reference class (class 24 months): *p < 0.05; **p < 0.01.
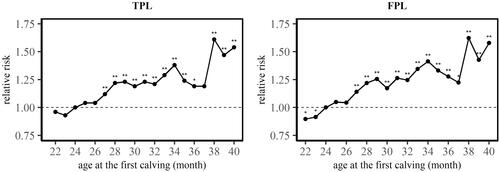
The estimated RR of being culled for milk production traits are shown in Figure . Relative risks for MILK (Figure ), decreased continuously from class 1 (worst within herd-year deviation for 305-day milk yield) to class 10 (best within herd-year deviation for 305-day milk yield). In particular, animals with positive within herd-year deviations for the trait (classes 6–10) exhibited a significantly lower risk to be culled with respect to the reference class (MILK = 5). This suggests that cows with a high milk yield are somewhat protected from culling, which is also in line with the findings from previous studies (e.g. Hadley et al. Citation2006; Pinedo et al. Citation2010) that reported a lower risk of culling for high-producing or higher-than-average producing cows. Conversely, the highest risk ratios were estimated for cows with the worst within herd-year deviation for 305-day milk yield (MILK = 1) and cows with lactation length <60 days. This is in agreement with the study of Rostellato et al. (Citation2021).
Figure 2. (A–C) Relative risk of culling for within herd-year deviations for milk yield (MILK), protein (PROT) and fat (FAT) contents. Significance of the χ2 statistic, testing the difference between risk ratios associated to each level and that one of the reference class (class 5 for MILK; class 3 for FAT and PROT): **p < 0.01. MILK was classified in 10 classes using within herd-year deviations of 305 days milk yield from bottom 10% (class 1) to top 10% (class 10). The class MILK = 11 included cows with lactation shorter than 60 days. PROT and FAT were classified in 5 classes using within herd-year deviations of 305 days PROT or FAT contents from bottom 20% (class 1) to top 20% (class 5). The sixth class included cows with lactation shorter than 60 days. Relative risk for class 6 for PROT and FAT were fixed to 1 because they are not estimable at the same time with class 11 for MILK. In fact, they strictly correspond to the same cows.
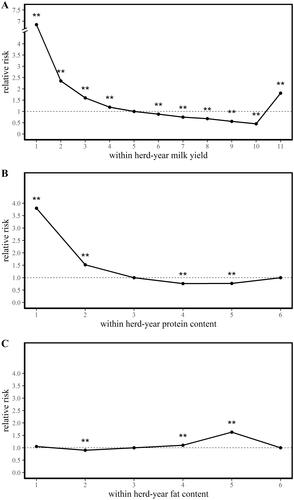
Similarly to MILK, RR for PROT (Figure ) decreased from class 1 (worst within herd-year deviation for 305-day protein content) to class 5 (best within herd-year deviation for 305-day protein content). The lower risk of culling associated with high protein content reflects the breeding goals of the Italian Holstein-Friesian cows, where great importance is given to the protein content in milk, which is mainly used for cheese production (Cassandro et al. Citation2016). Particularly, about 48% of the milk produced in Italy is intended for PDO (protected designation of origin) cheese production and another relevant share of the total domestic production goes to other cheese products (CLAL – ITALIA Citation2019).
Relative risks of culling for FAT are reported in Figure . Cows with a negative within herd-year deviation for 305-day fat content (FAT = 2) showed a slightly lower RR with respect to animals in the reference class (FAT = 3). In contrast, the risk to be culled for cows with positive within herd-year deviation for 305-day fat content (classes 4 and 5) increased. The opposite trend for FAT with respect to MILK and PROT was unexpected. Additional investigations, on a larger dataset, to evaluate how the interaction between the three production traits influence risk of culling would be useful to clarify the influence of FAT on PL.
Table reports RR for INS. The highest risk of being culled was estimated for cows not inseminated (INS = 0). It can be explained by the presence, in that class, of animals that were very early considered not suitable for another lactation due to low production, reproductive and health problems, or poor conformation. Cows with an unknown outcome for the AI (classes 1–4) showed higher RR with respect to animals with known pregnancy status (classes 5–8). In particular, cows with a known outcome after the 2nd to 4th AI exhibited a significantly lower risk of being culled with respect to the reference class (INS = 1). Moreover, risk ratios associated with TPL and FPL were very close. The lower risk of being culled for cows with known pregnancy status reflects the presence of animals with successful AI, which were kept in the herd until calving. In contrast, the higher RR for cows with an unknown outcome for AI suggests that a cow may be culled before knowing the AI result, probably because the animal exhibits some severe health troubles.
Table 2. Relative risk of culling for artificial insemination (AI) status associated to true (TPL) and functional (FPL) productive lifespana.
Risk ratios for SCC are presented in Table . Cows with one or more TD having SCC >400,000 cell/ml have a higher risk to be culled with respect to the reference class (SCC = 0). In particular, the risk of being culled increased from class 1 to class 4. The slight decrease of RR for classes 4–6, can be explained by the low number of uncensored failures for those classes. Our results agree with the previous studies investigating the influence of SCC on cow longevity (Samoré et al. Citation2003; Sewalem et al. Citation2006). They suggest that farmers seem to be more prone to cull cows with repeated events of high concentration of somatic cells in milk, which can be associated with a likely decrease in milk yield caused by subclinical mastitis. Moreover, high SCC in bulk milk leads to a reduction in profits due to the penalties established by the Italian milk payment system for milk of poor quality. The variation of RR across SCC classes was quite similar between TPL and FPL. The smaller differences in risk of being culled associated with TPL and FLP with respect to the previous study of Rostellato et al. (Citation2021) can be due to the short investigated period considered in the present work.
Table 3. Relative risk of culling for somatic cell count (SCC) associated to true (TPL) and functional (FPL) productive lifespana.
Table reports the RR for BHB. The class representing the absence of TD with BHB concentration ≥0.2 mmol/l during a given lactation was chosen as the reference class. Cows with at least one TD having BHB ≥0.2 mmol/l (i.e. animals that experienced CK) showed a higher risk to be culled with respect to cows in the reference class (BHB = 0). This is in agreement with the previous studies in the literature, where CK was identified as a risk factor for the culling of dairy cows (Gröhn et al. Citation1998; Seifi et al. Citation2011). Ketosis has detrimental effects on the production, reproduction and health status of the animals, increasing their risk of being culled (McArt et al. Citation2013; Raboisson et al. Citation2014). In the case of BHB, RR associated with TPL were higher than for FPL, suggesting that farmers are more prone to cull cows that experienced the disease and that are not able to maintain high milk production.
Table 4. Relative risk of culling for β-hydroxybutyrate (BHB) associated to true (TPL) and functional (FPL) productive lifespana.
Implications and further investigations
According to the data from the National Breeders Association (Associazione Italiana Allevatori Citation2020), about 1.13 million Holstein cows are reared in Italy, 88% of which are in northern Italy. The Italian Holstein farming system is quite standardised throughout the country, with cows mainly reared indoors in loose housing stalls and fed a total mixed ration. Data used in this study correspond to the Holstein cows population of the Veneto region, which can be considered a reliable sample of the Italian Holstein herds.
The time span covered by the dataset was shorter (4-years period) compared to other studies (e.g. Rostellato et al. Citation2021). It constrained the analysis of TPL and FPL for cows that stayed longer in the herds and the investigation of possible effects due to changes in herd management. However, it is worth noticing that the current average national PL of Italian Holstein cows is quite short (2.33 lactations). In addition, 39% of the national population is made of primiparous cows, whereas 30% consists of second parity cows. This means that a very large proportion of culling decisions (both voluntary and involuntary) occur early in the productive life of Italian Holsteins. This leads to several disadvantages from economical, environmental, and animal welfare points of view (Boulton et al. Citation2017; De Vries Citation2020; De Vries and Marcondes Citation2020). In order to guarantee to pay back the heifer rearing costs and start generating profit, farmers should keep cows in the herd at least until the third lactation. In addition, the reduction in the number of replacement stock needed to be reared may reduce the environmental footprint. Finally, longer PL reflects better animal welfare. In this context, the identified factors affecting TPL and FPL may be exploited to improve current management practices and breeding actions to limit culling in early life and to improve cows’ PL.
Further investigations on a larger dataset and including data recorded during a longer period of time could be useful to better understand the difference in RR associated with TPL and FPL (e.g. for SCC) and to evaluate whether the management practices that can change over time can affect our conclusions. Moreover, the investigation of additional traits (e.g. morphology traits), would be beneficial to better understanding factors affecting TPL and FPL in the Italian Hostein-Friesian breed.
Conclusions
We investigated the influence of several productive, reproductive and health traits gained from the official TD on the productive lifespan of Italian Holstein-Friesian cows in order to identify risk factors for culling. Milk production traits, insemination status, somatic cell count and concentration of β-hydroxybutyrate in milk showed a significant relationship with true (TPL) and functional productive lifespan (FLP). Hence, they play an important role in culling decisions. The huge difference in risk ratios between high- and low-producing cows, as well as differences in RR between TPL and FPL for BHB, confirms the determining role of milk yield on the farmers’ decision to cull a cow.
Ethical approval
Data used in this study were gathered from the archives of the Italian Holstein Breed Association, therefore no in-vivo cow manipulation was performed and no approval by any ethics committee was required.
Disclosure statement
No potential conflict of interest was reported by the author(s).
Data availability statement
The data that support the findings of this study were provided by the Italian Holstein breed association (ANAFIBJ). Restrictions apply to the availability of these data, which were used under licence for this study. Data are available from the authors with the permission of ANAFIBJ.
Additional information
Funding
References
- Associazione Italiana Allevatori. 2020. Bollettino on line. Rome (Italy): AIA; [accessed 2021 June 23]. http://bollettino.aia.it/
- Beaudeau F, Seegers H, Ducrocq V, Fourichon C, Bareille N. 2000. Effect of health disorders on culling in dairy cows/ a review and a critical discussion. Ann. Zootech. 49:293–311.
- Benedet A, Manuelian CL, Zidi A, Penasa M, De Marchi M. 2019. Invited review: β-hydroxybutyrate concentration in blood and milk and its associations with cow performance. Animal. 13(8):1676–1689.
- Boulton AC, Rushton J, Wathes DC. 2017. An empirical analysis of the cost of rearing dairy heifers from birth to first calving and the time taken to repay these costs. Animal. 11(8):1372–1380.
- Cassandro M, Pretto D, Lopez-Villalobos N, De Marchi M, Penasa M. 2016. Estimation of economic values for milk coagulation properties in Italian Holstein-Friesian cattle. J Dairy Sci. 99(8):6619–6626.
- CLAL – ITALIA. 2019. Produzione latte ed utilizzazione. Modena (Italy): CLAL; [accessed 2021 September 8]. https://www.clal.it/.
- Dallago GM, Wade KM, Cue RI, McClure JT, Lacroix R, Pellerin D, Vasseur E. 2021. Keeping dairy cows for longer: a critical literature review on dairy cow longevity in high milk-producing countries. Animals. 11(3):808.
- De Vries A, Marcondes MI. 2020. Review: overview of factors affecting productive lifespan of dairy cows. Animal. 14(S1):s155–s164.
- De Vries A. 2020. Symposium review: why revisit dairy cattle productive lifespan? J Dairy Sci. 103(4):3838–3845.
- Ducrocq V. 2005. An improved model for the French genetic evaluation of dairy bulls on length of productive life of their daughters. Anim Sci. 80(3):249–256.
- Ducrocq V, Quaas RL, Pollak EJ, Casella G. 1988. Length of productive life of dairy cows. 1. Justification of a Weibull model. J Dairy Sci. 71(11):3061–3070.
- Gröhn TY, Eicker SW, Ducrocq V, Hertl JA. 1998. Effect of diseases on the culling of Holstein dairy cows in New York State. J Dairy Sci. 81(4):966–978.
- Hadley GL, Wolf CA, Harsh SB. 2006. Dairy cattle culling patterns, explanations, and implications. J Dairy Sci. 89(6):2286–2296.
- McArt JAA, Nydam DV, Oetzel GR, Overton TR, Ospina PA. 2013. Elevated non-esterified fatty acids and β-hydroxybutyrate and their association with transition dairy cows performance. Vet J. 198(3):560–570.
- Mészàros G, Sölkner J, Ducrocq V. 2013. The survival kit: software to analyze survival data including possibly correlated random effects. Comput Methods Programs Biomed. 110(3):503–510.
- Olechnowicz J, Kneblewski PJ, Jaśkowski M, Wlodarek J. 2016. Effect of selected factors on longevity in cattle: a review. The J Anim Plant Sci. 26(6):1533–1541.
- Pinedo PJ, De Vries A, Webb DV. 2010. Dynamics of culling risk with disposal codes reported by Dairy Herd Improvement dairy herds. J Dairy Sci. 93(5):2250–2261.
- R Core Team. 2018. R: A language and environment for statistical computing. Vienna, Austria: R Foundation for Statistical Computing. [accessed 2020 September 10] https://www.R-project.org/
- Raboisson D, Mounié M, Maigné E. 2014. Diseases, reproductive performance, and changes in milk production associated with subclinical ketosis in dairy cows: a meta-analysis and review. J Dairy Sci. 97(12):7547–7563.
- Rostellato R, Promp J, Leclerc H, Mattalia S, Friggens NC, Boichard D, Ducrocq V. 2021. Influence of production, reproduction, type and health traits on true and functional longevity in French dairy cattle. J Dairy Sci. 104(12):12664–12678.
- Samoré AB, Schneider M, Canavesi F, Bagnato A, Groen AF. 2003. Relationship between somatic cell count and functional longevity assessed using survival analysis in Italian Holstein-Friesian cows. Livest Prod Sci. 80(3):211–220.
- Schuster CJ, Barkema HW, De Vries A, Kelton DF, Orsel K. 2020. Invited review: academic and applied approach to evaluating longevity in dairy cows. J Dairy Sci. 103(12):11008–11024.
- Seifi HA, LeBlanc SJ, Leslie KE, Duffield TF. 2011. Metabolic predictors of post-partum disease and culling risk in dairy cattle. Vet J. 188(2):216–220.
- Sewalem A, Miglior F, Kistemaker GJ, Van Doormaal BJ. 2006. Analysis of the relationship between somatic cell score and functional longevity in Canadian dairy cattle. J Dairy Sci. 89(9):3609–3614.
- Zavadilová L, Štípková M. 2013. Effect of age at first calving on longevity and fertility traits for Holstein cattle. Czech J Anim Sci. 58(2):47–57.