Abstract
We use quantile treatment effects estimation to examine the consequences of the random-assignment New York City School Choice Scholarship Program across the distribution of student achievement. Our analyses suggest that the program had negligible and statistically insignificant effects across the skill distribution. In addition to contributing to the literature on school choice, the article illustrates several ways in which distributional effects estimation can enrich educational research: First, we demonstrate that moving beyond a focus on mean effects estimation makes it possible to generate and test new hypotheses about the heterogeneity of educational treatment effects that speak to the justification for many interventions. Second, we demonstrate that distributional effects can uncover issues even with well-studied data sets by forcing analysts to view their data in new ways. Finally, such estimates highlight where in the overall national achievement distribution test scores of children exposed to particular interventions lie; this is important for exploring the external validity of the intervention's effects.
Notes
Other domestic voucher studies that have used random assignment include the voucher experiments in Dayton, Ohio, and Washington, DC (Howell & Peterson, Citation2000; Howell, Wolf, Campbell, & Peterson, Citation2002; Wolf, Howell, & Peterson Citation2000). Internationally, experiments were also conducted in Chile (Lara, Mizala, & Repetto, Citation2011; McEwan & Carnoy Citation2000) and Colombia (Angrist, Bettinger, & Kremer, Citation2006). The Milwaukee voucher program also took advantage of a legally required lottery policy to assign vouchers, although voucher assignment was overseen by administrators and not independent evaluators (Greene, Peterson, & Du, 1997, 1998; Rouse, Citation1998; Witte, Citation1998). In addition, several studies have examined voucher programs using observational data. Domestically, these include Cleveland (Greene, Howell, & Peterson, Citation1997; Peterson, Howell, & Greene, Citation1999), Florida (Chakrabarti, Citation2013; Greene & Winters, Citation2003; Kupermintz, Citation2002), Milwaukee (Rouse, Citation1998), and San Antonio (Peterson, Myers, & Howell, Citation1999), and internationally, New Zealand (Ladd & Fiske, Citation2003).
When analysts classify only students with African American mothers as African American, voucher receipt has a positive effect on their achievement. However, this effect is not significantly different from zero when students with either African American mothers or fathers are included in the pool of African American students (Krueger & Zhu, Citation2004a). Furthermore, Krueger and Zhu (2004a, 2004b) demonstrated that positive effects for African Americans (however defined) hold only when controlling for students’ baseline test scores. Krueger and Zhu pointed out that controlling for baseline test scores is not required to gain valid estimates of the effect of voucher receipt on student achievement, because assignment to treatment and control conditions is independent of student test scores. Furthermore, they maintained that controlling for baseline test scores while omitting observations without baseline scores may introduce bias, because a sizable proportion of students are missing these scores and they appear to be neither randomly selected from the student population nor evenly split between the treatment and control groups.
Note that such predictions are often made assuming that the prospective voucher applicants are drawn from the full test score distribution; we explore this below.
Fully testing this hypothesis would require data identifying the schools that voucher recipients attend pre- and post-random assignment. We lack access to any information identifying the schools that NYCSCSP recipients attended.
MPR discovered after randomization that some families misreported their family size and were placed into the wrong strata. The initial sample weights corrected for the revised sample sizes in the strata. The corrected weights return the families to their originally assigned strata from the point of randomization. Krueger and Zhu (Citation2004a) discovered that the baseline weights did not correctly adjust for the size of the underlying assignment strata. These weights were revised to include poststratification adjustments, which eliminated previously identified baseline test score differences between the treatment and control groups (see p. 663 for a detailed discussion).
NPR scores are calculated from raw scores which are then normed based on grade and quarter of the school year (fall, winter, or spring) and converted into rankings as a percentile of the national distribution based on the normed sample of the ITBS. This allows for cross-age and cross-grade comparisons of scores.
According to the ITBS website from the publisher, Riverside Publishing, and confirmed through telephone communication with customer support, students are given tests of increasing difficulty depending on age and skill level in timed sessions that do not exceed 30 min. Raw scores are calculated from each test level. Although the total number of questions varies somewhat by level, the highest possible raw score in reading at any level is 44 and the highest possible raw score in math is 50 (Hoover, Dunbar, & Frisbie Citation2013).
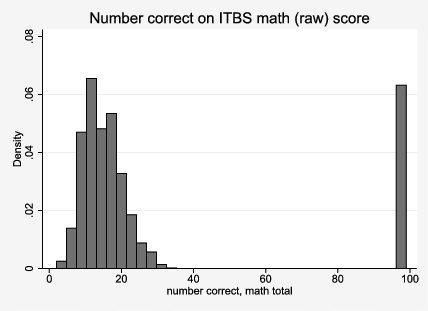
Both Krueger and Zhu (Citation2004a) and Mayer et al. (Citation2002) identified that many students received an NPR score of 0. Neither points out that this score corresponds to a raw score of 99. (See Mayer et al., Citation2002, p. 32, footnote 10; students with a score of 0 were included in the generation of composite scores.) Page 32 also suggests that they include NPR scores ranging from 0 to 100. See also endnote 4 in Krueger and Zhu's replication and extension of Mayer et al.'s finding, which identifies the large concentration of scores of 0 that are included in the analysis while suggesting that these are not valid scores.
Note that the number of observations overall and in the treatment and control groups by year with valid scores, detailed data regarding attrition, missing scores, and invalid 99 scores are reported in the appendix Tables 1 and 2.
We have also estimated two stage least squares (2SLS) estimates of the overall effect of private school attendance on math scores. Because of the missing data on whether control group members attended private schools, we compute the instrumental variables estimates using several assumptions about their public versus private school attendance—we find zero impact of private school attendance across the board. Table 2 in the appendix reports the number of observations in each group that reported attending public or private school, or, in the case of the control group, did not report what kind of school they attended. We treated the missing data in three ways. First, we dropped control observations not reporting the type of school they attended from our 2SLS estimates. Second, we estimated 2SLS assuming that all control students missing this variable actually attended private school. Third, we estimated 2SLS assuming the opposite—that no such children attended private school. All three approaches yielded instrumental variables impacts that were indistinguishable from zero.
For the ECLS-K, we constructed the low-income public school distribution so as to best match the sample of children in the voucher experiment while still having sufficient sample size. The voucher children are all eligible for free lunch. Our comparison children are either obtaining free lunch or on welfare or under poverty (the closest proxy in the public use ECLS-K data to being under 130% of the poverty guideline and thus free lunch eligible). The ECLS-K scores are for spring of first and third grade, about midway between the grades at which baseline voucher scores were collected, which are Grades 1 to 4.
![Figure 7 Histogram of scores falling at various points in the overall national distribution for a national sample of low-income children in public schools (Early Childhood Longitudinal Study, Kindergarten Cohort [ECLS-K] Spring 1st and 3rd), all children in Catholic schools (ECLS-K Spring 1st and 3rd), and New York City School Choice Scholarships Program (NYCSCSP) children at baseline in 1st, 2nd, 3rd, and 4th. Note. This figure shows histogram of percentiles of from the ELCS-K overall public school distribution among poor kids (income low enough for free lunch or on welfare) and for all Catholic school attendees in spring of first and third grades and well as percentiles of the National Percentile Ranking from the ITBS for the pretests for Grades 1, 2, 3, and 4 from the New York City School Choice Scholarships Program evaluation conducted by Mathematica Policy Research. Baseline scores unavailable for kindergarten students in NYCSCSP and scores for end of second grade unavailable in the ECLS K. Statistics weighted to reflect nonresponse and complex sampling.](/cms/asset/df7e33f9-a7bf-4599-b880-15eb7c801f93/uree_a_921259_f0007_b.gif)
The recoding described in Krueger and Zhu provides some contradictory information about which cases were recoded. In the text, it suggests that students were recoded if (a) their mother listed her race as African American in a subsequent wave, (b) if the father listed his race as African American in the baseline wave, and (c) if a parent indicated that his or her race was “other” and wrote in an entry that included the words Black or African American in combination with something else or abbreviated in an obvious manner. In a footnote, they suggested this recoding occurred only if the mother, not the father, used a write-in response. To match their sample sizes as closely as possible, we used only the mother's write-in responses. If the father's write-in responses were included, the sample size was too large. Given that we do not know exactly which write-in cases for either the mother or the father were recoded, our replication of the coefficients in this table is not exact. Their coefficients and standard errors for the alternative version of African American subgroup including the full sample and controls for randomization block presented in Table 5, Panel 2, for reading are 1.36 (1.82) in Year 1, 1.57 (1.81) in Year 2, and 0.99 (1.84) in Year 3; for math they are 3.34 (1.63) in Year 1, 1.15 (1.93) in Year 2, and 3.04 (1.85) in Year 3.