ABSTRACT
Enrichment programs provide learning opportunities for a broader or deeper examination of curricular or extracurricular topics and are popular in gifted education. Herein, we investigated the effectiveness of a statewide extracurricular enrichment program for gifted elementary school children in Germany. The program implemented a ”grass roots“ strategy by which local units developed and offered the enrichment courses, which spanned a broad array of topics. The courses targeted different outcomes, including students' cognitive abilities, school achievement, interests, creativity, self-control, self-concept, and social competencies. We compared third-grade students attending the enrichment program (N =423) with nonattending third-grade students (N = 2,328) by means of a propensity score analysis. Specifically, we controlled for potential selection effects and estimated the average causal effect of the enrichment program for children attending the program. The findings revealed positive program effects on academic achievement but not on the other targeted outcomes.
Curricular and extracurricular activities targeting the needs of gifted children are commonly believed to be a viable means to support gifted children in their academic and socioemotional development (e.g., Plucker & Callahan, Citation2014; Subotnik, Olszewski-Kubilius, & Worrell, Citation2011, Citation2012). Around the world, however, several associations such as the Asian-Pacific Federation on Giftedness (APFG), the European Council for High Ability (ECHA), and the National Association for Gifted Children (NAGC) have argued that gifted children's specific needs are often neglected, a practice that subsequently leads to the shriveling of their abilities and potential. Correspondingly, these associations and others have repeatedly called for the implementation of programs that are aimed at appropriately fostering gifted children (promotion programs; e.g., via acceleration, grouping, or enrichment).
However, the implementation of promotion programs in and of itself does not guarantee positive effects on the development of gifted children. Rather, the effectiveness of such opportunities needs to be investigated. Most likely due to some methodological challenges that tend to occur in giftedness research (Subotnik et al., Citation2011, Citation2012; Thompson & Subotnik, Citation2010), the majority of promotion programs for gifted children have not been systematically evaluated. Consequently, researchers have called for more systematic efforts in investigating the effectiveness of such programs (e.g., Plucker & Callahan, Citation2014).
Herein, we investigated the effectiveness of a statewide extracurricular enrichment program for gifted elementary school children in Germany. The program, called the Hector Children's Academy Program (HCAP), represents the first German statewide enrichment program that was developed to specifically meet the needs of gifted children in elementary schools. The purpose of the HCAP is to foster gifted students on a broad level, comprising abilities, interests, creativity, and a number of other psychological variables. The HCAP is different from many other enrichment programs in its implementation of a “grass roots” strategy for course development. Specifically, local units—namely, academies participating in the HCAP—develop and offer the enrichment courses, with no or only very limited guidelines from the HCAP steering group that coordinates and organizes the entire HCAP (e.g., budgeting, networking, press work, selection of local units). Accordingly, the HCAP courses cover a wide range of topics and contents, although some emphasis is placed on STEM (science, technology, engineering, and mathematics)-related contents, and any evaluation of the program needs to take the breadth of the program into account.
In the current study, we used propensity score matching and subsequent regression analyses to estimate the effect of the HCAP on participating children. Thus, gifted children who were attending the HCAP during one specific term were compared with a control group of children with similar characteristics who did not attend the HCAP. The effects of the HCAP were estimated with respect to eight domains that the principals of the Hector Children's Academies had identified as potential outcome variables that were targeted by the HCAP courses, namely, students' general cognitive abilities, academic achievement, investigative vocational interest, epistemic curiosity, creativity, self-control, self-concept, and social competencies.
Conceptualizing Giftedness
In recent decades, researchers have developed an increasing interest in describing, identifying, and fostering gifted children. There are various definitions of giftedness, and depending on a society's particular values, different aspects are important for considering somebody to be gifted (see Subotnik et al., Citation2011). The traditional single-factor approach equates giftedness with very high levels of general cognitive abilities (Terman, Citation1925; for a current use of this approach, see Wirthwein, Becker, Loehr, & Rost, Citation2011). In this framework, a cutoff value determines whether a person is considered gifted or not. Other conceptions agree with the idea that high cognitive abilities are important for identifying gifted students but have not regarded them as sufficient for defining giftedness by themselves. Rather, giftedness is described as a multidimensional construct that includes several characteristics of a person such as high general cognitive abilities, academic achievement, creativity, or motivation (e.g., Feldman, Citation1986; Gagné, Citation2005; Piirto, Citation1994; Renzulli, Citation1978; Stanley, Citation1976; Sternberg, Citation2003; Tannenbaum, Citation1983; for an overview, see Sternberg & Davidson, Citation2005). For instance, in Renzulli's (Citation1978) three-ring conception, giftedness is related to high levels of general ability, task commitment, and creativity. Besides multidimensionality, many conceptualizations suppose that domain-specific outstanding potential or skills may develop over time and can result in high performance and productivity. The development of potential is assumed to be influenced by internal as well as external or environmental factors such as peers, family, and school (Gagné, Citation2005; Heller, Citation2005; Heller, Perleth, & Keng Lim, Citation2005; Piirto, Citation1994; Stanley, Citation1976; Subotnik et al., Citation2011, Citation2012; Tannenbaum, Citation1983; Ziegler & Phillipson, Citation2012; for an overview, see Sternberg & Davidson, Citation2005; Subotnik et al., Citation2011).
Several approaches that are believed to be able to provide adequate support for gifted students' development have been proposed. Among the most prominent approaches are enrichment programs (i.e., learning opportunities that go beyond the school curriculum). Enrichment programs can encompass two strategies for providing learning opportunities: vertical and horizontal enrichment (Newland, Citation1976). Vertical enrichment indicates a broader or deeper examination of topics that are already included in the regular curriculum such as extra math classes over the weekend. Horizontal enrichment refers to learning about topics outside of the regular curriculum such as classes for learning a language that is not part of the curriculum.
Effectiveness of Enrichment Programs for Gifted Students
Although there are many different enrichment programs for gifted children around the world, knowledge about the effectiveness of enrichment programs is still scarce. Indeed, there have been calls for more thorough investigations in giftedness research in general and on the effectiveness of enrichment programs in particular. On the basis of reviews by Dai, Swanson, and Cheng (Citation2011) and VanTassel-Baska (Citation2006), Plucker and Callahan (Citation2014) summarized that research in gifted education is “descriptive and correlational” (p. 393). Small sample sizes, no control groups, no randomization, and unclear definitions of giftedness are methodological problems that affect giftedness research in general and pose an obstacle for estimating the effectiveness of enrichment programs for gifted children in particular (see, e.g., Plucker & Callahan, Citation2014; Subotnik et al., Citation2011, Citation2012; Thompson & Subotnik, Citation2010).
Moreover, empirical studies on enrichment activities have often focused on specific, narrowly focused learning opportunities (e.g., an enrichment opportunity concerning mathematics) and hardly ever on enrichment opportunities that cover a broad array of topics, although many enrichment programs exist that aim at fostering students on a broad set of characteristics. For instance, weekend or summer enrichment programs at the Center for Talent Development at Northwestern University focus on fostering curiosity and passion on a specific topic and the courses cover a wide array of themes (e.g., mathematics and social science; for more information see: http://www.ctd.northwestern.edu/program/saturday-sunday-enrichment-programs). Further examples are the Stanford Education program for Gifted Youth (https://epgy.stanford.edu) or the Saturday Enrichment Program of University of Virginia (http://curry.virginia.edu/community-programs/student-enrichment/sep/saturday). Many similar programs exist. Recent work put forward the accessibility for disadvantaged students, such as poor or minority pupils, in such programs (Olszewski-Kubilius & Thomson, Citation2010; Siegle et al., Citation2016) and specific programs meeting the needs of these groups were developed (Kaul, Johnsen, Saxon, & Witte, Citation2016; Olszewski-Kubilius & Thomson, Citation2010). However, the overall effects of such programs are hardly evaluated. Indeed, to the best of our knowledge, studies have yet to be conducted on the effectiveness of a statewide extracurricular enrichment program that is aimed at fostering different gifted elementary school students' needs by offering a broad spectrum of diverse courses. The most challenging aspect is the definition of outcomes that are used for the effectiveness evaluation.
Previous meta-analyses and review articles on the effects of enrichment programs on gifted children's development have indicated positive effects on school achievement (Kim, Citation2016; Kulik & Kulik, Citation1992; Rogers, Citation1991; Vaughn, Feldhusen, & Asher, Citation1991), whereas the effects on social-emotional variables, attitudes, and self-concept are less clear (Byers, Citation1961; Ekstrom, Citation1961; Feldhusen & Moon, Citation1992; Kim, Citation2006; Neihart, Citation2007; Rogers, Citation2007; Vaughn et al., Citation1991). The first meta-analysis that, among other things, considered “enrichment programs” was conducted by Vaughn et al. in 1991 to investigate the effectiveness of pull-out programs. It included nine research studies published between 1959 and 1989. Inclusion criteria for the studies were: true or quasi-experimental design and a control group of gifted children. Vaughn et al. (Citation1991) reported that pull-out programs had positive effects on achievement, critical thinking, and creativity. No effects were found for self-concepts.
The most recent meta-analysis on the effectiveness of enrichment programs included 26 empirical studies that have been published since 1985 (Kim, Citation2016). There were several inclusion criteria, and the most important ones were: the practice of the enrichment programs had to be indicated, sufficient quantitative information for calculating effect sizes had to be provided, and the study had to use control and treatment groups or repeated-measures designs. Thirteen studies investigated the effects of an enrichment program on academic achievement (e.g., reading comprehension, analytic skills, math achievement), and 16 studies looked at effects on socioemotional outcomes (e.g., intrinsic value, social skills, self-concept, attitude toward learning). Three studies included both types of outcome measures. It is interesting that all studies reported either a quasi-experimental or a pre–post design without a control group. Findings revealed a positive effect of enrichment programs on academic achievement, average effect size = 0.96 [0.64 to 1.30], and socioemotional outcomes, average effect size = 0.55 [0.32 to 0.79].
To obtain greater insight into recently published studies, we systematically reviewed empirical studies on the effectiveness of enrichment programs for gifted students that were published between 2010 and 2015 in six journals devoted to giftedness research (Gifted Child Quarterly, High Ability Studies, Journal for the Education of the Gifted, Journal of Advanced Academics, Roeper Review) and in five more general educational journals (American Educational Research Journal, Educational Evaluation and Policy Analysis, Journal of Educational Psychology, Journal of Educational Research, Journal of Research on Educational Effectiveness). Table S1 in the supplemental online material (SOM) provides an overview.
We found a total of 19 research articles from this time period (seven studies overlapped with the meta-analysis reported by Kim, Citation2016). Thirteen of the 19 studies used quantitative methods. Nine of these 13 articles described either experimental (five) or quasi-experimental (four) designs, including a control group, and eight of these studies used pretest and posttest measurements. The remaining four of these thirteen quantitative studies used repeated measurements in one group. The majority of the studies investigated the effectiveness of specific enrichment trainings aimed at fostering cognitive, mathematical, spatial, verbal, or socioemotional abilities. In general, the findings revealed positive achievement effects of moderate sizes for cognitive (Gubbels, Segers, & Verhoeven, Citation2014), mathematical (McCoach, Gubbins, Foreman, Rubenstein, & Rambo-Hernandez, Citation2014), and verbal domains (Lee, Olszewski-Kubilius, & Peternel, Citation2010). For one intervention, large effects were reported for spatial ability (Coxon, Citation2012). Besides the programs' efficacy on achievement scores, positive effects were also found on attitudes and perceptions related to group experiences (Peterson & Lorimer, Citation2011), an enhancement of self-reported enjoyment of science (Gubbels et al., Citation2014), and an increase in planned careers in science (Fraleigh-Lohrfink, Schneider, Whittington, & Feinberg, Citation2013), as well as a reduction in self-critical evaluative tendencies (Mofield & Chakraborti-Ghosh, Citation2010). Overall, previous studies suggest positive effects on academic achievement and socioemotional variables. However, none of these studies investigated the effectiveness—with a large sample and a quasi-experimental pretest–posttest design—of a statewide extracurricular enrichment program for gifted elementary school children that offers a wide array of course topics.
The Hector Children's Academy Program
In 2010, the extracurricular enrichment program for gifted elementary school children called the Hector Children's Academy Program was established in the German state of Baden-Württemberg. Funded by the Hector Foundation II, the HCAP was introduced to meet the needs of gifted elementary school children. For easy accessibility, the program is implemented at several local sites, so-called Hector Children's Academies, which are typically located at regular elementary schools across Baden-Württemberg (as of the beginning of 2016, a total of 61 Hector Children's Academies had been established). An agreement between the state of Baden-Württemberg and the Hector Foundation II has provided general guidelines for establishing a Hector children's academy (Citation2010). The guidelines involve topics such as the selection of students for the program, the focus and aim of the program, clarification of responsibilities, and financial support.
Students can participate in the HCAP after being nominated as gifted by their teachers from their regular school. More precisely, teachers from any elementary school in Baden-Württemberg can suggest students for participation by enrolling them in one academy (Rothenbusch, Zettler, Voss, Lösch, & Trautwein, Citation2016). In line with more comprehensive conceptualizations of giftedness and to avoid nominations that are based on school achievement or intelligence only, teachers are instructed to nominate children for the whole program (and not a specific course) by considering a broad range of characteristics involving high (cognitive) abilities, creativity, interests, and motivation (Agreement, Citation2010). The program does not prescribe a specific description of giftedness (e.g., “the 2%–3% most intelligent students” (for similiar approaches see, e.g., Gear, Citation1978; Neber, Citation2004; Schulthess-Singeisen, Neuenschwander, & Herzog, Citation2008). The Hector Foundation II is aimed at providing access to the program for approximately 10% of all students from Baden-Württemberg in each grade (see Agreement, Citation2010). To allow access to the program that is independent of the gifted children's social background, participation in the HCAP program is free of charge. Although the local academies make the final decision about acceptance, in the past, almost all nominated students were accepted in the end if space permitted it (see Rothenbusch et al., Citation2016).
The purpose of the HCAP as defined by educators and administrators is to foster students' development in a broad sense, comprising general cognitive abilities, domain-specific abilities, domain-specific interests, self-concept/performance motivation, self-regulation/self-control, and social competencies. To enable the broad promotion of gifted students, it is necessary to offer a broad selection of enrichment courses. An important characteristic of the enrichment program is its “grass roots” approach by which courses are developed and offered at a local level, with only a limited number of general guidelines. In contrast to interventions in which one or only a few more narrow courses are similarly administered, the HCAP provides many diverse course topics that address a broad spectrum of gifted elementary school children's interests and needs. The courses offer additional learning opportunities that go beyond the regular curriculum and include vertical and horizontal enrichment. The course topics range from curriculum-related subjects such as arts, languages (i.e., English, French), mathematics, or sports to topics that are completely new to the students such as astronomy, chess, or computer science. According to the general guidelines of the HCAP, at least 60% of the courses that are offered by local academies should focus on STEM-related contents (Agreement, Citation2010). Gifted children are brought together at scheduled intervals (typically 2 hours a week) during one school term. Courses commonly last one term and are provided for first- to fourth-grade elementary school children. Each academy runs its own program (e.g., selects teachers by itself, is responsible for the courses that are offered). Although many programs for gifted students around the world seem to implement such an approach (e.g., summer and Saturday programs), research on such programs is sparse.
The Present Investigation
In this study, we examined the effectiveness of the HCAP program. We used a quasi-experimental research design (control and enrichment group, no randomization) to investigate the effects of the HCAP in a large sample of elementary school children (N = 2,751). Given its size (statewide implementation) and the variety of courses (e.g., chess, mathematics, science), the HCAP provides a unique opportunity for evaluating the effectiveness of a large enrichment program in a natural setting.
Because we could not randomly assign students to groups, statistical methods that allow for causal inferences even in the absence of a randomized controlled trial (Plucker & Callahan, Citation2014) were required to test the program's effectiveness. One approach that is designed for this purpose is propensity score adjustment (Rosenbaum & Rubin, Citation1983). Propensity score adjustment attempts to balance systematic and potentially confounding group differences between the treatment (in our case, enrichment program participants) and control groups before the treatment begins.
Due to the grass roots character of the course offerings and the multiple outcomes, we broadly investigated whether the program affected students' general cognitive abilities, academic achievement, investigative vocational interest, epistemic curiosity, creativity, self-control, self-concept, and social competencies because the program was developed to foster students broadly.
Method
Procedure and Participants
Data for the current investigation were collected in the 2012–2013 school year. At that time, all of the 48 established Hector Children's Academies were asked for their participation, and finally, 45 agreed. They were spread across Baden-Württemberg (http://www.hector-kinderakademie.de/,Lde/Startseite/Kinderakademien), a state with an area of 13,804 sq mi and approximately 2,300 elementary schools. Children from the (approximately) 2,300 schools could attend the HCAP at one of the 48 academies (i.e., at one of the 48 elementary schools that served as “hosts” for a Hector children's academy). The catchment area of each academy varied to a small degree, but there is no detailed information available on this variation.
For each of these academies, the schools that were chosen to participate typically consisted of the elementary school in which the academy was located as well as up to four elementary schools from the catchment area—randomly selected out of a pool of schools that had recommended students for the academy in a previous school year. By applying this strategy, we aimed to avoid sampling a large percentage of schools that had not nominated any children for the HCAP at all. Half of the schools agreed to participate in the study. Instead of 225 schools, 111 schools finally participated. From each school, we included two classrooms of third-grade students in the sample. When a school had more than two classrooms of third graders, the two were chosen randomly. However, some (35) schools had only one classroom of third graders, and for practical reasons, two schools contributed a total of three classrooms. The sample consisted of 2,883 students from 189 different classes at 111 schools. For our analyses, we excluded cases for which we had no information about their treatment assignment. Thus, our final sample included 2,751 third graders enrolled in 181 classes at 109 schools. From these students, 423 (15%) attended at least one course offered by the HCAP (treatment group). The remaining 2,328 (85%) children did not attend the enrichment program (control group). The demographic characteristics of the sample are displayed in .
Table 1. Descriptive statistics (pretest measurement).
The enrichment opportunities at the academies began approximately four weeks after the beginning of the school year and were held during its first term (i.e., they lasted approximately four months). Teachers nominated students prior to the beginning of the enrichment activities (for further information about the nomination procedure, please see Rothenbusch et al., Citation2016). Students were asked to complete standardized tests and to fill out several questionnaires at the beginning of the school year (i.e., before the courses began) and after the first term (i.e., after the courses ended) in classroom assessments.
Measures
Outcome Variables. Educators and administrators of the HCAP determined the outcome measures on a conceptual level by defining the purposes of the HCAP. Specifically, at the beginning of the HCAP, they stated that the program should foster students' development in a broad sense, comprising general cognitive abilities, domain-specific abilities, domain-specific interests, self-concept/performance motivation, self-regulation/self-control, and social competencies. We consequently opted to assess constructs in these seven domains, using measures of intelligence (to assess general cognitive abilities), school grades in mathematics and German (to assess academic achievement as a domain-specific ability), investigative vocational interest and epistemic curiosity (to assess domain-specific interests), self-concept (to assess self-concept/performance motivation), self-control (to assess self-regulation/self-control), and social competencies (to assess social competencies). Further, we assessed creativity because this is a construct that is often linked to giftedness (e.g., Renzulli, Citation1978), and it is relevant for recommending students for the HCAP (Agreement, Citation2010).
Thus, the student characteristics that we assessed were chosen because they reflect constructs that had been identified as relevant outcomes of the HCAP when the HCAP was initiated and, in turn, might be positively influenced by attending the HCAP in general without considering the specifics of an HCAP course. For all measures (except intelligence, creativity, and school grades), we used mean scores across single items to compute the scales (i.e., variables). If there were missing values on 50% or more items, the scale was considered missing. Overall, the number of missing values per item did not differ from the number of missing values per scale (for more details, see Table S2 in the SOM). Means, standard deviations, rates of missing values, and internal consistency estimates for all variables are listed in and . Correlations between all outcome variables at the first and second measurement occasions as well as the differences in correlations are presented in Tables S3 to S5 in the SOM. Unless stated otherwise, the tests and scales were administered to the students at both measurement points. More details about the outcome measures are provided in the SOM.
Table 2. Descriptive statistics (posttest measurement).
General Cognitive Abilities. We measured students' fluid and crystallized intelligence via an adaptation of the Berlin Test of Fluid and Crystallized Intelligence for Grades 8–10 (Wilhelm, Schroeders, & Schipolowski, Citation2014) so that the measure was appropriate for elementary school children in Grades 3 and 4 (Schröders, Schipolowski, Zettler, Golle, & Wilhelm, Citation2016). We used two parallel test versions. Each version consisted of three subtests (34 items in total) measuring the verbal, numeric, and figural parts of fluid intelligence (Version A pretest: M = 15.89, SD = 5.47, α = .79; Version B pretest: M = 15.56, SD = 5.19, α = .77) plus a subtest for crystallized intelligence (42 items; Version A pretest: M = 19.12, SD = 5.86, α = .75; Version B pretest: M = 18.56, SD = 5.72, α = .74). Each child completed one version, and the versions were randomized across classes. The fluid and crystallized intelligence scores for each version were then standardized (M = 100 and SD = 10) and combined into one fluid intelligence score and one crystallized intelligence score. At the second measurement point, half of the classes were given the same version, whereas the other half of the classes were given the parallel version (fluid: Version A posttest: M = 19.32, SD = 5.53; Version B posttest: M = 18.55, SD = 5.38, α = .79; crystallized: Version A posttest: M = 22.42, SD = 5.73, α = .75; Version B posttest: M = 21.64, SD = 5.75, α = .74). Pilot versions of our adaptation were pretested in several samples that totaled to more than 3,000 students.
Academic Achievement. Schools provided students' school grades in German and mathematics at the end of Grade 2 (pretest) and when midterm grades were given in Grade 3 (posttest). In German elementary schools, a 6-point grading scale is used to assess students' performance. School grades range from 1 = very good to 6 = insufficient (1 = very good, 2 = good, 3 = satisfactory, 4 = sufficient, 5 = deficient, 6 = insufficient). There is no statewide standardized testing at elementary schools in Baden-Württemberg, making these school grades the most meaningful achievement indicators in these schools. These school grades are also used to make decisions about grade retention and placing students in different tracks at the end of elementary school.
Investigative Vocational Interest. On the basis of Holland's (Citation1997) work on vocational interests and on corresponding measures for adults and teenagers (e.g., Bergmann & Eder, Citation1992; Tracey & Ward, Citation1998), we developed an inventory to assess students' realistic, investigative, artistic, social, enterprising, and conventional interests via self-reports. Therein, each interest domain was assessed via seven items, presented with a 5-point Likert scale ranging from 1 (not at all) to 5 (very much). We estimated the treatment effect for investigative interest. We decided to use this scale only because the other five domains were not targeted by a majority of courses (e.g., in many courses, conventional interests are not addressed at all).
Epistemic Curiosity. On the basis of corresponding scales for adults (e.g., Litman & Spielberg, Citation2003) and older children (e.g., Piotrowski, Litman, & Valkenburg, Citation2014), we developed a 10-item scale for assessing elementary school children's epistemic curiosity. A sample item is “It is fun to learn something about a new topic.” Again, we used a Likert scale response format ranging from 1 (never) to 4 (always). We used a composite score for epistemic curiosity.
Creativity. In line with Guilford's Alternative Uses Task (Guilford, Citation1967), students were given 2 min to come up with ideas for what one could do with a wooden board. Answers were checked for meaningfulness and duplicates before calculating the sum of all answers (representing “fluency,” see Kim, Citation2006). Higher scores indicate higher creativity.
Self-Control. On the basis of the self-control scale by Tangney, Baumeister, and Boone (Citation2004) and its German adaptation (as a short version) by Bertrams and Dickhäuser (Citation2009) as well as the self-control scale (including items devoted to childhood) by Marcus (Citation2003), we developed a self-control scale consisting of 26 items with a 5-point Likert scale ranging from 1 (wrong) to 5 (true). Sample items are “If I do not want to do my homework, I will play,” or “If I see candies, I will eat them.”
Self-Concept. Students' self-concept was measured via six facets from the German version (Arens, Trautwein, & Hasselhorn, Citation2011; Arens, Yeung, Craven, & Hasselhorn, Citation2011) of the Self-Description Questionnaire I (SDQ I; Marsh, Citation1990), namely, concerning (a) physical appearance, (b) physical ability, (c) peer relationships, (d) parent relationships, (e) school, and (f) self-esteem. All scales consisted of three items with a response scale ranging from 1 (wrong) to 5 (true). As the dependent variable at the second measurement point, we used school self-concept because many courses did not address the other domains at all.
Social Competence. We translated the 12-item Social Competence Scale in Preschool-Age Children (Gouley, Brotman, Huang, & Shrout, Citation2008), which asks parents to assess their child's social competence. The response scale ranged from 1 (disagree) to 5 (agree). An example item is “My child autonomously solves problems with friends or siblings.”
Covariates. All variables that were potentially important for the treatment assignment and all pretest measures of the dependent variables were used as covariates (cf. Schafer & Kang, Citation2008; Steiner, Cook, Shadish, & Clark, Citation2010). That is, in addition to the pretest measures of all dependent variables (academic achievement, interests, creativity, self-control, self-concept, social competence), we considered demographic variables, academic boredom, academic interests, intrinsic motivation, personality traits (assessed via parent ratings), school engagement, stressors, and mean class intelligence as covariates that were potentially important for the allocation procedure. We chose these variables because teachers were instructed to nominate children by considering a broad range of characteristics such as high (cognitive) abilities, creativity, interests, and motivation; we also used contextual factors such as mean class abilities (see Rothenbusch et al., Citation2016). It was important to include an extensive set of covariates to reduce bias in the treatment effect (see Thoemmes & Kim, Citation2011). Due to the fact that we did not know which variables the teachers used to nominate children, we included variables that have previously been associated with teacher nominations and teacher beliefs about giftedness (e.g., Endepohls-Ulpe & Ruf, Citation2005; Hany, Citation1997; Harradine, Coleman, & Winn, Citation2014; Rothenbusch et al., Citation2016). For a complete overview of these covariates, see Table A1 in the appendix (for descriptive statistics, see Table S6 in the SOM). Again, note that neither a specific definition nor standardized test criteria were used to nominate children for the HCAP.
Booklet Design
As we intended to collect a large set of background variables from all students and simultaneously reduce students' burden and avoid fatigue effects, we used a booklet design with planned missing data (Graham, Taylor, Olchowski, & Cumsille, Citation2006; Little & Rhemtulla, Citation2013). The booklets consisted of different tests and questionnaires and were randomly administered across classes (for the pretest: eight booklets; for the posttest: 17 booklets). Each class was tested on two days for both the pretest and posttest measures.
Students' parents were also asked to fill out several questionnaires at both measurement points (these questionnaires were administered via the participating schools). A professional contractor, independent of the research team, was responsible for collecting the data.
Analysis
Although propensity score adjustment methods were introduced about 30 years ago (Rosenbaum & Rubin, Citation1983), they have only recently become tremendously popular in psychology and other social sciences (Adelson, Citation2013; Thoemmes & Kim, Citation2011). They have been suggested to be useful for quasi-experimental designs in giftedness research in particular (Fan & Nowell, Citation2011; Thompson & Subotnik, Citation2010). Adelson, McCoach, and Gavin (Citation2012), for instance, used stratification based on propensity score estimation to investigate the effects of a gifted programming policy in mathematics and reading for third to fifth graders. Also, propensity score matching was very recently applied to investigate the effects of acceleration for gifted students (Kretschmann, Vock, & Lüdtke, Citation2014; Park, Lubinski, & Benbow, Citation2013).
Due to the nonrandomized allocation of participants to the treatment (HCAP participants) and control groups (no HCAP participants), propensity score matching was used (Rosenbaum & Rubin, Citation1983; Stuart, Citation2010) because this practice allowed us to estimate the average treatment effect on the treated (ATT) and, thus, the average effect of the HCAP for children attending the program in the matched data. Propensity score matching was aimed at identifying a well-matched control group for the existing treatment group regarding potential confounding variables measured prior to the intervention (i.e., at the beginning of the school term). The advantage of applying propensity score matching to this study design is that we were able to compare children who participated in this program with control group children who showed almost identical characteristics. For the analysis, we followed the recommendations by Thoemmes and Kim (Citation2011). A summary of these recommendations and our procedure is reported in .
Table 3. Details about the propensity score analysis according to Thoemmes and Kim (Citation2011).
In order to apply propensity score matching, it is necessary to have complete data sets. In our sample, missing data occurred for different reasons but mostly because the test design was a planned missing data design (see Graham et al., Citation2006). Given that the booklets were randomly assigned to students, the resulting missing data could be assumed to be missing completely at random (MCAR). However, there were additional item nonresponses that resulted from items that were omitted or that were not reached. For the student questionnaires, the data that were missing by design ranged from 5% to 56%, and nonresponses from omitted and not-reached items ranged from 2% to 24%. For the parent questionnaires, missing data ranged from 28% to 32%. For more details, see and .
To handle the data that were missing by design as well as the additional nonresponses, we assumed that the data were missing at random (MAR; i.e., missingness depends exclusively on observed variables; Schafer & Graham, Citation2002). Thus, the probability of missing responses was determined by the observable covariates. Given all the observable covariates, the variable Y and the occurrence of item nonresponses in Y (including missing values due to items that were not reached) were conditionally stochastically independent. Multiple imputation using chained equations was used to generate filled-in data sets, which were used for further analyses (Cham & West, Citation2016; Mitra & Reiter, Citation2012; Rubin, Citation1987). Multiple imputation is one of the state-of-the-art methods that can be used to account for item nonresponses if the missing data mechanism is ignorable (Schafer & Graham, Citation2002). We generated 20 imputed data sets with the software IVEware (Raghunathan, Solenberger, & van Hoewyk, Citation2002). In order to stabilize the imputation model, two criteria were specified: (a) a maximum of the 30 most predictive variables was chosen for the imputation model of each variable Xi, and (b) a variable Xj≠i was included as a predictor of Xi only if Xj≠i explained at least 1% of the variance in Xi. In addition, cluster means of all variables were included as potential predictors in the imputation model to account for the possible multilevel structure of the data (see Becker et al., Citation2014).
The next step involved estimating the propensity score (conditional probability for treatment assignment given a vector of covariates; Rosenbaum & Rubin, Citation1983) by computing a logistic multilevel regression in each of the 20 data sets separately (see Thoemmes & West, Citation2011). To this end, the binary treatment variable (assigned vs. not assigned to the enrichment program) was predicted by all variables measured at the beginning of the study, potentially relevant for the assignment and outcome prediction (see Caliendo & Kopeinig, Citation2008; Cook, Steiner, & Pohl, Citation2009; Rosenbaum, Citation1984; Schafer & Kang, Citation2008; Steiner et al., Citation2010). One important aspect about the design of the study is the hierarchical structure of the data: students were nested in classes, schools, and academies. Table A2 in the appendix shows the proportion of variance explained by the class, school, and academy levels for all posttest measures. The SOM (Tables S7 to S9) contains the intraclass correlations for each level and all variables and the proportion of variance explained by the class, school, and academy levels for the nomination variable as well as for all pretest variables. To account for the multilevel structure of the data (Thoemmes & West, Citation2011) and the contextual effects on students' nominations (Rothenbusch et al., Citation2016), we calculated a multilevel logistic regression (random intercept model) and included the class means (cluster means) of fluid intelligence and crystallized intelligence as predictors in this regression analysis. For an overview of all predictor variables, see Table A1 in the appendix. Estimated propensity scores were obtained as predicted values in the multilevel logistic regression.
Propensity score matching was done in R (package: MatchIt; Ho, Imai, King, & Stuart, Citation2013; Ho, Imai, King, & Stuart, Citation2011) separately for each of the 20 imputed data sets (see Kretschmann et al., Citation2014; Nagengast, Marsh, & Hau, Citation2013; Park et al., Citation2013). We compared three matching procedures for their effectiveness in balancing covariate distributions and the propensity scores by following Stuart's (Citation2010) recommendations: nearest neighbor matching, optimal 1:1 matching, and full matching. For all matching procedures, we used a logistic regression model in MatchIt that included all pretest variables that were relevant for the assignment and outcome prediction (see Table A1). The distance measure in all three procedures was the previously calculated propensity score.
For nearest neighbor matching, each treated unit was matched to the control unit (without replacement) with the smallest distance (Ho et al., Citation2011). The order for finding appropriate matching pairs was the default setting: largest to smallest. In contrast to nearest neighbor matching, optimal matching was used to minimize the average absolute distance across all matched pairs (Gu & Rosenbaum, Citation1993; Ho et al., Citation2011). All other properties were the same for optimal and nearest neighbor matching. The third procedure was full matching, a flexible type of subclassification (Hansen, Citation2004; Rosenbaum, Citation2002). One subclass or set of matched units contained one treated unit and at least one control unit or one control unit and at least one treated unit without any replacement. The control units were weighted to minimize the weighted average of the distance measure within each subclass. Control units that were outside the area of common support were discarded (Ho et al., Citation2011). For all three matching procedures, we compared the quality of the matching on the basis of standardized mean differences between the treatment and control groups for all variables that were used to estimate the propensity score (covariates) and the propensity score itself (see Thoemmes & Kim, Citation2011). In line with the What Works Clearinghouse (WWC) guidelines (Citation2014), baseline differences between the two groups were categorized into three levels. According to the guidelines, two groups can be considered equivalent (Level 1) if the absolute value of their standardized mean difference is 0.05 or less. Two groups can be considered equivalent but in need of statistical adjustment (Level 2) if the absolute value is between 0.05 and 0.25 standard deviations. Two groups cannot be considered equivalent at baseline (Level 3) if the absolute value is greater than 0.25. The two groups must be considered equivalent at baseline in order for an unbiased treatment effect to be estimated.
To estimate the ATT on the basis of the matched data, we computed multiple linear regression analyses and corrected the standard errors for the multilevel structure of the data (students nested in classes, schools, and academies). These analyses were also implemented in R (package: survey; Lumley, Citation2016). To account for residual bias, all variables that had been used to estimate the propensity score were included as covariates in the regression model (see Cochran & Rubin, Citation1973; Rubin & Thomas, Citation2000). This approach is called doubly robust (Schafer & Kang, Citation2008). The advantage of this procedure is to estimate the average treatment effect on the treated while simultaneously controlling for any remaining imbalances in the covariate distributions. The final parameter estimates and statistics were obtained by pooling the coefficients and standard errors across the imputed data sets by means of Rubin's rules (Rubin, Citation1987).
Results
Propensity Score Matching
To compare the differences between the treatment and control groups before and after matching, mean differences were standardized with the pooled standard deviation (“average” standard deviation) in the denominator (see WWC guidelines, Citation2014). Averaged standardized mean differences across all imputed data sets are displayed in to describe the differences between the two groups before the program started and to compare the quality of the matching after nearest neighbor, optimal, and full matching (for similiar applications see Kretschmann et al., Citation2014; Park et al., Citation2013). An exhaustive list of all standardized mean differences is presented in Table A1.
Figure 1. Standardized mean differences before matching, after nearest neighbor matching, after optimal matching, and after full matching are presented as average across all imputed data sets.
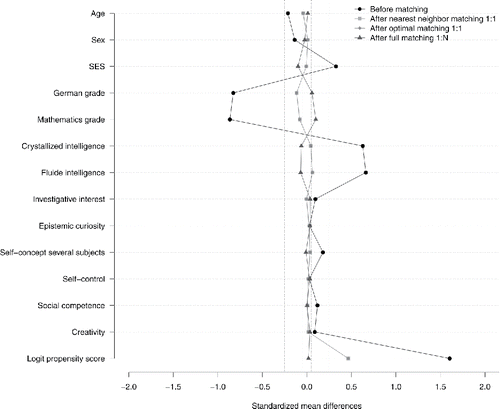
According to the standards described in the WWC guidelines (Citation2014), substantial differences (absolute standardized mean differences > 0.25) between the treatment and control groups before matching were observed for fluid and crystallized intelligence, academic achievement, openness to experience, conscientiousness, and socioeconomic status (SES). For more details, see Table A1 and . In comparison with the control group, the children assigned to the treatment group had higher scores on fluid and crystallized intelligence, had better marks, were more open to new experiences, and were more conscientious. These findings indicate that the process of nominating children to the HCAP was largely valid because the empirical group differences corresponded to the previous nomination instructions for teachers (to consider a broad range of characteristics, i.e., high [cognitive] abilities, creativity, interests, and motivation). Furthermore, the HCAP participants lived in families with a higher SES. As expected, the standardized mean difference for the propensity score was high and positive: Students who participated in the program compared with those who did not had a higher probability of being assigned to the treatment given all the covariates measured at pretest.
After matching, we considered the sample sizes of the treatment and control groups, the weights in each group if they were used, and balancing characteristics. For nearest neighbor and optimal matching, 432 matched pairs were created in each imputed data set, and 1,905 control units were discarded. The number of matched pairs was determined by the number of treated units because each treated unit was matched to a control unit (Ho et al., Citation2011). Full matching allowed more students from the control group to be included in the analysis by applying appropriate weights for the matched cases. When full matching was used, the number of discarded control units ranged from 27 to 312 (M = 125.00) across all imputed data sets. The number of identified subclasses ranged between 262 and 285 (M = 272.95). A subclass represents a matched “pair” formed by two or more observational units from the sample; this pair is then used in future analyses. Each subclass consisted of either one control unit and at least one treated unit or of at least one control unit and one treated unit. Each treated unit had a weight of 1. The control unit weights ranged from 0.02 to 148.29 with a mean value of 1 and standard deviations ranging from 3.67 to 4.75. Thus, some control units received large weights, but the majority of cases had a weight below 20.
To assess the quality of the matching and, thus, to assess the balancing of the covariate distributions, we used standardized mean differences. According to Rubin (Citation2001), standardized mean differences should not exceed 0.25 for an acceptable balance statistic. This criterion is in line with the WWC guidelines (Citation2014) because an absolute value of a standardized mean difference greater than 0.25 indicates a violation of baseline equivalence. The quality of the matching results was acceptable (>−0.25 and <0.25) for all covariates across all matching procedures (, Table A1). However, the balancing of the propensity score was different between nearest neighbor, optimal, and full matching. Only for full matching—the chosen procedure—the criterion of less than 0.25 standard deviations in the propensity score between the treatment and control groups was fulfilled.
After full matching, the absolute standardized mean differences ranged from <0.001 to 0.100 across all covariates and the propensity score. According to the WWC standards (Citation2014), statistical adjustment is necessary if the absolute standardized mean differences fall between 0.05 and 0.25. Thus, the treatment and control groups could be considered equivalent, but statistical adjustment was still necessary. Across schools and classes, some teachers did not nominate children at all, and some teachers nominated several students, explaining the substantial overlap between the two groups. To further ensure the comparability of students in the control and treatment groups, students' affiliations with classes, schools, and academies were itemized (see Table S10 in the SOM). The majority of children in the matched data sets as well as in the original data set came from the same classes, schools, and academies. In the final analyses, we therefore decided to control for the remaining imbalance in variables measured at the first measurement occasion (see the next paragraph for more details). We did not include quadratic or interaction terms in our final propensity score model because there was no improvement in the quality of the matching when such terms were included. Furthermore, differences in the propensity score distributions almost disappeared between the two groups after full matching ( and ). In line with Thoemmes and Kim's (Citation2011) recommendations, the list of the 14 characteristics of a propensity score analysis is presented in .
Figure 2. Propensity score (logit metric) distribution before matching. This figure presents the nonparametrically estimated density of the propensity scores based on all imputed data sets. To obtain a density distribution across all data sets, we combined them.
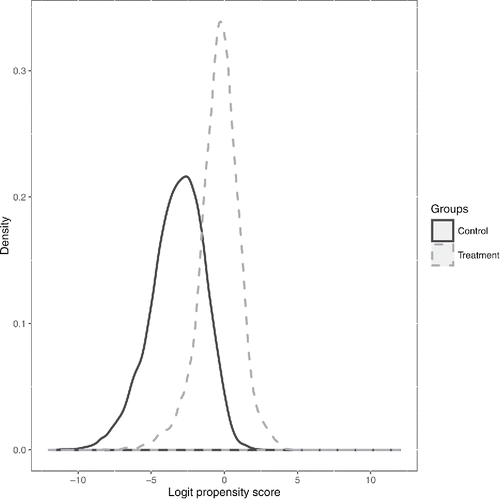
Figure 3. Propensity score (logit metric) distribution after full matching. This figure presents the nonparametrically estimated density of the propensity scores based on all matched data sets. To obtain an average density distribution across all data sets, we combined them and adjusted the weights by the sum of the weights in each group.
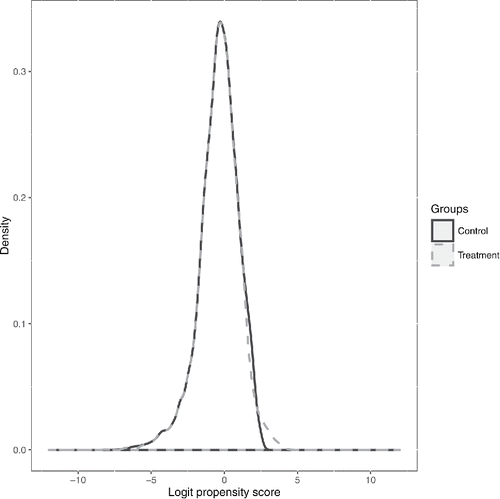
Estimating the HCAP's Effectiveness
Because the aim of the HCAP is to foster students broadly, we investigated the effects of the program on fluid and crystallized intelligence (representing general cognitive abilities), school grades in the main subjects in elementary school (representing academic achievement), interest in investigative vocational activities and epistemic curiosity (representing interests), creativity, self-control, self-concept for several subjects (representing self-concept), and social competencies. The independent variables consisted of the group assignment and all variables that had already been considered for the matching procedure.
The ATT is represented by the multiple regression coefficient of the group variable (0 = did not attend the program, 1 = attended the program). To estimate the size of this effect, we standardized this coefficient by the pooled standard deviation of the dependent variable averaged across the 20 matched data sets. The findings, focused on the treatment effects, are summarized in . For more details about all regression coefficients, see Tables S11 to S20 in the SOM.
Table 4. Average treatment effect on the treated (pooled across 20 imputed data sets)—Standard error correction.
The findings revealed an effect on academic achievement as represented by school grades. Children who participated in the enrichment program received significantly better (lower) German grades, b = −0.12, SE = 0.04, t(286.86) = −2.67, p = .008, ES = −0.20, and mathematics grades, b = −0.12, SE = 0.05, t(101.19) = 2.51, p = .014, ES = −0.21, at the end of the school term than children who did not attend the program. These subjects are the core academic disciplines in elementary school. Although the HCAP is an extracurricular enrichment program, it positively affected one of the key educational outcomes.
With respect to the other outcomes, there was no other statistically significant effect. More specifically, there was no effect of the enrichment program on self-reported school self-concept, b = 0.03, SE = 0.09, t(62.29) = 0.31, p = .761. Students attending the program did not report doing better in all school subjects than peers who did not participate in one of the enrichment courses, although the students in the program got better marks. There was no significant effect of the enrichment program on general cognitive abilities: fluid, b = 1.27, SE = 0.85, t(69.46) = 1.49, p = .142, and crystallized intelligence, b = 0.63, SE = 0.87, t(62.03) = 0.72, p = .472. Children from both groups, although comparable on various characteristics except for their participation in the program for gifted children, did not significantly differ in their group means of fluid and crystallized intelligence scores at the end of the school term. Furthermore, there were no significant effects for self-reported domain-specific interests as represented by interest in investigative activities, b = 0.11, SE = 0.10, t(90.82) = 1.14, p = .256, and epistemic curiosity, b = 0.07, SE = 0.05, t(99.43) = 1.29, p = .201. At the end of the school term, children who participated in the program did not report being more interested in investigative activities or more curious about new topics or challenges compared with the matched group of children who attended only regular school classes without enrichment courses. The children in the program were also not more creative after the school term than the children in the control group, b = 0.11, SE = 0.26, t(69.36) = 0.43, p = .665. The HCAP students were not able to come up with significantly more ideas about what they could do with a wooden board compared with the students in the regular classes. There was also no advantage for students attending the program in self-control, b = 0.01, SE = 0.06, t(52.83) = 0.16, p = .873. At the end of the term, their self-reports indicated neither more nor less self-control with respect to school behaviors such as homework or resisting candies. Parent-reported social competence was also not affected by students' attendance in the enrichment program, b < 0.01, SE = 0.05, t(83.83) = −0.05, p = .961. For instance, parents of HCAP children did not report that their children were able to use better coping strategies at the end of the school term compared with children with similar characteristics in regular classes.
Variation in HCAP Effects Across Academies
The multiple linear regression model described above (with corrected standard errors) did not account for possible variation in treatment effects across academies. However, the enrichment program was offered at a local level, and the question of whether the treatment effect varied across the different local units is interesting and important. For instance, local units have different teachers/instructors and different student compositions, might emphasize different aims of the HCAP, and might be organized differently. Thus, it is possible that the effect of the HCAP could differ across academies for each outcome. Therefore, we also analyzed the data by applying a two-level model with a random intercept for academies and a random slope for the treatment effect that allowed the treatment effects to vary across academies.Footnote1
The pattern of results for the average effect estimates, based on the two-level analysis, was very similar to the findings reported for the main analysis (see and ). Across all imputed data sets, there was still an effect of program attendance on school grades. No other effects were statistically significant. In order to test whether the random slope variance differed from zero, we computed likelihood ratio tests in all imputed data sets and compared a model with both a random intercept and a random slope (full model) with a model with only a random intercept (restricted model) to test for differences across academies. For all outcome variables and in each data set, the likelihood ratio test was significant. Thus, there was significant random slope variance for all target outcomes. To describe the variation in treatment effects across the academies for each outcome, we calculated a 95% range of the random slope of the treatment variable based on the estimated variance of the random coefficient model. This 95% range contains the central 95% of the treatment effects around the average treatment effect (for similiar procedures see Lee & Thompson, Citation2005; Lingsma et al., Citation2011). For all outcomes, a large variation in treatment effects was observed across the academies (see ). This finding suggested a substantial amount of variability in the effect of the enrichment program depending on the academy in which the program was implemented.
Table 5. Average treatment effect on the treated (pooled across 20 imputed data sets)—Two-level model.
Discussion
The aim of the current study was to investigate the effectiveness of a statewide extracurricular enrichment program with respect to a broad range of students' characteristics. The program was developed to meet the needs of gifted elementary school children. In contrast to many recent empirical studies on the effectiveness of enrichment programs, we did not focus on the effectiveness of single courses with narrow topics. We evaluated the entire program, which had implemented what can be called a “grass roots strategy” in creating the courses. In line with calls for robust research designs in research on gifted students (Plucker & Callahan, Citation2014), we systematically investigated the effects of this program across one school term by employing a quasi-experimental design with two measurement points, data from several sources (teachers, parents, students), a large sample of third-grade students, and sophisticated statistical methods.
Program Effects on School Grades
Overall, the study's findings indicated a small but significant positive effect of the enrichment program on academic achievement (mathematics and German grades). No other effects were statistically significant. Children who had attended the enrichment program got better grades in mathematics and German than children in the control group with similar characteristics (e.g., intelligence, personality, and family background). School grades are very important in Germany because they form the basis of relevant decisions in school such as grade retention (European Commission, Citation2011) and secondary school track selection (see Maaz, Trautwein, Lüdtke, & Baumert, Citation2008). Depending on their school grades, elementary school children get a teacher's recommendation to attend a Gymnasium (academic track). If students attend a Gymnasium, they usually graduate after Grades 12 or 13, and passing the final exam (Abitur) is a prerequisite for university entrance.
There are several mechanisms that could explain this effect on academic achievement. First, it is possible that spending more time working on school-relevant topics (even if not elementary-school-relevant) than in unstructured leisure activities might account for the positive association between school grades and enrichment program attendance. Previous research that has investigated the effectiveness of structured extracurricular activities reported a benefit in school achievement for students who participated in such activities (e.g., Gerber, Citation1996; Marsh & Kleitman, Citation2002; Posner & Vandell, Citation1994, Citation1999). These findings can be explained by the identification/commitment model (Marsh, Citation1992), which is based on the participation-identification model (Finn, Citation1989). Finn posited that an increase in identification with school would predict positive academic and nonacademic outcomes (empirical support: Barber, Eccles, & Stone, Citation2001; Eccles & Barber, Citation1999). On the basis of Finn's idea, Marsh (Citation1991, Citation1992) further argued that school identification and school-related values are fostered by structured extracurricular activities and that this positively affects academic and nonacademic outcomes.
Second, the impact of the students' family environment may change (Stoeger, Steinbach, Obergriesser, & Matthes, Citation2014). Potentially, families become more supportive or more achievement oriented if they know that a child has been nominated for a program that was developed to meet the needs of gifted children (see Cornell, Citation1983; Cornell & Grossberg, Citation1989). Parents may spend (more) time and money on school-related topics. For instance, they might buy more books and newspapers for their children or support their child's attendance in additional courses or activities that are aimed at fostering their children. Children and parents might talk about school and school-related issues more often, and parents might reinforce good school grades even more than before.
The two above-mentioned explanations assume that academic achievement indeed actually improved because the students attended the enrichment program. Alternatively, it is also possible that teachers knew which students were taking extracurricular courses and that the effect on school grades was an expression of an expectation bias such as a halo effect (see Foster & Ysseldyke, Citation1976; Nisbett & Wilson, Citation1977). Here, the assumption is that school performance did not actually increase, but teachers' perceptions changed on the basis of students' program attendance. Effects of labeling a child as gifted are discussed in the literature and may influence the child him-/herself, the teachers, and family members (Berlin, Citation2009; for a short review see Coleman, Micko, & Cross, Citation2015). However, none of these studies suggests a direct link between program effectiveness and labeling. A closer look at the pattern of correlations between the outcome variables at pretest and posttest and the difference between these correlations showed that the correlation coefficients between grades and all other variables were not higher than −.45 (see Tables S3 and S4 in the SOM), and the differences between the pretest and posttest correlations were very small (see Table S5 in the SOM). This may indicate that the program could have affected grades without affecting investigative vocational interest, epistemic curiosity, creativity, self-control, self-concept, and social competence. In sum, given that the correlations between grades and the other measures were fairly similar at pretest and posttest, there is no indication that teachers systematically distorted students' grades.
Strengths and Limitations
The strengths of this study are its broad set of variables, large sample size, analysis approach, and the fact that this study was conducted in a natural in-school setting. Because of these aspects, we were able to estimate the program's effectiveness for children who attended the enrichment program compared with children with similar characteristics who did not attend the program without the use of a randomized controlled trial (Adelson, Citation2013; Fan & Nowell, Citation2011; Rubin & Thomas, Citation2000). Hence, for our target sample and under natural conditions, we were able to identify the (partial) effectiveness of the HCAP (see Flay, Citation1986; Flay et al., Citation2005; Gottfredson et al., Citation2015).
Some caution is necessary if researchers wish to generalize these findings to other programs and/or populations of gifted students (Ho et al., Citation2011; Lim, Marcus, Singh, Harris, & Seligson, Citation2014). First of all, compared with other evaluation studies of enrichment activities for gifted children (e.g., Mofield & Chakraborti-Ghosh, Citation2010; Peterson & Lorimer, Citation2011), the presented program evaluation did not examine the efficacy of an intervention under optimal conditions but instead investigated the effectiveness of the HCAP under “real-world” conditions (Flay et al., Citation2005). The enrichment program was applied as an extracurricular in-school program whose variety of course topics could be characterized as a “grass roots” course approach. The advantage is that many children with various interests were able to find a course that fit their specific needs. However, this study was less likely to find clear effects on specific outcome variables (see also Dai, Rinn, & Tan, Citation2012). A program that is aimed at fostering several interests and competencies may lack specific efficacy as evidenced in a particular domain, especially if the program effects are estimated across the entire range of courses that were offered. This is probably the reason why many empirical studies focus on specific intervention approaches (enrichment trainings) instead of programs with extraordinarily wide-ranging course topics. These studies—focusing on narrower course topics—also often provide evidence for positive intervention effects on a selected set of dependent variables (e.g., Callahan, Moon, Oh, Azano, & Hailey, Citation2015; Reis, McCoach, Little, Muller, & Kaniskan, Citation2011). However, investigating the effects of an enrichment program with various topics and examining its effects on a broad range of students' characteristics is similarly or even more interesting for the whole school system. It provides information about program effectiveness on variables that are not just similar to the ones used in the intervention (no teaching to the test).
Second, we were interested in global program effects instead of specific course effects, and thus we did not focus on a single course (e.g., spatial ability training) and did not examine the effects on one specific outcome variable (e.g., paper-folding test performance). However, with a “broad program,” it is a challenge to match the overall program goals with sound measurement instruments. Furthermore, reflecting typical real-world pedagogical approaches, there are comparably weak theoretical links between the program goals and specific outcomes. The principals of the HCAP did not rely on a specific model when they identified the dimensions in which gifted children should be fostered. They reached an agreement that they wanted to foster children with regard to cognitive abilities, academic achievement, investigative vocational interest, epistemic curiosity, creativity, self-control, self-concept, and social competencies; and the research question of this investigation was whether the whole program—across all academies—influences children's development in these domains.
Third, the study used a sample of “gifted” students who were nominated by their teachers. Teacher nomination practices have been extensively discussed in the literature (e.g., Card & Giuliano, Citation2015; Endepohls-Ulpe & Ruf, Citation2005; Gagné, Citation1994; Hunsaker, Finley, & Frank, Citation1997; Schack & Starko, Citation1990; Siegle, Moore, Mann, & Wilson, Citation2010). They have many advantages, including issues of practicability. However, they also entail some disadvantages. Importantly, it is well known that teacher nominations for gifted programs oftentimes result in rather heterogeneous samples, and this was also the case in the HCAP.
Finally, the sample size, the number of variables, and the complexity of the multilevel structure of the data made it impossible to analyze the data with a saturated multilevel model (random coefficients) that could account for variation on all levels (classes, schools, academies). Therefore, we analyzed the data by using a two-level model with a random intercept for academies and a random slope for the treatment effect that allowed the treatment effects to vary across academies. However, some caution is necessary when interpreting these findings because we used a matching approach that matched individuals between academies as opposed to within academies (because we wanted to keep the sample size as large as possible for finding an acceptable number of matched pairs; see Thoemmes & West, Citation2011). Therefore, many of the matched pairs consisted of children from different academies. Studies focusing specifically on the effectiveness of single academies or treatment-effect heterogeneity across academies require either stronger designs or sufficient sample sizes in each academy for within-cluster matching procedures (for further information about within- and cross-cluster matching and treatment-effect estimation, see Thoemmes & West, Citation2011).Footnote2
Conclusion and Outlook
The findings of this study suggest some strengths but also some caution with the approach of engaging in a “grass roots strategy” to create an extracurricular enrichment program for gifted elementary school children that is aimed at fostering students on a broad set of variables (e.g., abilities, interests, and creativity). This finding is in line with the conclusions drawn in a study conducted by Adelson et al. (Citation2012). They investigated the average effects of schools' gifted program policies in mathematics and reading on overall school achievement and the achievement and attitudes of gifted students as well as nongifted students. The policies could have included different kinds of programs/strategies to foster gifted students (i.e., acceleration, enrichment). They found no average effects of gifted programs on gifted students and concluded that the current gifted programs in schools in the United States do not appear to positively affect gifted students' achievement. However, it was not possible to distinguish between the effects of acceleration versus enrichment programs because the data did not provide the necessary information. The authors emphasized that specific, effective programs should be used to foster gifted students.
However, the gifted program in our study exclusively involved enrichment courses with different topics, and attending this program indeed positively affected students' school grades in mathematics and German. This finding might not necessarily have been expected because enrichment programs commonly aim to go beyond the regular curriculum to enhance current and future engagement in topics that are interesting to the students. This finding is interesting and valuable, although the driving mechanisms are not clear (see previous discussion). Taken together, school is an important environment for children and influences their cognitive and personal development (e.g., Roeser, Eccles, & Sameroff, Citation2000). Also, if special needs cannot be addressed in the regular curriculum, extracurricular enrichment activities hosted in schools seem to be useful. The advantage of such an approach is its accessibility for a large number of pupils with various background characteristics (see also Marsh & Kleitman, Citation2002). However, the present study's findings revealed positive enrichment program effects on mathematics and German grades only. As Adelson et al. (Citation2012) suggested, a closer look at specific or single courses is necessary to identify the kinds of training programs that indeed foster students' development broadly. At the time when our study was conducted, there were not many restrictions or rules for the HCAP courses. However, since then, the HCAP has changed and has gained more structure. For instance, specific STEM courses were developed and implemented in the program. Future research is necessary to investigate the long-term effects of single courses and the entire program.
UREE_A_1402396_supplementary.docx
Download MS Word (339 KB)Additional information
Funding
Notes
1 We previously specified a comprehensive four-level random coefficients model (students nested in classes, schools, and academies), but the model did not converge due to model complexity and low variation in each cluster level (for more details, see Tables A2 in the appendix and Tables S7 to S9 in the SOM).
2 Using within-cluster matching in our study decreased the sample size substantially. The two-level models did not converge. The models used to estimate the intraclass correlation coefficients also failed to converge.
References
- Adelson, J. L. (2013). Educational research with real-world data: Reducing selection bias with propensity scores. Practical Assessment, Research & Evaluation, 18(15), 1–11.
- Adelson, J. L., McCoach, D. B., & Gavin, M. K. (2012). Examining the effects of gifted programming in mathematics and reading using the ECLS-K. Gifted Child Quarterly, 56(1), 25–39. doi:10.1177/0016986211431487.
- Agreement between the State of Baden-Württemberg and the Hector Foundation II. (2010). Retrieved from http://www.hector-kinderakademie.de/site/hector/get/documents/KULTUS.HKA-BW/KULTUS-HKA/Portal/Dokumente/vereinbarung/KM-Hector_Vereinbarung_Errichtung_und_Foerderung_von_Kinderakademien.pdf.
- Arens, A. K., Trautwein, U., & Hasselhorn, M. (2011). Erfassung des Selbstkonzepts im mittleren Kindesalter: Validierung einer deutschen Version des SDQ I [Measuring self-concept in middle childhood: Validation of a German version of the SDQ I]. Zeitschrift für Pädagogische Psychologie, 25(2), 131–144. doi:10.1024/1010-0652/a000030.
- Arens, A. K., Yeung, A. S., Craven, R. G., & Hasselhorn, M. (2011). The twofold multidimensionality of academic self-concept: Domain specificity and separation between competence and affect components. Journal of Educational Psychology, 103(4), 970–981. doi:10.1037/a0025047.
- Barber, B. L., Eccles, J. S., & Stone, M. R. (2001). Whatever happened to the “Jock,” the “Brain,” and the “Princess”? Young adult pathways linked to adolescent activity involvement and social identity. Journal of Adolescent Research, 16(5), 429–455. doi:10.1177/0743558401165002.
- Becker, M., Neumann, M., Tetzner, J., Böse, S., Knoppick, H., Maaz, K., … Lehmann, R. (2014). Is early ability grouping good for high-achieving students' psychosocial development? Effects of the transition into academically selective schools. Journal of Educational Psychology, 106(2), 555–568. doi:10.1037/a0035425.
- Bergmann, C., & Eder, F. (1992). Allgemeiner Interessen-Struktur-Test. Umwelt-Struktur-Test [General interests structure test. Environment structure test]. Weinheim, Germany: Beltz.
- Berlin, J. E. (2009). It's all a matter of perspective: Student perceptions on the impact of being labeled gifted and talented. Roeper Review, 31(4), 217–223. doi:10.1080/02783190903177580.
- Bertrams, A., & Dickhäuser, O. (2009). Messung dispositioneller Selbstkontroll-Kapazität. Eine deutsche Adaptation der Kurzform der Self-Control Scale (SCS-K-D) [Measuring dispositional self-control capacity. A German adaption of the short form of the Self-Control Scale (SCS-K-D)]. Diagnostica, 55(1), 2–10. doi:10.1026/0012-1924.55.1.2.
- Byers, L. (1961). Ability grouping: Help or hindrance to social and emotional growth? The School Review, 69(4), 449–456. doi:10.1086/442601.
- Caliendo, M., & Kopeinig, S. (2008). Some practical guidance for the implementation of propensity score matching. Journal of Economic Surveys, 22(1), 31–72. doi:10.1111/J.1467-6419.2007.00527.X.
- Callahan, C. M., Moon, T. R., Oh, S., Azano, A. P., & Hailey, E. P. (2015). What works in gifted education: Documenting the effects of an integrated curricular/instructional model for gifted students. American Educational Research Journal, 52(1), 137–167. doi:10.3102/0002831214549448.
- Card, D., & Giuliano, L. (2015). Can universal screening increase the representation of low income and minority students in gifted education? (NBER Working Paper No. 21519). Cambridge, MA: National Bureau of Economic Research.
- Cham, H., & West, S. G. (2016). Propensity score analysis with missing data. Psychological Methods, 21(3), 427–445. doi:10.1037/met0000076.
- Cochran, W. G., & Rubin, D. B. (1973). Controlling bias in observational studies: A review. Sankhyā: The Indian Journal of Statistics, Series A (1961–2002), 35(4), 417–446.
- Coleman, L. J., Micko, K. J., & Cross, T. L. (2015). Twenty-five years of research on the lived experience of being gifted in school: Capturing the students' voices. Journal for the Education of the Gifted, 38(4), 358–376. doi:10.1177/0162353215607322.
- Cook, T. D., Steiner, P. M., & Pohl, S. (2009). Assessing how bias reduction is influenced by covariate choice, unreliability, and data analytic mode: An analysis of different kinds of within-study comparisons in different substantive domains. Multivariate Behavioral Research, 44(6), 828–847. doi:10.1080/00273170903333673.
- Cornell, D. G. (1983). Gifted children: The impact of positive labeling on the family system. American Journal of Orthopsychiatry, 53(2), 322–335. doi:10.1111/j.1939-0025.1983.tb03376.x.
- Cornell, D. G., & Grossberg, I. N. (1989). Parent use of the term “gifted”: Correlates with family environment and child adjustment. Journal for the Education of the Gifted, 12(3), 218–230. doi:10.1177/016235328901200305.
- Coxon, S. V. (2012). The malleability of spatial ability under treatment of a FIRST LEGO League-based robotics simulation. Journal for the Education of the Gifted, 35(3), 291–316. doi:10.1177/0162353212451788.
- Dai, D. Y., Rinn, A. N., & Tan, X. (2012). When the big fish turns small: Effects of participating in gifted summer programs on academic self-concept. Journal of Advanced Academics, 24(1), 4–26. doi:10.1177/1932202X12473425.
- Dai, D. Y., Swanson, J. A., & Cheng, H. (2011). State of research on giftedness and gifted education: A survey of empirical studies published during 1998–2010. Gifted Child Quarterly, 55(2), 126–138. doi:10.1177/0016986210397831.
- Eccles, J. S., & Barber, B. L. (1999). Student council, volunteering, basketball, or marching band: What kind of extracurricular involvement matters? Journal of Adolescent Research, 14(1), 10–43. doi:10.1177/0743558499141003.
- Ekstrom, R. B. (1961). Experimental studies of homogeneous grouping: A critical review. The School Review, 69(2), 216–226. doi:10.1086/442582.
- Endepohls-Ulpe, M., & Ruf, H. (2005). Primary school teachers' criteria for the identification of gifted pupils. High Ability Studies, 16(2), 219–228. doi:10.1080/13598130600618140.
- European Commission. (2011). Grade retention during compulsory education in Europe: Regulations and statistics. Retrieved from http://www.eurydice.org
- Fan, X., & Nowell, D. L. (2011). Using propensity score matching in educational research. Gifted Child Quarterly, 55(1), 74–79. Retrieved from http://doi.org/10.1177/0016986210390635.
- Feldhusen, J. F., & Moon, S. M. (1992). Grouping gifted students—Issues and concerns. Gifted Child Quarterly, 36(2), 63–67. doi:10.1177/001698629203600202.
- Feldman, D. H. (1986). Nature's gambit: Child prodigies and the development of human potential. New York, NY: Basic Books.
- Finn, J. D. (1989). Withdrawing from school. Review of Educational Research, 59(2), 117–142. doi:10.3102/00346543059002117.
- Flay, B. R. (1986). Efficacy and effectiveness trials (and other phases of research) in the development of health promotion programs. Preventive Medicine, 15(5), 451–474. doi:10.1016/0091-7435(86)90024-1.
- Flay, B. R., Biglan, A., Boruch, R. F., Castro, F. G., Gottfredson, D., Kellam, S., . . . Ji, P. (2005). Standards of evidence: Criteria for efficacy, effectiveness and dissemination. Prevention Science, 6(3), 151–175. doi:10.1007/s11121-005-5553-y.
- Foster, G., & Ysseldyke, J. (1976). Expectancy and halo effects as a result of artificially induced teacher bias. Contemporary Educational Psychology, 1(1), 37–45. doi:10.1016/0361-476X(76)90005-9.
- Fraleigh-Lohrfink, K. J., Schneider, M. V., Whittington, D., & Feinberg, A. P. (2013). Increase in science research commitment in a didactic and laboratory-based program targeted to gifted minority high-school students. Roeper Review, 35(1), 18–26. doi:10.1080/02783193.2013.740599.
- Gagné, F. (1994). Are teachers really poor talent detectors—Comments on Pegnato and Birch's (1959) study of the effectiveness and efficiency of various identification techniques. Gifted Child Quarterly, 38(3), 124–126. doi:10.1177/001698629403800305.
- Gagné, F. (2005). From gifts to talents: The DMGT as a developmental model. In R. J. Sternberg & J. E. Davidson (Eds.), Conceptions of giftedness (2nd ed., pp. 98–119). Cambridge, England: Cambridge University Press.
- Gear, G. H. (1978). Effects of training on teachers' accuracy in the identification of gifted children. Gifted Child Quarterly, 22(1), 90–97. doi:10.1177/001698627802200121.
- Gerber, S. B. (1996). Extracurricular activities and academic achievement. Journal of Research and Development in Education, 30(1), 42–50.
- Gottfredson, D. C., Cook, T. D., Gardner, F. E., Gorman-Smith, D., Howe, G. W., Sandler, I. N., & Zafft, K. M. (2015). Standards of evidence for efficacy, effectiveness, and scale-up research in prevention science: Next generation. Prevention Science, 16(7), 893–926. doi:10.1007/s11121-015-0555-x.
- Gouley, K. K., Brotman, L. M., Huang, K.-Y., & Shrout, P. E. (2008). Construct validation of the social competence scale in preschool-age children. Social Development, 17(2), 380–398. doi:10.1111/j.1467-9507.2007.00430.x.
- Graham, J. W., Taylor, B. J., Olchowski, A. E., & Cumsille, P. E. (2006). Planned missing data designs in psychological research. Psychological Methods, 11(4), 323–343. doi:10.1037/1082-989x.11.4.323.
- Gu, X. S., & Rosenbaum, P. R. (1993). Comparison of multivariate matching methods: Structures, distances, and algorithms. Journal of Computational and Graphical Statistics, 2(4), 405–420. doi:10.1080/10618600.1993.10474623
- Gubbels, J., Segers, E., & Verhoeven, L. (2014). Cognitive, socioemotional, and attitudinal effects of a triarchic enrichment program for gifted children. Journal for the Education of the Gifted, 37(4), 378–397. doi:10.1177/0162353214552565.
- Guilford, J. P. (1967). The nature of human intelligence. New York, NY: McGraw-Hill.
- Hansen, B. B. (2004). Full matching in an observational study of coaching for the SAT. Journal of the American Statistical Association, 99(467), 609–618. doi:10.1198/0162145000000647.
- Hany, E. A. (1997). Modeling teachers' judgment of giftedness: A methodological inquiry of biased judgment. High Ability Studies, 8(2), 159–178. doi:10.1080/1359813970080203.
- Harradine, C. C., Coleman, M. R. B., & Winn, D. M. C. (2014). Recognizing academic potential in students of color: Findings of U-STARS similar to PLUS. Gifted Child Quarterly, 58(1), 24–34. doi:10.1177/0016986213506040.
- Heller, K. A. (2005). The Munich Model of Giftedness and its impact on identification and programming. Gifted and Talented International, 20, 30–36. doi:10.1080/15332276.2005.11673055.
- Heller, K. A., Perleth, C., & Keng Lim, T. (2005). The Munich Model of Giftedness designed to identify and promote gifted students. In R. J. Sternberg & J. E. Davidson (Eds.), Conceptions of giftedness (2nd ed.). Cambridge, England: Cambridge University Press.
- Ho, D. E., Imai, K., King, G., & Stuart, E. A. (2011). MatchIt: Nonparametric preprocessing for parametric causal inference. Journal of Statistical Software, 42(8), 1–28. doi:10.18637/jss.v042.i08.
- Ho, D. E., Imai, K., King, G., & Stuart, E. (2013). The MatchIt package (Version 2.4—20) [Computer software]. Retrieved from http://gking.harvard.edu/matchit/
- Holland, J. L. (1997). Making vocational choices: A theory of vocational personalities and work environments (3rd ed.). Odessa, FL: Psychological Assessment Resources.
- Hunsaker, S. L., Finley, V. S., & Frank, E. L. (1997). An analysis of teacher nominations and student performance in gifted programs. Gifted Child Quarterly, 41(2), 19–24. doi:10.1177/001698629704100203.
- Kaul, C. R., Johnsen, S. K., Saxon, T. F., & Witte, M. M. (2016). Project Promise: A long-term follow-up of low-income gifted students who participated in a summer enrichment program. Journal for the Education of the Gifted, 39(2), 1–20. doi:10.1177/0162353216640938.
- Kim, K. H. (2006). Can we trust creativity tests? A review of the Torrance Tests of Creative Thinking (TTCT). Creativity Research Journal, 18(1), 3–14. doi:10.1207/s15326934crj1801_2.
- Kim, M. (2016). A meta-analysis of the effects of enrichment programs on gifted students. Gifted Child Quarterly, 60(2), 102–116. doi:10.1177/0016986216630607.
- Kretschmann, J., Vock, M., & Lüdtke, O. (2014). Acceleration in elementary school: Using propensity score matching to estimate the effects on academic achievement. Journal of Educational Psychology, 106(4), 1080–1095. doi:10.1037/A0036631.
- Kulik, J. A., & Kulik, C-L. C. (1992). Meta-analytic findings on grouping programs. Gifted Child Quarterly, 36(2), 73–77. doi:10.1177/001698629203600204.
- Lee, K. J., & Thompson, S. G. (2005). The use of random effects models to allow for clustering in individually randomized trials. Clinical Trials, 2(2), 163–173. doi:10.1191/1740774505cn082oa.
- Lee, S.-Y., Olszewski-Kubilius, P., & Peternel, G. (2010). Achievement after participation in a preparatory program for verbally talented students. Roeper Review, 32(3), 150–163. doi:10.1080/02783193.2010.485301.
- Lim, S., Marcus, S. M., Singh, T. P., Harris, T. G., & Seligson, A. L. (2014). Bias due to sample selection in propensity score matching for a supportive housing program evaluation in New York City. Plos One, 9(10). doi:10.1371/journal.pone.0109112.
- Lingsma, H. F., Roozenbeek, B., Perel, P., Roberts, I., Maas, A. I. R., & Steyerberg, E. W. (2011). Between-centre differences and treatment effects in randomized controlled trials: A case study in traumatic brain injury. Trials, 12. doi:10.1186/1745-6215-12-201.
- Litman, J. A., & Spielberg, C. D. (2003). Measuring epistemic curiosity and its diversive and specific components. Journal of Personality Assessment, 80(1), 75–86. doi:10.1207/S15327752JPA8001_16.
- Little, T. D., & Rhemtulla, M. (2013). Planned missing data designs for developmental researchers. Child Development Perspectives, 7(4), 199–204. doi:10.1111/cdep.12043.
- Lumley, T. (2016). Survey: Analysis of complex survey samples. R package version 3.32.
- Maaz, K., Trautwein, U., Lüdtke, O., & Baumert, J. (2008). Educational transitions and differential learning environments: How explicit between-school tracking contributes to social inequality in educational outcomes. Child Development Perspectives, 2(2), 99–106. doi:10.1111/j.1750-8606.2008.00048.x.
- Marcus, B. (2003). An empirical examination of the construct validity of two alternative self-control measures. Educational and Psychological Measurement, 63(4), 674–706. doi:10.1177/0013164403251329.
- Marsh, H. W. (1990). A multidimensional, hierarchical model of self-concept: Theoretical and empirical justification. Educational Psychology Review, 2(2), 77–172. doi:10.1007/BF01322177.
- Marsh, H. W. (1991). Employment during high school: Character building or a subversion of academic goals. Sociology of Education, 64(3), 172–189. doi:10.2307/2112850.
- Marsh, H. W. (1992). Extracurricular activities: Beneficial extension of the traditional curriculum or subversion of academic goals. Journal of Educational Psychology, 84(4), 553–562. doi:10.1037/0022-0663.84.4.553.
- Marsh, H. W., & Kleitman, S. (2002). Extracurricular school activities: The good, the bad, and the nonlinear. Harvard Educational Review, 72(4), 464–514. doi:10.17763/haer.72.4.051388703v7v7736.
- McCoach, D. B., Gubbins, E. J., Foreman, J., Rubenstein, L. D., & Rambo-Hernandez, K. E. (2014). Evaluating the efficacy of using predifferentiated and enriched mathematics curricula for Grade 3 students: A multisite cluster-randomized trial. Gifted Child Quarterly, 58(4), 272–286. doi:10.1177/0016986214547631.
- Mitra, R., & Reiter, J. P. (2012). A comparison of two methods of estimating propensity scores after multiple imputation. Statistical Methods in Medical Research, 25(1), 188–204. doi:10.1177/0962280212445945.
- Mofield, E. L., & Chakraborti-Ghosh, S. (2010). Addressing multidimensional perfectionism in gifted adolescents with affective curriculum. Journal for the Education of the Gifted, 33(4), 479–513. doi:10.1177/016235321003300403.
- Nagengast, B., Marsh, H. W., & Hau, K-T. (2013). Effects of single-sex schooling in the final years of high school: A comparison of analysis of covariance and propensity score matching. Sex Roles, 69(7–8), 404–422. doi: 10.1007.s11199-013-0261-8.
- Neber, H. (2004). Teacher identification of students for gifted programs: Nominations to a summer school for highly-gifted students. Psychology Science, 46(3), 348–362.
- Neihart, M. (2007). The socioaffective impact of acceleration and ability grouping: Recommendations for best practice. Gifted Child Quarterly, 51(4), 330–341. doi:10.1177/0016986207306319.
- Newland, T. (1976). The gifted in historical perspective. Englewood Cliffs, NJ: Prentice Hall.
- Nisbett, R. E., & Wilson, T. D. (1977). The halo effect: Evidence for unconscious alteration of judgments. Journal of Personality and Social Psychology, 35(4). doi:10.1037/0022-3514.35.4.250.
- Olszewski-Kubilius, P., & Thomson, D. (2010). Gifted programming for poor and minority urban students: Issues and lessons learned. Gifted Child Today, 33, 58–64. doi:10.1177/107621751003300413.
- Park, G., Lubinski, D., & Benbow, C. P. (2013). When less is more: Effects of grade skipping on adult STEM productivity among mathematically precocious adolescents. Journal of Educational Psychology, 105(1), 176–198. doi:10.1037/a0029481.
- Peterson, J. S., & Lorimer, M. R. (2011). Student response to a small-group affective curriculum in a school for gifted children. Gifted Child Quarterly, 55(3), 167–180. doi:10.1177/0016986211412770.
- Piirto, J. (1994). Talented children and adults: Their development and education. New York, NY: Macmillan.
- Piotrowski, J. T., Litman, J. A., & Valkenburg, P. (2014). Measuring epistemic curiosity in young children. Infant and Child Development, 23(5), 542–553. doi:10.1002/icd.1847.
- Plucker, J. A., & Callahan, C. M. (2014). Research on giftedness and gifted education: Status of the field and considerations for the future. Exceptional Children, 80(4), 390–406. doi:10.1177/0014402914527244.
- Posner, J. K., & Vandell, D. L. (1994). Low-income children's after-school care: Are there beneficial effects of after-school programs? Child Development, 65(2), 440–456. doi:10.2307/1131395.
- Posner, J. K., & Vandell, D. L. (1999). After-school activities and the development of low-income urban children: A longitudinal study. Developmental Psychology, 35(3), 868–879. doi:10.1037/0012-1649.35.3.868.
- Raghunathan, T. E., Solenberger, P. W., & van Hoewyk, J. (2002). IVEware: Imputation and variance estimation software. User guide. Ann Arbor, MI: Survey Research Center, Institute for Social Research, University of Michigan.
- Reis, S. M., McCoach, D. B., Little, C. A., Muller, L. M., & Kaniskan, R. B. (2011). The effects of differentiated instruction and enrichment pedagogy on reading achievement in five elementary schools. American Educational Research Journal, 48(2), 462–501. doi:10.3102/0002831210382891.
- Renzulli, J. S. (1978). What makes giftedness? Reexamining a definition. Phi Delta Kappan, 60(3), 180–184. doi:10.1177/003172171109200821
- Roeser, R. W., Eccles, J. S., & Sameroff, A. J. (2000). School as a context of early adolescents' academic and social-emotional development: A summary of research findings. The Elementary School Journal, 100(5), 443–471. doi:10.1086/499650.
- Rogers, K. B. (1991). The relationship of grouping practices to the education of the gifted and talented learner: Executive summary. (Report No. 1). Storrs, CT: National Research Center on the Gifted and Talented.
- Rogers, K. B. (2007). Lessons learned about educating the gifted and talented: A synthesis of the research on educational practice. Gifted Child Quarterly, 51(4), 382–396. doi:10.1177/0016986207306324.
- Rosenbaum, P. R. (1984). The consequences of adjustment for a concomitant variable that has been affected by the treatment. Journal of the Royal Statistical Society. Series A (General), 147(5), 656–666. doi:10.2307/2981697.
- Rosenbaum, P. R. (2002). Observational studies (2nd ed.). New York, NY: Springer Verlag.
- Rosenbaum, P. R., & Rubin, S. B. (1983). The central role of the propensity score in observation studies for causal effects. Biometrika, 70(1), 41–55. doi:10.1093/biomet/70.1.41.
- Rothenbusch, S., Zettler, I., Voss, T., Lösch, T., & Trautwein, U. (2016). Exploring reference group effects on teachers' nominations of gifted students. Journal of Educational Psychology, 108(6), 883–897. doi:10.1037/edu0000085.
- Rubin, D. B. (1987). Multiple imputation for nonresponse in surveys. New York, NY: J. Wiley & Sons.
- Rubin, D. B. (2001). Using propensity scores to help design observational studies: Application to the tobacco litigation. Health Services & Outcomes Research Methodology, 2(3), 169–188. doi:10.2277/0521674360.
- Rubin, D. B., & Thomas, N. (2000). Combining propensity score matching with additional adjustments for prognostic covariates. Journal of the American Statistical Association, 95(450), 573–585. doi:10.2307/2669400.
- Schack, G. D., & Starko, A. J. (1990). Identification of gifted students: An analysis of criteria preferred by preservice teachers, classroom teachers, and teachers of the gifted. Journal for the Education of the Gifted, 13(4), 346–363. doi:10.1177/016235329001300405 .
- Schafer, J. L., & Graham, J. W. (2002). Missing data: Our view of the state of the art. Psychological Methods, 7(2), 147–177. doi:10.1037//1082-989x.7.2.147.
- Schafer, J. L., & Kang, J. (2008). Average causal effects from nonrandomized studies: A practical guide and simulated example. Psychological Methods, 13(4), 279–313. doi:10.1037/A0014268.
- Schröders, U., Schipolowski, S., Zettler, I., Golle, J., & Wilhelm, O. (2016). Do the smart get smarter? Development of fluid and crystallized intelligence in 3rd grade. Intelligence, 59, 84–95. doi:10.1016/j.intell.2016.08.003.
- Schulthess-Singeisen, L., Neuenschwander, M. P., & Herzog, W. (2008). Entwicklung des schulischen Fähigkeitsselbstkonzepts bei Primarschulkindern mit einer Lehrernomination als hochbegabt [Development of academic self-concept of primary school children nominated as being gifted by their teachers]. Psychologie in Erziehung und Unterricht, 55(2), 143–151. doi:10.5167/uzh-6181
- Siegle, D., Gubbins, E. J., O'Rourke, P., Langley, S. D., Little, C. A., McCoach, D. B., . . . Plucker, J. A. (2016). Barriers to underserved students' participation in gifted programs and possible solutions. Journal for the Education of the Gifted, 39(2), 103–131. doi:10.1177/0162353216640930.
- Siegle, D., Moore, M. M., Mann, R. L., & Wilson, H. E. (2010). Factors that influence in-service and preservice teachers' nominations of students for gifted and talented programs. Journal for the Education of the Gifted, 33(3), 337–360. doi:10.1177/016235321003300303.
- Snijders, T. A. B., & Bosker, R. J. (2012). Multilevel analysis: An introduction to basic and advanced multilevel modeling (2nd ed.). London: Sage Publishers.
- Stanley, J. C. (1976). The case for extreme educational acceleration of intellectually brilliant youths. Gifted Child Quarterly, 20(1), 66–75. doi:10.1177/001698627602000120.
- Steiner, P. M., Cook, T. D., Shadish, W. R., & Clark, M. H. (2010). The importance of covariate selection in controlling for selection bias in observational studies. Psychological Methods, 15(3), 250–267. doi:10.1037/A0018719.
- Sternberg, R. J. (2003). WICS as a model of giftedness. High Ability Studies, 14(2), 109–137. doi:10.1080/1359813032000163807.
- Sternberg, R. J., & Davidson, J. E. (2005). Conceptions of giftedness (2nd ed). New York, NY: Cambridge University Press.
- Stoeger, H., Steinbach, J., Obergriesser, S., & Matthes, B. (2014). What is more important for fourth-grade primary school students for transforming their potential into achievement: The individual or the environmental box in multidimensional conceptions of giftedness? High Ability Studies, 25(1), 5–21. doi:10.1080/13598139.2014.914381.
- Stuart, E. A. (2010). Matching methods for causal inference: A review and a look forward. Statistical Science, 25(1), 1–21. doi:10.1214/09-STS313.
- Subotnik, R. F., Olszewski-Kubilius, P., & Worrell, F. C. (2011). Rethinking giftedness and gifted education: A proposed direction forward based on psychological science. Psychological Science in the Public Interest, 12(1), 3–54. doi:10.1177/1529100611418056.
- Subotnik, R. F., Olszewski-Kubilius, P., & Worrell, F. C. (2012). A proposed direction forward for gifted education based on psychological science. Gifted Child Quarterly, 56(4), 176–188. doi:10.1177/0016986212456079.
- Tangney, J. P., Baumeister, R. F., & Boone, A. L. (2004). High self-control predicts good adjustment, less pathology, better grades, and interpersonal success. Journal of Personality, 72(2), 271–324. doi:10.1111/j.0022-3506.2004.00263.x.
- Tannenbaum, A. J. (1983). Gifted children: Psychological and educational perspectives. New York, NY: Macmillan.
- Terman, L. M. (1925). Genetic studies of genius (Vol. I). Stanford, CA: Stanford University Press.
- Thoemmes, F. J., & Kim, E. S. (2011). A systematic review of propensity score methods in the social sciences. Multivariate Behavioral Research, 46(1), 90–118. doi:10.1080/00273171.2011.540475.
- Thoemmes, F. J., & West, S. G. (2011). The use of propensity scores for nonrandomized designs with clustered data. Multivariate Behavioral Research, 46(3), 514–543. doi:10.1080/00273171.2011.569395.
- Thompson, B., & Subotnik, R. F. (2010). Methodologies for conducting research on giftedness. Washington, DC: American Psychological Association.
- Tracey, T. J. G., & Ward, C. C. (1998). The structure of children's interests and competence perceptions. Journal of Counseling Psychology, 45(3), 290–303. doi:10.1037/0022-0167.45.3.290.
- VanTassel-Baska, J. (2006). A content analysis of evaluation findings across 20 gifted programs: A clarion call for enhanced gifted program development. Gifted Child Quarterly, 50(3), 199–215. doi:10.1177/001698620605000302.
- Vaughn, V. L., Feldhusen, J. F., & Asher, J. W. (1991). Meta-analyses and review of research on pull-out programs in gifted education. Gifted Child Quarterly, 35(2), 92–98. doi:10.1177/001698629103500208.
- What Works Clearinghouse. (2014). Procedures and Standards Handbook Version 3.0. Retrieved from What Works Clearinghouse: https://ies.ed.gov/ncee/wwc/Handbooks
- Wilhelm, O., Schroeders, U., & Schipolowski, S. (2014). Berliner Test zur Erfassung fluider und kristalliner Intelligenz für die 8. bis 10. Jahrgangsstufe (BEFKI 8–10) [Berlin test of fluid and crystallized intelligence for Grades 8–10]. Göttingen, Germany: Hogrefe.
- Wirthwein, L., Becker, C. V., Loehr, E-V., & Rost, D. H. (2011). Overexcitabilities in gifted and non-gifted adults: Does sex matter? High Abilities Studies, 22(2), 145–153. doi:10.1080/13598139.2011.622944.
- Ziegler, A., & Phillipson, S. N. (2012). “Towards a systemic theory of gifted education”: Connectedness and life skills development for all children. High Ability Studies, 23(1), 119–121. doi:10.1080/13598139.2012.679121.
Appendix
Table A1. Standardized mean differences before and after matching.
Table A2. Variance partition coefficients (VPCs) for all posttest measures.