ABSTRACT
Studies assessing the effects of social media use are largely based on measures of time spent on social media. In recent years, scholars increasingly ask for more insights in social media activities and content people engage with. Data Download Packages (DDPs), the archives of social media platforms that each European user has the right to download, provide a new and promising method to collect timestamped and content-based information about social media use. In this paper, we first detail the experiences and insights of a data collection of 110 Instagram DDPs gathered from 102 adolescents. We successively discuss the challenges and opportunities of collecting and analyzing DDPs to help future researchers in their consideration of whether and how to use DDPs. DDPs provide tremendous opportunities to get insight in the frequency, range, and content of social media activities, from browsing to searching and posting. Yet, collecting, processing, and analyzing DDPs is also complex and laborious, and demands numerous procedural and analytical choices and decisions.
How social media use affects people’s cognitions, emotions and behavior is a research question in progress. Thus far, most studies have focused on self-reported time spent on social media platforms as an indicator of social media use. Time-based measures of social media use do not provide insight in what people do or see on social media. Some studies have accounted for this limitation by focusing on self-reported time spent on specific social media activities, such as passive social media use – browsing through content of others – and active use, such as posting and sending messages (Thorisdottir et al., Citation2019; Tosun & Kaşdarma, Citation2020). Yet, the vast variety in conceptualizations and operationalizations of these activities across studies underlines a fundamental need for a more content-based assessment of what people do and see on social media (Valkenburg, van Driel et al., Citation2022).
To address the limitations of current time-based measures of social media use, researchers have been calling for methodologies to enhance our knowledge on the content that social media users share and encounter (Griffioen et al., Citation2020; Odgers & Jensen, Citation2020). In response to these calls, a variety of methodologies to assess content-based social media use have been explored, most of which utilize smartphone devices as measurement tool (Burnell et al., Citation2021; Lind et al., Citation2018; Reeves et al., Citation2021). Although promising and necessary, these methodologies also have their own limitations, such that they yield social media data that are confined to smartphone use.
The current study explores the promises and pitfalls of a new method to capture social media use based on data donations of social media archives: Data Download Packages (DDPs). Since the implementation in Europe of the General Data Protection Regulation in 2018, all platforms that store data of their users are legally mandated to share these data with their users upon their request (Boeschoten et al., Citation2020). Specifically, this paper explores the promises and pitfalls of DDPs using Instagram data donations as an example. Instagram is a suitable platform to explore the potential of DDPs due to its high popularity across all ages, and in particular adolescents, the sample on which the current study is based. It is also a platform with a wide variety of affordances, such as posting, private messaging, and updating stories.
The aim of this paper is twofold. Its first aim is to share the practical experiences of collecting Instagram DDPs and discuss what these DDPs can teach us about participants’ engagement in different Instagram activities. We will describe the steps of the DDP donation process, such as privacy and ethical considerations, participant recruitment and compliance, and we will provide a summary of exploratory analyses of the DDPs. The second aim of this paper is to discuss the potential of DDPs for social media research. We will discuss ethical and procedural challenges, the usability of the content of the DDPs, and their overall potential for informing future research. Whereas an earlier paper by Boeschoten et al. (Citation2020) has presented an error framework for data donation using a hypothetical dataset, in this paper we aim to demonstrate the potential and challenges of DDPs using an actual dataset collected from a sample of adolescents.
How data donations could complement existing measures of social media use
The potential of several methodologies to investigate social media use has been investigated in recent years (Griffioen et al., Citation2020). Research in which smartphone use is tracked is a major step forward and has uncovered meaningful discrepancies between self-report and smartphone logs (Parry et al., Citation2021; Johannes et al., Citation2021; Verbeij et al., Citation2021). Unfortunately though, most of these log-based measures are still limited to measuring overall time spent on specific social media platforms, and, thus, provide little insight in the specific activities and content that is shared and encountered (Valkenburg, Meier et al., Citation2022).
Acquiring social media use data that provide further insight in specific activities and content shared and encountered on social media platforms comes with obvious challenges as to how to access this content while safeguarding participants’ privacy. Researchers have found innovative solutions to this challenge, for example, by collecting content of social media accounts with the help of application programming interfaces (APIs) provided by the platforms (Bayer et al., Citation2018). A main drawback of this method is that it only provides access to the type of data that are selected by the platform and that it is restricted to publicly available data. This method works for platforms in which profiles are automatically set to public, such as Twitter, but not for platforms such as Facebook or Instagram where users can select their privacy settings (Batrinca & Treleaven, Citation2015).
Other promising approaches are mobile sensing (Lind et al., Citation2018) and Screenomics (Ram et al., Citation2019). Mobile sensing tools are custom-built applications that collect data through existing smartphone sensors (e.g., GPS, Bluetooth, microphone). Some mobile sensing tools make use of additional sensors that are relevant for measuring social media use. For example, the tool EARS adds a custom keyboard that captures all text entered through that keyboard (Lind et al., Citation2018). The Screenomics approach extracts visual as well as textual content by taking automated screenshots of participants’ smartphone every few seconds (Ram et al., Citation2019).
Although both the mobile sensing and the Screenomics approaches hold great promise to capture moment-to-moment involvement with social media, DDPs come with four additional advantages. First, DDPs provide a full overview of the uses of a platform regardless of whether it was accessed via the phone, tablet, or laptop. Second, DDPs capture all user interactions with the platform from the moment a user created an account until the moment of the download request. Third, because data of platform users are collected automatically by the social media companies, no (smartphone) applications need to be installed and thus researcher bias is limited. Fourth, all information is timestamped and separated into text and media files, categorized per social media activity.
In the current paper, we focus on Instagram DDPs because it affords a wide range of user activities that are also characteristic of other platforms, such as (a) engaging in private exchanges through direct messaging, (b) browsing through profiles and content of others, (c) sharing content publicly by means of posts, (d) sharing stories, which represent ephemeral content that disappears after 24 hours of posting, and (e) providing feedback on content of others, such as through hearts and comments. In addition, the content that is shared and viewed can be text-, photo- and video-based. Finally, Instagram DDPs provide important other types of information such as the date the account was first created, the number of followers, and account settings such as whether the account is private or public.
Obtaining and exploring Instagram data download packages (AIM 1)
The current Instagram data donation study is part of a larger research project on the psychosocial consequences of social media use that ran from 21 November 2019 to 1 July 2020. The data donation was the last component of this project and took place between the end of June and beginning of July 2020. The DDPs were collected at the end of the full study so that they would cover participants’ Instagram use of the entire study period. An overview of the timeline of the overarching project is available on the Open Science Framework (osf: https://osf.io/n8v9f). An overview of all relevant supplements to this paper is also available on osf (https://osf.io/7gfe4).
Participants were recruited via a large secondary school in the south of the Netherlands. Of the 388 participants who started the overall project, 102 participants provided 110 useable Instagram DDPs: 96 participants donated one account, four donated two accounts, and two shared three accounts. The sample of participants who donated their DDPs was comparable to the sample who did not donate in terms of age (M age donation = 14.04 vs M age no donation = 14.14, t(386) = −1.2, p = .23). But the DDP sample consisted of more girls (68% vs 52%, X2 (2, N = 388) = 14.03, p = .001) and fewer participants who followed a lower educational track (31% vs 48% of those who did not donate, X2 (2, N = 388) = 9.23, p = .01).
As the sample in this study concerned adolescents, the procedure also included steps relevant to studies involving minors. The following six steps will be discussed: (1) privacy and ethical preparations, (2) obtaining parental consent and participant assent, (3) obtaining the Instagram DDPs, (4) processing the DDPs, (5) coding and counting the DDPs, and (6) providing some preliminary insights of DDP content and participants’ social media activities.
(1) Privacy and Ethical Preparations
DDPs contain private information as well as information from other platform users who cannot provide consent for participation but are connected to the accounts of participants. Thus, careful consideration regarding privacy and ethical challenges in the process is warranted. As using DDPs for research purposes is an unexplored territory, no clear guidelines for the procedure yet existed at the start of this project and, in our experience, different privacy officers interpret the European General Data Protection Regulation (GDPR) in different ways.
We carefully examined every step in the data donation process together with a data manager and privacy officer of the university in the months prior to the start of the overall study. Together with these officers, we agreed that the following four points were most essential for safeguarding ethical research practices and participants’ privacy: (a) transparency about the content, process, risks, and benefits of the data donation to the prospective participants and their parents/caregivers, (b) secure data processing and storage, (c) deidentification of the data, and (d) a limited number of researchers with data access for a limited amount of time. We will return to these points when discussing the next four steps.
(2) Obtaining Parental Consent and Informed Participant Assent
Before the start of the project, parents of all 745 potential participants (all 8th and 9th graders of the participating school) received information from the researchers about the full study, including the data donation component. Of the 400 participants who received parental consent, 388 provided assent for the larger study. Of these 388 participants, 287 (74%) obtained parental consent for the data download portion of the study. Of these 287 participants, 209 indicated to have an Instagram account and still took part in the larger study in June 2020. These participants received information about (a) what they would be sharing with the researchers if they agreed to participate in the DDP study, (b) the pseudonymization process, with examples of anonymized images, (c) how the data would be stored, and (d) the type of information the researchers were interested in (see Supplement 1A, https://osf.io/bnqfw). The reward for their data donation was 5 euros. Of the 209 participants, 148 provided informed assent and automatically proceeded with the data donation process (see Flowchart https://osf.io/8dqfu).
(3) Obtaining the Instagram DDPs
After providing informed assent, participants automatically proceeded to an online survey environment. They first received questions about how many and what type of accounts they wanted to share, for example, regular accounts, fan accounts, or hobby accounts. Next, they received detailed, visual instructions on how to download and share their Instagram DDPs via the Instagram website or the smartphone app (see Supplement 1B for our visual instructions, https://osf.io/bnqfw). The actual DDP procedure consisted of two main steps: requesting and downloading the DDP from Instagram and uploading the DDP to the research drive. At both steps, researchers answered participants’ questions and sent reminders if the step was not completed. Of the 148 participants who provided assent, 44 did not complete the two steps, due to the following set of challenges.
At the first step, participants had to log into their Instagram accounts to request the download and to confirm the e-mail address at which they wanted to receive the data. This turned out to be a first challenge. Some participants did not know their Instagram username and password as they were used to log in automatically on their smartphone Instagram app. These adolescents had to reset their password. Others needed to ask permission from their parents to log into Instagram, and yet others did not know the e-mail address that they had used to create the Instagram account. In our attempt to prepare participants to have this information at hand before starting the data download, some participants thought they had to share the login information with the researchers, making them distrustful of the process.
At the second step of the download, within a maximum of 48 hours after participants’ request, Instagram sent the data download zip files to the e-mail address participants had provided. Some participants received one and others up to 12 zip files, depending on the size of their DDP and the number of Instagram accounts. Once the DDPs arrived, participants needed to download the zip files from their e-mail and upload them to a protected server of the university via a private link. Each participant received their own individualized link to a data folder that was pre-labeled with their participant number.
The second step came with a second set of challenges. Some participants did not have enough space on their phone for the zip files to download, or they could not find the zip files on their phone once it was downloaded. Others were not able to upload complete zip files and instead had to open the zip file and upload individual subfolders and files. Large Instagram accounts consisted of multiple zip files, each of which also had to be uploaded separately. Depending on the individual zip file sizes and internet connection, this uploading process could take a few seconds to several minutes. In some cases, the home or school connection was not sufficiently stable or fast for the files to upload, resulting in files that were not fully uploaded and could not be opened.
In total, 104 participants donated 112 accounts. Of the 44 participants who ended up not sharing their account(s), 30 participants informed us that they no longer wanted to donate their account, either due to login troubles (n = 1), failed upload attempts (n = 5), or an unknown reason (n = 24). The other 14 participants simply stopped replying to prompts from the research team. Of the 112 accounts, two accounts were removed from further analysis due to technical issues, resulting in a final total of 110 accounts from 102 participants. Of the 110 accounts, 13 accounts were incomplete, because they contained parts that could not be opened, had one or more parts that were missing, and/or had empty parts.
(4) Processing the DDPs
By means of Python scripts, all DDPs were opened to prepare for deidentification and analyses. Each unzipped DDP contained folders and text files (JavaScript Object Notation [JSON]). presents an example of the structure of a DDP in 2020. An important discovery was that the composition of the DDPs depends on the time of donation. Instagram regularly adds features, affecting what is in the DDPs. This may result in either new folders and/or text files or in new information that is distributed across several existing folders and files. At the time of this study, the features “guides,” “fundraisers,” and “events” (see ) were fairly new and hardly used by the participants. Moreover, and importantly, these new folders and text files are typically not rolled out simultaneously across all users. This means that the structure and content of the DDP is not stable across time and participants.
Figure 1 (a). An Instagram story in a DDP from 2020. (b). The same Instagram story in a DDP from 2022.
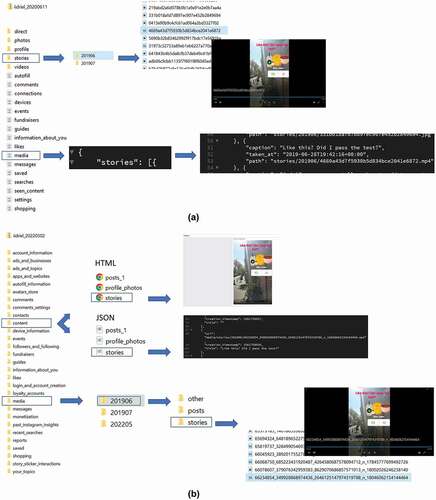
Since the time of data donation in 2020, Instagram added many new features and changed the structure of the packages (see ) for a comparison of a 2020 and a 2022 package). The DDPs from 2020 contain media folders and JSON files, in 2022 text files are stored in folders. Moreover, in 2022 users have the option to download their DDP in JSON or HTML format. The HTML format allows users to see the content of their DDPs on an Instagram webpage (see ) for a comparison of JSON and HTML formats). In the current study, only the JSON files were used as they contained all information needed to explore the type and frequency of the Instagram activities adolescents engaged in.
In line with the ethical and privacy agreements, the DDPs were deidentified with a Python script that combined the anonymization and pseudonymization steps. A detailed discussion of the deidentification procedure is provided in Boeschoten et al. (Citation2021) and the specific deidentification script used in this study is available at the ZENODO repository at https://doi.org/10.5281/zenodo.5211335. The script removed complete files from the DDPs that contained private information and were not needed for analysis, such as “uploaded contacts” and “devices,” which, respectively, provided contact information of others and listed the devices from which the Instagram account was accessed. In the remaining files, the scripts replaced names, phone numbers, e-mail addresses and URLs with the codes “_name,” “_phone number,” and “_URL,” respectively. In addition, participants’ usernames were deidentified by replacing them with a participant number. Finally, on the videos, faces and text on images that contained usernames were covered.
(5) Coding and Counting the Instagram DDPs
A promise of Instagram DDPs is that they provide insights in account characteristics, such as privacy settings, number of followers, and activities the participants engaged in. Python scripts were used to count the (1) overall characteristics of the account (e.g., number of followers, private vs public account, date of creation of account) and (2) activities of the account (e.g., number of posts, stories, likes, and comments) during the eight-month period of the project. shows a description of the text files used in this study and the characteristics and activities we obtained from them. Even though providing descriptive statistics of these text files seems a straightforward task, five main challenges arose during the coding and counting of the DDPs.
Table 1. Overview of text files and information obtained from each text file.
A first challenge pertained to decisions of the researchers as to how to assign frequencies to the Instagram activities. For example, one post can contain multiple images or videos. In the DDPs, these components are stored separately, but they have the same timestamp. We decided that all information with the exact same timestamp should be counted as one post. For stories, this is more complex. For example, a participant can post multiple images to one story, but this can only be done one by one and therefore each update is stored with a slightly different timestamp. Because participants sometimes updated their stories several times throughout a day and each individual update disappears after 24 hours, it proved impossible to determine what the user had intended as “one story update.” We therefore counted each listing as a separate story post.
The same challenge applied to counting direct messages. We were able to identify and use seven different types of direct messages in the DDPs in our sample, including text messages, GIFs, and post and stories that were shared in a direct message. These direct messages were each listed as separate messages with their own timestamps. Some messages contained multiple message types, for example, an image with text. We decided to take the same approach as we did with stories and posts and counted each message with its own timestamp as one direct message (see Supplement 2 for a complete overview, https://osf.io/bnqfw).
Identifying and operationalizing a chat was even more challenging. Chats are more or less synchronous exchanges of direct messages between two or more fellow users within a certain time frame. However, it proved to be impossible to determine when chats started or ended. We found that direct messages were exchanged within seconds to a few days, which begs the question how to operationalize chatting. Moreover, the messages text files contained numerous types of messages, from regular text messages to hearts (i.e., the like button in Instagram) and links to webpages. Some participants sent only hearts and others received but never sent messages. Therefore, we counted the number of different chats based on composition of the chat groups and the direct messages with individual timestamps that were sent by the participants in each group.
A second challenge was the lack of clarity about the Instagram functionality that each text file in the DDP referred to, as the name did not always reveal its exact function. For example, for the text file “seen_content” it is uncertain what counts as “seen.” Based on our assessments, it most likely is the content the participant actively clicked on. As with all text files, it is uncertain to what extent this information is complete. Certain is that, for example, stories that are seen are not in the text file “seen_content.”
A third challenge was that the names of the sections do not optimally describe their content. For example, the text file “seen_content” starts with “chaining_seen,” which is a timestamped list of profile names of others, seemingly all profiles a participant has clicked on while browsing. However, at the time of writing this paper it is unclear if these are all or only a selection of profiles the participant ever clicked on. Moreover, in more recently downloaded DDPs, a “seen_content” folder seemed absent (see ). After contacting Meta’s Academic Partnership Team (research.facebook.com), we were informed that the “seen_content” folder had recently been merged with a new folder that is now called “ads and topics.”
A fourth challenge was that not all accounts turned out to be complete. Two DDPs contained only one text file and missed all others, whereas four other accounts missed one text file. It is possible that participants decided not to share these specific files, or that they simply got lost for those who uploaded individual files instead of the full DDP zip file.
A fifth and final challenge related to the time of account creation, which was between September 2013 and June 2020. Six accounts were created during the eight-month period of the study, meaning that these accounts were not active during the full 8 months of the study and thus may skew average activity levels. It is possible that the accounts were created in response to this study, but it is more likely that they are illustrative of natural fluctuations in use among adolescents. Another important insight was that some accounts were created before the participants were of legal age to use Instagram. With accounts created in 2012, some participants must have been eight years old at that time.
(6) Preliminary Insights About Instagram Use
It is important to establish the frequencies with which participants engage in activities not only to better understand what Instagram activities could be studied, but also to determine how these activities should be studied. For example, to examine how each individual person is influenced by their media use, and how these associations vary from person to person, a large number of datapoints (>50-100) from a large group of individuals (300+) is warranted (Schultzberg & Muthén, Citation2018). As such, it is important to get insight in (a) how many accounts engage in certain activities (b) how frequently these accounts engage in these activities, and (c) how these activities fluctuate over time. We have shared a detailed overview of the activity statistics of our sample in Supplement 2 (https://osf.io/bnqfw) as well as the frequency data file (https://doi.org/10.21942/uva.19747042.v1). Below, we will highlight the most important findings.
As shows, not all Instagram activities were equally popular. Perhaps most remarkably, posting was relatively uncommon: In the eight month-period, only 57% of the accounts had ever posted and 43% did not post at all. On average, participants posted once a month, updated their stories twice a week, sent 36 direct messages per week, commented on posts four times a week, and liked no fewer than 135 posts a week. But not all accounts contributed equally to these activities. For example, 43% of the already infrequent number of posts and 40% of all story updates came from only two accounts. This skewed balance even held for direct messaging: More than half (54%) of all direct messages came from seven accounts, owned by six adolescents. Although there was some overlap in the accounts that were active across all activities, different accounts dominated different activities. Some accounts engaged mostly in sending direct messages, whereas others preferred to update their stories, or to watch content of others, and yet others engaged in all activities fairly equally over the 8-month period.
Figure 2. Frequencies of different Instagram activities.
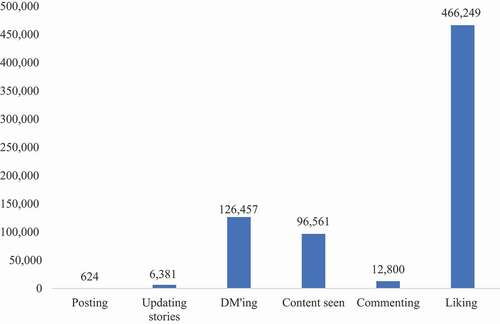
A final notable trend is that the activity levels fluctuated over time, with, for example, a peak in messages and posts around public and school holidays (i.e., December and May). But these fluctuations also differed across account over time. Some accounts were extremely active at one point in time and far less active at other moments in time. For example, shows how one account (the orange line) peaks in the number of stories around the month of April and is far less active before and after that peak.
Promises and pitfalls of social media data donations (AIM 2)
Data Download Packages (DDPs) come with a host of promises, but also with several important challenges that deserve further elaboration and reflection. Whether the benefits outweigh the disadvantages may depend on the research project. For colleagues who consider collecting DDPs, we evaluate (1) ethical considerations, (2) design and procedural considerations, (3) the usability of DDP content and activities, and (4) their potential for future research.
(1) Ethical Considerations
The biggest asset of DDPs also poses their largest challenge: The packages contain all content that was ever entered or shared by the participant. This puts forward three major ethical considerations. The first is the privacy-sensitive information in the DDPs, such as private messages or any content the participant shared from a private account. In fact, it is possible that participants share highly sensitive information, concerning, for example, bullying, sex, or suicide. The second consideration is that people who are connected to the participants’ account do not get the opportunity to provide consent for content and activities, like being tagged in a text or photo, private message exchanges, or being part of the uploaded contact list. The third consideration is that participants may have used their account from a young age, potentially when they and the persons they interacted with were not yet of legal age to do so. The legal age to use social media differs per platform and country. For example, the legal age to use Instagram is 16 in the Netherlands, unless users have permission from their parents, and 13 for Snapchat and TikTok. The level of intrusiveness that DDPs may evoke can be partially minimized by taking a few precautionary steps, such as a thorough informed consent process, anonymizing the data set, and collecting only the data that are needed for the study.
Although deidentification minimizes some risks, it comes with new challenges. For example, deidentification can also lead to removing information that may be relevant for studying social media uses and effects. In our data all words following a tag (e.g., @iidriel) were replaced by a code to ensure all account names were deidentified. Yet, names from companies (e.g., @Nike), influencers (e.g., @Beyonce), and locations (e.g., @home) were also removed. Moreover, by covering all faces in images, studying emotions that are shared through facial expressions is impossible. In addition, despite rigorous deidentification approaches, participants may still give away identifiable information about themselves or others by, for example, discussing their sexual identity or political preferences with a friend, or simply due to recognizable usage patterns (daily posters). In the context of open science, as researchers we need to have a good understanding about what data can be shared with other researchers given the privacy-sensitive data.
Since we started our project, other researchers have made progress in conceptualizing a tool that would allow for many of these precautionary steps. For example, Araujo et al., Citationin press are working on a web-based platform to which study participants can upload their files, see what they have uploaded, select what data they are willing to share, and subsequently have the data deidentified before they are sent to the researcher. Alternatively, researchers could process DDPs locally directly at the device of the participant (Boeschoten et al., Citation2022). And Foucault Welles (Citation2016) suggested to expand current research databases with DDPs from children and their parents that meet privacy standards and can be made available to all researchers. This way, the data cannot as easily be linked to specific data collection samples. In realizing such databases, researchers have to address the challenge to make them as inclusive as possible, with donations from those who are not tech savvy, have no easy access to technology or would not easily be reached by the calls for sharing data (Foucault Welles, Citation2016).
Overall, DDPs may provide a great opportunity to educate users about all the information that social media companies store, such as information that users may think is removed within 24 hours, such as Instagram stories. And DDPs can be used to provide users insight in their own social media use that goes beyond time spent on each app. We feel that the lessons that can be learned from the DDPs when they are shared with other researchers and users may outweigh the fact that the data are highly privacy-sensitive. We therefore highly recommend creating a learning opportunity for participants who share their DDPs. To do so, we need collaborative efforts to optimize the process of safeguarding privacy while also having access to the data we as researchers need (Foucault Welles, Citation2016; Stier et al., Citation2019).
(2) Design and Procedural Considerations
Understanding social media activities gathered via DDPs can be important in its own right. But it may be even more valuable when linked to predictors, mediators/moderators, and/or outcome variables measured via self-reports (Stier et al., Citation2019). This comes with several design considerations. Collecting DDPs should logically take place at the end of a data collection period to link multiple data collection methods, which adds to the laboriousness. For example, at the end of our eight-month study period, only one out of four participants who started the study shared their DDPs (see Flowchart, https://osf.io/8dqfu). Low consent rates are inherent to studies with digital trace data (Stier et al., Citation2019). Moreover, in longitudinal studies, participants may drop out over time or become less compliant and may not care to engage in another study activity. For example, in our study a predictor for donation was the level of compliance in the overall study (Struminskaya, Citation2022). Given that collecting DDPs inherently leads to low compliance rates, intensive recruitment and compliance procedures are even more pivotal than in any other study design.
Another important design question is what platform fits best with the research question and sample. Given that people use on average about five social media platforms to chat with friends and family and/or to present themselves to the broader audience (Waterloo et al., Citation2018), a focus on a single platform does not suffice. Ideally, to capture participants’ actual social media use, studies should collect DDPs of all social media platforms they use. However, a multiplication of DDPs leads to a multiplication of the complexity and laboriousness of the DDP gathering process. Therefore, gathering and analyzing DDPs can best be accomplished by a team of researchers who can collectively reap the rewards of their investments.
Acquiring DDPs also comes with several procedural steps that cannot be avoided even if an upload tool is at hand, and, thus, participants need to be carefully instructed. First, only participants themselves can request their DDPs. And second, they need to share it with the researcher once they have received it. The instructions for these steps depend first and foremost on the time of data donation as the way to request and download DDPs and the structure of DDPs changes over time (see Supplement 1B, https://osf.io/bnqfw/, and . Other factors that are important to consider are the size of the participant’s DDP, whether the participant requests the DDP via the app or a browser, and the number of accounts the participant chooses to download and share. Moreover, the time between the DDP request and receiving the DDP varies significantly across participants. These differences require tailoring the instructions to each individual participant.
In our study, we instructed participants via stepwise online visual instructions (see Supplement 1B, https://osf.io/bnqfw/). A disadvantage of such an online procedure is that it increases the chance of dropouts as it is harder to provide clear explanations and support from a distance. Moreover, it increases the chance of corrupt or incomplete DDPs. Much of these challenges could be solved with face-to-face or live video instructions. But participants would need to be visited twice, once to instruct them how to download the DDPs and once again to share their DDP with the researcher. Although researchers may simplify the download and upload process in the future, successful DDP donation will likely always be heavily dependent on participants’ compliance. We have collected our lessons learned and present a checklist of practicalities to consider when collecting DDPs in .
(3) The Usability of DDP Content and Activities
Table 2 Checklist of challenges to take into account when collecting (Instagram) DDPs
DDPs could supplement self-reports by providing insight in frequencies and timing of interactions with a variety of social media platforms, and, with a few exceptions such as Snapchat, give access to the content participants engaged with. Moreover, insights in these frequencies are in turn useful to decide what type of social media activities can be studied in what type of research designs. For example, consistent with many earlier studies that relied on self-reports (Faelens et al., Citation2019; Frison & Eggermont, Citation2020) and digital trace data of social media use (Marengo et al., Citation2021), we found that posting occurred with such a low frequency that it may not be fruitful to assess it in an experience sampling or diary study (Valkenburg, van Driel et al., Citation2022). Liking and direct messaging, in contrast, are suited for frequent measurement.
Coding the content of DDPs comes with five challenges. First, a manual to the content of the DDPs is typically absent. It is quite a burden to learn what each folder, file, and code in the DDP stands for. Second, the coding of the DDPs to measure participants’ social media use requires subjective choices of researchers. For example, counting chats and stories is challenging as there is no clear boundary indicating when one chat or story has started or ended. Moreover, participants can be part of a chat and receive messages of others, but never send a message. Third, as shows, the content and structure of DDPs are inconsistent over time. For example, new features are added, and these features are not available for all participants at a specific time. Because this inconsistency may also challenge future DDP research, one way to address potential problems may be to contact platforms, such as Meta’s recently established Academic Partnership Team (research.facebook.com). We contacted Meta's Team and asked for a guide to the content and structure of the current and future DDPs. They informed us that they did not have any guides to provide us because “they are tracking this internally through some internal groups.”
A fourth challenge is that DDPs do not contain all user data. For example, Instagram DDPs contain no to limited information on content that is seen, feedback from others, or use of filters. The only exception is for direct messages: DDPs contain the messages that were sent by others. Snapchat only stores meta-data, such as timestamps, but the content itself is not stored and can thus not be accessed. Moreover, DDPs do not provide insight in time spent on specific activities, such as how much time participants spent on crafting an Instagram post. This could be important as, for example, particularly playing with filters but not posting images is associated with well-being and body image (Vandenbosch et al., Citation2022). Such information can only be obtained when DDPs are used in combination with other methods, such as the Screenomics approach (Reeves et al., Citation2021). However, either method may not reveal what other data may be stored by social media companies that could be relevant to social media effects research.
Finally, a fifth challenge involves the labor intensiveness of coding social media activities. For example, to get insight in the impressive amount of textual data, manual content analysis may be unfeasible and automated textual content analyses may be necessary. However, the current lexicon-based sentiment analysis tools are not up to that challenge (Van Atteveldt et al., Citation2021). Reliable analyses of the DDPs require optimization of current automated textual content analyses. Moreover, since social media use is increasingly based on visuals, textual content analysis should be done in combination with visual analysis (Araujo et al., Citation2020).
(4) Potential for Future Research
DDPs can complement self-reports by more or less objectively establishing what people do and see on social media and inform future studies on what type of social media use can be studied with what type of research design. Some social media activities may be especially useful to assess as between-person differences, while others would be more suitable to study as within-person or person-specific effects (Valkenburg et al., Citation2021) or may warrant a focus on a specific subset of users (e.g., those who post frequently). Most importantly, across a variety of activities only a few participants seem highly active while the majority are “likers” and “lurkers” (Park & Macy, Citation2015; Van Driel et al., Citation2019). Moreover, the DDPs showed that levels of activity may vary greatly over time, between and within individual users. These varying patterns of use are important to consider when assessing social media use in the future and DDPs can assist with getting insight in these patterns.
Another relevant consideration for future research is why, how, and when to assess concepts such as “passive” and “active use.” Although posting may happen with low frequency, and be conceptualized as passive use by some (Valkenburg, van Driel et al., Citation2022), our DDPs showed that it was an activity that many engaged in with high frequency. And browsing, seen as a passive activity, involved actively selecting content of interest for many users. A similar consideration is how to think about and assess “public activity” and “private activity” when users of most social media platforms can adapt who can see their content. For example, posts can be made from public accounts with a high number of followers, but only made visible to others who are marked as close connections. Likewise, stories and messages can be shared one-to-one, but also with many followers. And importantly, many users own multiple accounts. One account may be for private activity, and the other for public activity.
Ultimately, of course, in the future researchers should implement multiple methods to assess a variety of social media uses across multiple platforms and combine these data with other measures such as those assessing psychosocial well-being (Parry et al., Citation2022; Stier et al., Citation2019; Tsugawa et al., Citation2015). However, next to the obvious privacy and feasibility challenges of these types of research endeavors, we should also consider reliable ways to automatically code social media content – images and text – such as obtained with DDPs, and ultimately have sufficiently large samples to apply the latest machine learning and deep learning techniques to effectively study patterns of social media use and its effects. This also means that we are in need of more skilled computational scientists to handle these data (Foucault Welles, Citation2016).
Conclusion
DDPs come with several clear challenges. But no one can deny that they offer tremendous opportunities to assess the content of social media interaction in a naturalistic environment. We hope that our honest discussion of the pitfalls encountered in the data collection procedure and coding process of DDPs may stimulate future researchers to follow up on these challenges, for example, by simplifying the process of sharing and extracting relevant features from DDPs, optimizing techniques for reliable automated textual and visual content analyses, collecting data on social media activities across platforms, and linking all relevant activities with self-report data. In the end, together we need to determine what data we can and what data we should collect.
Credit author statement
Irene van Driel: Conceptualization, data collection and coding, writing, revising original and subsequent drafts. Anastasia Giachanou: Script building and analysis of DDP data, creating graphs, reviewing drafts. Loes Pouwels: Conceptualization, methodological advice, revising original and final draft. Laura Boeschoten: Conceptualization, script building for anonymizing DDPs, reviewing final draft. Ine Beyens: Conceptualization, reviewing original and revising subsequent drafts. Patti Valkenburg: Conceptualization, writing, revising original and subsequent drafts.
Acknowledgments
We would like to thank Tim Verbeij, Teun Siebers, and Wieneke Rollman for their contribution to the data collection and/or coding of this study.
Disclosure statement
No potential conflict of interest was reported by the authors.
Additional information
Funding
Notes on contributors
Irene I. van Driel
Irene I. van Driel is a postdoctoral researcher in the Amsterdam School of Communication Research (ASCoR) at the University of Amsterdam. Her research focuses on the relationship between media and the psychosocial well-being of youth.
Anastasia Giachanou
Anastasia Giachanou is a postdoc fellow at the Human Data Science group at Utrecht University. She is particularly interested in research topics of Applied Data Science and Applied Text Mining.
J. Loes Pouwels
J. Loes Pouwels is an assistant professor at the Behavioural Science Institute at Radboud University, Nijmegen, The Netherlands. Her research interests include adolescents, social media use, psychosocial functioning, and peer relations.
Laura Boeschoten
Laura Boeschoten is an assistant professor at the Department of Methodology and Statistics at Utrecht University. Her research interests include digital trace data collection through data donation and estimating en correcting for measurement error in combined datasets.
Ine Beyens
Ine Beyens is an assistant professor in the Amsterdam School of Communication Research (ASCoR) at the University of Amsterdam. Her research focuses on the effects of media on the cognitive, affective, and social well-being of children and adolescents, with a special focus on differential susceptibility to media influences.
Patti M. Valkenburg
Patti M. Valkenburg is a University Distinguished Professor at the University of Amsterdam. Her research focuses on the cognitive, emotional, and social effects of (social) media on youth and adults. She is particularly interested in theorizing and studying how adolescents and adults differ in their susceptibility to the effects of (social) media.
References
- Araujo, T., Ausloos, J., van Atteveldt, W., Loecherbach, F., Moeller, J., Ohme, J., Trilling, D., van de Velde, B., de Vreese, C., & Welbers, K. (In press). OSD2F: An open-source data donation framework. Computational Communication Research. https://doi.org/10.31235/osf.io/xjk6t
- Araujo, T., Lock, I., & van de Velde, B. (2020). Automated Visual Content Analysis (AVCA) in communication research: A protocol for large scale image classification with pre-trained computer vision models. Communication Methods and Measures, 14(4), 239–265. https://doi.org/10.1080/19312458.2020.1810648
- Batrinca, B., & Treleaven, P. C. (2015). Social media analytics: A survey of techniques, tools and platforms. Ai & Society, 30(1), 89–116. https://doi.org/10.1007/s00146-014-0549-4
- Bayer, J. B., Ellison, N., Schoenebeck, S., Brady, E., & Falk, E. B. (2018). Facebook in context(s): Measuring emotional responses across time and space. New Media & Society, 20(3), 1047–1067. https://doi.org/10.1177/1461444816681522
- Boeschoten, L., Ausloos, J., Moeller, J., Araujo, T., & Oberski, D. L. (2020). Digital trace data collection through data donation. arXiv. https://doi.org/10.48550/arXiv.2011.09851
- Boeschoten, L., Mendrik, A., van der Veen, E., Vloothuis, J., Hu, H., Voorvaart, R., & Oberski, D. L. (2022). Privacy-preserving local analysis of digital trace data: A proof-of-concept. Patterns, 3(3). https://doi.org/10.1016/j.patter.2022.100444
- Boeschoten, L., Voorvaart, R., Kaandorp, C., Goorbergh, R. V. D., & De Vos, M. (2021). Automatic de-identification of data download packages. Data Science, 4(2), 101–120. https://doi.org/10.3233/DS-210035
- Burnell, K., George, M. J., Kurup, A. R., Underwood, M. K., & Ackerman, R. A. (2021). Associations between self-reports and device-reports of social networking site use: An application of the truth and bias model. Communication Methods and Measures, 15(2), 156–163. https://doi.org/10.1080/19312458.2021.1918654
- Faelens, L., Hoorelbeke, K., Fried, E., De Raedt, R., & Koster, E. H. W. (2019, February 5). Negative influences of Facebook use through the lens of network analysis. Computers in Human Behavior, 96, 13–22. https://doi.org/10.1016/j.chb.2019.02.002
- Foucault Welles, B. (2016). Computational CAM: Studying children and media in the age of big data. Journal of Children and Media, 10(1), 72–80. https://doi.org/10.1080/17482798.2015.1123165
- Frison, E., & Eggermont, S. (2020). Toward an integrated and differential approach to the relationships between loneliness, different types of Facebook use, and adolescents’ depressed mood. Communication Research, 47(5), 701–728. https://doi.org/10.1177/0093650215617506
- Griffioen, N., Rooij, M. V., Lichtwarck-Aschoff, A., & Granic, I. (2020). Toward improved methods in social media research. Technology, Mind, and Behavior, 1(1). https://doi.org/10.1037/tmb0000005
- Johannes, N., Nguyen, T.-V., Weinstein, N., & Przybylski, A. K. (2021). Objective, subjective, and accurate reporting of social media use: No evidence that daily social media use correlates with personality traits, motivational states, or well-being. Technology, Mind, and Behavior, 2(2). https://doi.org/10.1037/tmb0000035
- Lind, M. N., Byrne, M. L., Wicks, G., Smidt, A. M., & Allen, N. B. (2018). The Effortless Assessment of Risk States (EARS) tool: An interpersonal approach to mobile sensing. JMIR Ment Health, 5(3), e10334. https://doi.org/10.2196/10334
- Marengo, D., Montag, C., Sindermann, C., Elhai, J. D., & Settanni, M. (2021). Examining the links between active Facebook use, received likes, self-esteem and happiness: A study using objective social media data. Telematics and Informatics, 58. https://doi.org/10.1016/j.tele.2020.101523
- Odgers, C. L., & Jensen, M. R. (2020, January 17). Annual research review: Adolescent mental health in the digital age: Facts, fears, and future directions. Journal of Child Psychology and Psychiatry, 61(3), 336–348. https://doi.org/10.1111/jcpp.13190
- Park, P., & Macy, M. (2015). The paradox of active users. Big Data & Society, 2(2), 205395171560616. https://doi.org/10.1177/2053951715606164
- Parry, D. A., Davidson, B. I., Sewall, C. J. R., Fisher, J. T., Mieczkowski, H., & Quintana, D. S. (2021). A systematic review and meta-analysis of discrepancies between logged and self-reported digital media use. Nature Human Behaviour, 5(11), 1535–1547. https://doi.org/10.1038/s41562-021-01117-5
- Parry, D. A., Fisher, J., Mieczkowski, H., Sewall, C., & Davidson, B. I. (2022). Social media and well-being: A methodological perspective. Current Opinion in Psychology, 45, 101285. https://doi.org/10.1016/j.copsyc.2021.11.005
- Ram, N., Yang, X., Cho, M.-J., Brinberg, M., Muirhead, F., Reeves, B., & Robinson, T. N. (2019). Screenomics: A new approach for observing and studying individuals’ digital lives. Journal of Adolescent Research, 35(1), 16–50. https://doi.org/10.1177/0743558419883362
- Reeves, B., Ram, N., Robinson, T. N., Cummings, J. J., Giles, C. L., Pan, J., Chiatti, A., Cho, M., Roehrick, K., Yang, X., Gagneja, A., Brinberg, M., Muise, D., Lu, Y., Luo, M., Fitzgerald, A., & Yeykelis, L. (2021). Screenomics: A framework to capture and analyze personal life experiences and the ways that technology shapes them. Human–Computer Interaction, 36(2), 150–201. https://doi.org/10.1080/07370024.2019.1578652
- Schultzberg, M., & Muthén, B. (2018). Number of subjects and time points needed for multilevel time-series analysis: A simulation study of dynamic structural equation modeling. Structural Equation Modeling: A Multidisciplinary Journal, 25(4), 495–515. https://doi.org/10.1080/10705511.2017.1392862
- Stier, S., Breuer, J., Siegers, P., & Thorson, K. (2019). Integrating survey data and digital trace data: Key issues in developing an emerging field. Social Science Computer Review, 38(5), 503–516. https://doi.org/10.1177/0894439319843669
- Struminskaya, B. (2022). Willingness and consent to data donation: Methodological and practical aspects. Data Donation Day, Utrecht University. https://hds.sites.uu.nl/2022/01/15/data-donation-day/
- Thorisdottir, I. E., Sigurvinsdottir, R., Asgeirsdottir, B. B., Allegrante, J. P., & Sigfusdottir, I. D. (2019, August 1). Active and passive social media use and symptoms of anxiety and depressed mood among icelandic adolescents. Cyberpsychology, Behavior and Social Networking, 22(8), 535–542. https://doi.org/10.1089/cyber.2019.0079
- Tosun, L. P., & Kaşdarma, E. (2020). Passive Facebook use and depression: A study of the roles of upward comparisons, emotions, and friendship type. Journal of Media Psychology: Theories, Methods, and Applications, 32(4), 165–175. https://doi.org/10.1027/1864-1105/a000269
- Tsugawa, S., Kikuchi, Y., Kishino, F., Nakajima, K., Itoh, Y., & Ohsaki, H. (2015). Recognizing depression from twitter activity. Proceedings of the 33rd annual ACM conference on human factors in computing systems. https://doi.org/10.1145/2702123.2702280
- Valkenburg, P. M., Beyens, I., Pouwels, J. L., van Driel, I. I., & Keijsers, L. (2021). Social media and adolescents’ self-esteem: Heading for a person-specific media effects paradigm. Journal of Communication, 71(1), 56–78. https://doi.org/10.1093/joc/jqaa/039
- Valkenburg, P. M., Meier, A., & Beyens, I. (2022). Social media use and its impact on adolescent mental health: An umbrella review of the evidence. Current Opinion in Psychology, 44, 58–68. https://doi.org/10.1016/j.copsyc.2021.08.017
- Valkenburg, P. M., van Driel, I. I., & Beyens, I. (2022). The associations of active and passive social media use with well-being: A critical scoping review. New Media & Society, 24(2), 530–549. https://doi.org/10.31234/osf.io/j6xqz
- van Atteveldt, W., van der Velden, M. A. C. G., & Boukes, M. (2021). The validity of sentiment analysis: Comparing manual annotation, crowd-coding, dictionary approaches, and machine learning algorithms. Communication Methods and Measures, 15(2), 121–140. https://doi.org/10.1080/19312458.2020.1869198
- van Driel, I. I., Pouwels, J. L., Beyens, I., Keijsers, L., & Valkenburg, P. M. (2019). Posting, scrolling, chatting, and Snapping: Youth (14-15) and social media in 2019. Center for Research on Children, Adolescents, and the Media (CcaM), University of Amsterdam. https://www.project-awesome.nl/images/Posting-scrolling-chatting-and-snapping.pdf
- Vandenbosch, L., Fardouly, J., & Tiggemann, M. (2022, June 1). Social media and body image: Recent trends and future directions. Current Opinion in Psychology, 45, 101289. https://doi.org/10.1016/j.copsyc.2021.12.002
- Verbeij, T., Pouwels, J. L., Beyens, I., & Valkenburg, P. M. (2021). postin. Computers in Human Behavior Reports, 3, 100090. https://doi.org/10.1016/j.chbr.2021.100090
- Waterloo, S. F., Baumgartner, S. E., Peter, J., & Valkenburg, P. M. (2018). Norms of online expressions of emotion: Comparing Facebook, Twitter, Instagram, and WhatsApp. New Media & Society, 20(5), 1813–1831. https://doi.org/10.1177/1461444817707349