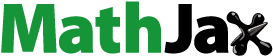
ABSTRACT
Rock fragmentation is a common physical and mechanical phenomenon that exists in a variety of ranges from the mining and processing. Communication is an important operation in coal processing whereby the coal block coming from coalmines is crushed into fragmentations with reduced size, which accounts for 80% of the electricity consumption of mineral processing circuits. Fragmentation energy, which is related to the coal particle size distribution, is an essential parameter for improving the efficiency of shearer, road-header, and crusher. Coal particles are generally simplified into fine or near spherical shapes for analytical and numerical simulation purposes. This research aims to establish the fragmentation energy calculation model for coal fragmentations with coarse and wide size distribution based on the analysis of size distribution and energy-size relationships of coal particles. It has been demonstrated by the results that the fragmentation energy of impact load crushed coal can be determined based on Rittinger’s theory with fractal or RR model particle size distribution.
Introduction
Rock fragmentation is a common physical and mechanical phenomenon that exists in a variety of ranges from the mining and processing (Trechera et al. Citation2022; Zhu et al. Citation2023). In underground mining or development face, cutting of coal is the key step of mining process, which consumes 80%-90% power of the entire shearer and road-header (X. H. Liu, Liu, and Tang Citation2015). Communication is an important operation in coal processing whereby the coal block coming from coalmines is crushed into fragmentations with reduced size, which accounts for 80% of the electricity consumption of mineral processing circuits (Numbi and Xia Citation2015, Citation2016). More importantly, the cracks within coal blocks formed by impact and seismic load will lead to the sudden fragmentation of underground structures, which will significantly affect mining safety and efficiency (J. W. Cheng et al. Citation2023; Yang, Ren, and Tan Citation2020a). Hence, thorough research has been made by researchers around the energy consumption of rock/coal fragmentation process.
Fragmentation energy, which is related to the coal particle size distribution, is an essential parameter for improving the efficiency of shearer, road-header, and crusher. The relationship between the fragmentation energy and the particle size for single-sized particles has been extensively researched over the last century. Energy-size equations based on theoretical and experimental studies have been put forward by Rittinger, Kick and Bond (Morrell Citation2008), known as the three theories of comminution. However, these experimental data and theoretical equations mentioned in the literature are mostly limited to rock or other ores, or pulverized coal (Kossovich et al. Citation2023). Besides, in many researches, coal particles are generally simplified into fine or near spherical shapes for analytical and numerical simulation purposes (Chen et al. Citation2020; Z. F. Cheng and Zhu Citation2015). Zhou et al. proposed six different agglomerate polyhedrons to characterize the shape of coal particles and discussed the shape effect on pneumatic conveying process (Zhou, Liu, Du, et al. Citation2017; Zhou, Liu, Liu, et al. Citation2017). In these particle size and shape characteristic models, fractal equation is widely used to describe the particle size distribution of coal/rock particles (Qin et al. Citation2022).
This research aims to establish the fragmentation energy calculation model for coal fragmentations with coarse and wide size distribution based on the analysis of size distribution and energy-size relationships of coal particles. A drop weight impact system was used to crush the coal into particles with designed energy input. The statistical mass-size curves of coal particles crushed by impact load were obtained based on manual sieving and digital image processing technique, which is used to validate the fractal and Rosin-Ramler (RR) model distribution curve of coal particles. The fragmentation energy calculated based on different size distribution model and energy-size relationships were compared with the impact energy input to determine the effectiveness of each method. It has been demonstrated by the results that the fragmentation energy of impact load crushed coal can be determined based on Rittinger’s theory with fractal or RR model PSD distribution.
Analytical method
Particle size distribution
For coal fragmentation and crushing process, the coal fragments satisfy specific size distribution law. Hence, the particle size distribution (PSD) needs to be taken into consideration for the calculation of fragmentation energy. The most widely used mathematical equations mainly used to describe PSD are the Rosin-Ramler (R-R), the Gate-Gaudin-Schuhmann (G-G-S) and the fractal distribution.
The GGS model can be given by the following expression (MacıMacıAs-Garcıa, Cuerda-Correa, and Dıaz-Dıez Citation2004; Petrakis, Stamboliadis, and Komnitsas Citation2017):
Where is the cumulative mass fraction of the fragments smaller than size
,
is the maximum size of PSD and
is the adjustable parameter depended on rock properties.
The RR model is defined by (Bu et al. Citation2020; Rosin and Rammler Citation1933):
Where is the characteristic fragment size in mm usually corresponding to 63.2% of the total volume of distribution
.
The Fractal model can be written as (Ullah et al. Citation2022):
Where is the fractal dimension of PSD, which is related to rock properties.
It can be seen from the equations that the mathematical expressions of GGS model and fractal model are the same concept. Hence, only fractal and RR models are compared for the calculation of coal fragmentation energy in this study.
Energy-size relationship
The relationships between fragmentation size and specific energy consumption have been thoroughly studied by many researchers. Rittinger believed that the energy consumed by mineral fragmentation is proportional to the new surface area generated as all the energy is dissipated by overcoming the molecular cohesion among new fragments surfaces (Du et al. Citation2022). Taking the coal fragment as platonic solid or sphere, the volume of fragment is directly proportional to the cubic of fragment size while the surface area is directly proportional to the square of size. The surface area change of coal after fragmentation can be expressed as:
Where is the surface area change of coal,
is the total volume of framents,
is the fragment size after failure,
is the equivalent size of total fragments before failure and
is shape factor which is the constant related to fragment shape.
Hence, Rittinger’s theory can be written in the following equation:
Where is fragmentation energy consumed by formation of per unit surface area, also called Rittinger constant, which is only related to the mineral properties.
Kick proposed that the energy required for mineral fragmentation with a given size is proportional to the volume of resulting fragments (Locat et al. Citation2003). Tavares et al. believe that Kick’s theory is suitable for fragmentation energy calculation of relatively large particles based on experimental data (Tavares and King Citation1998). Kick’s theory can be expressed as:
Where is energy index in Kick’s theory, which depends on mineral properties.
Bond’s theory suggests that deformation happens initially inside the intact rock or ore under applied forces until a threshold is reached at which the crack emerges (J. X. Liu et al. Citation2016). Hence, the fragmentation energy is proportional to the total length of the new cracks generated during coal fragmentation. The resulting equation based on Bond’s theory is:
Where is a constant named Bond’s index, which is related to the mineral properties.
However, the above fragmentation energy calculation equations are for single sized particles. To determine the fragmentation energy for wide range of particle sizes, the equation needs to be in differential form by considering the particle size distribution, as listed in . In , W is the total weight of coal fragments and is density of coal.
Table 1. Fragmentation energy differential formula.
Experimental
Impact crushing tests
The impact crushing test of coal was conducted in laboratory to get the crushed coal particles. The impact load was achieved by a drop weight system as shown in . The drop weight with 73.35 kg was released from a height of 2.5 m, which can accelerate the drop weight maximum to 7 m/s. It has been verified by previous application this test system that the energy loss due to the internal frictions of drop hammer system can be negligible (Yang et al. Citation2021). To guide the direction of impact load, an additional test set consists of transmission bar, bolt and base was placed right under the drop weight. The transmitted and reflected energy of transmission bar subject to different impact velocity have been thoroughly studied (Feng et al. Citation2018). Based on energy conservation, the energy dissipation can be defined using Equationequation (8)(8)
(8) :
where is the fragmentation energy,
is the kinetic energy driving the coal particle movement,
is the total impact energy,
is the reflected energy of reflected wave
is the transmitted energy of transmitted wave.
The sum of fragmentation and kinetic energy also called dissipated energy as this is the energy dissipated by rock fracture and projectile subject to dynamic loads.
Coal sample was placed between the transmission bar and the base. Coal sample used in this test was 50 mm *50 mm *100 mm cuboid coal blocks as shown in . Coal samples were sourced from local Australian coal mines with 7-10MPa uniaxial compression strength. The crushed coal particles were collected after particle size distribution analysis once the impact test was done.
Digital image processing
Generally, the size distribution of coal particles can be measured by manual sieving, which is time-consuming and low-efficiency (Hou et al. Citation2015). Besides, further damage to coal particles may be caused by vibration during sieving process, which will affect the accuracy of measurement results. Especially for larger coal particles with natural weaknesses or bedding, manual sieving will break coal particles into smaller size. Digital image processing method, developed based on the application of digital image acquisition equipment, process software package and mathematical analysis algorithm, enables the fast and accurate measurement of size distribution of solid particles (Wu and Yu Citation2012). Previous research has demonstrated the application feasibility of digital image processing method for size distribution measurement of coal particles ranging from 2.5 to 80 mm (Yang, Ren, and Tan Citation2020b).
To determine the particle distribution curve for fractal and RR models, the cumulative distribution curve of coal particles was measured by combined manual and digital sieving. Manual sieving was used to determine the cumulative mass at three sizes including 2.5, 5 and 10 mm. All other particles larger than 10 mm were measured by digital image processing. As shown in , digital image processing includes two main steps: image acquisition and image analysis. The acquisition of high-resolution image is achieved by a fixed Nikon D60 camera remotely controlled by mobile phone through wireless connection. The wireless control of the camera can ensure the image quality by avoiding the shadow caused by the camera shakiness. The image analysis is conducted by Image Processing Toolbar of MATLAB R2020a software. More detailed introduction of digital image processing procedure can be found in the literature (Yang, Ren, and Tan Citation2020b). The image analysis code is attached as Appendix A at the end of this paper.
Figure 2. Procedure of Digital Image Processing (after (Yang, Ren, and Tan Citation2020b)).
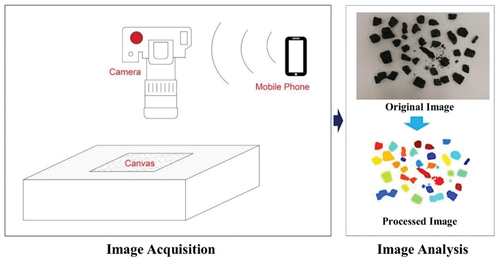
The data acquired by digital image analysis includes maximum axis length, minor axis length and area of each particle. The particle area, which is the two-dimensional pixel area forming each particle on the image, will be used to calculation the shape parameter for example equivalent diameter of each particle. All the data are originally in pixels and then transformed into millimeter through scale calibration.
Results
According to Equationequation (1)(1)
(1) , the particle size distribution determined by manual and digital sieving is plotted in . The measured data was used to calibrate the fractal and RR particle distribution model. To get the best fitting results to the measured data, the fractal and RR model can be evaluated by the root mean square error (RMSE) and coefficient of determination (R2) from the following equations (Bu et al. Citation2019):
where is the cumulative mass fraction measured by manual or digital sieving,
is the cumulative mass fraction estimated by fractal distribution or RR model,
is the mean value of all experimental data, and
is the number of data points.
According to the reference value of distribution parameter for each model in , the RMSE is calculated for both fractal and RR distribution models with a distribution parameter range from 0 to 3, as shown in . The lowest RMSE to achieve best fitting results of each model can be identified from the . The RMSE of fractal model is more sensitive to the distribution parameter with a minimum error can be achieved with fractal dimension equals to 2.45, while distribution parameter for RR model is 1.17 for the minimum error. Besides, the minimum error of RR model is slightly lower than fractal model, which means RR model can get a better fitting curve of particle size distribution than fractal model. The determined distribution parameter for RR model is very close to the reference value 1.32 from literature, while the fractal dimension of fractal model is much higher than the reference value 0.91 from literature.
The particle size distribution curve for each model is plotted in based on the manual and digital sieving data points. Most of the coal particles crushed by impact load are smaller 10 mm size as the mass of particles below 10 mm is over 60% of the total weight. It can be seen from that both fractal and RR model can well describe the particle size distribution while the curve of RR model demonstrate a better fitting result than that of fractal model.
As the distribution parameter has been determined based on particle size distribution, the other parameters for the calculation of crushing energy are W, , a, KR, KK and KB. The average weight of sample is 338 grams. The density of coal samples is 1.37 g/cm2. Considering all particles as sphere shape (Cai and Xiong Citation2005), the shape factor a is 1.5. The value of crushing energy constant, for each calculation model e.g., KR, KK and KB, for coal and rock has been determined by previous researcher, which is shown in .
Table 2. Value of KR, KK and KB in literatures.
Hence, according to the formula listed in , the fragmentation energy determined by each formula is calculated as listed in . It can be seen from the determined value that the selected energy-size relationship and particle size distribution model can significantly affect the calculated value of fragmentation energy. For formula 1 and 4 based on Rittinger theory, the fragmentation energy values are in similar range for fractal and RR distribution models. For Kick and Bond theory, the fragmentation energy tends to be underestimated with fractal model while it tends to be overestimated with RR model.
Table 3. Fragmentation energy determined by different formulas.
The tested results can be compared with the previous research as well. As shown in , the coal samples have been tested by Split Hopkinson Bar (SHPB) system (Z. Y. Liu et al. Citation2022) with different impact velocity and energy. Most of these tests were using the small disc sample to determine the tensile strength, fracture toughness or elastic modulus of coal samples subject to dynamic loads. Due to the different test nature such as sample size, impact velocity and test aims, some of the tests is not comparable to this research. The dissipated energy is also in a wide range in different literatures as illustrated in . The tests done by Gong et al. (Citation2021). have determined the dissipated energy rate per unit volume of coal samples with different angles between loading direction and bedding planes. The unit dissipated energy rate based on the tests results is plotted in , which suggested that the dissipated energy rate for coal is averagely around 511 J/m3. The fragmentation energy rate was also calculated based on the tests results of this study, as listed in . Although the angle between impact loading direction and bedding plane can change the determined dissipated energy rate (Li et al. Citation2022), it can be seen from the and that formula 1 and 4 are within the reasonable range of fragmentation calculation. It can be concluded that Rittinger Theory is more suitable for the fragmentation energy calculation for coal crushed by impact loads.
Conclusions
Although the relationship between the fragmentation energy and the particle size for single sized particles has been extensively researched over the last century. The fragmentation energy calculation model for coal fragmentations with coarse and wide size distribution has not been well established. In this research, the differential form of energy-size formula is proposed based on the analysis of size distribution and energy-size relationships of coal particles. The drop weight tests were conducted to validate the effectiveness of each formula. The following conclusions can be summarized based on the research:
The statistical mass-size curves of coal particles crushed by impact load were obtained based on manual sieving and digital image processing technique, which is used to validate the fractal and Rosin-Ramler (RR) model distribution curve of coal particles. It can be seen from that both fractal and RR model can well describe the particle size distribution while the curve of RR model demonstrate a better fitting result than that of fractal model. The fitting error of fractal model is very sensitive to fractal dimension while that of RR model is much less sensitive to distribution parameters. Besides, the optimal value of distribution parameters of RR model is very close to the reference value in literature.
The selected energy-size relationship and particle size distribution model can significantly affect the calculated value of fragmentation energy. The fragmentation energy rate per unit volume of coal is within a reasonable range compared with the impact tests of coal done by Split Hopkinson Bar (SHPB) system. For formula 1 and 4 based on Rittinger theory, the fragmentation energy values are in similar range for fractal and RR distribution models. For Kick and Bond theory, the fragmentation energy tends to be underestimated with fractal model while it tends to be overestimated with RR model. It can further support the communication assumption that crushing work is positively related to the area of generated new fracture surface.
It is worth to do more research of the impact crushing tests of coal samples with different sizes depends on the size requirement of coal crushing. Besides, it has been noted by SHPB tests that the impact velocity will affect fragmentation energy per unit volume as well, which can be further investigated.
Highlights
Particle size distribution curve of impact crushed coal determined by manual and digital sieving.
Fragmentation energy calculation model for coal fragmentations with coarse and wide size distribution.
Impact crushing test of coal with designed impact energy input achieved by a drop weight system.
Acknowledgements
This research is supported by financial grants from Hunan Provincial Department of Public Education (23B0442).
Disclosure statement
No potential conflict of interest was reported by the author(s).
Additional information
Funding
References
- Bu, X. N., Y. Chen, G. Ma, Y. Sun, C. Ni, and G. Xie. 2020. “Wet and Dry Grinding of Coal in a Laboratory-Scale Ball Mill: Particle-Size Distributions.” Powder Technology 359:305–313. https://doi.org/10.1016/j.powtec.2019.09.062.
- Bu, X. N., G. Ma, Y. Peng, G. Xie, H. Zhan, and B. Liu. 2019. “Grinding Kinetics of Coal in Wet Ball-Milling Using the Taguchi Method.” International Journal of Coal Preparation and Utilization 42 (3): 369–388. https://doi.org/10.1080/19392699.2019.1603147.
- Cai, C. G., and Y. X. Xiong. 2005. “Theoretical and Experimental Study on Crushing Energy of Outburst-Proneness Coal.” Journal of the China Coal Society 30 (1): 63–66.
- Chen, Q., R. Li, W. Z. Xiu, G. Zheng, V. Zivkovic, and H. Yang. 2020. “Dynamics of Irregular Particles in the Passive Layer Under the Slumping Regime.” Powder Technology 372:32–39. https://doi.org/10.1016/j.powtec.2020.05.089.
- Cheng, J. W., Q. Fu, Y. Wang, Z. Ma, D. Ran, S. Sheng, Y. Ma, et al. 2023. “Development of Non-Coagulable Grout Long-Term Sealing Material for Gas Drainage.” Inorganic Chemistry Communications 154:110882. https://doi.org/10.1016/j.inoche.2023.110882.
- Cheng, Z. F., and M. Y. Zhu. 2015. “Analyzing the Effect of Wall Roughness on Gas–Particle Flow in Confined Channels Based on a Virtual-Wall-Group Concept.” International Journal of Multiphase Flow 77:158–170. https://doi.org/10.1016/j.ijmultiphaseflow.2015.08.013.
- Deniz, V. 2013. “Comparisons of Dry Grinding Kinetics of Lignite, Bituminous Coal, and Petroleum Coke. Energy Sources, Part A: Recovery.” Utilization, and Environmental Effects 35 (10): 913–920. https://doi.org/10.1080/15567036.2010.514591.
- Du, F., J. Ma, X. Guo, T. Wang, X. Dong, J. Li, S. He, et al. 2022. “Rockburst Mechanism and the Law of Energy Accumulation and Release in Mining Roadway: A Case Study.” International Journal of Coal Science & Technology 9 (1): 67. https://doi.org/10.1007/s40789-022-00521-0.
- Feng, W. H., F. Liu, F. Yang, L. Li, and L. Jing. 2018. “Experimental Study on Dynamic Split Tensile Properties of Rubber Concrete.” Construction and Building Materials 165:675–687. https://doi.org/10.1016/j.conbuildmat.2018.01.073.
- Gong, S., L. Zhou, Z. Wang, and W. Wang. 2021. “Effect of Bedding Structure on the Energy Dissipation Characteristics of Dynamic Tensile Fracture for Water-Saturated Coal.” Geofluids 2021:1–10. https://doi.org/10.1155/2021/5592672.
- Hou, T. X., Q. Xu, X.-G. Yang, P.-Y. Lu, and J.-W. Zhou. 2015. “Experimental Study of the Fragmentation Characteristics of Brittle Rocks by the Effect of a Freefall Round Hammer.” International Journal of Fracture 194 (2): 169–185. https://doi.org/10.1007/s10704-015-0046-x.
- Kossovich, E., et al. 2023. “Effects of Coals Microscale Structural Features on Their Mechanical Properties, Propensity to Crushing and Fine Dust Formation.” International Journal of Coal Science & Technology 10 (1): 20. https://doi.org/10.1007/s40789-023-00578-5.
- Li, Y. J., L. Song, Y. Tang, J. Zuo, and D. Xue. 2022. “Evaluating the Mechanical Properties of Anisotropic Shale Containing Bedding and Natural Fractures with Discrete Element Modeling.” International Journal of Coal Science & Technology 9 (1): 18. https://doi.org/10.1007/s40789-022-00473-5.
- Liu, J. X., H. Zhang, J. Liu, and X. Jiang. 2016. “Comminution Theory of Superfine Pulverized Coal Based on Fractal Analysis of Aggregate Structures.” Energy & Fuels 30 (12): 10244–10252. https://doi.org/10.1021/acs.energyfuels.6b02097.
- Liu, X. H., S. Y. Liu, and P. Tang. 2015. “Coal Fragment Size Model in Cutting Process.” Powder Technology 272:282–289. https://doi.org/10.1016/j.powtec.2014.12.015.
- Liu, Z. Y., G. Wang, J. Li, H. Li, H. Zhao, H. Shi, J. Lan, et al. 2022. “Water-Immersion Softening Mechanism of Coal Rock Mass Based on Split Hopkinson Pressure Bar Experiment.” International Journal of Coal Science & Technology 9 (1): 61. https://doi.org/10.1007/s40789-022-00532-x.
- Locat, P., et al. Assessment of the Fragmentation Energy in Rock Avalanches. in 3rd Canadian conference on Geotechnique and Geohazards. 2003. Citeseer.
- MacıMacıAs-Garcıa, A., E. M. Cuerda-Correa, and M. A. Dıaz-Dıez. 2004. “Application of the Rosin–Rammler and Gates–Gaudin–Schuhmann Models to the Particle Size Distribution Analysis of Agglomerated Cork.” Materials Characterization 52 (2): 159–164. https://doi.org/10.1016/j.matchar.2004.04.007.
- Morrell, S. 2008. “A Method for Predicting the Specific Energy Requirement of Comminution Circuits and Assessing Their Energy Utilisation Efficiency.” Minerals Engineering 21 (3): 224–233. https://doi.org/10.1016/j.mineng.2007.10.001.
- Numbi, B. P., and X. Xia. 2015. “Systems Optimization Model for Energy Management of a Parallel HPGR Crushing Process.” Applied energy 149:133–147. https://doi.org/10.1016/j.apenergy.2015.03.129.
- Numbi, B. P., and X. Xia. 2016. “Optimal Energy Control of a Crushing Process Based on Vertical Shaft Impactor.” Applied energy 162:1653–1661. https://doi.org/10.1016/j.apenergy.2014.12.017.
- Peng, R. D., Y. Ju, J. G. Wang, H. Xie, F. Gao, and L. Mao. 2015. “Energy Dissipation and Release During Coal Failure Under Conventional Triaxial Compression.” Rock Mechanics & Rock Engineering 48 (2): 509–526. https://doi.org/10.1007/s00603-014-0602-0.
- Petrakis, E., E. Stamboliadis, and K. Komnitsas. 2017. “Evaluation of the Relationship Between Energy Input and Particle Size Distribution in Comminution with the Use of Piecewise Regression Analysis.” Particulate Science and Technology 35 (4): 479–489. https://doi.org/10.1080/02726351.2016.1168894.
- Qin, Y. H., Y. Han, P. Gao, Y. Li, and S. Yuan. 2022. “Characterization of Chalcopyrite Ore Under High Voltage Pulse Discharge: Particle Size Distribution, Fractal Dimension, Specific Energy Consumption, Grinding Kinetics.” Minerals Engineering 184:107631. https://doi.org/10.1016/j.mineng.2022.107631.
- Rosin, P., and E. Rammler. 1933. “Laws Governing the Fineness of Powdered Coal.” Journal of the Institute of Fuel 7.
- Sahoo, B. K., S. De, and B. C. Meikap. 2011. “Improvement of Grinding Characteristics of Indian Coal by Microwave Pre-Treatment.” Fuel Processing Technology 92 (10): 1920–1928. https://doi.org/10.1016/j.fuproc.2011.05.012.
- Tavares, L. M., and R. P. King. 1998. “Single-Particle Fracture Under Impact Loading.” International Journal of Mineral Processing 54 (1): 1–28. https://doi.org/10.1016/S0301-7516(98)00005-2.
- Trechera, P., X. Querol, R. Lah, D. Johnson, A. Wrana, B. Williamson, T. Moreno, et al. 2022. “Chemistry and Particle Size Distribution of Respirable Coal Dust in Underground Mines in Central Eastern Europe.” International Journal of Coal Science & Technology 9 (1): 3. https://doi.org/10.1007/s40789-022-00468-2.
- Ullah, B., Y. Cheng, L. Wang, W. Yang, I. M. Jiskani, and B. Hu. 2022. “Experimental Analysis of Pore Structure and Fractal Characteristics of Soft and Hard Coals with Same Coalification.” International Journal of Coal Science & Technology 9 (1): 58. https://doi.org/10.1007/s40789-022-00530-z.
- Wu, L. C., and C. Yu. 2012. “Powder Particle Size Measurement with Digital Image Processing Using Matlab.” Advanced Materials Research 443-444:589–593. Translated by Tech Publ. https://doi.org/10.4028/www.scientific.net/AMR.443-444.589.
- Yang, X. H., T. Ren, L. Tan, and A. Remennikov. 2021. “Fragmentation Characteristic and Energy Dissipation of Coal Under Impact Load.” International Journal of Geomechanics 21 (5): 04021057. https://doi.org/10.1061/(ASCE)GM.1943-5622.0002007.
- Yang, X. H., T. Ren, and L. H. Tan. 2020a. “Estimation of Average Ejection Velocity Generated by Rib Burst Under Compression Load.” International Journal of Rock Mechanics and Mining Sciences 128:104277. https://doi.org/10.1016/j.ijrmms.2020.104277.
- Yang, X. H., T. Ren, and L. H. Tan. 2020b. “Size Distribution Measurement of Coal Fragments Using Digital Imaging Processing.” Measurement 160:107867. https://doi.org/10.1016/j.measurement.2020.107867.
- Zambrano, A. R. 2002. “General Work Input Function for Sizing Grinding Ball Mills.” Mining, Metallurgy & Exploration 19 (4): 178–186. https://doi.org/10.1007/BF03403266.
- Zhou, J. W., Y. Liu, C.-L. Du, and S.-Y. Liu. 2017. “Effect of the Particle Shape and Swirling Intensity on the Breakage of Lump Coal Particle in Pneumatic Conveying.” Powder Technology 317:438–448. https://doi.org/10.1016/j.powtec.2017.05.034.
- Zhou, J. W., Y. Liu, S.-Y. Liu, C.-L. Du, and J.-P. Li. 2017. “Effects of Particle Shape and Swirling Intensity on Elbow Erosion in Dilute-Phase Pneumatic Conveying.” Wear 380:66–77. https://doi.org/10.1016/j.wear.2017.03.009.
- Zhu, J. T., M. Chen, L. Wang, H. Sun, C. Wang, N. Azhar, N. B. Oduro, et al. 2023. “Characterization of 10 Nm–10 μm Coal Dust Particles Generated by Simulated Different Cutting and Drilling Parameters: Mass Concentration Distribution, Number Concentration Distribution, and Fractal Dimension.” International Journal of Coal Science & Technology 10 (1): 89. https://doi.org/10.1007/s40789-023-00632-2.
Appendix A
“Code for the digital image processing
clc
clear
rgb = imread(‘file name’);
I = rgb2gray(rgb);
gmag = imgradient(I);
L = watershed(gmag);
Lrgb = label2rgb(L);
se = strel(‘disk’,20);
Io = imopen(I,se);
Ie = imerode(I,se);
Iobr = imreconstruct(Ie,I);
Ioc = imclose(Io,se);
Iobrd = imdilate(Iobr,se);
Iobrcbr = imreconstruct(imcomplement(Iobrd),imcomplement(Iobr));
Iobrcbr = imcomplement(Iobrcbr);
fgm = imregionalmax(Iobrcbr);
I2 = labeloverlay(I,fgm);
se2 = strel(ones(5,5));
fgm2 = imclose(fgm,se2);
fgm3 = imerode(fgm2,se2);
fgm4 = bwareaopen(fgm3,20);
I3 = labeloverlay(I,fgm4);
bw = imbinarize(Iobrcbr);
A = 1-bw
Conn = 8;
[s1,s2]=size(A);
A=~bwmorph(A,‘majority’,10);
D=-bwdist(A,‘cityblock’);
Pr=zeros(s1,s2);
for I = 1:s1
for J = 1:s2
if A(I,J)= = 0 && D(I,J)~ = 0
Pr(I,J) = 1;
end
end
end
Pr=bwareaopen(Pr,9,Conn);
[Pr_L,Pr_n]=bwlabel(Pr,Conn);
s = regionprops(Pr_L, ‘Orientation’, ‘MajorAxisLength’, …
‘MinorAxisLength’, ‘Eccentricity’, ‘Centroid’, ‘Area’);
RGB=label2rgb(Pr_L,‘jet’, ‘w’, ‘shuffle’);
writetable(struct2table(s),‘test.xlsx’)