ABSTRACT
Embryo implantation is one of the most inefficient steps in assisted reproduction, so the identifying drugs with a potential clinical application to improve it has a strong interest. This work applies artificial intelligence and systems biology-based mathematical modeling strategies to unveil potential treatments by computationally analyzing and integrating available molecular and clinical data from patients. The mathematical models of embryo implantation computationally generated here simulate the molecular networks underneath this biological process. Once generated, these models were analyzed in order to identify potential repositioned drugs (drugs already used for other indications) able to improve embryo implantation by modulating the molecular pathways involved. Interestingly, the repositioning analysis has identified drugs considering two endpoints: (1) drugs able to modulate the activity of proteins whose role in embryo implantation is already bibliographically acknowledged, and (2) drugs that modulate key proteins in embryo implantation previously predicted through a mechanistic analysis of the mathematical models. This second approach increases the scope open for examination and potential novelty of the repositioning strategy. As a result, a list of 23 drug candidates to improve embryo implantation after IVF was identified by the mathematical models. This list includes many of the compounds already tested for this purpose, which reinforces the predictive capacity of our approach, together with novel repositioned candidates (e.g., Infliximab, Polaprezinc, and Amrinone). In conclusion, the present study exploits existing molecular and clinical information to offer new hypotheses regarding molecular mechanisms in embryo implantation and therapeutic candidates to improve it. This information will be very useful to guide future research.
Abbreviations: IVF: in vitro fertilization; EI: Embryo implantation; TPMS: Therapeutic Performance Mapping System; MM: mathematical models; ANN: Artificial Neuronal Networks; TNFα: tumour necrosis factor factor-alpha; HSPs: heat shock proteins; VEGF: vascular endothelial growth factor; PPARA: peroxisome proliferator activated receptor-α PXR: pregnane X receptor; TTR: transthyretin; BED: Biological Effectors Database; MLP: multilayer perceptron
Introduction
Embryo implantation (EI) is one of the most inefficient steps in assisted reproduction (Matorras et al. Citation2002). Even with euploid embryos, pregnancy rates are usually under 70% (Goldman et al. Citation2018; Kushnir et al. Citation2018; Simon et al. Citation2018). A number of approaches have been proposed to improve embryo quality and/or to better select the embryos (Meseguer et al. Citation2011; Lee et al. Citation2015; Sfontouris et al. Citation2016). Concerning the endometrial side of implantation, in recent years, some strategies have been proposed to identify the optimal moment to perform the transfer both in frozen-thawed cycles (Haouzi et al. Citation2009; Diaz-Gimeno et al. Citation2011) as in fresh embryo cycles (Azkargorta et al. Citation2018; Matorras et al. Citation2018, Citation2019). The standard tool for endometrial evaluation is vaginal ultrasound. However, when the endometrium is ultrasonographically normal, the standard therapy is progesterone in fresh cycles (Van Der Linden et al. Citation2015) and progesterone with or without estrogens in frozen/thawed cycles (Zarei et al. Citation2017). Different complementary treatments have been proposed in specific conditions such as enoxaparin/heparin, intralipid, corticosteroids, melatonin, coenzyme Q10, filgrastim, androgens, growth hormone, antibiotics, hCG infusion, aspirin, dopamine agonists, sildenafil, microbiota modifications, vitamin D, whose benefits are controversial (Shirlow et al. Citation2017; Bashiri et al. Citation2018). EI is a very complex mechanism with a delicate cross-talk between the embryo and the endometrium (Haouzi et al. Citation2011). More than 230 genes have been reported as differentially expressed in the endometrial tissue of receptive endometrium (Diaz-Gimeno et al. Citation2011; Li et al. Citation2011) and, concerning the endometrial fluid, more than 2200 proteins (Azkargorta et al. Citation2020), and more than 270 lipids (Matorras et al. Citation2019) have been reported. Moreover, a different proteomic (Azkargorta et al. Citation2018; Matorras et al. Citation2018) and lipidic pattern (Matorras et al. Citation2019) has been reported in implantation cycles, that is in cycles where implantation occurred (Matorras et al. Citation2018). Thus, one could speculate that some of the components of the endometrial fluid or some endometrial function could be modified by some medical therapy and perhaps increase pregnancy rates.
Drug repositioning is based on identifying and developing new uses for existing drugs. In the past, drug repositioning has been an unpredictable process when a physician noticed the beneficial side effect for a different indication. Considering the lower investment and faster drug approval due to previous knowledge on the drug, many knowledge-driven approaches have been developed to systematically predict drug repositioning based on available compound, disease, and human physiology information (Loging et al. Citation2011). Advances in data digitalization and storage in accessible databases, and the development of artificial intelligence techniques, have reinforced these strategies. Among them, those focused on a systems biology perspective are especially interesting. They try to understand and mathematically model the complex relationship between the different cellular components to predict how existing drugs could modulate them. In other words, through computationally simulating the molecular pathways involved in the biological processes studied, these systems predict the clinical effect of existing drugs on them. The advantage of these repurposing strategies is that they can integrate data within and across diverse data types, which may improve the predictive performance and provide a fuller picture of a drug’s pharmacological action. However, it is important to take into consideration that their performance is limited by the amount of available information regarding the biological event that aims to be computationally simulated and that false positives may be predicted due to mathematical artifacts (Hodos et al. Citation2016). Therefore, critical review of the resulting model predictions is always required. In this work, we have applied one of these technologies (TPMS; therapeutic performance mapping system) to identify potential therapies to improve IVF implantation. The mathematical models (MM) of EI we generated result from integrating molecular information about human physiology and their clinical responses. To increase the MM and their human counterpart’s molecular behavior similarity, the models integrated endometrial fluid-proteomics data from women collected immediately before embryo transfer and linked to a known pregnancy outcome (Azkargorta et al. Citation2018; Matorras et al. Citation2018). An extensive bibliographic search has been performed around the model’s solutions to increase candidate selection success. Candidates can then be filtered based on mechanistic plausibility.
Results
Mathematical modeling of EI
EI is a complex biological process fulfilling several physiological motives (). The role of several proteins has already been experimentally associated with each of these motives. These proteins (from now on referred to as effector proteins) have been collected through an extensive bibliographic search and are listed in . To computationally screen the potential effect of existing drugs on EI, MM simulating this biological process was generated by applying the TPMS technology (Jorba et al. Citation2020). This methodology has been successfully applied for drug repositioning in different diseases (Herrando-Grabulosa et al. Citation2016; Romeo-Guitart et al. Citation2018; Moncunill et al. Citation2020; Villalba et al. Citation2020). It combines interactomics and protein networks with information about their known response to drugs in humans using artificial intelligence and deep learning approaches to build MM of the molecular mechanisms associated with the biological processes. Interestingly, these MM can also integrate the molecular information regarding the proteomic pattern reported in implantation cycles. Once the models were generated, they were analyzed to identify key proteins involved in EI (). The different steps carried out are briefly represented in (and described in detail in the methodology section).
Table 1. EI molecular characterization
Table 2. List of the key proteins in successful EI identified by the mathematical models
Figure 1. Visual representation of the physiological motives involved in EI. Proteins reported to be associated with each of the motives (according to scientific bibliography) are indicated
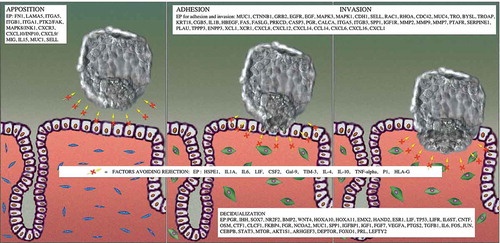
Figure 2. Steps followed to generate mathematical models of EI. First, proteins with a known role in EI (namely protein effectors) were determined by literature search. An exhaustive protein network was grown around these proteins incorporating information from several scientific databases. The protein network was then trained with functional information in humans and two types of mathematical models were generated, one mimicking successful EI and another simulating unsuccessful EI after IVF (this one trained with endometrial fluid-proteomics data from women collected immediately before embryo transfer in IVF cycles, linked to a known pregnancy outcome (pregnant or non‐pregnant). The analyses of these models through two different mathematical approaches (triggering analysis and model reversion analysis) allowed the identification of key proteins in successful EI
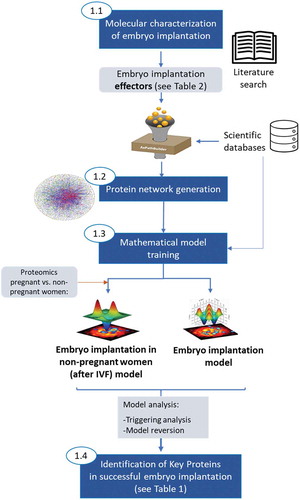
Drug repositioning screening
The generated mathematical models were able to predict and score novel potential drugs. The effect on EI of drugs in the Drugbank database (Wishart et al. Citation2008) was screened. Considering that the desired treatment is aimed at healthy individuals and that embryos are highly susceptible to external stimuli, the list of available drugs was preliminarily subjected to strict filtering to remove chemical entities that clearly may be of harm. To this end, inappropriate drug categories and dosage forms were automatically excluded from the analysis (see Supplemental Table S1). After this prefilter, a list of 6337 drugs was selected for the repositioning study ().
The Artificial Neuronal Networks (ANN) (Iborra-Egea et al. Citation2017; Jorba et al. Citation2020) strategy was employed to identify and rank the drug candidates with a potential for preventing implantation failure. In brief, this strategy assigns predictive values to individual compounds based on the likelihood that they effectively target proteins related to the condition of interest. In this case, two different outputs were analyzed: (i) drugs that modulate proteins effectors in EI (i.e., protein(s) with a known role in this process according to the bibliography, see ), and (ii) drugs that modulate the key proteins predicted as a successful IVF outcome, that were trained with proteomic data by the mathematical models (). From these two complementary strategies, we expect to resolve drug candidates based on (i) validated scientific knowledge and/or (ii) based on omics data, focusing on IVF success.
Drug candidates with a probability value p-value < 0.1 (which corresponds to a predictive value > 70%) to modulate either EI effectors or the key proteins identified were pre-selected for subsequent manual curation ().
Filtering of the list of candidate drugs
To ensure the usefulness of the candidate drugs, some biologically, medically, and market-relevant parameters were also taken into account. These encompassed:
Market access: Only candidates annotated as ‘approved’ in Drugbank (Wishart et al. Citation2008) were selected. Nutraceutical compounds were also considered. Safety: The information about safety issues was collected from SIDER database (Kuhn et al. Citation2016). Candidates causing severe adverse drug reactions in > 1% of patients or linked to reproductive failure were discarded (see Supplemental Table S1). Administration feasibility: substances whose administration is hardly feasible were discarded, such as foreskin keratinocytes and fibroblasts. FDA Pregnancy Category (Boothby and Doering Citation2001): Drugs labeled as X (Boothby and Doering Citation2001) (meaning that adequate studies in animals or pregnant women have demonstrated evidence of fetal abnormalities or risks) were also discarded. Using this filtering protocol, the list of 1202 compounds with a predictive value > 70% for either EI effectors or the identified key proteins reduced to 23 drugs ().
Next, the plausibility of a pro-implantation mechanism of action for these 23 drugs was determined by a literature-driven analysis, paying particular attention to drugs that directly modulate the identified key proteins. According to this analysis, 13 potential candidates may have a clear mechanistic relationship with EI (while the relationship was less justified by current literature for 10 of them ().
Table 3. List of repositioned candidates
also shows the FDA Pregnancy Category (letter risk category) (Boothby and Doering Citation2001) assigned to each candidate, using drug FDA labels as the source., Only drugs with categories A, B, and C appeared in the list. Some other candidates were not categorized according to the FDA criteria because they are considered dietary supplements.
Discussion
Several complementary treatments have been suggested to increase IVF pregnancy rates. These include enoxaparin/heparin, intralipid, corticosteroids, melatonin, coenzyme Q10, filgrastim, androgens, growth hormone, antibiotics, hCG infusion, aspirin, dopamine agonists, sildenafil, microbiota modifications, vitamin D, whose benefits are controversial (Shirlow et al. Citation2017; Bashiri et al. Citation2018). It is interesting that many of them appear as repositioning candidates according to our results (), reinforcing our approach’s predictive capacity.
Of note, most of the candidates show similar scores for the two repositioning endpoints studied: (i) effector proteins (i.e., proteins with a known role in EI) and (ii) key proteins predicted by the mathematical models trained with endometrial fluid-proteomics data. However, some candidates passed only the ‘key protein’ filter, among them vitamin D-related drugs, strengthening the use of these model predictions based on integrating proteomics data into the models as repositioning endpoint. Indeed, since the two different strategies exploit biological information from different sources (bibliography and/or proteomics from patients), they should be considered complementary and equally valid.
Focusing on the drugs with a plausible pro-implantation mechanism of action () showed that Infliximab was the drug with a higher predicted effect on EI effectors proteins. Infliximab is a chimeric human-murine monoclonal antibody directed against tumor necrosis factor-alpha (TNFα), approved for use in several therapeutic areas. TNFα has also been associated with inflammatory mechanisms related to implantation, placentation, and pregnancy outcome (Alijotas-Reig et al. Citation2017). A correlation between the production of TNFα and an increased risk of IVF failure has been reported (Winger et al. Citation2011). Accordingly, TNFα blockers have been used to correct excessive levels of TNFα that would lead to pregnancy loss (Poovassery et al. Citation2009). Notably, TNFα inhibition can be safely carried out during the implantation period (Alijotas-Reig et al. Citation2017) and pregnancy (Johansen et al. Citation2018).
Polaprezinc is a chelated form of zinc and L-carnosine that increases the expression of various antioxidant enzymes. It also inhibits the activity of the transcription factor nuclear factor-kappaB (NF-kB) and decreases the expression of various inflammatory cytokines, including different interleukins and TNFα (Wishart et al. Citation2006). Zinc dietary supplementation has been suggested to improve fertility (Ozkaya et al. Citation2011). This pleiotropic action involves multiple targets related to EI. Although the relationship between TNFα activity and increased risk of IVF failure has been established (Winger et al. Citation2011), the role of interleukins is less clear (Altun et al. Citation2011; Kelley and Gardner Citation2017).
Polaprezinc also stimulates the expression of heat shock proteins (HSPs), which prevents apoptotic cell-death by various stressors, including oxidative stress (Esfandiari et al. Citation2007). In mouse models, lowering of embryonic expression of HSPs induces DNA damage, leading to the degeneration of preimplantation embryos and the uterus’s inability for implantation (Jaiswal et al. Citation2013). Polaprezinc may counteract the damage induced by IVF-related stress, which generally leads to increased HSP70 expression (Grigore and Indrei Citation2001). HSP105 has also been implicated in the regulation of endometrial differentiation for EI in mouse models (Jaiswal et al. Citation2013).
Polaprezinc also leads to higher expression of numerous growth factors that participate in uterine receptivity and vascular hyperpermeability necessary for blastocyst implantation. One of the key players among those is vascular endothelial growth factor (VEGF), an angiogenic factor known to be essential for endometrial repair (Chen et al. Citation2015). The corresponding receptors are expressed at peri-implantation stages and mediate vascular hyperpermeability necessary for blastocyst implantation (Torry et al. Citation2007). It has been argued that VEGF levels are tightly regulated in the decidua, and disruption of this balance could lead to implantation failure (Torry et al. Citation2007). In addition, it has been proposed as a useful addition to embryo culture medium (Streiter et al. Citation2016). This is a very interesting candidate with various concomitant benefits on EI and high predictive value for both the condition (70.99%) and the key points of intervention (69.28%). However, the use of the drug to prevent implantation failure has not been reported.
Amrinone is a phosphodiesterase inhibitor that prevents the degradation of cAMP. Since high intracellular cAMP levels are required for the initiation of decidualization, the drug may support this biological process. Amrinone also inhibits the production of TNFα, which plays a role in implantation, as discussed above. Amrinone is an excellent candidate that may promote decidualization while averting implantation failure induced by excessive TNF.
Next, Clofibrate, Gemfibrozil, Ciporfibrate and Caprylic acid four equivalent drugs with a high predicted score for the modulation of the EI key proteins identified by the mathematical models are highlighted in . These four equivalent drugs lead to the activation of peroxisome proliferator activated receptor-α (PPARA), involved in metabolism of carbohydrates and fats (Wishart et al. Citation2006)(Cullingford et al. Citation2002). (Vanden Heuvel et al. Citation2006).
PPARs have a wide range of functions in reproduction, and PPARA in particular inhibits gene expression of enzymes involved in ovarian estrogen synthesis in mouse models (Toda et al. Citation2003). In humans, PPAR-α and PPAR-β/δ are observed in villous trophoblasts and in particular in syncytiotrophoblasts (Vitti et al. Citation2016), which participate in endometrial invasion by the blastocyst. PPARA knockout mice are associated with increased rates of abortion, although surviving pups develop normally (Vitti et al. Citation2016). Arguably, the most important member of the family in implantation is PPAR-δ, which has been proposed as a mediator of prostaglandin action in EI (Huang et al. Citation2007). Implantation of cultured embryos is enhanced by PPAR-δ activation, which led the authors to propose activation of embryo PPAR-δ is a novel therapeutic strategy to improve IVF outcome (Huang et al. Citation2007). PPAR-γ also appears to play an important role in embryonic development. A PPAR deficient subtype in mice is embryonic lethal, presumably, a result of a major defect in placental development (Youssef and Badr Citation2004).
In conclusion, Clofibrate and its equivalents Gemfibrozil, Ciprofibrate and Caprylic acid are potential candidates that mainly act via activation of PPARA. These drugs have a high predictive value for EI (63.81%) and key proteins (72.34%). The latter is consistent with the fact that it activates PPARA. Use of these drugs to prevent implantation failure has not been directly reported, with the exception of Caprylic acid.
Other drug candidates that target PPARA are Fenofibrate and its active metabolite Fenofibric acid. Fenofibrate exerts its therapeutic effects through the activation of PPARA. Additionally, Fenofibrate activates the pregnane X receptor (PXR), a nuclear receptor and transcription factor that activates multiple genes involved in xenobiotic’s metabolism (Wang et al. Citation2014). Expression of PXR in the placenta seems to be low (Geenes et al. Citation2011). It may have the ability to mediate the genomic effects of steroid hormones such as progesterone or glucocorticoids (Masuyama et al. Citation2001). Namely, progesterone may act via a PXR-mediated mechanism to regulate uterine contractility (Mitchell et al. Citation2005).
Tafamidis works by stabilizing transthyretin (TTR). Although the connection with EI is not directly apparent, TTR is abundant in the uterine cavity, uterine secretions, placenta, and serum of pregnant females in the peri-implantation uterus and the first trimester of pregnancy (Wang et al. Citation2016). Moreover, its expression increases in the preimplantation mouse uterus (Diao et al. Citation2010).
Among the drug candidates in , three vitamin D-related compounds, doxercalciferol, calcidiol, and dihydrotachysterol, were identified. The decidua produces calcitriol in response to IL-1B secreted by the blastocyst. It may help support implantation by attenuating decidual T-cell function (Barrera et al. Citation2015; Thangamani et al. Citation2015). Decidual NK cells treated with calcitriol show decreased cytokine synthesis, such as IL-6 and TNFα, which are linked to pregnancy failure (Tamblyn et al. Citation2015). Additionally, vitamin D may play an important autocrine role through its regulation of the transcription of genes such as HOXA10, critical for EI and placentation (Evans et al. Citation2004). Several studies have shown worse IVF outcomes in women with deficient vitamin D levels (Chu et al. Citation2018; Iliuta et al. Citation2020), and vitamin D supplementation is recommended in IVF deficient and insufficient women (Chu et al. Citation2018; Iliuta et al. Citation2020).
Other repositioned candidates summarized in include dydrogesterone, allylestrenol, heparin, and melatonin. Dydrogesterone showed a higher predicted efficacy in our analysis. This drug is a more selective progesterone receptor agonist than progesterone, with a lower affinity for androgen and glucocorticoid receptors (Rizner et al. Citation2011). It has been reported that luteal phase support in IVF cycles with dydrogesterone displays a favorable risk/benefit profile compared with progesterone (Tournaye et al. Citation2017; Griesinger et al. Citation2018). Allylestrenol is a synthetic steroid with progestational activity, corresponding to the 19-nortestosterone series without androgenic properties (Wu Citation1962; Bergink et al. Citation1985). In studies performed in rabbits more than 60 years ago, implantation and pregnancy were reported to be normal (Wu Citation1962). Heparin has a well-known anticoagulation effect, and it has been postulated that it could promote the continuation of pregnancy in women with recurrent miscarriage (Nelson and Greer Citation2008). It has been shown that heparin modulates endometrial receptivity and decidualization of endometrial stromal cells and improves implantation (Tamada et al. Citation1999; Fluhr et al. Citation2010). Although results are controversial, it has been suggested that heparin and heparin derivatives may be helpful in i) patients with thrombophilia ii) in patients with recurrent implantation failure or recurrent miscarriage without known abnormalities (Akhtar et al. Citation2013; Potdar et al. Citation2013). Melatonin possesses multifunctional bioactivities having an antioxidant effect (Eryilmaz et al. Citation2011). However, in two small sample-sized randomized trials, no effect of melatonin coadministration in IVF cycles was observed (Eryilmaz et al. Citation2011; Batioglu et al. Citation2012).
The available literature on EI collected and databases, with the high-performance molecular data collected from IVF patients, has allowed us to propose drugs with a possible effect on this process. Using computational approaches that exploit available scientific data, they can generate especially relevant data-driven hypotheses enhancing the chance of validation. This study provides important information about the EI process itself. (1) On the one hand, from the MM here generated, we have extracted a list of key proteins, which expands the number of proteins currently associated with EI. (2) On the other hand, the revision of the list of drug candidates allows us to observe the main points where this process can be modulated. This includes the TNFα, PPARA, HSP90, HSP70, and vitamin D pathways. This new information will undoubtedly help research in this field.
The clinical application of compounds to improve EI is very limited due to the danger of possible effects on the embryo. Validation of the proposed compounds in animal models and/or patients is technically very complex. Our theoretical model has revealed a series of drugs already used in the human species that could be useful in EI. This opens the possibility of clinical studies aimed at improving the success rate of embryo transfer. However, the dose and its duration and the subject to be treated (woman or embryo at culture) remain to be established using the doses used in other therapies as a starting point.
Materials and methods
Mathematical models that computationally simulate molecular mechanisms in embryo implantation were generated using Therapeutic Performance Mapping System approach (TPMS, Anaxomics Biotech)(Jorba et al. Citation2020), an Anaxomics proprietary technology offered by the company. This approach is based on artificial intelligence and pattern recognition techniques and creates mathematical models that integrate available biological, pharmacological and medical knowledge to simulate human physiology in silico (Badiola et al. Citation2013; Perera et al. Citation2014; Iborra-Egea et al. Citation2017). The process encompassed four steps ():
Molecular characterization of embryo implantation by manual curation of scientific literature (, Step 1)
The literature-based molecular characterization of embryo implantation was performed as described in (Azkargorta et al. Citation2018). Briefly, the main pathophysiological processes believed to be involved in embryo implantation were defined: (a) Embryo apposition, (b) Decidualization, (c) Embryo adhesion to and invasion into the endometrial epithelium and (d) Factors to avoid embryo rejection. These processes are referred to as motives. Subsequently, each pathophysiological process/motive was further characterized at protein level (). A total of 107 unique proteins were defined as effector proteins of embryo implantation, and were used to focus subsequent analyses in the human biological network.
Preparation of a human biological network focused in the area around embryo implantation (, Step 2)
A protein-protein network was generated around the effector proteins identified in the previous step, by incorporating in recurrent network growing steps all the available relationships between proteins from a regularly updated in-house database drawn from public sources: KEGG (Kanehisa and Goto Citation2000), REACTOME (Croft et al. Citation2014), INTACT(Orchard et al. Citation2014), BIOGRID (Salwinski et al. Citation2009), HDPR (Keshava Prasad et al. Citation2009), MATRIXDB (Chautard et al. Citation2011), MIPS (Mewes et al. Citation2011), DIP (Xenarios et al. Citation2000) and MINT(Licata et al. Citation2011). At the same time, the network was embedded with all sorts of biological information (drug targets, tissue expression, biomarkers, …) about nodes (i.e., proteins) and edges (i.e., connections) from public sources.
Mathematical model generation (, Step 3)
The biological map was transformed into a mathematical model capable of both reproducing existing knowledge and predicting new data. For this purpose, Artificial Neural Network (ANNs) strategies were used. ANNs are supervised algorithms which identify relations between drug targets and clinical elements of the network that are used for training a classifier with the information contained in DrugBank database (information about drugs and clinical indications) (Wishart et al. Citation2008). In other words, input-output pairs formed by the approved drugs and their associated indications retrieved from Drugbank database were used as training data. This way, the drugs included in the training were those (1) whose annotated targets are present as nodes in the topology of the model (i.e., present in the protein-protein network) (input data), and (2) whose associated indication is molecularly characterized in the Anaxomics proprietary Biological Effectors Database (BED) (output data). Drugs previously associated with embryo implantation were included in the training, if they fulfil these two premises (e.g., progesterone).
The learning methodology was applied on a model that consists on an architecture of stratified ensembles of neural networks, and was trained with a gradient descent algorithm to approximates the values of the given training information. The neural network model used was a multilayer perceptron (MLP) neural network classifier. MLP gradient descent training depends on randomization initialization, and to avoid random errors, 1000 MLPs were trained with the training subset and the best 100 MLPs were used. In order to correctly predict the effect of a drug without being biased by the number of targets, different ensembles of neural networks were trained for different subsets of drugs according to their number of targets (drugs with 1 target, 2 targets, 3 targets …). Then, the predictions for a query drug are calculated by all the ensembles, and pondered according to the number of targets of the query drug (the difference between the number of targets of the query and the number of targets of the drugs used to calculate each ensemble is used to ponder the result of each ensemble). As an internal control, the accuracy of the ANNs to reproduce the indications of Drugbank was 98% for those drugs with all targets in the human biological network. Interestingly, in addition to the information collected from Drugbank database, we also used as model training information endometrial fluid-proteomics data from women collected immediately before embryo transfer in IVF cycles, linked to a known pregnancy outcome (pregnant or non‐pregnant). These proteomics data had been previously published as two datasets (discovery and validation sets in (Azkargorta et al. Citation2018)) and were unified according to Stouffer’s method (Stouffer Citation1949; Tseng et al. Citation2012), considering a q value threshold ≤ 0.1. This strategy allowed the identification of 64 differential proteins that define the molecular state of women pregnant after IVF process (Supplemental table S2) and that were used as restrictions during the model training step.
This way, two different types of embryo implantation-models were generated, (i) a pregnant women (after IVF) model (‘implantative cycles’), that complied with a given set of restrictions, corresponding to the available biological knowledge about the constructed networks, together with knowledge derived from DrugBank database(Wishart et al. Citation2008), and (ii) a non-pregnant women (after IVF) (‘non implantative’ cycles) model that, in addition to the abovementioned restrictions, it also included the proteomics derived information (pregnant vs non-pregnant differentially expressed proteins).
Identification of key proteins in embryo implantation (, Step 4)
Once the mathematical models were generated, we analyzed them in order to identify key protein involved in embryo implantation, that could later be used as an endpoint in the drug repositioning analysis (i.e., searching for drugs with a functional impact on these key proteins). Two different strategies were performed: (i) Triggering analysis strategy and (ii) Model Reversion analysis. The first one was used to identify, among the 64 differential proteins from the proteomics data comparison (women who achieved pregnancy versus women who did not achieve pregnancy), the upstream regulators of embryo implantation. In other words, to find those differentially expressed proteins whose modulation causes as a downstream effect the activation of the highest number of effector proteins (coverage) according to the molecular definition of embryo implantation performed in step 1.1. In this case, the maximum coverage achieved, obtained with the 11 proteins selected as key proteins, was 91% of the effectors included in the previous molecular characterization of embryo implantation.
The second strategy, Model Reversion, was a model comparison analysis (women who achieved pregnancy versus women who did not achieve pregnancy) to identify proteins included in the models that could promote the shift from non ‘implantative cycles’ model to ‘implantative cycles’ model. The statistical significance of the reversion capability of each protein in the model was calculated, and 155 proteins were selected as key proteins according to an associated p-value ≤ 0.05.
According to the results obtained by the two strategies, a total of 166 key proteins were identified, 11 by applying the triggering analysis strategy and the remaining 155 key proteins by applying the model reversion strategy ().
Ethics approval
This article presents a biocomputational analysis of biomedical public data (from databases or already published high-throughput data). It does not contain any studies with human and animal subjects performed by the any of the authors, and therefore it does not require ethical approval.
Disclosure of potential conflicts of interest
No potential conflict of interest was reported by the authors.
Authors' contributions
Designed the study and performed the research: RV, JMM, TS. Analyzed data: RM, RV, MA, FE, TS. Drafted the article: RM, TS, JB. Revised the paper and approved the final version: all authors.
Supplemental Material
Download MS Word (26.4 KB)Acknowledgments
This study was partially funded by a Grant for Fertility Innovation (GFI, 2011) from Merck (Darmstadt, Germany). Proteomics Platform at CIC bioGUNE is supported by Basque Department of Industry, Tourism and Trade (Etortek and Elkartek programs), the Innovation Technology Department of the Bizkaia County, the ProteoRed-ISCIII (Grant PRB3 IPT17/0019), CIBERehd Network and Severo Ochoa Grant (SEV-2016-0644).
Supplementary material
Supplemental data for this article can be accessed here.
Additional information
Funding
References
- Akhtar MA, Sur S, Raine-Fenning N, Jayaprakasan K, Thornton JG, Quenby S. 2013. Heparin for assisted reproduction. Cochr Database Sys Rev. (8):CD009452. doi:10.1002/14651858.CD009452.pub2.
- Alijotas-Reig J, Esteve-Valverde E, Ferrer-Oliveras R, Llurba E, Gris JM. 2017. Tumor necrosis factor-alpha and pregnancy: focus on biologics. an updated and comprehensive review. Clin Rev Allergy Immunol. 53(1):40–53. doi:10.1007/s12016-016-8596-x.
- Altun T, Jindal S, Greenseid K, Shu J, Pal L. 2011. Low follicular fluid IL-6 levels in IVF patients are associated with increased likelihood of clinical pregnancy. J Assist Reprod Genet. 28(3):245–251. doi:10.1007/s10815-010-9502-8.
- Azkargorta M, Bregon-Villahoz M, Escobes I, Ibanez-Perez J, Iloro I, Iglesias M, Diez-Zapirain M, Rabanal A, Prieto B, Moragues MD, et al. 2020. In-depth proteomics and natural peptidomics analyses reveal antibacterial peptides in human endometrial fluid. J Proteomics. 216:103652. doi:10.1016/j.jprot.2020.103652.
- Azkargorta M, Escobes I, Iloro I, Osinalde N, Corral B, Ibanez-Perez J, Exposito A, Prieto B, Elortza F, Matorras R. 2018. Differential proteomic analysis of endometrial fluid suggests increased inflammation and impaired glucose metabolism in non-implantative IVF cycles and pinpoints PYGB as a putative implantation marker. Human Rep. 33(10):1898–1906. doi:10.1093/humrep/dey274.
- Badiola N, Alcalde V, Pujol A, Munter LM, Multhaup G, Lleo A, Coma M, Soler-Lopez M, Aloy P. 2013. The proton-pump inhibitor lansoprazole enhances amyloid beta production. PloS One. 8(3):e58837. doi:10.1371/journal.pone.0058837.
- Barrera D, Diaz L, Noyola-martinez N, Halhali A. 2015. Vitamin D and inflammatory cytokines in healthy and preeclamptic pregnancies. Nutrients. 7(8):6465–6490. doi:10.3390/nu7085293.
- Bashiri A, Halper KI, Orvieto R. 2018. Recurrent Implantation Failure-update overview on etiology, diagnosis, treatment and future directions. Rep Bio Endocrinol: RB&E. 16(1):121. doi:10.1186/s12958-018-0414-2.
- Batioglu AS, Sahin U, Gurlek B, Ozturk N, Unsal E. 2012. The efficacy of melatonin administration on oocyte quality. Gynecol Endocrinol: Off J Int Soc Gynecol Endocrinol. 28(2):91–93. doi:10.3109/09513590.2011.589925.
- Bergink EW, Loonen PB, Kloosterboer HJ. 1985. Receptor binding of allylestrenol, a progestagen of the 19-nortestosterone series without androgenic properties. J Steroid Biochem. 23(2):165–168. doi:10.1016/0022-4731(85)90232-8.
- Boothby LA, Doering PL. 2001. FDA labeling system for drugs in pregnancy. Ann Pharmacother. 35(11):1485–1489. doi:10.1345/aph.1A034.
- Chautard E, Fatoux-Ardore M, Ballut L, Thierry-Mieg N, Ricard-Blum S. 2011. MatrixDB, the extracellular matrix interaction database. Nucleic Acids Res. 39(Database):D235–240. doi:10.1093/nar/gkq830.
- Chen X, Liu J, He B, Li Y, Liu S, Wu B, Wang S, Zhang S, Xu X, Wang J. 2015. Vascular endothelial growth factor (VEGF) regulation by hypoxia inducible factor-1 alpha (HIF1A) starts and peaks during endometrial breakdown, not repair, in a mouse menstrual-like model. Human Rep. 30(9):2160–2170. doi:10.1093/humrep/dev156.
- Chu J, Gallos I, Tobias A, Tan B, Eapen A, Coomarasamy A. 2018. Vitamin D and assisted reproductive treatment outcome: a systematic review and meta-analysis. Human Rep. 33(1):65–80. doi:10.1093/humrep/dex326.
- Croft D, Mundo AF, Haw R, Milacic M, Weiser J, Wu G, Caudy M, Garapati P, Gillespie M, Kamdar MR, et al. 2014. The Reactome pathway knowledgebase. Nucleic Acids Res. 42(D1):D472–477. doi:10.1093/nar/gkt1102
- Cullingford TE, Dolphin CT, Sato H. 2002. The peroxisome proliferator-activated receptor alpha-selective activator ciprofibrate upregulates expression of genes encoding fatty acid oxidation and ketogenesis enzymes in rat brain. Neuropharmacology. 42(5):724–730. doi:10.1016/S0028-3908(02)00014-X.
- Diao H, Xiao S, Cui J, Chun J, Xu Y, Ye X. 2010. Progesterone receptor-mediated up-regulation of transthyretin in preimplantation mouse uterus. Fertil Steril. 93(8):2750–2753. doi:10.1016/j.fertnstert.2010.01.009.
- Diaz-Gimeno P, Horcajadas JA, Martinez-Conejero JA, Esteban FJ, Alama P, Pellicer A, Simon C. 2011. A genomic diagnostic tool for human endometrial receptivity based on the transcriptomic signature. Fertil Steril. 95(1):50–60, 60 e51-15. doi:10.1016/j.fertnstert.2010.04.063.
- Eryilmaz OG, Devran A, Sarikaya E, Aksakal FN, Mollamahmutoglu L, Cicek N. 2011. Melatonin improves the oocyte and the embryo in IVF patients with sleep disturbances, but does not improve the sleeping problems. J Assist Reprod Genet. 28(9):815–820. doi:10.1007/s10815-011-9604-y.
- Esfandiari N, Falcone T, Goldberg JM, Agarwal A, Sharma RK. 2007. Heat-shock proteins modulate the incidence of apoptosis and oxidative stress in preimplantation mouse embryos. Fertil Steril. 87(5):1214–1217. doi:10.1016/j.fertnstert.2006.07.1536.
- Evans KN, Bulmer JN, Kilby MD, Hewison M. 2004. Vitamin D and placental-decidual function. J Soc Gynecol Investig. 11(5):263–271. doi:10.1016/j.jsgi.2004.02.002.
- Fluhr H, Spratte J, Ehrhardt J, Steinmuller F, Licht P, Zygmunt M. 2010. Heparin and low-molecular-weight heparins modulate the decidualization of human endometrial stromal cells. Fertil Steril. 93(8):2581–2587. doi:10.1016/j.fertnstert.2009.10.025.
- Geenes VL, Dixon PH, Chambers J, Raguz S, Marin JJ, Bhakoo KK, Williamson C. 2011. Characterisation of the nuclear receptors FXR, PXR and CAR in normal and cholestatic placenta. Placenta. 32(7):535–537. doi:10.1016/j.placenta.2011.04.014.
- Goldman RH, Racowsky C, Farland LV, Fox JH, Munne S, Ribustello L, Ginsburg ES. 2018. The cost of a euploid embryo identified from preimplantation genetic testing for aneuploidy (PGT-A): a counseling tool. J Assist Reprod Genet. 35(9):1641–1650. doi:10.1007/s10815-018-1275-5.
- Griesinger G, Blockeel C, Sukhikh GT, Patki A, Dhorepatil B, Yang DZ, Chen ZJ, Kahler E, Pexman-Fieth C, Tournaye H. 2018. Oral dydrogesterone versus intravaginal micronized progesterone gel for luteal phase support in IVF: a randomized clinical trial. Human Rep. 33(12):2212–2221. doi:10.1093/humrep/dey306.
- Grigore M, Indrei A. 2001. The role of heat shock proteins in reproduction. Rev Med Chir Soc Med Nat Iasi. 105(4):674–676.
- Haouzi D, Dechaud H, Assou S, Monzo C, De Vos J, Hamamah S. 2011. Transcriptome analysis reveals dialogues between human trophectoderm and endometrial cells during the implantation period. Human Rep. 26(6):1440–1449. doi:10.1093/humrep/der075.
- Haouzi D, Mahmoud K, Fourar M, Bendhaou K, Dechaud H, De Vos J, Reme T, Dewailly D, Hamamah S. 2009. Identification of new biomarkers of human endometrial receptivity in the natural cycle. Human Rep. 24(1):198–205. doi:10.1093/humrep/den360.
- Herrando-Grabulosa M, Mulet R, Pujol A, Mas JM, Navarro X, Aloy P, Coma M, Casas C. 2016. Novel neuroprotective multicomponent therapy for amyotrophic lateral sclerosis designed by networked systems. PloS One. 11(1):e0147626. doi:10.1371/journal.pone.0147626.
- Hodos RA, Kidd BA, Shameer K, Readhead BP, Dudley JT. 2016. In silico methods for drug repurposing and pharmacology. Wiley Interdiscip Rev Syst Biol Med. 8(3):186–210. doi:10.1002/wsbm.1337.
- Huang JC, Wun WS, Goldsby JS, Wun IC, Noorhasan D, Wu KK. 2007. Stimulation of embryo hatching and implantation by prostacyclin and peroxisome proliferator-activated receptor delta activation: implication in IVF. Human Rep. 22(3):807–814. doi:10.1093/humrep/del429.
- Iborra-Egea O, Galvez-Monton C, Roura S, Perea-Gil I, Prat-Vidal C, Soler-Botija C, Bayes-Genis A. 2017. Mechanisms of action of sacubitril/valsartan on cardiac remodeling: a systems biology approach. NPJ Sys Bio App. 3(1):12. doi:10.1038/s41540-017-0013-4.
- Iliuta FPJ, Lainz L, Exposito A, Matorras R. 2020. Women’s vitamin D levels and IVF results. Systematic review of the literature and meta-analysis, considering three categories of vitamin status (replete, insufficient and deficient). Hum Fertil (Camb). 14:1–19. doi:10.1080/14647273.2020.1807618.
- Jaiswal MK, Agrawal V, Jaiswal YK. 2013. Lipopolysaccharide drives alternation of heat shock proteins and induces failure of blastocyst implantation in mouse. Biol Reprod. 88(6):162. doi:10.1095/biolreprod.113.108068.
- Johansen CB, Jimenez-Solem E, Haerskjold A, Sand FL, Thomsen SF. 2018. The use and safety of TNF inhibitors during pregnancy in women with psoriasis: a review. Int J Mol Sci. 19(5):5. doi:10.3390/ijms19051349.
- Jorba G, Aguirre-Plans J, Junet V, Segu-Verges C, Ruiz JL, Pujol A, Fernandez-Fuentes N, Mas JM, Oliva B. 2020. In-silico simulated prototype-patients using TPMS technology to study a potential adverse effect of sacubitril and valsartan. PloS One. 15(2):e0228926. doi:10.1371/journal.pone.0228926.
- Kanehisa M, Goto S. 2000. KEGG: kyoto encyclopedia of genes and genomes. Nucleic Acids Res. 28(1):27–30. doi:10.1093/nar/28.1.27.
- Kelley RL, Gardner DK. 2017. Addition of interleukin-6 to mouse embryo culture increases blastocyst cell number and influences the inner cell mass to trophectoderm ratio. Clin Exp Reprod Med. 44(3):119–125. doi:10.5653/cerm.2017.44.3.119.
- Keshava Prasad TS, Goel R, Kandasamy K, Keerthikumar S, Kumar S, Mathivanan S, Telikicherla D, Raju R, Shafreen B, Venugopal A, et al. 2009. Human protein reference database–2009 update. Nucleic Acids Res. 37(Database):D767–772. doi:10.1093/nar/gkn892
- Kuhn M, Letunic I, Jensen LJ, Bork P. 2016. The SIDER database of drugs and side effects. Nucleic Acids Res. 44(D1):D1075–1079. doi:10.1093/nar/gkv1075.
- Kushnir VA, Darmon SK, Barad DH, Gleicher N. 2018. Degree of mosaicism in trophectoderm does not predict pregnancy potential: a corrected analysis of pregnancy outcomes following transfer of mosaic embryos. Rep Bio Endocrinol: RB&E. 16(1):6. doi:10.1186/s12958-018-0322-5.
- Lee E, Illingworth P, Wilton L, Chambers GM. 2015. The clinical effectiveness of preimplantation genetic diagnosis for aneuploidy in all 24 chromosomes (PGD-A): systematic review. Human Rep. 30(2):473–483. doi:10.1093/humrep/deu303.
- Li J, Tan Z, Li M, Xia T, Liu P, Yu W. 2011. Proteomic analysis of endometrium in fertile women during the prereceptive and receptive phases after luteinizing hormone surge. Fertil Steril. 95(3):1161–1163. doi:10.1016/j.fertnstert.2010.09.033.
- Licata L, Briganti L, Peluso D, Perfetto L, Iannuccelli M, Galeota E, Sacco F, Palma A, Nardozza AP, Santonico E, et al. 2011. MINT, the molecular interaction database: 2012 update. Nucleic Acids Res. 40(D1):D857–D861. doi:10.1093/nar/gkr930
- Loging W, Rodriguez-Esteban R, Hill J, Freeman T, Miglietta J. 2011. Cheminformatic/bioinformatic analysis of large corporate databases: application to drug repurposing. Drug Discov Today Ther Strateg. 8(3):109–116.
- Masuyama H, Hiramatsu Y, Mizutani Y, Inoshita H, Kudo T. 2001. The expression of pregnane X receptor and its target gene, cytochrome P450 3A1, in perinatal mouse. Mol Cell Endocrinol. 172(1–2):47–56. doi:10.1016/S0303-7207(00)00395-6.
- Matorras R, Martinez-Arranz I, Arretxe E, Iruarrizaga-Lejarreta M, Corral B, Ibanez-Perez J, Exposito A, Prieto B, Elortza F, Alonso C. 2019. The lipidome of endometrial fluid differs between implantative and non-implantative IVF cycles. J Assist Reprod Genet. 37(2):385–394. doi:10.1007/s10815-019-01670-z.
- Matorras R, Quevedo S, Corral B, Prieto B, Exposito A, Mendoza R, Rabanal A, Diaz-Nunez M, Ferrando M, Elortza F, et al. 2018. Proteomic pattern of implantative human endometrial fluid in in vitro fertilization cycles. Arch Gynecol Obstet. 297(6):1577–1586. doi:10.1007/s00404-018-4753-1
- Matorras R, Urquijo E, Mendoza R, Corcostegui B, Exposito A, Rodriguez-Escudero FJ. 2002. Ultrasound-guided embryo transfer improves pregnancy rates and increases the frequency of easy transfers. Human Rep. 17(7):1762–1766. doi:10.1093/humrep/17.7.1762.
- Meseguer M, Herrero J, Tejera A, Hilligsoe KM, Ramsing NB, Remohi J. 2011. The use of morphokinetics as a predictor of embryo implantation. Human Rep. 26(10):2658–2671. doi:10.1093/humrep/der256.
- Mewes HW, Ruepp A, Theis F, Rattei T, Walter M, Frishman D, Suhre K, Spannagl M, Mayer KF, Stumpflen V, et al. 2011. MIPS: curated databases and comprehensive secondary data resources in 2010. Nucleic Acids Res. 39:D220–224. doi:10.1093/nar/gkq1157
- Mitchell BF, Mitchell JM, Chowdhury J, Tougas M, Engelen SM, Senff N, Heijnen I, Moore JT, Goodwin B, Wong S, et al. 2005. Metabolites of progesterone and the pregnane X receptor: a novel pathway regulating uterine contractility in pregnancy? Am J Obstet Gynecol. 192(4):1304–1313. discussion 1313-1305. doi:10.1016/j.ajog.2005.01.040.
- Moncunill G, Scholzen A, Mpina M, Nhabomba A, Hounkpatin AB, Osaba L, Valls R, Campo JJ, Sanz H, Jairoce C, et al. 2020. Antigen-stimulated PBMC transcriptional protective signatures for malaria immunization. Sci Transl Med. 12:543. doi:10.1126/scitranslmed.aay8924.
- Nelson SM, Greer IA. 2008. The potential role of heparin in assisted conception. Human Reproduction Update. 14(6):623–645.
- Orchard S, Ammari M, Aranda B, Breuza L, Briganti L, Broackes-Carter F, Campbell NH, Chavali G, Chen C, Del-toro N, et al. 2014. The MIntAct project–IntAct as a common curation platform for 11 molecular interaction databases. Nucleic Acids Res. 42:D358–363. doi:10.1093/nar/gkt1115
- Ozkaya MO, Naziroglu M, Barak C, Berkkanoglu M. 2011. Effects of multivitamin/mineral supplementation on trace element levels in serum and follicular fluid of women undergoing in vitro fertilization (IVF). Biol Trace Elem Res. 139(1):1–9. doi:10.1007/s12011-010-8637-x.
- Perera S, Artigas L, Mulet R, Mas JM, Sardón T. 2014. Systems biology applied to non-alcoholic fatty liver disease (NAFLD): treatment selection based on the mechanism of action of nutraceuticals. Nutrafoods. 13(2):61–68. doi:10.1007/s13749-014-0022-5.
- Poovassery JS, Sarr D, Smith G, Nagy T, Moore JM. 2009. Malaria-induced murine pregnancy failure: distinct roles for IFN-gamma and TNF. J Immunol. 183(8):5342–5349. doi:10.4049/jimmunol.0901669.
- Potdar N, Gelbaya TA, Konje JC, Nardo LG. 2013. Adjunct low-molecular-weight heparin to improve live birth rate after recurrent implantation failure: a systematic review and meta-analysis. Human Reproduction Update. 19(6):674–684. doi:10.1093/humupd/dmt032.
- Rizner TL, Brozic P, Doucette C, Turek-Etienne T, Muller-Vieira U, Sonneveld E, Van Der Burg B, Bocker C, Husen B. 2011. Selectivity and potency of the retroprogesterone dydrogesterone in vitro. Steroids. 76(6):607–615. doi:10.1016/j.steroids.2011.02.043.
- Romeo-Guitart D, Fores J, Herrando-Grabulosa M, Valls R, Leiva-Rodriguez T, Galea E, Gonzalez-Perez F, Navarro X, Petegnief V, Bosch A, et al. 2018. Neuroprotective drug for nerve trauma revealed using artificial intelligence. Sci Rep. 8(1):1879. doi:10.1038/s41598-018-19767-3
- Salwinski L, Licata L, Winter A, Thorneycroft D, Khadake J, Ceol A, Aryamontri AC, Oughtred R, Livstone M, Boucher L, et al. 2009. Recurated protein interaction datasets. Nat Methods. 6(12):860–861. doi:10.1038/nmeth1209-860
- Sfontouris IA, Martins WP, Nastri CO, Viana IG, Navarro PA, Raine-Fenning N, Van Der Poel S, Rienzi L, Racowsky C. 2016. Blastocyst culture using single versus sequential media in clinical IVF: a systematic review and meta-analysis of randomized controlled trials. J Assist Reprod Genet. 33(10):1261–1272. doi:10.1007/s10815-016-0774-5.
- Shirlow R, Healey M, Volovsky M, MacLachlan V, Vollenhoven B. 2017. The effects of adjuvant therapies on embryo transfer success. Journal of Rep & Infertility. 18(4):368–378.
- Simon AL, Kiehl M, Fischer E, Proctor JG, Bush MR, Givens C, Rabinowitz M, Demko ZP. 2018. Pregnancy outcomes from more than 1,800 in vitro fertilization cycles with the use of 24-chromosome single-nucleotide polymorphism-based preimplantation genetic testing for aneuploidy. Fertil Steril. 110(1):113–121. doi:10.1016/j.fertnstert.2018.03.026.
- Stouffer SA. 1949. A study of attitudes. Sci Am. 180(5):11–15. doi:10.1038/scientificamerican0549-11.
- Streiter S, Fisch B, Sabbah B, Ao A, Abir R. 2016. The importance of neuronal growth factors in the ovary. Mol Hum Reprod. 22(1):3–17. doi:10.1093/molehr/gav057.
- Tamada H, Higashiyama C, Takano H, Kawate N, Inaba T, Sawada T. 1999. The effects of heparin-binding epidermal growth factor-like growth factor on preimplantation-embryo development and implantation in the rat. Life Sci. 64(22):1967–1973. doi:10.1016/S0024-3205(99)00128-9.
- Tamblyn JA, Hewison M, Wagner CL, Bulmer JN, Kilby MD. 2015. Immunological role of vitamin D at the maternal-fetal interface. J Endocrinol. 224(3):R107–121. doi:10.1530/JOE-14-0642.
- Thangamani S, Kim M, Son Y, Huang X, Kim H, Lee JH, Cho J, Ulrich B, Broxmeyer HE, Kim CH. 2015. Cutting edge: progesterone directly upregulates vitamin d receptor gene expression for efficient regulation of T cells by calcitriol. J Immunol. 194(3):883–886. doi:10.4049/jimmunol.1401923.
- Toda K, Okada T, Miyaura C, Saibara T. 2003. Fenofibrate, a ligand for PPARalpha, inhibits aromatase cytochrome P450 expression in the ovary of mouse. J Lipid Res. 44(2):265–270. doi:10.1194/jlr.M200327-JLR200.
- Torry DS, Leavenworth J, Chang M, Maheshwari V, Groesch K, Ball ER, Torry RJ. 2007. Angiogenesis in implantation. J Assist Reprod Genet. 24(7):303–315. doi:10.1007/s10815-007-9152-7.
- Tournaye H, Sukhikh GT, Kahler E, Griesinger G. 2017. A Phase III randomized controlled trial comparing the efficacy, safety and tolerability of oral dydrogesterone versus micronized vaginal progesterone for luteal support in in vitro fertilization. Human Rep. 32(5):1019–1027. doi:10.1093/humrep/dex023.
- Tseng GC, Ghosh D, Feingold E. 2012. Comprehensive literature review and statistical considerations for microarray meta-analysis. Nucleic Acids Res. 40(9):3785–3799. doi:10.1093/nar/gkr1265.
- Van Der Linden M, Buckingham K, Farquhar C, Kremer JA, Metwally M. 2015. Luteal phase support for assisted reproduction cycles. Cochr Database Sys Rev. (7):CD009154.
- Vanden Heuvel JP, Thompson JT, Frame SR, Gillies PJ. 2006. Differential activation of nuclear receptors by perfluorinated fatty acid analogs and natural fatty acids: a comparison of human, mouse, and rat peroxisome proliferator-activated receptor-alpha, -beta, and -gamma, liver X receptor-beta, and retinoid X receptor-alpha. Toxicol Sci. 92(2):476–489. doi:10.1093/toxsci/kfl014.
- Villalba A, Rodriguez-Fernandez S, Perna-Barrull D, Ampudia R-M, Gomez-Muñoz L, Pujol-Autonell I, Aguilera E, Coma M, Cano-Sarabia M, Vázquez F, et al. 2020. Repurposed analog of GLP-1 ameliorates hyperglycemia in type 1 diabetic mice through pancreatic cell reprogramming [original research]. Front Endocrinol (Lausanne). 11(258). English. doi:10.3389/fendo.2020.00258
- Vitti M, Di Emidio G, Di Carlo M, Carta G, Antonosante A, Artini PG, Cimini A, Tatone C, Benedetti E. 2016. Peroxisome proliferator-activated receptors in female reproduction and fertility. PPAR Res. 2016:4612306. doi:10.1155/2016/4612306.
- Wang Q, Liu C, Zhang Z. 2016. Transthyretin and normal human pregnancy: mini review. Crit Rev Eukaryot Gene Expr. 26(3):273–277. doi:10.1615/CritRevEukaryotGeneExpr.2016017323.
- Wang S, Lei T, Zhang K, Zhao W, Fang L, Lai B, Han J, Xiao L, Wang N. 2014. Xenobiotic pregnane X receptor (PXR) regulates innate immunity via activation of NLRP3 inflammasome in vascular endothelial cells. J Biol Chem. 289(43):30075–30081. doi:10.1074/jbc.M114.578781.
- Winger EE, Reed JL, Ashoush S, El-Toukhy T, Ahuja S, Taranissi M. 2011. Degree of TNF-alpha/IL-10 cytokine elevation correlates with IVF success rates in women undergoing treatment with adalimumab (humira) and IVIG. Am J Rep Immunol. 65(6):610–618. doi:10.1111/j.1600-0897.2010.00946.x.
- Wishart DS, Knox C, Guo AC, Cheng D, Shrivastava S, Tzur D, Gautam B, Hassanali M. 2008. DrugBank: a knowledgebase for drugs, drug actions and drug targets. Nucleic Acids Res. 36:D901–906. doi:10.1093/nar/gkm958.
- Wishart DS, Knox C, Guo AC, Shrivastava S, Hassanali M, Stothard P, Chang Z, Woolsey J. 2006. DrugBank: a comprehensive resource for in silico drug discovery and exploration. Nucleic Acids Res. 34:D668–672. doi:10.1093/nar/gkj067.
- Wu DH. 1962. Gestational effect of allylestrenol. Endocrinol Jpn. 65(3):187–192. doi:10.1507/endocrj1954.9.187.
- Xenarios I, Rice DW, Salwinski L, Baron MK, Marcotte EM, Eisenberg D. 2000. DIP: the database of interacting proteins. Nucleic Acids Res. 28(1):289–291. doi:10.1093/nar/28.1.289.
- Youssef J, Badr M. 2004. Role of peroxisome proliferator-activated receptors in inflammation control. J Biomed Biotechnol. 2004(3):156–166. doi:10.1155/S1110724304308065.
- Zarei A, Sohail P, Parsanezhad ME, Alborzi S, Samsami A, Azizi M. 2017. Comparison of four protocols for luteal phase support in frozen-thawed Embryo transfer cycles: a randomized clinical trial. Arch Gynecol Obstet. 295(1):239–246. doi:10.1007/s00404-016-4217-4.