ABSTRACT
Melanoma is a type of cancer with a relatively poor prognosis. The development of immunotherapy for the treatment of patients with melanoma has drawn considerable attention in recent years. It is of great clinical significance to identify novel promising prognostic biomarkers and to explore their roles in the immune microenvironment. The solute carrier (SLC) superfamily is a group of transporters predominantly expressed on the cell membrane and are involved in substance transport. SLC16A1 is a member of the SLC family, participating in the transport of lactate, pyruvate, amino acids, ketone bodies, etc. The role of SLC16A1 in tumor immunity has been recently elucidated, while its role in melanoma remains unclear. In this study, bioinformatics analysis was performed to explore the role of SLC16A1 in melanoma. The results showed that high SLC16A1 expression was correlated with decreased overall survival in patients with melanoma. The genes co-expressed with SLC16A1 were significantly enriched in metabolic regulation, protein ubiquitination, and substance localization. Moreover, SLC16A1 was correlated with the infiltration of immune cells. In conclusion, SLC16A1 is a robust prognostic biomarker for melanoma and may be used as a novel target in immunotherapy.
Introduction
The transmembrane delivery of substances is mediated by various transporters, which play an important role in maintaining metabolic homeostasis in cells [Citation1]. These transporters are located in the plasma membrane of cells and organelles [Citation2]. The ATP-binding cassette (ABC) superfamily and the solute carrier (SLC) superfamily are the two main transporter superfamilies [Citation3]. The ABC transporters are powered by ATP hydrolysis and mainly responsible for outward transport [Citation4], while the SLC transporters are predominantly secondary active, relying on a previously established electrochemical gradient to transport substrates across the membrane [Citation5,Citation6]. According to their solute specificity, the SLC transporters can be divided into highly selective transporters, which transport a narrow group of substrates, and lowly selective transporters, which interact with a broad range of substrates [Citation7,Citation8]. SLC transporters play an important role in tumorigenesis, tumor progression, and metastasis [Citation9]. They provide new insights into targeted therapy and gene therapy [Citation10]. However, the current understanding of SLC transporters is far from enough. SLC16A1 (MCT1), a monocarboxylate transporter that belongs to the SLC superfamily, has recently been reported to play a critical role in tumor immunity [Citation11]. Therefore, we hypothesized that SLC16A1 may be used to predict the prognosis of cancer patients and evaluate immune infiltration, thereby promoting the development of tumor therapy.
Melanoma is a highly malignant tumor often associated with a poor prognosis, especially after metastasis [Citation12]. It causes approximately 55,500 deaths per year [Citation13]. The identification of novel biomarkers may contribute to early diagnosis, treatment development, and prognosis improvement of melanoma. At present, PD1/PDL1 inhibitors and BRAF mutation inhibitors have shown promising results in the treatment of melanoma; however, drug resistance remains a significant problem [Citation14]. It is of great importance to search for new targets.
In this study, bioinformatic data mining was performed to investigate the expression and co-expression pattern of SLC16A1, and the correlation of SLC16A1 expression with the prognosis and immune infiltration in melanoma. These results have implications for the development of melanoma treatment.
Methods
ONCOMINE
ONCOMINE is a public database containing DNA microarray data that can be used to explore potential target genes and their expression patterns [Citation15]. An overview analysis of SLC16A1 in a variety of cancers was performed using the ONCOMINE database. The search formula was set to: “Gene: SLC16A1; P-value: 1E-4; Fold change: 2”.
GEPIA
GEPIA is a database of high-throughput RNA sequencing data that can be used to analyze gene transcription [Citation16]. The RNA sequencing data of tumor and normal tissues from The Cancer Genome Atlas (TCGA) and GTEX databases and relevant patient information were included in the GEPIA database. The transcription of SLC16A1 in different types of cancers and its association with the prognosis of melanoma patients were analyzed using GEPIA.
UALCAN
UALCAN is an open, user-friendly web tool based on the TCGA database [Citation17]. It was used to analyze the expression of SLC16A1 in a variety of cancers and to identify the co-expression pattern of SLC16A1.
Human protein atlas (HPA)
The HPA database provides protein expression data of diseased and normal tissues. The expression of SLC16A1 in melanoma was validated using the immunohistochemical data in the HPA database.
cBioPortal
cBioPortal is a powerful web tool that can be used to explore gene expression and genetic alteration frequency, and to perform survival analysis, co-expression analysis, and signaling pathway analysis [Citation18]. The “mutation” module was used to explore the mutation frequency of SLC16A1 in different types of cancers, including melanoma.
R software
The data of melanoma and normal groups obtained from TCGA were analyzed using the R software (version 4.0.3) . The R package “Clusterprofiler” was used for Gene Ontology (GO) and Kyoto Encyclopedia of Genes and Genomes (KEGG) enrichment analyses of co-expressed genes.
STRING database and cytoscape software
The STRING is a database of protein-protein interactions [Citation19]. The protein patterns of co-expressed genes were explored using the STRING database and visualized by the Cytoscape software.
TIMER
TIMER is an open, easy-to-use online tool for investigating the association of genes in immune infiltration [Citation20]. The “Gene” module of TIMER was used in this study. The study gene was SLC16A1 and the immune cells were B cells, CD4+ T cells, CD8+ T cells, macrophages, neutrophils, and dendritic cells.
Results
Expression of SLC16A1 across cancers
A valuable biomarker is usually differentially expressed in tumors. We first compared the expression of SLC16A1 in tumors and normal tissues. To reduce bias, three databases (GEPIA, UALCAN, and ONCOMINE) were used to analyze the expression of SLC16A1 in different types of cancers. The results showed that SLC16A1 was upregulated in many cancers, including melanoma (–c).
Figure 1. (a) Differential expression of SLC16A1 in multiple cancers in GEPIA database: SLC16A1 was over-expressed in skin cutaneous melanoma (SKCM) compared with normal tissues (P < 0.05). (b) Expression of SLC16A1 across TCGA cancers: SLC16A1 was significantly expressed in melanoma compared to normal tissue. (c)Overview of SCL16A1 in multiple cancers in Oncomine database: compared with normal tissue, the fold change of SLC16A1 expression in melanoma was 3, with a statistically significant difference
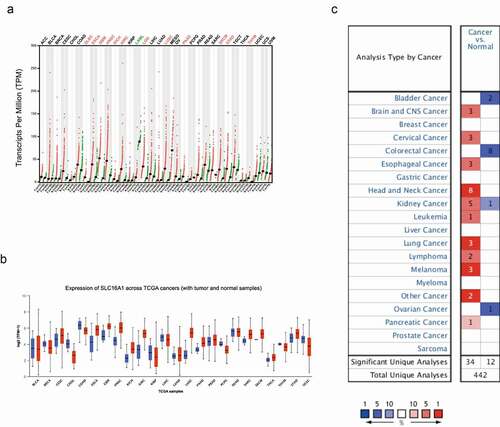
Expression of SLC16A1 in melanoma
To verify the upregulation of SLC16A1 in melanoma, we further compared the expression of SLC16A1 in 461 melanoma patients and 558 healthy subjects using the “Boxplots” module in GEPIA. The results showed that the expression of SLC16A1 was significantly increased in melanoma tissues compared with normal tissues (p < 0.05) (). Then, we used the “Gene Comparison” function of GEPIA to analyze the expression of SLC16A1 in melanoma and the results showed that SLC16A1 was highly expressed in melanoma cells ().
Figure 2. (a) Box plot from GEPIA database: analysis of 461 melanomas and 558 normal tissues showed that SLC16A1 was significantly overexpressed in melanoma (P <0.05). (b) Expression of SLC16A1 in melanoma in GEPIA database: The expression of SLC16A1 in melanoma was about 4.5 times higher than that in normal tissue. (c) Overall survival analysis of SLC16A1 expression in melanoma (GEPIA): Analysis of 229 melanoma patients with high SLC16A1 expression and 229 patients with low SLC16A1 expression showed that high SLC16A1 expression significantly reduced overall survival in melanoma patients (HR = 1.4, P = 0.013). (d) Disease-free survival analysis of SLC16A1 expression in melanoma (GEPIA): Analysis of 229 melanoma patients with high SLC16A1 expression and 229 patients with low SLC16A1 expression showed no statistically significant association between high SLC16A1 expression and disease-free survival in melanoma patients (HR = 1, P = 0.8)
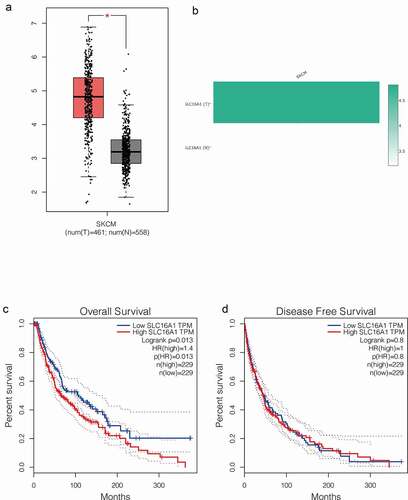
SLC16A1-related survival analysis in melanoma
To investigate the prognostic value of SLC16A1 in melanoma, we performed survival analysis. The “Survival” module of GEPIA was used to determine whether the expression of SLC16A1 was associated with the prognosis of melanoma patients. We found that high SLC16A1 expression significantly reduced the overall survival of melanoma patients (p = 0.013) (), but had no effect on disease-free survival (p = 0.8) ().
Co-expression pattern of SLC16A1 in melanoma
The expression of SLC16A1 in tumors may be affected by other genes. The identification of the co-expression pattern of SLC16A1 can elucidate its function and potential clinical significance in melanoma. The co-expression module in the UALCAN database was used and the top 100 genes co-expressed with SLC16A1 are shown in heat maps (–d).
GO and KEGG enrichment analyses of co-expressed genes
After obtaining the genes co-expressed with SLC16A1, we performed GO and KEGG enrichment analyses. The GO enrichment analysis showed that the co-expressed genes were significantly enriched in biological process (BP), cellular component (CC), and molecular function (MF) of melanoma (). In BP, the co-expressed genes were closely related to the regulation of mRNA metabolic process, proteasomal protein catabolic process, proteasome-mediated ubiquitin-dependent protein catabolic process, protein poly-ubiquitination, and nucleocytoplasmic transport. In CC, the co-expressed genes were significantly correlated with nuclear envelope, ribonucleoprotein granule, ubiquitin ligase complex, and nuclear speck. In MF, the co-expressed genes were significantly correlated to ubiquitin-like protein transferase activity, kinase regulator activity, and ribonucleoprotein complex binding. The KEGG analysis revealed that the co-expressed genes were mainly enriched in human cytomegalovirus infection, ubiquitin-mediated proteolysis, RNA transport, ErbB signaling pathway, and regulation of actin cytoskeleton ().
Protein-protein interaction network of co-expressed genes
To identify the key proteins of co-expressed pattern involved in melanoma, the protein-protein interactions of co-expressed genes were explored. The protein-protein interaction network of genes co-expressed with SLC16A1 is shown in . TRIP12, HNRNPU, and DHX15 are the key proteins in the network, which warrant further exploration.
Correlation between SLC16A1 and immune infiltration in melanoma
The pathogenesis of melanoma is highly correlated with tumor immunity. Thus, we investigated whether the expression of SLC16A1 was associated with immune cell infiltration in melanoma. The results of TIMER analysis showed that the expression level of SLC16A1 was significantly correlated with the infiltration of CD8+ T cells (p = 9.40e-05), macrophages (p = 5.66e-05), and neutrophils (p = 5.03e-09) (), suggesting the potential role of SLC16A1 in tumor immunity.
Genetic alteration frequency of SLC16A1 across cancers
To determine the sensitivity of SLC16A1 as a biomarker for melanoma, we analyzed the alteration frequency of the SLC16A1 gene in different types of melanoma using the Cbioportal database. The results showed that the alteration frequency of SLC16A1 was high in metastatic melanoma (7.8%) ().
Validation of protein expression of SLC16A1
We further analyzed the protein expression of SLC16A1 using the immunohistochemical data from the HPA database. The results showed that SLC16A1 was barely stained in two normal tissue samples, but medium-stained in four primary melanoma tissue samples. In two metastatic melanoma tissue samples, one was moderately stained and the other was strongly stained ().
Figure 9. Immunohistochemistry sections of normal skin tissues and melanomas in Human Protein Atlas: (a, b) Immunohistochemistry of two normal skin tissues showed that SLC16A1 was almost undetectable in normal skin tissues. (c-f) Immunohistochemistry sections of four primary melanoma patients showed moderate staining of SLC16A1 in the primary melanoma. (g, h) Immunohistochemistry sections of two patients with metastatic melanoma. Figure H shows the strong staining of SLC16A1 in metastatic melanoma
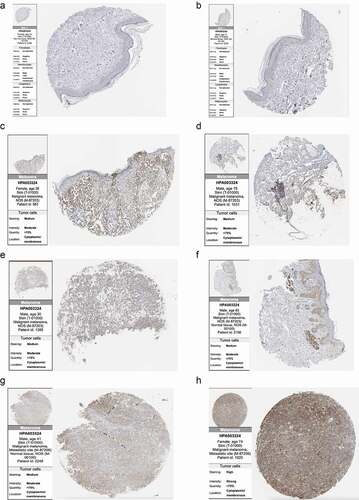
Discussion
Cancer cells prefer anaerobic metabolism over the aerobic respiration pathway, a phenomenon termed the “Warburg Effect” [Citation21]. In 1924, Otto Warburg published an essay in Biochemische Zeitschrift, showing that cancer cells, especially the highly malignant ones, can switch glucose metabolism from oxidative phosphorylation to anaerobic glycolysis, which is essential for cancer cell survival and proliferation. Since then, anaerobic metabolism and enhanced lactate production have been recognized as cancer’s “Achilles’ heel”, attracting considerable attention for decades [Citation22,Citation23]. Increased glycolysis and anaerobic oxidation favor the establishment of a lactate-rich microenvironment within highly malignant tumors, including melanoma.
Lactate was seen as a metabolic waste product for many years. However, recent evidence has shown that lactate is partially responsible for the immunosuppressive microenvironment in malignant melanoma [Citation24]. A study of the tumor microenvironment and tumor infiltration in malignant melanoma showed that Treg cells in the tumor microenvironment mainly relied on lactic acid, which was transported by SLC16A1, for energy supply, and thus mediated tumor immune tolerance and reduced the efficacy of effector T cells [Citation11]. The transport of lactate to the tumor microenvironment by SLC16A1 greatly enhances cellular metabolism and the survival of melanoma cells. Accordingly, we assumed that SLC16A1 might act as a therapeutic target for melanoma.
In this study, we found that SLC16A1 was highly expressed in various types of cancers, including melanoma. The upregulation of SLC16A1 in melanoma was verified by the protein expression data from the HPA database. Further analysis revealed that high SLC16A1 expression was associated with poor overall survival in patients with melanoma, and therefore, it might be used as a prognostic indicator for melanoma. The genes that co-expressed with SLC16A1 were mainly enriched in RNA metabolic process, proteasomal protein catabolic process, proteasome-mediated ubiquitin-dependent protein catabolic process, protein poly-ubiquitination, ubiquitin-like protein transferase activity, etc. The KEGG pathway enrichment analysis showed that the gene sets were mainly enriched in cytomegalovirus infection, ubiquitin-mediated proteolysis, RNA transport, ErbB signaling pathway, and regulation of actin cytoskeleton pathways. Additionally, the alteration frequency of the SLC16A1 gene was higher in metastatic melanoma compared to non-metastatic melanoma.
The occurrence of cancer requires a series of cellular phenotypic changes [Citation25]. Both genetic and environmental factors are involved in tumorigenesis [Citation26]. The epigenetic characteristics of cancers may differ due to different pathogenic factors. Tumor heterogeneity is also observed in various cancers [Citation27]. A better understanding of the biological characteristics of tumors may facilitate the development of cancer treatment. The hallmarks of tumor progression include over-activation of growth signals, insensitivity to growth-inhibitory signals, avoidance of apoptosis, unrestricted replication potential, continuous vascular growth, tissue invasion, and immune reediting [Citation26]. Melanoma is a highly immune-related tumor. SLC16A1 has recently been reported to be associated with immune tolerance of tumors. In the current study, we investigated the potential of SLC16A1 as a prognostic biomarker for melanoma.
In this era of precision medicine, the identification of novel biomarkers for cancers has become a significant goal in oncology research. Here, we found that SLC16A1 was significantly overexpressed in melanoma, and the alteration frequency of the SLC16A1 gene was higher in metastatic melanoma, indicating the potential of SLC16A1 as a diagnostic marker of melanoma and an indicator of metastasis. Whether high SLC16A1 expression can increase the invasion capacity of melanoma cells warrants further investigation.
The functions of the genes co-expressed with SLC16A1 include metabolic regulation, protein ubiquitination, and substance localization. Ubiquitination is one of the most common post-transcriptional modifications [Citation28], through which proteins are degraded by proteasomes. Ubiquitination occurs in both normal and diseased cells [Citation29]. It is a process closely related to protein localization, protein transport, protein interaction, DNA repair, cell cycle regulation, and autophagy [Citation30]. Ubiquitin-mediated degradation of P27, P53, and NF-κB has been shown to promote the occurrence and development of tumors [Citation31]. Therefore, the association between the co-expression pattern of SLC16A1 and ubiquitination may partly explain why melanoma cells show increased invasion capacity and escape from apoptosis. The co-expression pattern of SLC16A1 also provides new insights into the treatment of melanoma, that is, inhibiting excessive growth of tumor cells by targeted ubiquitination. Two proteasome inhibitors, bortezomib (Velcade) and carfilzomib (Kyprolis) have been approved by the U.S. Food and Drug Administration [Citation32]. The ubiquitin inhibitors of P53 and NF-κB are under evaluation in clinical trials [Citation33]. The development of this therapeutic approach is promising.
The over-activation of growth signals, such as the ErbB, VEGF, and PI3K signaling pathways [Citation25], leads to tumorigenesis, tumor progression, and metastasis [Citation26]. The ErbB receptors include ERBB1 (EGFR/HER1), ERBB2 (HER2), ERBB3, and ERBB4. The ERBB1- and ERBB2-targeted therapies have shown promising results in clinical trials [Citation34]. In our study, the KEGG enrichment analysis demonstrated that the co-expression pattern of SLC16A1 was associated with the ErbB signaling pathway. These findings not only revealed the potential pathogenic mechanisms of melanoma but also supports the development of melanoma treatment.
The interaction between the tumor and the immune system is fundamental to immunotherapy [Citation35]. Both innate and acquired immunity are involved in the formation of the tumor immune microenvironment [Citation36]. The studies of tumor-infiltrating immune cells can guide the development of immunotherapy strategies and predict the response of cancer patients to immunotherapy. SLC16A1 is a lactic acid transporter in Treg cells, thus mediating tumor immune tolerance [Citation11]. Our results showed that SLC16A1 was associated with the infiltration of CD8+ T cells, macrophages, and neutrophils in melanoma, indicating the involvement of SLC16A1 in both innate and acquired immunity in melanoma. These findings may provide some guidance for future investigations of tumor immunity in melanoma.
Therapeutic approaches regulating the function of SLC transporters are currently being tested. For patients with impaired SLC transporter function, an activator of SLC transporters may be designed to improve their function [Citation37]. In diseases with excessive SLC transporter activity, inhibitors that target the SLC channel can be used to block these transporters [Citation38]. Other methods, such as gene therapy and bypass transport, can also be used to regulate the activity of SLC transporters [Citation39]. In the future, melanoma patients with mutant SLC genes may receive treatments targeting SLC mutations.
Our study has some limitations. First, in vivo or in vitro experiments were not performed to verify the function of SLC16A1 in melanoma. Second, the alteration rate of the SLC16A1 gene was relatively low, which reduced the sensitivity of the analysis. Future in-depth exploration of the role of SLC16A1 in melanoma is needed.
Conclusion
In conclusion, for tumors with a poor prognosis, such as melanoma, the identification of a sensitive prognostic indicator may greatly improve the therapeutic effect and the quality of life of patients. Our study shows that SLC16A1, a member of the SLC transporter superfamily, is a promising prognostic indicator for melanoma. SLC16A1 is significantly overexpressed in melanoma, especially in metastatic sites, and its co-expression genes are mainly associated with RNA metabolic process, ubiquitination, ErbB signaling pathway, and material transport. SLC16A1 is also associated with immune cell infiltration. Further exploration of SLC16A1 may facilitate the development of precision treatment for melanoma patients and improve the clinical effect of melanoma treatment.
Acknowledgments
We are very grateful for data provided by databases such as TCGA, GEO, GEPIA.
Disclosure statement
No potential conflict of interest was reported by the authors.
References
- Tai W, Gao X. Noncovalent tagging of siRNA with steroids for transmembrane delivery. Biomaterials. 2018;178:720–727.
- Ma S. Membrane transporter research in times of countless structures. Biochim Biophys Acta Biomembr. 2018;1860(4):804–808.
- Me M, Rodriguez-Cruz V, Felmlee MA, et al. SLC and ABC transporters: expression, Localization, and species differences at the blood-brain and the blood-cerebrospinal fluid barriers. AAPS J. 2017;19(5):1317–1331.
- Amawi H, Sim HM, Tiwari AK, et al. ABC transporter-mediated multidrug-resistant cancer. Adv Exp Med Biol. 2019;1141:549–580.
- Zhang Y, Wang J. Targeting uptake transporters for cancer imaging and treatment. Acta Pharm Sin B. 2020;10(1):79–90.
- Ya B, Costales C, Mathialagan S, et al. Quantitative contribution of six major transporters to the hepatic uptake of drugs: “SLC-Phenotyping” using primary human hepatocytes. [published correction appears in J pharmacol exp ther. J Pharmacol Exp Ther. 2019;370(1):72–83. 2020 Mar;372(3):354].
- Ww W, Gallo L, Jadhav A, et al. The druggability of solute carriers. J Med Chem. 2020;63(8):3834–3867. .
- Zimmermann I, Egloff P, Hutter CA, et al. Synthetic single domain antibodies for the conformational trapping of membrane proteins. Elife. 2018;7:e34317. . Published 2018 May 24.
- Liu X. SLC family transporters. Adv Exp Med Biol. 2019;1141:101–202.
- Liu X. Transporter-mediated drug-drug interactions and their significance. Adv Exp Med Biol. 2019;1141:241–291.
- MJ W, Vignali PDA, Mullett SJ, et al. Metabolic support of tumour-infiltrating regulatory T cells by lactic acid. Nature. 2021;591(7851):645–651. .
- Schadendorf D, ACJ VA, Berking C, et al. Melanoma [published correction appears in lancet. Lancet. 2018;392(10151):971–984. . 2019 Feb 23;393(10173):746].
- International Agency for Research on Cancer, WHO. GLOBOCAN 2012: estimated cancer incidence, mortality, and prevalence worldwide in 2012. 2013. http://globocan.iarc.fr.
- Schadendorf D, Fisher DE, Garbe C, et al. Melanoma. Nat Rev Dis Primers. 2015;1:15003. Published 2015 Apr 23.
- Dr R, Yu J, Shanker K, et al. ONCOMINE: a cancer microarray database and integrated data-mining platform. Neoplasia. 2004;6(1):1–6.
- Tang Z, Li C, Kang B, et al. GEPIA: a web server for cancer and normal gene expression profiling and interactive analyses. Nucleic Acids Res. 2017;45(W1):W98–W102. .
- DS C, Bashel B, Balasubramanya SAH, et al. UALCAN: a portal for facilitating tumor subgroup gene expression and survival analyses. Neoplasia. 2017;19(8):649–658.
- Gao J, Aksoy BA, Dogrusoz U, et al. Integrative analysis of complex cancer genomics and clinical profiles using the cBioPortal. Sci Signal. 2013;6(269):pl1. Published 2013 Apr 2.
- Szklarczyk D, Morris JH, Cook H, et al. The STRING database in 2017: quality-controlled protein-protein association networks, made broadly accessible. Nucleic Acids Res. 2017;45(D1):D362–D368. .
- Li T, Fan J, Wang B, et al. TIMER: a web server for comprehensive analysis of tumor-infiltrating immune cells. Cancer Res. 2017;77(21):e108–e110.
- Schwartz L, Supuran CT, Alfarouk KO, et al. The warburg effect and the hallmarks of cancer. Anticancer Agents Med Chem. 2017;17(2):164–170. .
- Vander HMG, Cantley LC, Thompson CB, et al. Understanding the warburg effect: the metabolic requirements of cell proliferation. Science. 2009;324(5930):1029–1033. .
- Kroemer G, Pouyssegur J. Tumor cell metabolism: cancer’s Achilles’ heel. Cancer Cell. 2008;13(6):472–482.
- Tasdogan A, Faubert B, Ramesh V, et al. Metabolic heterogeneity confers differences in melanoma metastatic potential. Nature. 2020;577(7788):115–120. .
- Ia C. Cancer biology. Methods Mol Biol. 2011;731:1–11.
- Faratian D, Bown JL, Smith VA, et al. Cancer systems biology. Methods Mol Biol. 2010;662:245–263.
- Ma D, Kouzarides T. Cancer epigenetics: from mechanism to therapy. Cell. 2012;150(1):12–27.
- Balaji V, Pokrzywa W, Hoppe T, et al. Ubiquitylation pathways in insulin signaling and organismal homeostasis. Bioessays. 2018;40(5):e1700223. .
- Popovic D, Vucic D, Dikic I, et al. Ubiquitination in disease pathogenesis and treatment. Nat Med. 2014;20(11):1242–1253. .
- Rape M. Ubiquitylation at the crossroads of development and disease. Nat Rev Mol Cell Biol. 2018;19(1):59–70.
- Sa W, Beli P, Weinert BT, et al. A proteome-wide, quantitative survey of in vivo ubiquitylation sites reveals widespread regulatory roles. Mol Cell Proteomics. 2011;10(10):M111.013284. .
- Fan Y, You G. Proteasome inhibitors bortezomib and carfilzomib stimulate the transport activity of human organic anion transporter 1. Mol Pharmacol. 2020;97(6):384–391.
- Katsuya K, Oikawa D, Iio K, et al. Small-molecule inhibitors of linear ubiquitin chain assembly complex (LUBAC), HOIPINs, suppress NF-κB signaling. Biochem Biophys Res Commun. 2019;509(3):700–706. .
- Wang Z. ErbB receptors and cancer. Methods Mol Biol. 2017;1652:3–35.
- Sasidharan NV, Elkord E. Immune checkpoint inhibitors in cancer therapy: a focus on T-regulatory cells [published correction appears in immunol cell biol. Immunol Cell Biol. 2018;96(1):21–33. 2018 Feb;96(2):236].
- Burugu S, Asleh-Aburaya K, Nielsen TO, et al. Immune infiltrates in the breast cancer microenvironment: detection, characterization and clinical implication. Breast Cancer. 2017;24(1):3–15. .
- Bc C, Vucic S, Krishnan AV, et al. Riluzole, neuroprotection and amyotrophic lateral sclerosis. Curr Med Chem. 2010;17(18):1942-199.
- Hu G, Henke A, Karpowicz RJ Jr, et al. New fluorescent substrate enables quantitative and high-throughput examination of vesicular monoamine transporter 2 (VMAT2). ACS Chem Biol. 2013;8(9):1947–1954. .
- Lin L, Yee SW, Kim RB, et al. SLC transporters as therapeutic targets: emerging opportunities. Nat Rev Drug Discov. 2015;14(8):543–560.