ABSTRACT
To plan group-randomized trials where treatment conditions are assigned to schools, researchers need design parameters that provide information about between-school differences in outcomes as well as the amount of variance that can be explained by covariates at the student (L1) and school (L2) levels. Most previous research has offered these parameters for U.S. samples and for achievement as the outcome. This paper and the online supplementary materials provide design parameters for 81 countries in three broad outcome categories (achievement, affect and motivation, and learning strategies) for domain-general and domain-specific (mathematics, reading, and science) measures. Sociodemographic characteristics were used as covariates. Data from representative samples of 15-year-old students stemmed from five cycles of the Programme for International Student Assessment (PISA; total number of students/schools: 1,905,147/70,098). Between-school differences as well as the amount of variance explained at L1 and L2 varied widely across countries and educational outcomes, demonstrating the limited generalizability of design parameters across these dimensions. The use of the design parameters to plan group-randomized trials is illustrated.
No school is like any other. Schools differ, for example, with respect to the sociodemographic composition of the student body but also with respect to vital educational outcomes such as students' achievement, learning-related motivation and affect, and learning strategies. But how large are these between-school differences? Do differences in educational outcomes also hold when the sociodemographic composition of the student body is taken into account? And are these differences the same in each country, or do they vary across countries? The answers to these questions provide vital design parameters that educational researchers need to plan intervention studies where educational treatments will be randomized at the school level, so-called group-randomized or cluster-randomized trials (Hedges & Hedberg, Citation2007). Group-randomized trials are particularly important for the development of evidence-based educational policies because they allow strong causal inferences to be made about whether educational treatments work on a larger scale (Institute of Education Sciences & National Science Foundation, Citation2013; Slavin, Citation2002; Spybrook, Westine, & Taylor, Citation2016). For instance, the group-randomized trials by Gersten et al., (Citation2015) and Gaspard et al. (Citation2015) are excellent examples of large-scale interventions that foster students' achievement and achievement motivation, respectively.
Relative to the field of medicine with more than 1,000 group-randomized trials conducted so far (see Ivers et al., Citation2011, ), the number of group-randomized trials in education (referring to K-12 students) is relatively small. More specifically, a search in the Education Resources Information Center (ERIC) identified 167 group-randomized intervention studies, most of which were conducted in the United States on students in secondary education (see ). Most of these studies were related to health, a smaller number to achievement, and only a few to students' motivation. This pattern of results is (at least somewhat) surprising for two reasons. First, the demand for rigorous research that can help to develop evidence-based educational policies and knowledge-based innovations in education is not tied to the United States but is actually worldwide (Organisation for Economic Co-operation & Development [OECD], Citation2007; Slavin, Citation2002; Spybrook, Shi, & Kelcey, Citation2016). Second, many educational interventions (e.g., whole-school reforms; see Cook, Murphy, & Hunt, Citation2000; West et al., Citation2015) may affect several outcomes. Thus, evaluations that are aimed at drawing a differentiated picture of intervention effects as well as of mediating causal mechanisms are needed to assess a broad range of educational outcomes (Lipsey & Cordray, Citation2000). Specifically, students' learning-related affect (e.g., enjoyment or anxiety) and motivation (e.g., interests, values, or self-concepts) influence their choice of learning environments as well as the persistence and effort they invest in learning (Eccles & Wigfield, Citation2002). Further, (meta-cognitive) learning strategies are supposed to affect the quality of learning outcomes (Boekaerts, Citation1996; Hattie, Biggs, & Purdie, Citation1996). Hence, given their pivotal role in student learning, these student characteristics are considered vital educational outcomes in addition to achievement or health (Durlak, Weissberg, Dymnicki, Taylor, & Schellinger, Citation2011; OECD, Citation2004; Wang, Haertel, & Walberg, Citation1993).
Figure 1. Results from a review of the literature: (a) ERIC literature search on the number of cluster-randomized intervention studies in education and (b) distribution of between-school differences in achievement in the United States by domain and grade level as reported in previous research. Notes. The literature search for was performed in ERIC on April 28, 2017. We used the search string (“cluster randomized” or “group randomized”) in conjunction with the operator AND to identify K-12 students with the term LV(Elementary Secondary Education) or students in secondary education with the term LV(Secondary Education), respectively. To obtain more information on the field of study, we added either “health,” “achievement,” or “motivation” with the operator AND to the search string. We used the “Intervention” descriptor and “Foreign Countries” descriptor to limit the search results to intervention studies and studies conducted outside the United States, respectively. In , the black diamonds represent the median values of each distribution. The distributions in mathematics/reading/science are based on 34/34/3 values for elementary students (Grades K-6), 8/6/3 values for middle school students (Grades 7–8), and 12/7/4 values for high school students (Grades 9–12), respectively. Most values for ρ are based on two-level hierarchical linear models (schools at Level 2 and students at Level 1) as reported in the following studies: Bloom et al. (Citation2005), Hedges and Hedberg (Citation2007, Citation2013), Schochet, (Citation2008), Spybrook et al. (Citation2016), Westine et al. (Citation2013), and Zhu et al. (Citation2012). Only the study by Jacob et al. (Citation2010) reported ρ on the basis of a three-level hierarchical linear model (schools at Level 3, classes at Level 2, and students at Level 1); their study contributed two values to this figure (mathematics and reading achievement in Grade 3). When reported, we used values of ρ that were averaged across several states (Hedges & Hedberg, Citation2013) or districts (Bloom et al., Citation2005).
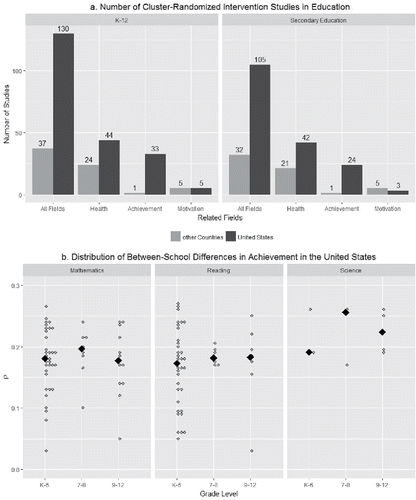
It is important to mention that most previous research on design parameters has offered these parameters for U.S. student samples and for achievement as the outcome (see, e.g., Bloom, Richburg-Hayes, & Black, Citation2007; Hedges & Hedberg, Citation2007, Citation2013; Schochet, Citation2008; Spybrook et al., Citation2016). The overarching goal of this paper is therefore to expand this body of knowledge in three major directions: We examine design parameters (a) at an international level with data from 81 countries, (b) for adolescent students' achievement, motivation, affect, and learning strategies, and (c) to learn the extent to which findings on design parameters generalize across countries and outcome measures. In doing so, we provide necessary information to educational researchers around the world to plan new group-randomized interventions targeting a broad range of educational outcomes.
Between-School Differences: Measures and Study Planning
When planning group-randomized trials, researchers need design parameters that provide information about between-school differences as well as the amount of variance that can be explained at the school level and the individual student level by means of vital covariates, including sociodemographic characteristics (Bloom, Citation2006; Hedges & Hedberg, Citation2007; Raudenbush, Martinez, & Spybrook, Citation2007). Between-school differences are typically measured by the intraclass correlation ρ:(1) where
represents the true variance observed between schools (e.g., achievement differences between schools at the mean level), and
represents the variance observed between students within schools (e.g., achievement differences between individual students within schools). The total variance between individual students (i.e.,
) is the sum of the between-school and within-school variances:
(2)
The intraclass correlation varies between 0 and 1. A value of ρ = 0 implies that there are no between-school differences on a particular outcome measure; the total variance in the outcome is among individuals within schools. A value of ρ = 1, on the other hand, implies that students within a certain school do not show any individual differences; the total variance in the outcome is between schools.
Estimates for and
can be obtained from hierarchical linear models where the variance of an educational outcome Yij is decomposed into the variance of individual students i located at Level 1 (L1) and the variance between schools j at Level 2 (L2).
(3)
(4) where eij represents the residual term of student i in school j (which is assumed to be normally distributed with mean zero and variance
), β0j represents the intercept of school j, γ00 represents the grand mean of the outcome Yij, and uj represents the residual term of school j (which is assumed to be normally distributed with mean zero and variance
).
Of note, the present article deals with between-school differences. In other words, this article does not provide, for example, variance estimates for mean-level differences between classes in the same school or differences between districts. Variance decomposition as well as variance estimates for these more complex multilevel designs can be found in Bloom et al. (Citation2008), Schochet (Citation2008), Spybrook and Raudenbush (Citation2009), and Hedberg and Hedges (Citation2014). It is notable that Zhu, Jacob, Bloom, and Xu (Citation2012) showed that ignoring the class level in analyses hardly affects the planning or the analysis of two-level intervention studies with students at L1 and schools at L2.
As noted above, the values of ρ are a vital element in the planning of group-randomized trials: ρ enters the computation for the minimally detectable effect size (MDES; Bloom, Citation1995) because it determines (among other parameters) the precision of estimates of educational intervention effects (i.e., their standard error). A nontechnical description by Jacob, Zhu, and Bloom (Citation2010), p. 165) conceives of the MDES as the smallest (standardized) intervention effect that a study with a certain sample size can detect with confidence. More technically, by convention, the MDES is defined as the smallest intervention effect that has an 80% probability of being detected (i.e., statistical power is .80) with a two-tailed testing procedure and a level of statistical significance (an alpha level) of .05 (Bloom, Citation1995; Hedges & Hedberg, Citation2007; Jacob et al., Citation2010; Schochet, Citation2008). Values of the MDES can be interpreted as any standardized effect size measure with the MDES standardized with reference to the total student-level standard deviation in the outcome (Jacob et al., Citation2010, p. 166). For example, an MDES of 0.25 represents a treatment effect of one quarter of a student-level standard deviation (see Bloom et al., Citation2007, p. 34). When no covariates are used and when educational interventions are assigned at the school level, the MDES of a group-randomized trial is computed as follows (Bloom, Citation2006, Equation 20):(5)
In Equation Equation5(5) , J represents the total number of schools randomized to treatment or control status; MJ-2 represents a multiplier that is based on t distributions specific to the chosen value of statistical significance and power for J - 2 degrees of freedom (see Schochet, Citation2008, Table 1 for MJ-2 as computed for various degrees of freedom), P represents the proportion of schools randomly assigned to the educational intervention, and n represents the harmonic mean of the number of students per school. Of note, M J-2 equals approximately 2.8 when 20 or more schools are randomly assigned to the educational intervention and when 20 (different) schools are assigned to the comparison group, yielding J = 40 (Bloom et al., Citation2008, p. 24; Schochet, Citation2008, Table 1).
Table 1. Description of the PISA data: Number of participating countries, students, and schools.
Equation Equation5(5) shows that (everything else being equal) larger values of the intraclass correlation are associated with larger values of the MDES. Thus, to increase the precision of a study and to decrease the MDES, smaller values of the intraclass correlation are desirable. This can be achieved by using covariates to explain variance between or within schools. The proportion of explained variance
at L1 and
at L2 (each having a range from 0% to 100%) is computed as:
(6)
(7) with
as the covariate-adjusted within-school variance at L1 and
as the covariate-adjusted between-school variance at L2.
Further, using covariates also yields an adjusted MDESadj that is (typically) smaller in size than the MDES that is obtained without covariates (Bloom, Citation2006, Equation 21).(8) where g* represents the number of group-level covariates used. Of note, the number of individual-level covariates does not enter into the degrees of freedom computation for the multiplier
(Bloom, Citation2006, p. 16).
Design Parameters to Plan Educational Intervention Studies
The Need For Appropriate Normative Distributions
When planning a new intervention study, Equations Equation5(5) and Equation8
(8) provide important insights. Specifically, once the desired level of MDES or MDESadj is determined (see the Applications section), researchers need to decide which values of ρ,
, and
should be entered into these equations to determine the required sample size. One good approach is to use existing estimates of these design parameters from a similar research context (i.e., similar outcome measures and target populations). To judge what constitutes a small, medium, or large value of a certain design parameter, researchers can draw on (so-called) normative distributions that summarize the values of design parameters as obtained in past research (see Cohen, Citation1988, pp. 12-13, 534; Lipsey et al., Citation2012, p. 4).
Empirical Results On Design Parameters
Several studies have contributed to the empirical knowledge base on normative distributions of design parameters. Given the research focus of the present study, we summarize the results for ρ, , and
as obtained for two-level models (with schools at L2 and students at L1) and when sociodemographic covariates are used. We focus on sociodemographic covariates because data for these covariates can be relatively easily obtained in cross-sectional research designs, whereas data for pretests, for example, require longitudinal designs. Using this focus, the extant body of research on design parameters for educational outcomes can be summarized as follows.
First, most previous studies have analyzed design parameters for domain-specific achievement as measured by standardized tests. We are aware of only a single systematic analysis of between-school differences in students' (self-reported) motivation: Martin, Bobis, Anderson, Way, and Vellar (Citation2011) investigated between-school differences in students' mathematics motivation and engagement, drawing on student self-report data from 47 Catholic schools in Australia with students attending Grades 6, 7, and 8. Between-school differences (ρ) in their study varied from .00 to .03. These between-school differences were not further reduced when the authors adjusted for sociodemographic covariates. It is notable that a few other studies have also examined outcomes other than achievement. For example, Hedberg (Citation2016) provided design parameters for teacher reports of kindergarten children's learning-related or general behaviors, and Jacob et al. (Citation2010) provided design parameters for parent and teacher reports of elementary school students' emotional and behavioral outcomes. Information on design parameters for health-related outcomes can be found in Murray, Varnell, and Blitstein (Citation2004, Table 1), and Jacob et al. (Citation2010).
Second, most studies on between-school differences in achievement have been based on student samples from the United States (Bloom, Richburg-Hayes, & Black, Citation2005; Hedges & Hedberg, Citation2007, Citation2013; Jacob et al., Citation2010; Schochet, Citation2008; Spybrook et al., Citation2016; Westine, Spybrook, & Taylor, Citation2013; Zhu et al., Citation2012). shows the distribution of ρ as reported in these studies. Despite some expected variation of ρ across studies or samples, grade levels, and achievement domains, the results of previous research indicate that the variance in between-school differences in achievement in the United States is typically less than .25 (i.e., ρ < .25) for (a) all achievement domains and (b) grade levels. With one exception, all median values were about ρ = .20 (+/−.02).
Third, only a few studies systematically investigated between-school differences in achievement at an international level (e.g., Kelcey, Shen, & Spybrook, Citation2016; Zopluoglu, Citation2012). The results of these international studies clearly show that between-school differences in achievement can vary widely across countries. Drawing on representative national samples of sixth graders in 15 sub-Saharan African countries, Kelcey et al. (Citation2016) found that the range of between-school differences was .08 ≤ ρ ≤ .60 (Mdn ρ = .30) in reading achievement and .08 ≤ ρ ≤ .55 (Mdn ρ = .26) in mathematics achievement. Drawing on representative national samples of students attending Grade 4 or Grade 8, Zopluoglu's (Citation2012) results also empirically underscored the wide range of between-school differences in reading, mathematics, and science achievement across countries at both grade levels. For example, in Grade 8, the range of between-school differences across 57 countries was .08 ≤ ρ ≤ .60 (average ρ = .31) in mathematics achievement and .06 ≤ ρ ≤ .61 (average ρ = .29) in science achievement.
Fourth, when sociodemographic covariates have been used, design parameters are again available mostly (a) for achievement measures and (b) from studies conducted in the United States (Bloom et al., Citation2005; Hedges & Hedberg, Citation2013; Spybrook et al., Citation2016). At all grade levels, a substantial part of between-school differences in achievement can be explained by mean differences in students' sociodemographic characteristics between schools: Median values of were Mdn
= .64 for reading achievement, Mdn
= .54 for mathematics achievement, and Mdn
= .71 for science achievement. At the individual student level, sociodemographic characteristics explained considerably smaller proportions of variance: Median values of
were about Mdn
= .10 for all three achievement domains. This general pattern of results was also supported by Kelcey et al. (Citation2016) but with considerable heterogeneity across sub-Saharan countries. When they adjusted reading achievement for students' socioeconomic status by applying a composite measure of socioeconomic status,
ranged from 0 to .13 with a median value of Mdn
= .02 (mathematics achievement: range: 0 ≤
≤ .14 with Mdn
= .01), whereas values of
ranged from .06 to .74 with a median value of
= .45 (mathematics achievement: range: 0 ≤
≤ .68 with Mdn
= .36).
The Present Study
To determine the sample size needed for group-randomized trials, researchers need (normative distributions of) design parameters that approximate key characteristics of the target student populations and educational outcomes under investigation (Lipsey et al., Citation2012). Our literature review of extant studies on design parameters showed that: (a) We have limited knowledge about between-school differences in vital educational outcomes other than achievement, including students' learning-related affect and motivation as well as their learning strategies, (b) The extent to which sociodemographic covariates help reduce between-school differences in students' affective and motivational student characteristics or learning strategies is a relatively open question, and (c) There is scarce empirical knowledge on the extent to which findings on the amount of variance explained by sociodemographic covariates at the student or school levels generalize to countries other than the United States or sub-Saharan African countries. This paper aims to fill these gaps by analyzing between-school differences in adolescent students' achievement, motivation, affect, and learning strategies for 81 different countries. We also provide examples of the application of these design parameters under various planning scenarios.
Method
Sample and Procedure
The data used in this study were obtained from the Programme for International Student Assessment (PISA) conducted by the OECD. PISA is a triennial international survey that assesses the achievement of 15-year-old students at the end of compulsory education. Students from OECD and non-OECD countries or economic regions can participate in PISA. (For ease of presentation, we refer to both “countries” and “economic regions” as “countries” in this article). We reanalyzed data from the 2000, 2003, 2006, 2009, and 2012 cycles of PISA. PISA sets high-quality standards for collecting representative probability samples (OECD, Citation2014c). Specifically, at least 4,500 students in each country participated in each PISA cycle, or the full student population was included if it was smaller than this size. To this end, most countries applied a two-stage sampling design in all PISA cycles. At the first sampling stage, individual schools with 15-year-old students were systematically sampled from a stratified list of all schools with sampling probabilities proportional to the number of 15-year-old students enrolled. Strata were specific for each country and included, for example, geographic regions or school type. In each country, a minimum of 150 schools had to be selected; if a country had fewer than 150 schools, all schools were selected. Further, in most countries, around 35 students who were 15 years of age were randomly selected within schools; if a school had fewer than 35 students at age 15, all students in this age group were selected. shows that 1,905,147 students attending 70,098 schools in 81 different countries participated in the PISA cycles from 2000 to 2012. The number of countries varied between PISA cycles, with a minimum of 41 countries in PISA 2003 and a maximum of 74 countries in PISA 2009.
Measures
All PISA measures (i.e., standardized tests and self-report instruments) were developed on the basis of advice from substantive and statistical expert panels and the results of extensive pilot studies.
Sociodemographic Covariates
Information on students' gender, immigration background, and socioeconomic background was collected by means of a student questionnaire. Immigration background was determined by asking each student about their own country of birth as well as that of their mother and father. An (interval-scaled) index on students' socioeconomic background summarized rich information on parents' education, occupational status, wealth, and cultural possessions (e.g., OECD, Citation2009).
Achievement
Each PISA cycle focused on one of three domains: mathematics (PISA 2003 and 2012), reading (PISA 2000), and science (PISA 2006). Standardized achievement tests (comprising multiple-choice as well as closed- and open-constructed response items) were administered to assess students' proficiency in applying their domain-specific knowledge and skills to solve problems of varying complexity (see OECD, Citation2014b, for sample test items). Students worked on paper-and-pencil tests from all three domains in every PISA cycle. To this end, in each PISA cycle, a so-called multimatrix design was applied where one of several test booklets was randomly assigned to students; test booklets varied in the test domains that were covered with most test items measuring the domain of focus (e.g., OECD, Citation2014c). The number of available data points on students' achievement varied somewhat between domains (see ) due to the treatment of planned missing data as implied by the multimatrix designs. Specifically, in PISA 2000 (in contrast to all later PISA cycles), domain-specific achievement scores were estimated only when students worked on test booklets containing test items from that domain. In later PISA cycles, achievement scores were estimated for all students in all domains (e.g., OECD, Citation2014c). The achievement scores demonstrated at least satisfactory levels of internal consistency (see Table S1 in the supplementary material): The average/median reliability estimates ranged from .78 (science achievement; PISA 2000) to .92 (mathematics achievement; PISA 2012).
Table 2. Between-school differences in educational outcomes by constructs and domains: Distribution of intraclass correlations (ρ) and variance explained by sociodemographic characteristics at the individual student () and school level (
).
Affect and Motivation
The self-report scales for affective and motivational outcomes (see Table S1 for example items) were used to assess constructs that were related to students' motivation and drive (e.g., perseverance, openness, interest, and instrumental motivation to learn), the beliefs they held about themselves as learners (e.g., self-efficacy, self-concept), as well as their affective experiences (e.g., enjoyment, anxiety) while learning in each domain (OECD, Citation2014a). We examined a total of 26 different scales. Except for PISA 2000, we used scales that assessed educational outcomes in the focus domain only or domain-general outcomes. In contrast to previous PISA cycles where students worked on the same questionnaire form, PISA 2012 implemented a multimatrix design for the student questionnaire: Students worked on one of three questionnaire booklets; the booklets were randomly assigned to students and varied with regard to the constructs included. The scale scores of motivational and affective measures demonstrated at least acceptable levels of internal consistency (Table S1): The average/median reliability estimates ranged from .66 (“Attribution of Failure” in mathematics; PISA 2012) to .92 (“Enjoyment” in science; PISA 2006).
Learning Strategies
Self-report scales for learning strategies were used to assess how students study mathematics or how they learn in general (OECD, Citation2004). Distinctions were made between three strategies: elaboration, memorization, and control strategies, with the last one referring to how students manage their learning (see Table S1 for example items). The scale scores for learning strategies demonstrated at least acceptable levels of internal consistency (Table S1): The average/median reliability estimates ranged from .60 (“Memorization” in mathematics; PISA 2003) to .77 (domain-general “Elaboration”; PISA 2000).
Statistical Analyses
To determine the various design parameters, we used multilevel models (as specified in Equations Equation3(3) and Equations Equation4
(4) ) as well as a sociodemographic covariate model (as specified in Equation9
(9) and Equation10
(10) ) that we adapted from the seminal study by Hedges and Hedberg (Citation2007) for each educational outcome in a certain country and a certain PISA cycle. More specifically, the sociodemographic covariate model that we used in this study included indicator variables representing gender G (0 = girls, 1 = boys), status as a student with a first-generation immigration background IM1 (0 = otherwise, 1 = students and parents born outside the country of assessment), status as a student with a second-generation immigration background IM2 (0 = otherwise, 1 = child born in country of assessment with parent(s) born in a foreign country), and the index representing students' socioeconomic status S. Further, each variable at L1 was group-mean centered, where
,
,
, and
represent the respective mean values of these covariates in school j.
(9)
(10)
We used Mplus 8 (Muthén & Muthén, Citation2017) to test the multilevel models; we used R (R Core Team, Citation2017) for all remaining analyses and the R package ggplot2 (Wickham, Citation2009) to create and . In the multilevel analyses, we applied weights at both L1 and L2 to account for factors (at both levels) that led to unequal inclusion probabilities of students in the national samples. To this end, we followed the PISA analyses on school effectiveness (OECD, Citation2013, p. 221) and used the student final weights (W_FSTUWT). Specifically, the student final weights at L1 were rescaled so that, within each school, they would sum to the school sample size (Mplus command: “WTSCALE = CLUSTER”). The weights at L2 were computed as the sum of student final weights within each school. In the analyses, these weights were then rescaled so that the product of the between and the within weights summed to the total sample size (Mplus command: “BWTSCALE = sample”; Muthén & Muthén, Citation2017). When the same measures (e.g., achievement tests in mathematics) were applied in several PISA cycles (see Table S1), we followed Spybrook et al. (Citation2016) and computed country-specific values of a certain statistical parameter (i.e., ρ, , and
) and the corresponding standard errors by averaging the country-specific values across PISA cycles. A small proportion of values for
(1%) and
(3%) were negative. This could be the result of estimation error, particularly when the estimate of the between-school variance
was close to zero (see Jacob et al., Citation2010, p. 177). Following Hedges and Hedberg (Citation2013, p. 471), we truncated these negative values to zero in further analyses. To provide some empirical guidelines for what might constitute “small,” “medium,” and “large” values of a certain design parameter, we adopted the approach taken by Hemphill (Citation2003) and Bosco, Aguinis, Singh, Field, and Pierce (Citation2014): We computed the lower, middle, and upper thirds of each normative distribution, with the lower third comprising values up to the 33rd percentile, the medium third comprising values from the 33rd percentile to the 66th percentile, and the upper third comprising values above the 66th percentile.
Figure 2. Between-school differences in educational outcomes by construct clusters and domain: distribution of intraclass correlations (ρ). Notes. Affective Motivational Constructs: General: 1 = Control Expectations, 2 = Effort, 3 = Instrumental Motivation, 4 = Openness, 5 = Perseverance, 6 = Self-Concept, 7 = Self-Efficacy. Mathematics: 8 = Anxiety, 9 = Attribution of Failure, 10 = Intentions Future Career, 11 = Instrumental Motivation, 12 = Interest, 13 = Self-Concept, 14 = Self-Efficacy, 15 = Work Ethic. Reading: 16 = Enjoyment, 17 = Interest, 18 = Self-Concept. Science: 19 = Enjoyment, 20 = Future Orientation, 21 = General Value, 22 = Instrumental Motivation, 23 = Interest, 24 = Personal Value, 25 = Self-Concept, 26 = Self-Efficacy.
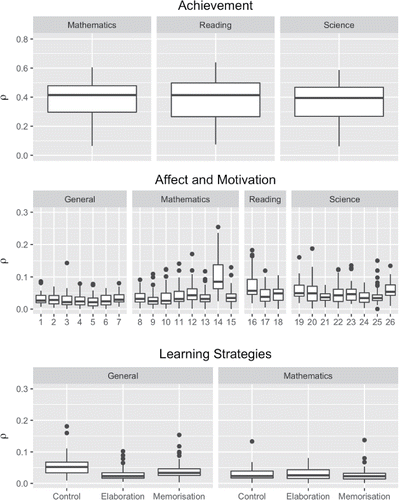
Results
In this section, we present normative distributions for the design parameters as obtained for each educational outcome ( and ). We further aggregated the results across specific measures to obtain normative distributions for three broad construct clusters (): (a) achievement, (b) motivation and affect, and (c) learning strategies. The distributions that underlie the results in are broken down for each of the 81 countries in Table S2 in the online supplementary materials. Furthermore, Table S3 in the online supplement presents the country-specific distributions of all design parameters for the three construct clusters with the distributions based on all available values for a certain country (e.g., Germany participated in five PISA cycles with three values of ρ obtained for achievement per cycle; combining these values across PISA cycles yielded a distribution with a total of 15 values for the distribution of ρ for achievement). In Tables S2 and S3, country names were coded according to the ISO 3166-1 ALPHA-3 classification, with a few exceptions (see and OECD Citation2014c, p. 399).
Table 3. Between-school differences in educational outcomes by construct clusters: Distribution of intraclass correlations (ρ) and variance explained by sociodemographic characteristics at the individual student () and school level (
).
Achievement
We observed considerable between-school differences in student achievement, with highly similar results obtained for mathematics, reading, and science ( and ): Median values of ρ were around ρ = .40 in all three domains, with considerable variation in between-school differences across countries. For example, between-school differences in mathematics varied from ρ = .06 (observed in Finland, see Table S2) to ρ = .61 (observed in the Netherlands). When we aggregated across domains, we found a normative distribution of between-school differences in achievement that was based on a total of k = 243 values (). Between-school differences in achievement up to ρ = .33 were located in the lower third of the distribution, the range .33 < ρ ≤ .46 constituted the middle third, and values of ρ > .46 comprised the upper third. Sociodemographic characteristics explained a substantial part of the variance in between-school differences and a smaller proportion of the variance in within-school differences in most countries. This pattern of results was similar in all three domains, yet the amount of variance explained at L1 and L2 varied considerable across countries (see , , and S2).
Affect and Motivation
Compared with the achievement measures, between-school differences in the scales measuring students' affect and motivation were much smaller ( and ). Median values of ρ varied from .02 (e.g., general openness) to .08 (mathematics self-efficacy). We also found some (but compared with the achievement measures, considerably less) variation between countries (see Table S2). When we aggregated across the affect and motivation measures (k = 1,454; ), the between-school differences in students' affect and motivation up to ρ = .03 were located in the lower third of the distribution, the range .03 < ρ ≤ .05 constituted the middle third, and values of ρ > .05 comprised the upper third. For all measures of students' affect and motivation, we found that sociodemographic characteristics explained a larger proportion of the variance in between-school differences and a smaller proportion of the variance in within-school differences in most countries (see , , and S2).
Learning Strategies
The pattern of results obtained for students' learning strategies was quite similar to that obtained for measures of students' affect and motivation. As shown in and , median values of ρ varied from .02 (e.g., memorization in mathematics) to .05 (general control). We also found some variation between countries (see Table S2). When aggregated across measures (k = 348, ), between-school differences in students' learning strategies up to ρ = .02 were located in the lower third of the distribution, the range .02 < ρ ≤ .04 constituted the middle third, and values of ρ > .04 comprised the upper third. Again, sociodemographic characteristics explained a larger proportion of the variance in between-school differences for all measures of students' learning strategies and a smaller proportion of the variance in within-school differences in most countries (see , , and S2).
Applications
In this section, we demonstrate various strategies for how the design parameters and their normative distributions can be used to plan group-randomized trials where educational treatment is assigned at the school level. We provide examples of these strategies under three scenarios targeting students' achievement, affect, and motivation as educational outcomes.
Setting the Desired Level of the Minimally Detectable Effect Size
When planning the sample size of a new group-randomized intervention study, one of the major challenges is to set the desired level of MDES. To this end, researchers ideally take into account several perspectives (Bloom, Citation2006, p. 7; Schochet, Citation2008, pp. 64–67). First, from an economic perspective, the (monetary) benefits associated with the proposed effect of the educational intervention should outweigh the costs of the intervention itself (see Schochet, Citation2008, p. 66, for an example).
Second, from a political perspective, the chosen MDES should be considered to be policy-relevant. One way to address its policy-relevance is to compare the desired MDES with differences (Δ) between weak and average schools (with Δ being standardized in terms of the student-level standard deviations). For example, in the study by Hill, Bloom, Black, and Lipsey (Citation2008, Table 5), the performance gaps between schools ranged from .07 ≤ Δ ≤ .43 (Mdn Δ = .25) in reading achievement to .14 ≤ Δ ≤ .41 (Mdn Δ = .23) in mathematics achievement. An expected standardized effect size (ES) of the intervention of ES = .25 would close the typical (i.e., median) achievement gap between weak and average schools. Thus, an ES of .25 but even smaller ESs (in the range .10 ≤ ES ≤ .20) may be relevant to policymakers (see Bloom, Citation2006, p. 7; Bloom et al., Citation2007, p. 39; Schochet, Citation2008, pp. 64–67). It is notable that an ES benchmark has yet to be established for students' motivation (e.g., in terms of mean-level differences between schools).
Third, from the perspective of an accumulated body of research evidence, the expected effects of the target intervention should lie in the range of what is known from previous research with similar interventions. To this end, researchers can draw on meta-analyses to determine the typical (e.g., mean or median) standardized ES estimates of educational interventions. When doing so, they should consider a few issues: (a) If the implementation dosage of the target intervention (e.g., the duration of the treatment) is stronger or weaker than that reported in previous research, stronger but also weaker effects of the target intervention should be expected. (b) Recall that the MDES is defined in terms of the total student-level standard deviation of the outcome (Jacob et al., Citation2010, p. 166). It is notable that other standardization methods for computing the ES can be found in the literature (Olejnik & Algina, 2000), for example, standardization with respect to the standard deviation in the control group or with respect to the pooled standard deviation across the experimental and comparison groups. Depending on the extent to which these standard deviations differ from each other, the very same effect (as measured in its original metric) may correspond to different ES values. Unfortunately, most meta-analyses lack sufficient information to derive crosswalks between the ESs that are based on different standardization procedures. When determining the MDES, we therefore recommend that researchers report how the ES in a meta-analysis was computed to make these potential differences transparent. (c) Likewise, when countries differ in the total student-level standard deviation of the outcome measures, the very same effect (as measured in its original metric) as obtained in a certain country translates into different ES values. For this reason, we also report student-level standard deviations for each outcome and each country in Table S2 (which also contains details about their computation) to facilitate cross-country comparisons of ES values when using these outcome measures in intervention studies.
Scenario 1: Required Number of Schools
For all scenarios, we assumed that schools were randomly assigned to conditions: 50% of schools participated in the intervention (i.e., P = 50), and the remaining 50% were in the comparison group. Furthermore, in all scenarios, we applied a two-sided testing procedure (with an alpha level of .05), and statistical power was set to .80. The number of sociodemographic covariates was set to g* = 4 (see Equation Equation7(7) ). We used the software PowerUp! (Dong & Maynard, Citation2013) to examine the sample size requirements or attainable values of the MDES for our scenarios.
Using these parameters, suppose Research Team A from Germany plans an educational intervention study involving a whole-school reform, with an intervention that is aimed at improving 15-year-old students' mathematics achievement. (In the Discussion section, we give some recommendations for strategies that can be used when a country was not included in the present analyses.) The review by Hill et al. (Citation2008, p. 176) showed that the typical standardized ES of educational intervention studies on achievement outcomes lies in the range of .20 ≤ ES ≤ .30 (with the ES defined in terms of the total student-level standard deviation). For planning purposes, Team A considers the various perspectives noted above and uses MDES = .25. Furthermore, Team A decides to sample n = 60 students per school (e.g., because most German schools at the secondary level have at least 60 students who are 15 years of age). It is important to mention that drawing on the paper, by Jacob et al. (Citation2010, p. 184), Team A wants to take into account the statistical uncertainty associated with the estimate of between-school differences in mathematics achievement (i.e., as reflected in the standard error of ρ). In doing so, Team A can obtain lower and upper bound estimates for J given the specified conditions. To this end, Team A computes the 95% confidence interval of ρ by using t distributions with degrees of freedom computed as df = J - 1 (Jacob et al., Citation2010). For example, the point estimate of ρ = .56 for mathematics achievement in Germany with a standard error of 0.024 was based on J = 1,117 schools (Table S2). Using these values, the lower bound of the 95% confidence interval of ρ is computed as .56 − 1.96*0.024 = .51; the upper bound of the 95% confidence interval is .56 + 1.96*0.024 = .61. These design parameters show that Team A needs J = 262 schools when considering the lower bound estimate of ρ; it needs J = 287 for the point estimate and J = 312 for the upper bound estimate. To increase statistical precision, Team A also takes into consideration assessments of students' sociodemographic characteristics: Table S2 shows that in Germany, sociodemographic covariates explain 9% of the variance in mathematics achievement at the student level () and 75% of the variance at the school level (
). Thus, when using the sociodemographic covariate adjustment, the required number of schools decreases considerably: J = 70 schools are needed when considering the lower bound estimate of ρ, J = 76 for the point estimate, and J = 82 for the upper bound estimate. To sum up, to achieve a statistical power of .80 for detecting a typical effect of educational interventions on achievement, Team A should use students' sociodemographic characteristics as covariates and draw a sample comprising (as a conservative upper bound estimate of J) 82 schools (with n = 60 students per school).
Scenario 2: Attainable MDES
In the second scenario, we assume that Research Team B from the United States is planning a group-randomized educational intervention study targeting students' mathematics self-efficacy. Design parameters are reported in Table S2. The research team plans the study with a fixed number of schools (i.e., J = 30 schools with n = 60 students per school), which is quite a typical situation because access to schools can be limited for pragmatic reasons (e.g., only a limited number of schools are within a reasonable distance) or due to budgetary constraints (see Spybrook et al., Citation2016). The primary interest of Team B is in whether the expected MDES lies in the range of the typical intervention effect on students' motivation. The meta-analysis by Lazowski and Hulleman (Citation2016, Table 3) indicated that the typical standardized ES of intervention studies on student motivation is ES = .43 (with experimental designs) and ES = .54 (with self-report measures). Both ES estimates are based on the pooled standard deviation across experimental and control groups. When determining the MDES of their study design, Team B takes into account the statistical uncertainty associated with the point estimate of ρ = .06 (with a standard error of .011; see Table S2) obtained for between-school differences in mathematics self-efficacy. Doing so yields a lower bound estimate of the 95% confidence interval of ρ = .04 and an upper bound of ρ = .08. Using these parameters yields MDES values of .25/.29/.33 for the lower bound, point, and upper bound estimates of ρ, respectively. In addition, assuming the sociodemographic covariate adjustment (with = .09 and
= .62; see Table S2), the estimated MDES values associated with the lower bound, point, and upper bound estimates decrease further, yielding estimates of MDES = .18/.21/.23, respectively. Obviously, the estimated upper bound of the MDES (particularly when assuming a sociodemographic covariate adjustment) is smaller than the typical intervention effect on students' motivation. Thus, Team B can be quite sure that the planned study design is sensitive enough to detect the intervention effect (if one exists).
Scenario 3: Required Sample Size When the Intervention Targets a Broad Range of Educational Outcomes
In the third scenario, we assume that Research Team C wants to examine the effects of a whole school reform that is supposed to affect students' achievement, affect, and motivation in several domains by means of a group-randomized trial (e.g., Cook et al., Citation2000; West et al., Citation2015). To determine the sample size, Team C considers the various perspectives noted above and chooses values of the MDES that are known to be typical for educational interventions (i.e., MDES = .25 for achievement; MDES = .45 for affect and motivation measures). Team C then systematically examines the sample-size requirements (in terms of J) for both educational outcome clusters under several conditions. To this end, Team C consults and uses values for the design parameters (ρ, , and
) that lie in the range of what constitutes “small” (i.e., the 20th percentile), “medium” (i.e., the median), and “large” values (i.e., the 80th percentile). In doing so, Team C obtains information about upper and lower bound estimates of the number of schools that are required to obtain a target MDES for measures of students' achievement or their affect and motivation under several conditions (). For example, when using covariates, sampling n = 30 students within schools, and assuming “small” proportions of explained variance in student achievement at L1 and L2 (i.e.,
= .04, and
= .56), Team C needs between J = 70 schools (with ρ = .25) and J = 121 schools (with ρ = .50). also shows that increasing the number of schools rather than increasing the number of students per school is a (much) more effective way to increase statistical power for intervention studies when the treatment is assigned at the school level (see Bloom, Citation2006, pp. 16–17). As for achievement measures, for example, the total sample size, assuming “small” values of ρ,
, and
, ranges from 70*30 = 2,100 to 62*90 = 5,580 students. When targeting students' affect and motivation, a much smaller number of schools would be needed because the target MDES is larger, and the between-school differences are smaller. It is important to mention that under all conditions, the required sample size for achievement measures is (much) larger than those estimated for measures of affect and motivation. Team C's final decision, then, should focus on the sample size requirements for achievement as these estimates safeguard the MDES set for outcomes measuring students' affect and motivation. When doing so, Team C should balance the required sample size against budgetary constraints, the relative costs of sampling schools versus students, and pragmatic factors (e.g., access to schools). To determine the optimal sample allocation, Team C may use the software Optimal Design (Spybrook et al., Citation2013), which allows the user to combine statistical power analyses with a cost perspective.
Table 4. Number of schools (J) needed to achieve MDES = .25 for achievement and MDES = .45 for affect and motivation with and without a sociodemographic covariate adjustment for “small,” “medium,” and “large” values of design parameters.
Discussion
Results from group-randomized trials provide vital information for evidence-based educational policies and knowledge-based innovations in educational practice. To plan such studies, researchers need design parameters for between-school differences as well as the amount of variance that can be explained at the school or individual student level by means of powerful covariates such as sociodemographic characteristics. Capitalizing on representative samples from 81 countries, this study extended the empirical knowledge base on design parameters in three major directions.
First, we examined students' achievement, learning-related affect and motivation, and learning strategies. Most previous studies have provided design parameters for domain-specific achievement as measured by standardized tests. However, students' learning-related affect (e.g., enjoyment), motivation (e.g., interests), and (meta-cognitive) learning strategies are considered to be vital educational outcomes too (Durlak et al., Citation2011; OECD, Citation2004; Wang et al., Citation1993). Our results showed that between-school differences in achievement were considerably larger than between-school differences in students' affect and motivation or learning strategies: Median values for achievement were around ρ = .40, whereas median values fell between .02 and .08 for affect and motivation and between .02 and .05 for learning strategies. This pattern of results was found for measures in the domains of mathematics, reading, and science, as well as for domain-general measures. The small between-school differences in students' affect and motivation found for many countries in this study are thus well-aligned with the results of previous research (Martin et al., Citation2011). Although small in absolute size, the values of between-school differences in affect and motivation as well as learning strategies can be put into perspective by comparing them to other research fields that have a longer tradition in conducting group-randomized trials. More specifically, the between-school differences that we found for affect and motivation as well as learning strategies in the present study are comparable in size to important public-health-related outcomes (e.g., smoking, drinking, drug abuse), where between-cluster differences typically range from .01 to .05 for clusters such as communities, firms, hospitals, or schools (Bloom et al., Citation2007; Murray & Blitstein, Citation2003).
Second, we investigated the extent to which sociodemographic covariates help to reduce between-school differences in educational outcomes at an international level. Previous research on design parameters when a sociodemographic covariate adjustment was applied has derived knowledge from achievement measures where most student samples came from the United States. This line of research showed that between-school differences in achievement (Hedges & Hedberg, Citation2007; Schochet, Citation2008) were considerably reduced when sociodemographic covariates were controlled for. It is notable that we are aware of only a single study that examined the effects of a sociodemographic covariate adjustment on between-school differences in motivation (Martin et al., Citation2011). This previous study found no further reduction in (the already small) between-school differences. It is important to mention that the present study showed that sociodemographic covariates can reduce between-school differences in all three educational outcomes under investigation but to different degrees and at different levels. Specifically, sociodemographic covariates explained a considerably larger proportion of variance at the school level than at the student level. We found this pattern for all three educational outcome clusters as well as for both domain-specific and domain-general measures. However, the values of and
varied considerably between educational outcomes: The median values of
were .06 for achievement, .03 for affect and motivation, and .02 for learning strategies. The median values of
were .67 for achievement, .34 for affect and motivation, and .31 for learning strategies. Thus, adjusting for sociodemographic covariates has a considerably larger effect on improving statistical precision for achievement measures than for measures of students' affect, motivation, or learning strategies (see also Scenario 3 in the Applications section).
Third, we determined the extent to which findings on design parameters generalize across countries. Previous research on design parameters for achievement measures mostly used student samples from the United States. Furthermore, Zopluoglu (Citation2012) and Kelcey et al. (Citation2016) conducted the largest international studies (so far) on between-school differences in student achievement with student data from up to 57 countries from all over the world and 15 sub-Saharan countries, respectively. However, to date, no study has examined between-school differences in students' affect and motivation or learning strategies at an international level. Except for results from sub-Saharan countries (see Kelcey et al., Citation2016), empirical results on design parameters after sociodemographic covariate adjustment are lacking as well. The present analyses clearly indicated that countries vary considerably in all design parameters (i.e., ρ, , and
) for all educational outcomes—students' achievement, affect and motivation, and learning strategies—as assessed by domain-specific (i.e., mathematics, reading, science) and domain-general measures. Thus, the results obtained for the United States do not generalize well to the large majority of other countries. For example, the 20th percentile of the normative distribution of between-school differences in achievement was .25 (). This value lies at the upper bound of what has been found for between-school differences in achievement in U.S. schools across achievement domains and grade levels (). In other words, this example shows that in about 80% of the countries that were included in the present analyses, between-school differences in achievement were (much) larger than those typically found for schools in the United States. It is notable that the median values for between-school differences in achievement were about ρ = .40 in the present study and thus larger than those reported by Zopluoglu (Citation2012), where mean between-school differences in achievement ranged from .23 to .31. Potential reasons for this discrepancy are manifold. They include the larger number of countries included in the present paper (representing a greater diversity of school systems), differences in the samples (e.g., grade-based sampling in TIMSS and PIRLS vs. age-based sampling in PISA), and differences in the nature of the achievement measures (Klieme, Citation2016; Wu, Citation2010).
Taken together, the present results empirically underscore the importance of the recommendations of leading scholars (see Cohen, Citation1988; Lipsey et al., Citation2012) to qualify the judgment of what constitutes “small,” “medium,” or “large” effects by taking into consideration the research context in question (e.g., target population or educational outcome). For example, conventional guidelines to interpret a value of ρ = .10 as “medium” (see LeBreton & Senter, Citation2008) are not well-aligned with the empirical distributions we obtained for achievement (where ρ = .10 is below the 10th percentile at an international level) or the distributions we obtained for affect and motivation measures (where ρ = .10 is above the 90th percentile). In planning educational intervention studies, educational researchers may therefore benefit considerably from consulting and in this paper as well as the country-specific Tables S2 and S3 in the online supplement.
Limitations and Outlook
This study has several limitations. First, we provided design parameters for the outcome measures applied in PISA for student samples of 15-year-olds, with most students attending either Grade 9 or 10. This has two implications for future applications of these parameters: (a) The present design parameters apply well to outcome measures that are identical or at least highly similar to the PISA measures (see Table S1). To the best of our knowledge, however, no studies have investigated the extent to which design parameters differ when different tests or self-report measures are applied to the very same student population. Given that estimates of students' academic growth depend to some extent on the standardized test that is used (see Bloom, Hill, Black, & Lipsey, Citation2008, Table 1), this suggests that researchers should be cautious when using the present design parameters for planning purposes when outcome measures differ in content from those applied in PISA. (b) As indicated by our own literature review () as well as the study by Hedges and Hedberg (Citation2007), there does not seem to be any strong systematic relation between design parameters and grade level. Yet, it is an open question whether this pattern of results obtained for the United States generalizes to other countries. Thus, the present normative distributions seem most appropriate for 15-year-old students or students in Grades 9 and 10.
Second, the present results were derived from national probability samples. States within countries as well as districts within states may vary in their mean levels of educational outcomes. The between-school differences reported in this paper contain some variance that may be located at those higher hierarchical levels. Thus, the values for ρ that we reported in this paper may be considered upper bound estimates rather than lower bound estimates (see also Hedges & Hedberg, Citation2007, Citation2013).
Third, this paper described the variation in design parameters between countries but did not attempt to explain it. Table S2, which provides all country-specific results, may therefore be a fruitful research source for exploring how characteristics of school systems (e.g., the age when students are sorted into different academic tracks) are linked to the size of between-school differences or the amount of variance in educational outcomes that can be explained by sociodemographic covariates.
Fourth, we provided design parameters for two-level experiments in which students are nested in schools. Given the available data in PISA, it was not possible to provide design parameters that take into account, for example, mean-level differences between classes in the same school (Bloom et al., Citation2008). Yet, as shown by Zhu et al. (Citation2012), even when ignoring this source of variance, the present design parameters are reliable for planning two-level intervention studies where educational treatments are assigned at the school level. Other study designs (e.g., a three-level intervention where students are nested within teachers, teachers are nested within schools, and treatment is assigned to teachers within schools), however, require additional information on the variance decomposition of educational outcomes (e.g., variance between classes within schools; see Jacob et al., Citation2010; Konstantopoulos, Citation2008).
Finally, we provided design parameters on the basis of a sociodemographic covariate model: We showed that sociodemographic covariates are powerful explanatory variables for increasing statistical precision across all educational outcomes (but particularly for achievement) in most countries. However, some studies have found that pretest data have even stronger explanatory power for between-school differences in achievement than sociodemographic covariates in reducing variance at the school and student levels in the United States (Bloom et al., Citation2007; Hedges & Hedberg, Citation2007). In particular, drawing on data from U.S. schools, the study by Bloom et al. (Citation2007) indicated that the increase in statistical precision by the use of pretests (a) is similar in size when pretest data are available at only the school level (compared with pretest data that are available at both the student level and the school level), (b) decreases only slightly with increasing time lags between pretests and posttests, and (c) is considerable even when the pretest and posttest differ in content. Using data from the United States, Hedges and Hedberg (Citation2007) as well as Bloom et al. (Citation2007, Table 6) found that demographic covariates provided (almost) no incremental gain in explaining mathematics achievement or reading achievement. This held true at both the student level and the school level once pretest data were controlled for at these levels. Because pretest data were not available in the PISA data, it was not possible to examine the extent to which these findings from the United States generalize across countries in the present study. Nevertheless, in light of the extant research evidence, we think that researchers are well-advised to aim to use pretest data (particularly at the school level) whenever these scores are available (and when data protection regulations permit). In addition to sociodemographic characteristics or even as their replacement, the inclusion of pretest scores in the analytic model may substantially improve the statistical precision and thus reduce the required number of schools or students or the attainable MDES.
Conclusion and Recommendations
This international study provides reliable design parameters for 81 countries across three vital outcomes (achievement, affect and motivation, and learning strategies) for a broad array of domain-specific (mathematics, reading, and science) and domain-general measures. These design parameters and their (country-specific) standard errors as well as normative distributions represent a rich source for planning two-level group-randomized educational interventions where schools are randomly assigned to experimental conditions. To this end, we provide the following recommendations.
1. | When design parameters are available for a certain outcome and a certain country, researchers may refer to the corresponding design parameters and standard errors that are provided in Table S2. In doing so, they can take into account the statistical uncertainty associated with these parameters to determine upper and lower bound estimates of the sample size that is needed to achieve a certain MDES (see Scenario 1). They can also use this information to determine the attainable MDES that is associated with the planned sample size, for example, whether it lies in the range of typical intervention effects (see Scenario 2). | ||||
2. | When design parameters are available for a certain outcome but not for a certain country, researchers may use one of two strategies: (a) They can use design parameters from the countries reported in Table S2 where the school system is similar in key parameters (e.g., the sociodemographic composition of the student body) to the school system of their home country (see Hedges & Hedberg, Citation2013; Spybrook et al., Citation2016), or (b) They can consult the normative distributions for the outcome-specific design parameters presented in and make informed choices for design parameters that constitute “small,” “medium,” and “large” values to systematically examine the implications for the design of the planned intervention study. | ||||
3. | When the intervention (e.g., a whole-school reform) targets a broad range of educational outcomes in several domains, informed estimates of what constitutes “small,” “medium,” or “large” effects for these outcomes are necessary (see Scenario 3). To this end, we recommend that researchers consult Table S3 when distributions of design parameters are available for the target country and when these distributions are based on a sufficient number of values. Alternatively, when the target country is not included in the present results or when the country-specific distribution of a certain design parameter is based on a rather small number of values, we recommend that researchers refer to . |
In summary, we hope that the design parameters and the strategies for how to apply them that we provided in this paper will help other researchers to plan group-randomized trials that have the potential to provide rigorous evidence for the development of evidence-based educational policies and knowledge-based educational innovations.
Online Supplemental Table S3: Country-Specific Distributions of Design Parameters
Download Zip (54.3 KB)Online Supplement Table S2: Country-Specific Design Parameters
Download Zip (218.8 KB)Table S1. Description of the Educational Outcome Measures
Download MS Word (33.8 KB)References
- Bloom, H. S. (1995). Minimum detectable effects: A simple way to report the statistical power of experimental designs. Evaluation Review, 19(5), 547–556.
- Bloom, H. S. (2006). The core analytics of randomized experiments for social research (MDRC Working Papers on Research Methodology). Retrieved from http://www.mdrc.org/sites/default/files/full_533.pdf
- Bloom, H. S., Hill, C. J., Black, A. R., & Lipsey, M. W. (2008). Performance trajectories and performance gaps as achievement effect-size benchmarks for educational interventions. Journal of Research on Educational Effectiveness, 1(4), 289–328. doi:10.1080/19345740802400072
- Bloom, H. S., Richburg-Hayes, L., & Black, A. R. (2005). Using covariates to improve precision. Empirical guidance for studies that randomize schools to measure the impacts of educational interventions. Retrieved from http://www.mdrc.org/sites/default/files/full_598.pdf
- Bloom, H. S., Richburg-Hayes, L., & Black, A. R. (2007). Using covariates to improve precision for studies that randomize schools to evaluate educational interventions. Educational Evaluation and Policy Analysis, 29(1), 30–59. doi:10.3102/0162373707299550
- Bloom, H. S., Zhu, P., Jacob, R., Raudenbush, S., Martinez, A., & Lin, F. (2008). Empirical issues in the design of group-randomized studies to measure the effects of interventions for children (MDRC Working Papers on Research Methodology). Retrieved from http://eric.ed.gov/?id=ED502531
- Boekaerts, M. (1996). Self-regulated learning at the junction of cognition and motivation. European Psychologist, 1(2), 100–112.
- Bosco, F. A., Aguinis, H., Singh, K., Field, J. G., & Pierce, C. A. (2014). Correlational effect size benchmarks. Journal of Applied Psychology, 100(2), 431–449. doi:10.1037/a0038047
- Cohen, J. (1988). Statistical power analysis for the behavioral sciences. Hillsdale, NJ: Lawrence Erlbaum.
- Cook, T. D., Murphy, R. F., & Hunt, H. D. (2000). Comer's school development program in Chicago: A theory-based evaluation. American Educational Research Journal, 37(2), 535–597. doi:10.3102/00028312037002535
- Dong, N., & Maynard, R. (2013). PowerUp!: A tool for calculating minimum detectable effect sizes and minimum required sample sizes for experimental and quasi-experimental design studies. Journal of Research on Educational Effectiveness, 6(1), 24–67. doi:10.1080/19345747.2012.673143
- Durlak, J. A., Weissberg, R. P., Dymnicki, A. B., Taylor, R. D., & Schellinger, K. B. (2011). The impact of enhancing students' social and emotional learning: A meta-analysis of school-based universal interventions. Child Development, 82(1), 405–432. doi:10.1111/j.1467-8624.2010.01564.x
- Eccles, J. S., & Wigfield, A. (2002). Motivational beliefs, values, and goals. Annual Review of Psychology, 53, 109–132.
- Gaspard, H., Dicke, A.-L., Flunger, B., Brisson, B. M., Häfner, I., Nagengast, B., & Trautwein, U. (2015). Fostering adolescents' value beliefs for mathematics with a relevance intervention in the classroom. Developmental Psychology, 51(9), 1226–1240. doi:10.1037/dev0000028
- Gersten, R., Rolfhus, E., Clarke, B., Decker, L. E., Wilkins, C., & Dimino, J. (2015). Intervention for first graders with limited number knowledge: Large-scale replication of a randomized controlled trial. American Educational Research Journal, 52(3), 516–546. doi:10.3102/0002831214565787
- Hattie, J., Biggs, J., & Purdie, N. (1996). Effects of learning skills interventions on student learning: A meta-analysis. Review of Educational Research, 66(2), 99–136. doi:10.2307/1170605
- Hedberg, E. C. (2016). Academic and behavioral design parameters for cluster randomized trials in kindergarten: An analysis of the early childhood longitudinal study 2011 kindergarten cohort (ECLS-K 2011). Evaluation Review, 40(4), 279–313. doi:10.1177/0193841X16655657
- Hedberg, E. C., & Hedges, L. V. (2014). Reference values of within-district intraclass correlations of academic achievement by district characteristics. Results from a meta-analysis of district-specific values. Evaluation Review, 38(6), 546–582. doi:10.1177/0193841X14554212
- Hedges, L. V., & Hedberg, E. C. (2007). Intraclass correlation values for planning group-randomized trials in education. Educational Evaluation and Policy Analysis, 29(1), 60–87. doi:10.3102/0162373707299706
- Hedges, L. V., & Hedberg, E. C. (2013). Intraclass correlations and covariate outcome correlations for planning two- and three-level cluster-randomized experiments in education. Evaluation Review, 37(6), 445–489. doi:10.1177/0193841X14529126
- Hemphill, J. F. (2003). Interpreting the magnitudes of correlation coefficients. American Psychologist, 58(1), 78–80.
- Hill, C. J., Bloom, H. S., Black, A. R., & Lipsey, M. W. (2008). Empirical benchmarks for interpreting effect sizes in research. Child Development Perspectives, 2(3), 172–177.
- Institute of Education Sciences, & National Science Foundation. (2013). Common guidelines for education research and development. Retrieved from http://ies.ed.gov/pdf/CommonGuidelines.pdf
- Ivers, N. M., Taljaard, M., Dixon, S., Bennett, C., McRae, A., Taleban, J., … Donner, A. (2011). Impact of CONSORT extension for cluster randomised trials on quality of reporting and study methodology: Review of random sample of 300 trials, 2000–8. BMJ, 343, d5886. doi:10.1136/bmj.d5886
- Jacob, R. T., Zhu, P., & Bloom, H. S. (2010). New empirical evidence for the design of group randomized trials in education. Journal of Research on Educational Effectiveness, 3(2), 157–198. doi:10.1080/19345741003592428
- Kelcey, B., Shen, Z., & Spybrook, J. (2016). Intraclass correlation coefficients for designing cluster-randomized trials in sub-Saharan Africa education. Evaluation Review, 40(6), 500–525. doi:10.1177/0193841X16660246
- Klieme, E. (2016). TIMSS 2015 and PISA 2015. How are they related on the country level? Retrieved from http://www.dipf.de/de/publikationen/pdf-publikationen/Klieme_TIMSS2015andPISA2015.pdf
- Konstantopoulos, S. (2008). The power of the test for treatment effects in three-level cluster randomized designs. Journal of Research on Educational Effectiveness, 1(1), 66–88. doi:10.1080/19345740701692522
- Lazowski, R. A., & Hulleman, C. S. (2016). Motivation interventions in education: A meta-analytic review. Review of Educational Research, 86(2), 602–640. doi:10.3102/0034654315617832
- LeBreton, J. M., & Senter, J. L. (2008). Answers to 20 questions about interrater reliability and interrater agreement. Organizational Research Methods, 11(4), 815–852. doi:10.1177/1094428106296642
- Lipsey, M. W., & Cordray, D. S. (2000). Evaluation methods for social intervention. Annual Review of Psychology, 51, 345–375.
- Lipsey, M. W., Puzio, K., Yun, C., Hebert, M. A., Steinka-Fry, K., Cole, M. W., … Busick, M. D. (2012). Translating the statistical representation of the effects of education interventions into more readily interpretable forms. National Center for Special Education Research. Retrieved from http://eric.ed.gov/?id=ED537446
- Martin, A. J., Bobis, J., Anderson, J., Way, J., & Vellar, R. (2011). Patterns of multilevel variance in psycho-educational phenomena: Comparing motivation, engagement, climate, teaching, and achievement factors. Zeitschrift Für Pädagogische Psychologie, 25(1), 49–61. doi:10.1024/1010-0652/a000029
- Murray, D. M., & Blitstein, J. L. (2003). Methods to reduce the impact of intraclass correlation in group-randomized trials. Evaluation Review, 27(1), 79–103. doi:10.1177/0193841X02239019
- Murray, D. M., Varnell, S. P., & Blitstein, J. L. (2004). Design and analysis of group-randomized trials: A review of recent methodological developments. American Journal of Public Health, 94(3), 423–432. doi:10.2105/AJPH.94.3.423
- Muthén, L. K., & Muthén, B. O. (2017). Mplus user's guide (8th ed.). Los Angeles, CA: Muthén & Muthén.
- Olejnik, S., & Algina, J. (2000). Measures of effect size for comparative studies: Applications, interpretations, and limitations. Contemporary Educational Psychology, 25(3), 241–286. doi:10.1006/ceps.2000.1040
- Organisation for Economic Co-operation and Development [OECD]. (2004). Learning for tomorrow's world: First results from PISA 2003. Paris, France: Author.
- Organisation for Economic Co-operation and Development (OECD). (2007). Evidence in education. Linking research and policy (2nd ed.). Paris, France: Author.
- Organisation for Economic Co-operation and Development (OECD). (2009). PISA 2006. Technical report. Paris, France: Author.
- Organisation for Economic Co-operation and Development (OECD). (2013). PISA 2012 results: What makes schools successful? Resources, policies and practices (Vol. IV). Paris, France: Author.
- Organisation for Economic Co-operation and Development (OECD). (2014a). PISA 2012 results. Ready to learn. Students' engagement, drive, and self-beliefs (Vol. III). Paris, France: Author.
- Organisation for Economic Co-operation and Development (OECD). (2014b). PISA 2012 results. What students know and can do. Student performance in mathematics, reading, and science (Vol. I). Paris, France: Author.
- Organisation for Economic Co-operation and Development (OECD). (2014c). PISA 2012. Technical report. Paris, France: Author.
- Raudenbush, S. W., Martinez, A., & Spybrook, J. (2007). Strategies for improving precision in group-randomized experiments. Educational Evaluation and Policy Analysis, 29(1), 5–29. doi:10.3102/0162373707299460
- R Core Team. (2017). R: A language and environment for statistical computing. Vienna, Austria: R Foundation for Statistical Computing. Retrieved from https://www.R-project.org/
- Schochet, P. Z. (2008). Statistical power for random assignment evaluations of education programs. Journal of Educational and Behavioral Statistics, 33(1), 62–87. doi:10.3102/1076998607302714
- Slavin, R. E. (2002). Evidence-based education policies: Transforming educational practice and research. Educational Researcher, 31(7), 15–21. doi:10.3102/0013189X031007015
- Spybrook, J., Bloom, H. S., Congdon, R., Hill, C., Liu, X., Martinez, A., & Raudenbush, S. W. (2013). Optimal Design Plus Version 3.0 [Computer Software]. Retrieved from http://hlmsoft.net/od/
- Spybrook, J., & Raudenbush, S. W. (2009). An examination of the precision and technical accuracy of the first wave of group-randomized trials funded by the Institute of Education Sciences. Educational Evaluation and Policy Analysis, 31(3), 298–318. doi:10.3102/0162373709339524
- Spybrook, J., Shi, R., & Kelcey, B. (2016). Progress in the past decade: An examination of the precision of cluster randomized trials funded by the U.S. Institute of Education Sciences. International Journal of Research & Method in Education, 39(3), 255–267. doi:10.1080/1743727X.2016.1150454
- Spybrook, J., Westine, C. D., & Taylor, J. A. (2016). Design parameters for impact research in science education. AERA Open, 2(1), 1–15. doi:10.1177/2332858415625975
- Wang, M. C., Haertel, G. D., & Walberg, H. J. (1993). Toward a knowledge base for school learning. Review of Educational Research, 63(3), 249–294.
- West, M. R., Kraft, M. A., Finn, A. S., Martin, R. E., Duckworth, A. L., Gabrieli, C. F. O., & Gabrieli, J. D. E. (2015). Promise and paradox measuring students' non-cognitive skills and the impact of schooling. Educational Evaluation and Policy Analysis, 38(1), 148–170. doi:10.3102/0162373715597298
- Westine, C. D., Spybrook, J., & Taylor, J. A. (2013). An empirical investigation of variance design parameters for planning cluster-randomized trials of science achievement. Evaluation Review, 37(6), 490–519. doi:10.1177/0193841X14531584
- Wickham, H. (2009). ggplot2: Elegant graphics for data analysis. New York, NY: Springer.
- Wu, M. (2010). Comparing the similarities and differences of PISA 2003 and TIMSS ( OECD Education Working Papers No. 32). Paris, France: OECD Publishing.
- Zhu, P., Jacob, R., Bloom, H., & Xu, Z. (2012). Designing and analyzing studies that randomize schools to estimate intervention effects on student academic outcomes without classroom-level information. Educational Evaluation and Policy Analysis, 34(1), 45–68. doi:10.3102/0162373711423786
- Zopluoglu, C. (2012). Across-national comparison of intra-class correlation coefficient in educational achievement outcomes. Journal of Measurement and Evaluation in Education and Psychology, 3(1), 242–278.