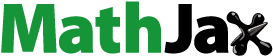
ABSTRACT
In light of the widespread use of big data analytics, internet users are increasingly confronted with algorithmic decision-making. Developing algorithm literacy is thus crucial to empower users to successfully navigate digital environments. In this paper, we present the development and validation of an algorithm literacy scale that consists of two interrelated dimensions: 1) awareness of algorithms use and 2) knowledge about algorithms. To validate the scale, we use item response theory and report findings from two studies. In study 1, we tested 46 items among N = 331 participants, resulting in a 32-item pool. These items were tested in a second study among N = 1,041 German internet users. The final scale consists of each 11 items measuring algorithm awareness and knowledge. Both subscales correlated positively with participants’ subjective coding skills and proved to be an appropriate predictor for participants’ handling of algorithmic curation in three test-scenarios.
Introduction
The use of and reliance on big data analytics has been growing in almost all areas of our society (Ball & Webster, Citation2020). For end users, these data-driven practices have become visible through the large-scale implementation of algorithms across the internet. Internet users almost constantly encounter algorithms tailoring the selection and presentation of online content (e.g., through the analysis of user and behavior related data, see Gran et al., Citation2021; Sundar, Citation2020). The implementation of personalization and recommendation services is widespread across social, communicative, and economic activities online, such as search engines, social media and content, or product recommender systems (Latzer et al., Citation2016). Research has started inquiring into the potential effects of algorithmically curated content in areas such as online news (Helberger, Citation2019; Thorson, Citation2020), music and video streaming (Hallinan & Striphas, Citation2016; Prey, Citation2017), or online information searching (Fletcher & Nielsen, Citation2018). Algorithm-based decisions largely govern how internet users perceive the world. Algorithms manage what news “finds us,” what entertainment content we are confronted with on social media, what music we listen to on our streaming services, which potential romantic partners we interact with, or what products we see and purchase online.
Acknowledging that algorithms largely govern our digital lives and can be considered powerful actors with more or less visible encoded values and assumptions of our world (Gillespie, Citation2014), raises the question of whether internet users are aware and understand algorithmic systems. Being aware of and knowing about algorithms can at least limit the exploitation and manipulation of users through algorithmic operations and allows them to maintain their autonomy in decision-making (Dogruel et al., Citation2020; Burrell et al., Citation2019; Susser et al., Citation2019). Understanding the role of the user has also implications for policy making and the governance of algorithmic systems (Katzenbach & Ulbricht, Citation2019; Latzer & Festic, Citation2019; Yeung, Citation2018). Besides policy measures that aim at increasing transparency and accountability related to algorithms (Ananny & Crawford, Citation2018; Hancock et al., Citation2020; Shin & Park, Citation2019), literacy has been regarded as a crucial factor in protecting individuals against the risks that come with algorithms (Gran et al., Citation2021; Kemper & Kolkman, Citation2018).
In light of this, we argue that studying internet users’ algorithm literacy is essential for understanding how users navigate and evaluate algorithmically curated digital spheres. We conceptualize algorithm literacy by focusing on its cognitive dimensions, which we define as the combination of being aware of the use of algorithms in online applications, platforms, and services and knowing how algorithms work (i.e., understanding the types, functions, and scopes of algorithmic systems on the internet; cf. Dogruel, Citation2021). While research has started to study users’ awareness and knowledge of algorithmically curated online environments, valid measures of internet users’ algorithm literacy are lacking (Hargittai et al., Citation2020; Hobbs, Citation2020). Therefore, we have little evidence for whether algorithm literacy is universally shared and whether this could be a new domain of potential inequalities regarding digital spheres (Cotter & Reisdorf, Citation2020; Klawitter & Hargittai, Citation2018).
In this paper, we develop and validate a comprehensive scale that measures two cognitive dimensions of algorithm literacy: awareness of algorithm use and b) knowledge about algorithms. In the following, we will outline the importance of studying algorithm literacy and discuss prior attempts to measure algorithm literacy. Subsequently, we will describe the scale development process in depth and present comprehensive analyses to assess the scale’s quality.
Why study algorithm literacy?
The capability to use new technologies and the media in a meaningful and purposeful way has largely been studied and discussed under the concept of (media) literacy (Koltay, Citation2011). The competent navigation of online environments that largely rely on algorithmic curation can thus be described as being algorithm literate. Assessing the required knowledge and skills in navigating such algorithmic-curated online environments is relevant for several reasons: First, the ubiquity of algorithmic applications that internet users encounter online can be cited as the prime reason for studying internet users’ awareness, knowledge, and hence literacy of these systems (Gran et al., Citation2021; Dogruel et al., Citation2020). Taking further into account that algorithms are socio-technological systems and that user-algorithm interactions are based on a mutual shaping of each other (Gillespie, Citation2014; Kitchin, Citation2017), users’ active role in algorithmic systems curation as well as their impact on algorithmic decision-making needs to be accounted for (Sundar, Citation2020). With their online behavior and data, users have a crucial role in contributing to algorithmic decision-making and their understanding of these systems has the potential to shape algorithmic operations.
Second, algorithmic decision-making is associated with a wide range of potential risks, ranging from privacy infringement, forms of political and economic manipulation, censorship, and discrimination to biases in computing outputs (Latzer et al., Citation2016). A lack of literacy may increase users’ vulnerability to potential negative effects, both on the individual but also the societal level (Cotter & Reisdorf, Citation2020; Gran et al., Citation2021), such as users being subject to manipulation and exploitation (Susser et al., Citation2019). Systematically studying users’ algorithm literacy allows for implementing evidence-based policy interventions (Potter & Thai, Citation2019) to strengthen users’ reflection and coping behaviors to finally uphold their autonomy in navigating online environments (Sundar, Citation2020).
Third, awareness and perceptions of algorithms have been found to affect online behavior (Bucher, Citation2017; Gillespie, Citation2014) as demonstrated by studies of algorithms on dating platforms (Sharabi, Citation2020) or on the Facebook newsfeed (Rader & Gray, Citation2015). This is closely related to the threat of a new digital divide as previous research has found that users’ skills in coping with algorithms’ impact can differ substantially. For instance, so-called “power users” have often developed their own specific ways of dealing with algorithms and manipulating them (Bishop, Citation2019; Bucher, Citation2012; Cotter, Citation2019). Not being able to identify, understand, and counter the impact of algorithmic decisions has the potential to reinforce a digital divide where some users benefit greatly from online services, while others’ engagement is limited and hardly self-determined (Gran et al., Citation2021; Lutz, Citation2019).
Fourth, a valid measure of algorithm literacy allows to address this aspect in empirical research design, e.g., by implementing it as a moderating or mediating factor to study the strength of algorithmic effects in online settings or investigating influence factors to interrogate to what degree algorithm literacy is determined by or related to other sociodemographic, attitudinal, or competence-related variables (Zarouali et al., Citation2021).
Existing approaches toward studying algorithm literacy
Most recently, a growing number of empirical studies have started to examine components of algorithm literacy, namely internet users’ perceptions and awareness of algorithmically curated online environments as well as users’ coping strategies. Most notably, studies have conceptualized users’ awareness and knowledge regarding algorithmic curation in connection with social network sites and the embedded newsfeed curation (Brodsky et al., Citation2020; Bucher, Citation2012, Citation2017; Eslami et al., Citation2015; Hamilton et al., Citation2014; Leong, Citation2020; Powers, Citation2017; Rader & Gray, Citation2015; Schwartz & Mahnke, Citation2020) as well as algorithm literacy during internet use in general (Hargittai et al., Citation2020; Klawitter & Hargittai, Citation2018). From a methodological point of view, most of these studies were qualitative analyses of user perceptions of algorithmic curation embedded in these particular services, partly through probing perceptions of algorithms at work (e.g., Bucher, Citation2017; Eslami et al., Citation2015; Hamilton et al., Citation2014; Rader & Gray, Citation2015). A few studies have started to operationalize algorithm literacy more generally by using standardized measures that capture different domains of algorithmic operations (Cotter & Reisdorf, Citation2020; Gran et al., Citation2021; Latzer et al., Citation2020). In these studies, algorithm literacy focused primarily on users’ awareness of algorithmic curation, such as personalization of search results, news, social media content, and product recommendations or tailored advertising.
Adopting a qualitative approach, yet focusing on a broad measure toward algorithm literacy, Hargittai et al. (Citation2020) relied on an interview protocol investigating internet users’ awareness of curation and personalization in different internet services (e.g., search engines, news sites, social media, and so on) and their knowledge about the type of data being used by these algorithmic systems. Adopting a similar approach, yet using standardized measures, Latzer et al. (Citation2020) investigated Swiss internet users’ algorithm awareness and perceptions using self-created item lists asking participants about their awareness of algorithms, their understanding of the term algorithm, and their knowledge about the risks associated with algorithmic selection. While claiming to study algorithmic knowledge, Cotter and Reisdorf (Citation2020) employed a self-developed scale consisting of six items to determine internet users’ knowledge about the personalization of search engine outputs. Investigating Norwegian internet users’ awareness and attitudes toward algorithm-driven functions, Gran et al. (Citation2021) relied on a single-item measure asking respondents to indicate their perceived level of awareness of “algorithms being used to present recommendations, advertisements and other content on the Internet” (p. 5). In a more recent study exploring internet users’ awareness of algorithmic content recommendations, Zarouali et al. (Citation2021) developed a scale to measure users’ awareness of content filtering based on four dimensions: (1) content filtering, (2) automated decision-making, (3) human-algorithm interplay, and (4) ethical considerations of algorithms (Algorithmic Media Content Awareness Scale, AMCA-scale). Each dimension consisted of three to four items, validated through confirmatory factor analysis. The scale was then tested for three different media platforms (Netflix, Facebook, and YouTube).
While these existing attempts to measure internet users’ algorithm literacy provide a viable starting ground, they come with some critical flaws. First, from a methodological point of view, capturing internet users’ algorithm literacy using a qualitative approach has some advantages as it not only allows for gathering a more in-depth examination of their understanding and skills when interacting with algorithm systems but further allows researchers to connect to users’ experiences and individual levels of knowledge. For instance, lay users are not necessarily familiar with the term “algorithm” itself or with abstract descriptions of algorithmic functions (e.g., filtering, sorting, personalization), which makes it more convenient for them to recount their concrete experiences (i.e., Hargittai et al., Citation2020). Yet such an in-depth approach necessarily comes at the cost of a lack of generalizability and does not allow for studying algorithm literacy on a large scale.
Second, previous attempts to conceptualize algorithm literacy were limited in scope as they focused on users’ knowledge about one specific application (search engines) and took only one type of algorithmic operation (personalization of output) into account (Cotter & Reisdorf, Citation2020). Such an approach is not suitable for representing the different areas and functions of algorithms. Third, instruments to address algorithm literacy were restricted to using single item measures to assess users’ self-reported level of awareness of algorithms employed online (Gran et al., Citation2021) or were rather generic as they used unvalidated lists of items (Latzer et al., Citation2020). With the AMCA scale, Zarouali et al. (Citation2021) provide a first validated measure. Yet, the authors focus on a specific area of algorithmic curation (content recommendations), which makes it difficult to assess algorithm literacy from a more general point of view.
Our study extends these previous efforts by introducing a validated measure that captures internet users’ knowledge about algorithms and awareness of algorithm use as crucial components of algorithm literacy from a generalized perspective. Consequently, our scale is applicable to a broad range of contexts and not limited to any specific application. The scale can be used to study antecedents and implications of individual algorithm literacy, but it also allows for the studying of the role of algorithm literacy in shaping how algorithmic decision-making and content curation affect user behavior and attitudes. Finally, we explicitly developed the scale using item-response theory, which results – in contrast to classical test theory-based scale developments – in a final measure that allows researchers to assess literacy independent of the specifically studied population. Such a scale is more appropriate to use to assess the prevalence of knowledge about algorithms within different user populations.
Defining algorithm literacy
From a theoretical point of view, previous research reveals some inconsistencies in the conceptualization of algorithm literacy (e.g., Hargittai et al., Citation2020; Hobbs, Citation2020). Our literature review showed that most studies have either focused on awareness of algorithm use or knowledge about algorithms, but have not elaborated on how they relate or constitute algorithm literacy as a presumptively broader concept. To define algorithm literacy, the first step is to carve out the breadth of the relevant concept (Carpenter, Citation2018). Therefore, we relied on the existing concepts of algorithm literacy outlined above as well as our findings from an independently conducted pre-study (Dogruel, Citation2021). In this study, we carried out a comprehensive literature review of concepts in media literacy research (e.g., Potter, Citation2010) as well as neighboring areas, such as digital/code literacy (Claes & Philippette, Citation2020; Livingstone et al., Citation2020; Pangrazio & Selwyn, Citation2019), new media literacy (e.g., Chen et al., Citation2011), and privacy literacy (e.g., Masur, Citation2018). We further collected data on how people perceive algorithms in a qualitative interview study of 30 internet users.
Our literature review resulted in four theory-driven dimensions that were deemed relevant for algorithm literacy, including (1) awareness and knowledge, (2) critical evaluation, (3) coping strategies, and (4) creation and design skills. Algorithm literacy can thus be defined as being aware of the use of algorithms in online applications, platforms, and services, knowing how algorithms work, being able to critically evaluate algorithmic decision-making as well as having the skills to cope with or even influence algorithmic operations. The combination of these cognitive and behavioral dimensions thus allows internet users to understand, evaluate, and cope with algorithms in a self-determined way. Practically speaking, this means that individuals are able to apply strategies that allow them to modify predefined settings in algorithmically curated environments, such as in their social media newsfeeds or search engines, to change algorithms’ outputs, compare the results of different algorithmic decisions, and protect their privacy.
We found that the derived dimensions of algorithm literacy are interrelated and, to some extent, have a procedural character that has been outlined in other domains of digital literacy as well (e.g., Masur, Citation2020; Pangrazio & Selwyn, Citation2019). In this regard, users’ awareness and knowledge of how algorithms operate is a crucial prerequisite for subsequently developing critical evaluation and coping skills (cf. Claes & Philippette, Citation2020; Hobbs, Citation2020).
Based on our qualitative study (Dogruel, Citation2021), we found that users’ awareness about the implementation of algorithms in different areas of internet use and their knowledge regarding the operation of algorithms are distinct from, yet related to subdimensions of users’ cognitive knowledge of algorithms. This mirrors literacy approaches in neighboring domains (e.g., data literacy) that distinguish the identification of data (i.e. awareness) from the understanding of data (i.e. knowledge; Pangrazio & Selwyn, Citation2019). Being aware that data is extracted from users, potentially without their knowledge, should be distinguished from users’ understanding of how and where these data were generated and the ways in which they are likely to be processed.
Although algorithm literacy is clearly a multidimensional construct that comprises cognitive, behavioral, and potentially affective dimensions, we focus primarily on the two cognitive dimensions – awareness and knowledge – in this study. This focus is motivated by two reasons. First, it is widely acknowledged among researchers studying users’ perceptions and interactions with algorithmic systems that awareness of algorithm use and knowledge about algorithms are considered meta-skills and preconditions for gaining other additional skills (Cotter & Reisdorf; Eslami et al., Citation2015; Gran et al., Citation2021; Hobbs, Citation2020; Klawitter & Hargittai, Citation2018; Powers, Citation2017; Schwartz & Mahnke, Citation2020). Second, it is considered insufficient to rely on standardized measures to address behaviors and context-sensitive understanding of algorithms (Cotter, Citation2020). Therefore, we focused on the more measurable, cognitive dimensions of algorithm literacy to develop a standardized and more general measure (cf. also Trepte et al., Citation2015, who developed a privacy literacy scale in a similar way). In the following, we describe both dimensions in more detail.
Dimension 1: Awareness of the use of algorithms
Awareness of algorithm use aims to capture the extent to which people are aware that algorithms are used in online applications (e.g., Eslami et al., Citation2015; Hamilton et al., Citation2014). Comparable to approaches in previous studies, this dimension of algorithmic awareness reflects the degree to which users know a) what algorithms can be used for and b) in what online contexts algorithms are actually used. Practically speaking, this dimension addresses to what extent internet users are aware of the areas in which algorithms are used (e.g., search engines, social network sites, personalized news, targeted advertising) and in which particular applications or devices (e.g., smartphones, internet browsers, social media, online banking platforms) algorithms are implemented. A user may be aware that algorithms exist and hence know where they are used and implemented without actually understanding what an algorithm is or does. Awareness of algorithm use is hence better understood as the knowledge that algorithms exist and are used rather than the knowledge of how they work.
Dimension 2: Knowledge about algorithms
Knowledge about algorithms, in contrast, addresses users’ advanced understanding of how algorithmic systems actually work (Dogruel, Citation2021; Cotter & Reisdorf, Citation2020). While it remains a challenge to know the degree to which algorithms are based on their own constantly evolving nature or to understand their context-dependency (Cotter, Citation2020), we argue that knowledge about algorithmic decision-making can be verified against objective details shared about prominent algorithmic operations across different areas of application – similar to Cotter and Reisdorf (Citation2020) focused on search engines. Knowledge about algorithms can be defined as internet users’ understanding a) that information is automatically personalized and customized based on information the system collects and processes (Hargittai et al., Citation2020), b) what type of information algorithms, in general, process, and c) how this impacts the content they encounter online (e.g., the customization and prioritizing of output based on prior behaviors). Here, algorithms’ filtering functions related to the prioritization of information and personalization of content were identified as relevant dimensions of user insights into algorithmic operations (e.g., Claes & Philippette, Citation2020; Cotter & Reisdorf, Citation2020; Hobbs, Citation2020; Powers, Citation2017). In addition to acknowledging the mechanisms of algorithms that center around the collection and processing of personal and use-related information, the interplay between users and algorithms needs to be addressed (Zarouali et al., Citation2021).
Predictors and outcomes of algorithm literacy
Although research on algorithm literacy is still in its infancy, we believe that we can make reasonable assumptions about potential correlates, predictors, and outcomes of algorithm literacy that will help to assert its validity. In general, knowledge and awareness of algorithms can be understood as cognitive concepts. As such, they may serve as types of defense mechanisms against potential media effects. In other words, users with higher algorithm literacy may be able to protect themselves against the potentially negative effects of algorithmic curation. Similar to related research that has investigated online privacy literacy (Masur, Citation2020; Park, 2013) and advertising literacy (Rozendaal et al., Citation2011), we see algorithm literacy as a potential buffering factor against the risks encountered in online environments.
Based on research from related fields (Trepte et al., Citation2015), we know that literacy is not independent from other cognitive, socio-demographic, or even motivational factors. For instance, so-called power users (e.g., individuals with media use of long duration, high frequency, and extensive experience as well as potentially highly developed skills in related areas such as programming, content creation, and online interactions) are likely to have developed skills to use algorithms to their benefit (Bishop, Citation2019; Cotter, Citation2019). Furthermore, we expect that users with a high level of algorithm literacy also think more about the use and application of algorithms. Specifically, a higher awareness of the dangers and risks of algorithmic curation should lead to reflections about the benefits and drawbacks of algorithms in general, as well as to reflections about one’s own behavior and how one might protect oneself against such risks.
Other types of literacy, such as online privacy literacy, are not independent from socio-demographic factors either, underlining the fact that people with a higher educational background are more likely to score better in comparable literacy tests (Trepte et al., Citation2015) and that, at least for computer and ICT literacy, younger participants score better than older ones (Cotter & Reisdorf, Citation2020). As a consequence, algorithm literacy should likewise be positively related to education, but potentially be negatively related to age. More specifically, we expect that younger and better-educated users as well as potential power users should score higher in our scale compared to older, less educated users and those without any further computer skills. To test convergent validity, we hence investigated the relationship between both dimensions of algorithm literacy and a) reflecting on algorithms, b) coding skills, c) frequency of internet use.
Finally, our approach differs from existing approaches in that it focuses on general algorithm literacy and does not focus on specific tasks and applications. Such a generalized measure should necessarily predict algorithm-related behavior in more specific scenarios. For example, people scoring high on algorithm literacy should be able to identify what other videos will be most likely recommended after a specific YouTube video. Similarly, they should be able to demonstrate how to prevent algorithm curation through specific browser settings (e.g., deleting the cache). To test criterion validity, we hence investigated whether higher algorithm awareness and knowledge predicted reasonable decision-making in such more task-oriented, specific scenarios.
Method
Scale development procedure
As a first step, we created a comprehensive item pool based on the extensive literature review and the previously discussed results of the independently conducted pre-study (Dogruel, Citation2021). This pool consisted of 46 items (see Appendix for the item formulations). We then carried out a two-step validation process that is commonly used in scale development: First, we tested the suitability of individual items by evaluating their difficulty and item-specific properties. For this purpose, we conducted an online survey with N = 331 German internet users (Study 1). After eliminating several redundant or less-fitting items using item response theory (IRT), we tested the reduced item pool (32 items in total, comprised of 14 items measuring awareness of algorithm use and 18 items measuring knowledge about algorithms) in a second study with N = 1,041 German internet users (Study 2). Based on this representative sample, we reevaluated and adjusted our item pool and validated the assumed two-dimensional structure using IRT, resulting in two 11-item subscales. In a last step, we correlated both scales with relevant concepts to test the convergent and criterion validity of both dimensions. All data is available from the corresponding author on request.
Measurement theory
Our goal was first to develop an algorithm literacy scale that objectively measures general awareness and knowledge about algorithms. We further aimed to develop a scale that can be used to assess literacy in a simple and efficient way. To address these challenges, we used item response theory (IRT; Bond & Fox, Citation2007; DeMars, Citation2010; Embretson & Reise, Citation2000) in the scale development process, which has several advantages over classical test theory (CTT). First, IRT models are explicitly designed to appropriately score individuals based on a test (e.g., a survey that tests a person’s knowledge). Therefore, an IRT score is often labeled as ability, trait, or proficiency (DeMars, Citation2010) that is commonly designated by the Greek letter theta (θ). Although IRT models can also be used with Likert-type scales, for example, they are particularly suited to estimating θ from dichotomous items (e.g., 0 = false, 1 = true) as the probability of a correct response is expressed as a function of θ. Second, IRT is particularly useful for test and scale development as it provides indices of item difficulty and item discrimination. Item difficulty denotes the proficiency at which 50% of the participant sample is expected to answer an item correctly (DeMars, Citation2010). Knowing items’ difficulties is useful in matching the test and trait levels of a target population and in ensuring that the entire range of the trait of interest is covered. Item discrimination refers to an item’s ability to differentiate between individuals with different levels of proficiency. In IRT models, it is represented by the slope (see also , left) as it indicates how steeply the probability of a correct response changes as the proficiency increases. A high discrimination in all items is desirable as it ensures that subjects are indeed scored based on their latent ability. Third, and in contrast to classical test theory (CTT), IRT models generally also allow differentiation of individuals at the extremes (i.e., at very low or very high literacy levels). More specifically, they scale with a broad difficulty distribution, which is often problematic in CTT (e.g., Bond & Fox, Citation2007).
Figure 1. Item Characteristics Curves (ICC, left) and Person-Item-Maps (PIM, right) for both dimensions of algorithm literacy (Study 2). ICCs visualize the probability of a correct response as a function of a person’s latent ability and the item’s difficulty and discrimination. The point of inflexion denotes the level of proficiency in the population at which the probability of solving the item is 50%. The slopes’ steepness represents the item discrimination (in Rasch models constrained across all items). The PIM plot ranks item difficulties (black dots) aligned with the actual proficiency distribution in the studied population. The slight S-curve indicates that the scales include more items at moderate difficulty levels and less items at lower or higher difficulty levels and thus adequately covers the proficiency range in the studied population
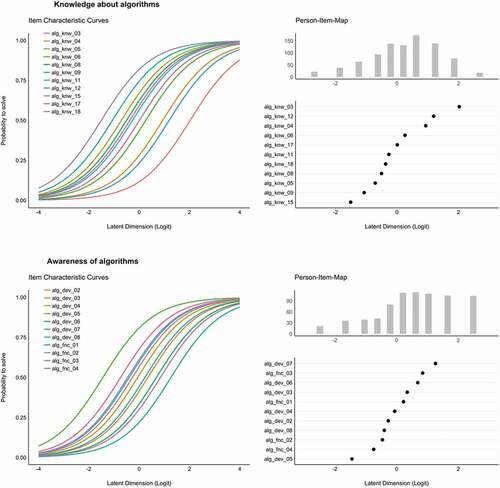
Although all IRT models (3PL, 2PL, and 1PL/Rasch model) are appropriate for capturing knowledge or literacy based on dichotomous items, we explicitly aimed to develop a scale that represents a so-called Rasch or 1PL model (Bond & Fox, Citation2007). In the special case of an IRT model, the relationship between individual proficiency and the probability of a correct response is mathematically formulated as follows (for detail see DeMars, Citation2010, pp.9–17):
Here, the natural logarithm of the proportion between the probability (pki) that a person (k) correctly answers an item i and the probability (1–pki) of not answering this item correctly is defined as the differential between the proficiency (e.g., knowledge) of a person (θk) and the item’s difficulty (δi). Using such a model thus allows us to estimate both the person’s algorithm literacy (the latent ability of the person) and the latent difficulty of each item and allows us to calculate the probability with which a person of a certain latent ability can solve a particular item.
A Rasch model is particularly suitable for our goals because of its specific objectivity (also known as “parameter invariance”; see DeMars, Citation2010): In a Rasch model, the estimation of a subject’s knowledge is independent of the used items and the item difficulties, in turn, are independent of the studied person. From this it follows that one can estimate comparable scores for each person even if different items are used and vice versa; one can estimate comparable item difficulties despite different populations. This also means that new items may easily be added to the scale, which is a considerable advantage given the changing nature of algorithmic processes.
Finally, a Rasch model assumes that all items have the same item discrimination quality (DeMars, Citation2010). From this, it follows that a well-fitting Rasch model has a high usability as the simple sum score of correct responses already adequately reflects the latent ability. In the following analyses, we hence estimated Rasch models for both awareness of algorithm use and knowledge about algorithms. We reduced the scales by focusing on item difficulty as well as maximizing both item and person fit indices. In contrast to CTT, IRT typically focuses on the fit of individual items and not the overall fit across all items. In line with methods from the extended literature on Rasch models, we calculated the difference between the observed response and the model-predicted response (standardized by dividing by its standard deviation). The squared standardized residual was then averaged over participants to assess item fit and averaged over items to assess person fit (Wright & Masters, Citation1982).
Study 1: Testing the comprehensive item pool
Participants and procedure
To evaluate the 46-item pool, we conducted an online survey with N = 331 German internet users in July 2019. We recruited participants using a commercial online access panel. Sampling relied on quotas for age, gender and education level based on German population data. In the final sample, the average age was M = 44.8 years (SD = 14.3, range = 18–70) and 49.8% of the participants were female. Regarding education, 35.6% had university entry level education, 38.7% had completed 10th grade education and 25.7% had no or no more than 9 years of formal education. On average, participants used the internet for M = 3.44 hours a day (SD = 1.39).
Measures
The algorithm literacy item pool consisted of 20 items focusing on awareness of algorithm use and 26 items focusing on knowledge about algorithms. All items were formulated as either multiple choice or true-/false-statements to test user knowledge objectively.
Items measuring awareness of algorithm use asked a) for which purposes (e.g., weather forecast, product recommendations, etc.) algorithms are used and b) what (media) products actually use algorithms to function properly (e.g., smart speakers, smart TV, certain health device, etc.). We deliberately included areas and applications that do not use algorithms (e.g., printed magazines or film production) to test whether individuals correctly labeled these domains as not related to algorithms.
Items measuring knowledge of algorithms tested participants’ understanding of how algorithms work and what implications they can have for users. We hence created a list of 26 items that required users to indicate a) whether certain results of algorithmic decision-making are plausible or not, b) whether the implementation of algorithms can have unwanted consequences, c) their understanding of how algorithms work (see items list in Appendix).
Results
The difficulty of the 20 items measuring awareness of algorithm use ranged from PFootnote1 = .25 to P = .97 (M = 0.66, SD = 0.22). In the first step, we excluded one item due to its very low difficulty: 97% of the participants correctly indicated that algorithms are not using “our dreams” as a data basis. As such an item does not discriminate between people (not even very non-literate people), it does not contribute to measuring algorithm literacy. We then proceeded to fit a Rasch model on the remaining 19 items. Inspection of the infit and outfit values for each item indicated that two items had particularly high outfit value (MSQ Outfit > 1.5), which indicate a potential distortion or degradation to the measurement system (whether algorithms use “radios” as a data basis and whether algorithms are used to write “journalistic reports”). We further excluded three items that had the same item difficulties as other, more fitting items. In a Rasch model, which assumes equal item discrimination, such items do not contribute to a better measurement of the latent ability as they essentially differentiate people at the same level of proficiency. The improved Rasch model indicated that another two items had a low item fit. Excluding both resulted in a satisfactory model with MSQ Infit and Outfit values between 0.68 and 1.28 (according to Linacre, Citation2002, p. 878, items productive for measurement should have mean-square values between 0.5 and 1.5). The two items that were excluded in the last step (i.e., whether algorithms used “smartwatches” and “printed newspapers” as data sources) both had a comparatively low difficulty. As we would otherwise reduce the ability to differentiate people with low awareness of algorithm use we decided to provisionally keep them in the scale despite their low item fit. In the end, we thus kept 14 items measuring awareness of algorithm use (see Appendix).
The difficulty of the 26 items measuring knowledge about algorithms ranged from P = .27 to P = .94 (M = 0.62, SD = 0.18). We again excluded one item due to its very low difficulty (“It is always indicated when an algorithm is used”) and thus low contribution to the estimation of the latent ability. Another item (“It is always people who start or stop algorithms”) was excluded because it had similar item properties (particularly item difficulty) as other items. For study 1, some items were measured with an option to indicate “I don’t know” while others were not (see Appendix). As we focused on knowledge, “I don’t know” options, albeit often criticized in survey designs (Beatty et al., Citation1998; Coombs & Coombs, Citation1976), make sense as they are not a sign of non-response or non-opinion, but a viable option – as the lack of such an option requires users to guess a solution. For study 1, which had included some items without “I don’t know” options, we hence had to estimate two separate Rasch models to account for items with and without “I don’t know” options. Yet, in both models the items showed good item fit indices (MSQ Infit and Outfit). To further limit the likelihood of guessing, we decided for study 2 to change all items to include a “I don’t know” category and to reduce the length of the scale, we also excluded redundant items (based on item difficulty). In the end, we kept a total of 18 items for the knowledge about algorithms scale.
Study 2: Validating the algorithm literacy scale
Participants and procedure
We conducted a second online survey in October 2019 with N = 1,041 German internet users. We again used the same commercial online access panel to recruit our participants and again our sampling relied on quotas for age, gender, and education level based on German population data. Participants in the resulting sample were on average M = 44.8 years old (SD = 14.5; range = 17–69). Roughly half of the participants (50.3%) were female. Again, roughly a third of the population (36.2%) had a high educational level (university entry level or above), 33.6% had completed 10th grade or equivalent and 30.2% had received 9th grade formal education. Participants indicated high levels of internet use. More than two thirds of the population (67.1%) used the internet more than 2 hours a day and 13% even used the internet more than 4 hours per day.
Measures
Algorithm literacy
In this second study, we tested the reduced item pool (overall 32 items, 14 items measuring algorithm awareness, 18 items measuring knowledge about algorithms; see Table A1) identified in Study 1. In contrast to study 1 and to reduce guessing in all items, we added an option to indicate “I don’t know” to all items.
Algorithm reflection
To study the convergent validity of our scale, we further assessed how often participants reflect about algorithms and their implications. Participants rated four items (e.g., “I have often thought about how algorithms influence my internet use”) on a 5-point scale ranging from 1 = totally disagree to 5 = fully agree. The initial uni-dimensional model revealed a slight misfit (□□(2) = 25.60, p < .001; CFI = .99, TLI = .96, RMSEA = .11, SRMR = .02). Despite high factor loadings of all four items, modification indices indicated problems with item 3 (“I often thought about how good, or bad Google’s search results are”). Excluding this item resulted in perfect model fit – even if all factor-loadings were constrained to be equal (i.e., tau-equivalency; □ (2) = 1.49, p = .475; CFI = 1.00, TLI = 1.00, RMSEA = 0.00, SRMR = .02). The three-item scale showed good composite reliability (McDonald’s ω = .84, Cronbach’s α = .84, AVE = .64).
Subjective coding skills
We further assessed coding skills with a single item (“How good is your programming knowledge?”). Participants indicated their knowledge on a 5-point scale ranging from 1 = none at all to 5 = very good programming knowledge. On average, the subjective coding skill was rather low (M = 1.78, SD = 1.05).
Procedural skills/scenarios
To investigate how far our newly developed scale correlated with other, more procedural, task-oriented demonstrations of algorithm literacy, we developed three scenarios in which participants engaged in algorithm-specific decision-making. In contrast to our general scale, these scenarios focused on concrete examples of algorithms in use.
In scenario 1, participants were confronted with six pictures of potential areas of application for algorithmic curation. They had to click on these pictures indicating an application of algorithms. Pictures were a search engine result, a GPS navigation, a weather forecast, and a MS Word auto correction form (all true) as well as a remote control and a journalist interviewing another person (both false).
In Scenario 2, participants were confronted with a screenshot of a YouTube video, showing a music video from a popular German female folk singer. Participants were informed that a female YouTube user had activated the auto-play mode and we asked participants to select the video they thought would most likely follow the first video. Participants had to choose between two videos or indicate they did not know (rated as false). This choice task was repeated three times. One choice was between an ad for a female (true) or male (false) perfume. The second choice was between another video by the same singer (true) and a popular male US rapper (false). The third choice was between two videos on natural make up, one by an influencer with high numbers of views (true) and one with only a few views (false).
Scenario 3 presented a (male) online shopper, who had bought a trekking backpack on an online platform and was consequently confronted with online advertisements of trekking gear on the websites he used. He wondered how he could get rid of these ads and we gave users five potential solutions. Three of those were useful actions, namely deleting cookies, deleting browser history and actively searching for other products. Two were generally not useful, namely to restart the computer and to close and re-open the web browser.
Results
Construct validity
In the first step, we again inspected the item difficulties. The 14 items measuring awareness of algorithm use showed difficulties ranging from P = .38 to P = .74 (M = 0.56, SD = 0.11). We decided to exclude the two items that we kept in the scale despite low item infit values in study 1 because they had similar item difficulties to two other items. We further excluded one item (“Smartphones”) because it was again redundant to another item with regard to item difficulty (see Appendix). We fitted a Rasch model with the remaining 11 items (see , lower panel). All items showed satisfactory MSQ Infit and Outfit values (Infit: 0.84 to 1.08; Outfit: 0.75 to 1.17). Similarly, only a few people had low person fit (z-Infit > 1.96: 1.4%; z-Outfit > 1.96: 1.8%). As (bottom) shows, the items appropriately cover the range of algorithm awareness in the population with IRT item difficulty parameters ranging from −1.44 to 1.26. On average, the resulting Rasch score was M = 0.33 (SD = 2.10, range = – 3.44 to 3.43), which is equivalent to answering 6.21 of 11 questions correctly (see ). As the scale’s midpoint is fixed onto the average item difficulty, the slight positive shift in the Rasch score indicates that the items were slightly too easy. In the future, easier items could hence be exchanged with more very difficult ones (see also difficulty distribution in ).
Table 1. Convergent validity of algorithm literacy: zero-order correlations
The 18 items measuring knowledge about algorithms showed difficulties ranging from P = .13 to P = .71 (M = 0.48, SD = 0.16). With the goal of further reducing the scale length, we excluded three items with redundant item difficulty parameters to other, more fitting items (see Appendix). We then fitted a Rasch model on the remaining 15 items. Despite overall good item and person fit indices, we excluded another 4 items with lower infit and outfit indices or because they again had similar item difficulties to other, better fitting items. The remaining 11 items were then used to fit the final Rasch model, which revealed appropriate MSQ Infit and Outfit values (Infit: 0.85 to 1.07; Outfit: 0.79 to 1.38) as well as an acceptable number of participants with a low person fit (z-Infit > 1.96: 2.2%; z-Outfit > 1.96: 4.7%). As shown in (top), the identified items adequately vary in their item difficulty and thus capture the variance in algorithm knowledge in the population (particularly as more items fall into the mid-range where most of the population is located). On average, the resulting Rasch score was M = – 0.32 (SD = 1.65, range = – 3.57 to 3.70), which is equivalent to answering 5.14 of 11 questions correctly (see ). The slight negative shift indicates that the items were slightly too difficult. In the future, very difficult items could hence be exchanged for very easy items (see difficulty distribution in ).
Reliability
In the IRT, the concept of reliability is fundamentally different than in CTT. That said, we can calculate the proportion of person variance that is not due to error. Such a concept is very similar to reliability estimates such as Cronbach’s alpha (Wright & Stone, Citation1999). The so-called person separation reliability for the dimension awareness of algorithm use was .86 and for knowledge about algorithms .80. As expected, both dimensions correlated significantly with each other (r = .70, p < .001).
Convergent and criterion validity
We expected that awareness and knowledge were higher for what power users, that is users that a) reflect more about algorithms, b) that use the internet more and c) that have some additional coding skills. Bivariate correlations of these three measures and the two dimensions of the algorithm scale are presented in . As expected, reflecting about algorithms correlated moderately positively with both awareness (r = .41, p < .001) as well as knowledge (r = .36, p < .001). Regarding internet use (awareness: r = .09, p = .005; knowledge: r = .09, p = .003) as well as coding skills (awareness: r = .12, p < .001; knowledge: r = .19, p < .001), the correlation was weaker but still significant and positive – therefore indicating that power users indeed had a higher algorithm literacy.
shows whether the level of algorithm literacy differs between males and females, various age groups, and different educational levels. First, age was significantly and negatively related to both dimensions of algorithm literacy (awareness: r = – .15, p < .001; knowledge: r = – .18, p < .001). This runs into the assumed direction of young internet users being more literate than older ones. As further proof that our measure was valid, we also found a significant positive correlation of moderate size between both dimensions of the algorithm literacy scale and participants’ education level (awareness: Spearman’s rho = .33, p < .001; knowledge: rho = .34, p < .001).Footnote2
Table 2. Distribution of algorithm literacy in different subpopulations (Study 2)
To test the criterion validity, we investigated whether our two scale dimensions could predict actions in procedural scenarios. We estimated independent logistic regression models to predict correct assessments in each scenario using either the Rasch scores of the awareness or the knowledge subscale. The results for all models are presented in . For scenario 1, in which we presented pictures of areas of application for algorithms, we generally found both awareness and knowledge to significantly predict a correct answer for our four correct answers. For the two wrong incidents – the remote control and the journalistic interview – with one exception awareness and knowledge did not predict a correct answer. For remote control – coded as false – we even saw a slight but significant tendency to predict the wrong answer, yet we have to keep in mind that although remote controls do not rely on algorithms, current SmartTVs and their ability to recommend content do. The picture was hence potentially misleading participants may have thought about SmartTVs instead of the remote control itself. One should bear this in mind when interpreting this somewhat counterintuitive.
Table 3. Criterion validity of algorithm literacy: logistic regression models predicting correct decision-making in each scenario
For the more complex second scenario, the prediction of the correct video to follow for a female YouTube user, awareness and knowledge were significant predictors for a correct answer. Interestingly, knowledge about algorithms was a slightly better predictor compared to awareness of algorithm use. In scenario 3, we asked participants to indicate which strategies could help to get rid of targeted advertisement after an online purchase. Both awareness and knowledge about algorithms significantly predicted choosing the correct answers – independently of whether the correct answer was indicating that an action helps (e.g., deleting the browser history) or indicating that an action (e.g., restarting the computer) does not help to get rid of the ads.
All in all, our scale showed the expected relationships with our criterion variables and proved to be a significant predictor for all our test scenarios, thus showing good convergent and criterion validity.
Discussion
By acknowledging that algorithms govern our everyday internet experiences, we also take responsibility for coping with the challenges of algorithmic curation. Being able to at least basically understand how such algorithms work and where they are encountered is very likely to impact users’ potential to successfully use the internet for their own benefit, which is the goal of digital media literacy (Claes & Philippette, Citation2020; Livingstone et al., Citation2020; Pangrazio & Selwyn, Citation2019). If algorithm literacy is important, a valid instrument that can measure it empirically is required, and as of now current approaches suffer major weaknesses that the presented scale here set out to overcome. To our knowledge, this is one of the first attempts to systematically develop and validate an algorithm literacy scale. We did so by identifying awareness of algorithm use and knowledge about algorithms as the two crucial components of internet users’ algorithm literacy. The awareness of algorithm use and knowledge about algorithms scale enables a standardization of the measurement of algorithm literacy and facilitates the generalization of findings in the long run.
Users’ awareness about the operation of algorithms and a basic knowledge of their functioning are central prerequisites for the development of critical reflection and of coping skills required in dealing with these systems. Being aware of and knowing how algorithms shape our online experiences can be considered a crucial determinant of potential digital inequalities (Lutz, Citation2019). That said, our own research (Dogruel, Citation2021) suggests that algorithm literacy as a broader concept encompasses further dimensions, such as critical evaluation, coping strategies, and creation and design skills, that a more comprehensive measure could address. Yet such dimensions are likely to require a substantially different approach in item generation, relying more on attitudinal measures or even approaches that focus on concrete behaviors, such as problem solving or design choices. Therefore, knowledge about algorithms and awareness of algorithm use as presented provide a crucial starting point for a better understanding of the concept of algorithm literacy in general.
The scale was developed in a multi-step process through item generation and confirmation using IRT. We calculated a Rasch model that allows us to consider an individual’s abilities and item difficulty. This made it possible to determine the best item fit and present a final scale that can be considered an economical, reliable, and robust self-report measure to assess internet users’ awareness and knowledge of algorithms. To determine the convergent and criterion validity of the scale, we demonstrated expected positive relationships with related measures of algorithm internet skills. Awareness and knowledge were positively related to individuals’ coding skills, their reflections about algorithms in general, and most importantly, acted as predictors for correct answers in natural scenarios of algorithmic curation.
Yet, some methodological limitations have to be taken into account. Natural scenarios had not been tested extensively and we also had to rely on rather condest, even one-item measures for validity tests. Future research should use more elaborate validity measures. Furthermore, our original scale was developed in German and the appendix presents the original version as well as an English translation. Items worked in the context of our study, but item length or use of words to increase or decrease item difficulty might be critical in other languages.
The implications of our scale development have to be defended in light of the dynamic nature of algorithms as socio-technical systems and the ongoing societal debate around the governing of algorithmic curation (Ananny & Crawford, Citation2018; Katzenbach & Ulbricht, Citation2019). From a technological perspective, algorithms are subject to evolvement over time and their mutual shaping with user interactions make them difficult to approach using predefined assumptions about factual knowledge (Cotter, Citation2020). Further successful changes in policy measures, such as implementing transparency and explainability to algorithmic systems, might impact users’ awareness of algorithm use in such a manner that adjustments to the scale might be necessary for future applications. Thus, we must acknowledge that item generation was necessarily influenced by the current status quo: how algorithms are employed in popular online use situations and which functions they serve, which is bound to evolve. Future technological development and social uses of algorithms will emerge, and the instrument is, to some extent, time sensitive. Yet, such a critique could be raised against all standardized measures focusing on users’ behaviors and perceptions regarding new media technology, and the only alternatives are either to provide measures that are so broad that they are no long time sensitive but considerably lack specificity, or to quit the task of developing a valid measure altogether. In our case, we aimed to mitigate the problem of time sensitivity by developing items that refer to different use contexts (instead of specific media platforms or applications). Still, scholars who employ our scale are advised to consider whether the items need to be adjusted to reflect the current status quo. Whereas we are positive that our subscale knowledge about algorithms addresses core functions of algorithms, which are likely to be rather stable over time, the items measuring awareness of algorithm use are more time sensitive and somewhat failed to account for participants who were very aware about the use and application of algorithms.
As a consequence, future research is invited to employ (and in the long run, adjust) our scale to determine internet users’ literacy as an outcome variable, e.g., to compare internet users’ literacy across different populations, cultural context, or over time. Based on our study, this is still an area that requires improvement as our validation study was based on a quota sample. As such, while it cannot be regarded as a truly representative sample of (German) internet users, it is albeit a rather good approximation. Still, we are hesitant to provide norm values for the proposed scale. Thus, future research is invited to provide such norm values based on representative population samples not only within the German context. As a next step, research focusing on literacy interventions to strengthen users’ competences regarding the operation of algorithms online may use this instrument to assess intervention measures, such as providing information or the training of end users. We are confident that our scale will provide scholars inquiring into users’ perceptions and methods of coping with algorithmic curation in different contexts with the opportunity to systematically address users’ literacy regarding various research questions in the domain of computer-mediated communication using algorithm literacy both as an outcome measure as well as an antecedent of online behavior and use.
Supplemental Material
Download MS Word (143.6 KB)Supplemental data
Supplemental data for this article can be accessed on the publisher’s website.
Disclosure statement
No potential conflict of interest was reported by the authors.
Additional information
Notes on contributors
Leyla Dogruel
Leyla Dogruel (Ph.D., Freie Universität Berlin, 2013) is Assistant Professor at the Department of Communication at Johannes Gutenberg-University Mainz, Germany. Her areas of research focus on privacy in digital media, user perceptions and governance of algorithmic curation, media innovation research, and news media markets.
Philipp Masur
Philipp K. Masur(Ph.D., Universität Hohenheim, 2018) is an Assistant Professor for Persuasive Communication in the Department of Communication Science at the Vrije Universiteit Amsterdam. His research focuses on different aspects of computer-mediated communication such as social influence and persuasion processes on social media, privacy and self-disclosure in networked publics, and media and communication effects on individual well-being.
Sven Joeckel
Sven Jöckel(PhD., TU Ilmenau, 2008) is a Professor for Communication with a focus on children, adolescents, and the media at the University of Erfurt. His research interests include digital privacy, the use and effects of digital media, as well as media and morality.
Notes
1 In classical test theory, P denotes the difficulty of a parameter. The estimate denotes the percentage of participants that were able to solve the questions. Thus, a low value denotes a high difficulty (i.e., only few people were able to solve the question) and a high value denotes a low difficulty (i.e., many people were able to solve the question).
2 As a further note, male participants scored slightly higher on the test than females (awareness: t(1157.30) = – 17.52, p < .001; knowledge: t(1229.30) = – 34.06, p < .001).
References
- Ananny, M., & Crawford, K. (2018). Seeing without knowing: Limitations of the transparency ideal and its application to algorithmic accountability. New Media & Society, 20(3), 973–989. https://doi.org/https://doi.org/10.1177/1461444816676645
- Ball, K., & Webster, W. (2020). Big data and surveillance: Hype, commercial logics and new intimate spheres. Big Data & Society, 7(1), 205395172092585. https://doi.org/https://doi.org/10.1177/2053951720925853
- Beatty, P., Herrmann, D., Puskar, C., & Kerwin, J. (1998). “Don’t know” responses in surveys: Is what I know what you want to know and do I want you to know it? Memory (Hove, England), 6(4), 407–426. https://doi.org/https://doi.org/10.1080/741942605
- Bishop, S. (2019). Managing visibility on YouTube through algorithmic gossip. New Media & Society, 21(11-12), 2589–2606. https://doi.org/https://doi.org/10.1177/1461444819854731
- Bond, T. G., & Fox, C. F. (2007). Applying the Rasch model: Fundamental measurement in the human sciences (2nd ed.). Routledge.
- Brodsky, J. E., Zomberg, D., Powers, K. L., & Brooks, P. J. (2020). Assessing and fostering college students’ algorithm awareness across online contexts. Journal of Media Literacy Education, 12(3), 43–57. https://doi.org/https://doi.org/10.23860/JMLE-2020-12-3-5
- Bucher, T. (2012). Want to be on the top? Algorithmic power and the threat of invisibility on Facebook. New Media & Society, 14(7), 1164–1180. https://doi.org/https://doi.org/10.1177/1461444812440159
- Bucher, T. (2017). The algorithmic imaginary: Exploring the ordinary affects of Facebook algorithms. Information, Communication & Society, 20(1), 30–44. https://doi.org/https://doi.org/10.1080/1369118X.2016.1154086
- Burrell, J., Kahn, Z., Jonas, A., & Griffin, D. (2019). When users control the algorithms. In A. Lampinen, D. Gergle, & D. A. Shamma (Eds.), Proceedings of the ACM on human-computer interaction (Vol. 3, pp. 1–20). Association for Computing Machinery Inc. (ACM). https://doi.org/https://doi.org/10.1145/3359240
- Carpenter, S. (2018). Ten steps in scale development and reporting: A guide for researchers. Communication Methods and Measures, 12(1), 25–44. https://doi.org/https://doi.org/10.1080/19312458.2017.1396583
- Chen, D.‑T.,Wu, J., & Wang, Y. (2011). Unpacking new media literacy. Journal of Systemics, Cybernetics and Informatics, 9(2), 84–88. http://www.iiisci.org/journal/sci/FullText.asp?var=&id=OL508KR
- Claes, A., & Philippette, T. (2020). Defining a critical data literacy for recommender systems: A media-grounded approach. Journal of Media Literacy Education, 12(3), 17–29. https://doi.org/https://doi.org/10.23860/JMLE-2020-12-3-3
- Coombs, C. H., Coombs, L. C. (1976). “Don’t know”: Item ambiguity or respondent uncertainty? Public Opinion Quarterly, 40(4), 497. https://doi.org/https://doi.org/10.1086/268336
- Cotter, K. (2019). Playing the visibility game: How digital influencers and algorithms negotiate influence on Instagram. New Media & Society, 21(4), 895–913. https://doi.org/https://doi.org/10.1177/1461444818815684
- Cotter, K., & Reisdorf, B. C. (2020). Algorithmic knowledge gaps: A new dimension of (Digital) inequality. International Journal of Communication (Ijoc), 2020(14), 745–765. https://ijoc.org/index.php/ijoc/article/view/12450
- Cotter, K. M. (2020). Critical algorithmic literacy: Power, epistemology, and platforms [Dissertation]. Michigan State University, Michigan. https://search.proquest.com/openview/3d5766d511ea8a1ffe54c53011acf4f2/1?pq-origsite=gscholar&cbl=18750&diss=y
- DeMars, C. (2010). Item response theory. Oxford University Press.
- Dogruel, L., Facciorusso, D., & Stark, B. (2020). ‘I’m still the master of the machine.’ Internet users’ awareness of algorithmic decision-making and their perception of its effect on their autonomy. Special Issue of Digital Communication Research.Information, Communication & Society. Advance online publication https://doi.org/https://doi.org/10.1080/1369118X.2020.1863999
- Dogruel, L. (2021). What is algorithm literacy? A conceptualization and challenges of its empirical measurement. In C. Schumann & M. Taddicken (Eds.), Algorithms and Communication. Special Issue of Digital Communication Research.
- Embretson, S. E., & Reise, S. P. (2000). Item response theory for psychologists. Lawrence Erlbaum.
- Eslami, M., Rickman, A., Vaccaro, K., Aleyasen, A., Vuong, A., Karahalios, K., Hamilton, K., & Sandvig, C. (2015). I always assumed that I wasn’t really that close to [her]. In B. Begole, J. Kim, K. Inkpen, & W. Woo ( Chairs), The 33rd annual ACM conference, Seoul, Republic of Korea.
- Fletcher, R., & Nielsen, R. K. (2018). Automated serendipity. Digital Journalism, 6(8), 976–989. https://doi.org/https://doi.org/10.1080/21670811.2018.1502045
- Gillespie, T. (2014). Relevance of algorithms. In T. Gillespie, P. J. Boczkowski, & K. A. Foot (Eds.), Inside technology. Media technologies: Essays on communication, materiality, and society (pp. 167–194). The MIT Press.
- Gran, A. ‑. B., Booth, P., & Bucher, T. (2021). To be or not to be algorithm aware: A question of a new digital divide? Information, Communication & Society, 24(12), 1779–1796. https://doi.org/https://doi.org/10.1080/1369118X.2020.1736124
- Hallinan, B., & Striphas, T. (2016). Recommended for you: The Netflix prize and the production of algorithmic culture. New Media & Society, 18(1), 117–137. https://doi.org/https://doi.org/10.1177/1461444814538646
- Hamilton, K., Karahalios, K., Sandvig, C., & Eslami, M. (2014). A path to understanding the effects of algorithm awareness. CHI’14 Extended Abstracts on Human Factors in Computing Systems, 631–642. https://doi.org/https://doi.org/10.1145/2559206.2578883
- Hancock, J. T., Naaman, M., & Levy, K. (2020). AI-mediated communication: Definition, research agenda, and ethical considerations. Journal of Computer-Mediated Communication, 25(1), 89–100. https://doi.org/https://doi.org/10.1093/jcmc/zmz022
- Hargittai, E., Gruber, J., Djukaric, T., Fuchs, J., & Brombach, L. (2020). Black box measures? How to study people’s algorithm skills. Information, Communication & Society, 23(5), 764–775. https://doi.org/https://doi.org/10.1080/1369118X.2020.1713846
- Helberger, N. (2019). On the democratic role of news recommenders. Digital Journalism, 7(8), 993–1012. https://doi.org/https://doi.org/10.1080/21670811.2019.1623700
- Hobbs, R. (2020). Propaganda in an age of algorithmic personalization: Expanding literacy research and practice. Reading Research Quarterly, 55(3), 521–533. https://doi.org/https://doi.org/10.1002/rrq.301
- Katzenbach, C., & Ulbricht, L. (2019). Algorithmic governance. Internet Policy Review, 8(4). https://doi.org/https://doi.org/10.14763/2019.4.1424
- Kemper, J., & Kolkman, D. (2018). Transparent to whom? No algorithmic accountability without a critical audience. Information, Communication & Society, 19(4), 1–16. https://doi.org/https://doi.org/10.1080/1369118X.2018.1477967
- Kitchin, R. (2017). Thinking critically about and researching algorithms. Information, Communication & Society, 20(1), 14–29. https://doi.org/https://doi.org/10.1080/1369118X.2016.1154087
- Klawitter, E., & Hargittai, E. (2018). “It’s like learning a whole other language:” The role of algorithmic skills in the curation of creative goods. International Journal of Communication, 12(2018), 3490–3510. https://ijoc.org/index.php/ijoc/article/view/7864
- Koltay, T. (2011). The media and the literacies: Media literacy, information literacy, digital literacy. Media, Culture & Society, 33(2), 211–221. https://doi.org/https://doi.org/10.1177/0163443710393382
- Latzer, M., Hollnbuchner, K., Just, N., & Saurwein, F. (2016). The economics of algorithmic selection on the internet. In J. M. Bauer & M. Latzer (Eds.), Handbook on the economics of the internet (pp. 395–424). Edward Elgar Publishing.
- Latzer, M., & Festic, N. (2019). A guideline for understanding and measuring algorithmic governance in everyday life. Internet Policy Review, 8(2). https://doi.org/https://doi.org/10.14763/2019.2.1415
- Latzer, M., Festic, N., & Kappeler, K. (2020). Awareness of algorithmic selection and attitudes in Switzerland. Report 2 from the project: The significance of algorithmic selection for everyday life: The case of Switzerland. University of Zurich. http://mediachange.ch/research/algosig
- Leong, L. (2020). Domesticating algorithms: An exploratory study of Facebook users in Myanmar. The Information Society, 36(2), 97–108. https://doi.org/https://doi.org/10.1080/01972243.2019.1709930
- Linacre, J. M. (2002). What do infit and outfit, mean-square and standardized mean? Rasch Measurement Transactions, 16(2), 878. https://www.rasch.org/rmt/rmt162f.htm
- Livingstone, S., Stoilova, M., & Nandagiri, R. (2020). Data and privacy literacy. The role of the school in educating children in a datafied society. In D. Frau‐Meigs, S. Kotilainen, M. Pathak‐Shelat, M. Hoechsmann, & S. R. Poyntz (Eds.), The handbook of media education research (pp. 413–426). Wiley.
- Lutz, C. (2019). Digital inequalities in the age of artificial intelligence and big data. Human Behavior and Emerging Technologies, 1(2), 141–148. https://doi.org/https://doi.org/10.1002/hbe2.140
- Masur, P. K. (2018). Situational privacy and self-disclosure: Communication processes in online environments. Cham, Switzerland: Springer.
- Masur, P. K. (2020). How online privacy literacy supports self-data protection and self-determination in the age of information. Media and Communication, 8(2), 258–269. https://doi.org/https://doi.org/10.17645/mac.v8i2.2855
- Pangrazio, L., & Selwyn, N. (2019). ‘Personal data literacies’: A critical literacies approach to enhancing understandings of personal digital data. New Media & Society, 21(2), 419–437. https://doi.org/https://doi.org/10.1177/1461444818799523
- Potter, W. J. (2010). The state of media literacy. Journal of Broadcasting & Electronic Media, 54(4), 675–696. https://doi.org/https://doi.org/10.1080/08838151.2011.521462
- Potter, W. J., & Thai, C. L. (2019). Reviewing media literacy intervention studies for validity. Review of Communication Research, 7, 38–66. https://doi.org/https://doi.org/10.12840/ISSN.2255-4165.018
- Powers, E. (2017). My news feed is filtered? Digital Journalism, 5(10), 1315–1335. https://doi.org/https://doi.org/10.1080/21670811.2017.1286943
- Prey, R. (2017). Nothing personal: Algorithmic individuation on music streaming platforms. Media, Culture & Society, 10(1), 016344371774514. https://doi.org/https://doi.org/10.1177/0163443717745147
- Rader, E., & Gray, R. (2015). Understanding user beliefs about algorithmic curation in the Facebook news feed. In Begole, J. Kim, K. Inkpen, & W. Woo (Eds.), Proceedings of the 33rd annual ACM conference on human factors in computing systems (pp. 173–182). ACM: New York. https://doi.org/https://doi.org/10.1145/2702123.2702174
- Rozendaal, E., Lapierre, M. A., van Reijmersdal, E. A., & Buijzen, M. (2011). Reconsidering advertising literacy as a defense against advertising effects. Media Psychology, 14(4), 333–354. https://doi.org/https://doi.org/10.1080/15213269.2011
- Schwartz, S. A., & Mahnke, M. S. (2020). Facebook use as a communicative relation: Exploring the relation between Facebook users and the algorithmic news feed. Information, Communication & Society. https://doi.org/https://doi.org/10.1080/1369118X.2020.1718179
- Sharabi, L. L. (2020). Exploring how beliefs about algorithms shape (Offline) success in online dating: A two-wave longitudinal investigation. Communication Research, 009365021989693. https://doi.org/https://doi.org/10.1177/0093650219896936
- Shin, D., & Park, Y. J. (2019). Role of fairness, accountability, and transparency in algorithmic affordance. Computers in Human Behavior, 98(September), 277–284. https://doi.org/https://doi.org/10.1016/j.chb.2019.04.019
- Sundar, S. S. (2020). Rise of machine agency: A framework for studying the psychology of human–AI interaction (HAII). Journal of Computer-Mediated Communication, 25(1), 74–88. https://doi.org/https://doi.org/10.1093/jcmc/zmz026
- Susser, D., Roessler, B., & Nissenbaum, H. (2019). Technology, autonomy, and manipulation. Internet Policy Review, 8(2). https://doi.org/https://doi.org/10.14763/2019.2.1410
- Thorson, K. (2020). Attracting the news: Algorithms, platforms, and reframing incidental exposure. Journalism: Theory, Practice & Criticism, 1067–1082. https://doi.org/https://doi.org/10.1177/1464884920915352
- Trepte, S., Teutsch, D., Masur, P. K., Eicher, C., Fischer, M., Hennhöfer, A., & Lind, F. (2015). Do people know about privacy and data protection strategies? Towards the “online privacy literacy scale” (OPLIS). In S. Gutwirth, R. Leenes, & P. de Hert (Eds.), Law, governance and technology series: Vol. 20. Reforming European data protection law (pp. 333–366). Springer.
- Wright, B. D., & Masters, G. N. (1982). Rating scale analysis. MESA Press.
- Wright, B. D., & Stone, M. H. (1999). Measurement Essentials. Wide Range, Inc.
- Yeung, K. (2018). Algorithmic regulation: A critical interrogation. Regulation & Governance, 12(4), 505–523. https://doi.org/https://doi.org/10.1111/rego.12158
- Zarouali, B., Boerman, S. C., & de Vreese, C. H. (2021). Is this recommended by an algorithm? The development and validation of the algorithmic media content awareness scale (AMCA-scale). Telematics and Informatics, 62. https://doi.org/https://doi.org/10.1016/j.tele.2021.101607