Abstract
This paper introduces a model that enables a comparison between universities based on sustainability indicators related to environmental, economic, social and educational aspects. The proposed model is based on a driving force-pressure-state-exposure-effect-action (DPSEEA) framework and is called uncertainty-based DPSEEA-Sustainability index Model (uD-SiM). The uD-SiM applies the concept of causality and develops sustainability index (SI), which is an outcome of nonlinear relationships of sustainability indicators in different stages of DPSEEA. In this paper, this fuzzy-based multi-criteria decision-making model is used to evaluate the sustainability of five Canadian universities, namely the University of British Columbia, the University of Toronto, the University of Alberta, the McGill University and the Memorial University. The final ranking results are compared with the Green report card ranking for 2010 through SI. The application of various actions and strategies that can be applied to different stages of the framework to improve sustainability in higher education institutions is also discussed.
1. Introduction
The concept of sustainability has been around for many decades now. The definition of sustainability varies depending on the context in which it is used. According to the Brundtland report (WCED Citation1987), sustainability refers to reducing footprint without compromising quality of life for the present and future generations. The most common framework that is used to illustrate sustainability is triple bottom line (TBL), which is about identifying improvements in the environment, social and economic performance by adopting short- and long-term policy decisions (Lozano Citation2008). In TBL, the environment relates to the impacts of policy decisions on the environment (e.g. natural resources, flora and fauna); economy relates to the impacts on financial or economical sustainability and society relates to the impact on a community as a whole (e.g. public health and safety, social equity and culture) (Mebratu Citation1998, Savitz and Weber Citation2006). Efforts towards sustainable pathways have been gaining momentum in all disciplines and institutions. Ideas and new actions are being developed, tested and disseminated by promoting discussions to define the exact nature of the concept of sustainability and its effective implementation.
Universities all over the world are promoting sustainability on campus by reflecting it in their missions and restructuring their research programmes, curriculum and life style on campus, and enhancing their trans-disciplinary activities with other societal institutions. The efforts vary from one campus to another; however, primary objectives of higher education institutions (HEIs) (particularly universities) are to educate students, preserve and advance knowledge, and find sustainable solutions for societal problems through research. A sustainable campus programme addresses all three components of the TBL approach, i.e. (1) improving economic efficiency, (2) protecting and restoring ecological systems and (3) enhancing the well-being of all people.
Like any other mission, the implementation of sustainability on campus has its own challenges and limitations. The sustainable development for universities is a relatively new phenomenon and is very challenging because of the complex set-up of universities. According to Lozano (Citation2006a), not only is the level of awareness among university leaders and faculty members worldwide about sustainable development goals and its principles still low, but the progress of implementing sustainability into their courses, curricula, research and outreach is also evolving slowly. Therefore, the first and foremost challenge faced by universities is finding ways and means for effective and efficient incorporation of sustainability concepts into policies, curricula, research, outreach and campus operations of a university (Lozano Citation2009). The second challenge is establishing a system that makes sustainable development an integral part of the university culture and creates a multiplying effect within the institution and in the society as a whole.
There is no single way of organising and viewing the interconnected aspects of socio-economic development, environmental impacts and human health indicators effectively, which are important ingredients of sustainability measurement according to the TBL approach. This determines the first and foremost aim of this paper, that is to extend these interconnected stages through a hierarchical causal linkage framework: driving force-pressure-state-exposure-effect-action (DPSEEA). This framework helps to assess the sustainability using a measure called sustainability index (SI) by developing an uncertainty-based model (uD-SiM), which stands for uncertainty-based DPSEEA-Sustainability index Model (uD-SiM) (introduced by Waheed et al. Citation2011). As higher education systems and academic environments are fundamentally similar in all universities, therefore, a framework for a given university will require similar types of indicators for sustainability assessment and decision-making.
In recent years, the emphasis of ranking charts for universities has changed from just providing information about the quality and other characteristics of HEIs to ranking them on the basis of their environmental performance. For HEIs, many methods for auditing and ranking sustainability performance are available (Cole Citation2003). This includes sustainability tracking, assessment and rating system (STARS) (AASHE Citation2010), and an environmental ranking system proposed by Lukman et al. (Citation2010). The 11 methods analysed by Shriberg (Citation2002) for evaluating the sustainable development at student campuses can be used for strategic planning but not for comparing campuses. The most renowned sustainability ranking card for universities is the College sustainability report card or Green Report Card (Citation2010). Green report card is the first website that provides an in-depth sustainability profile for hundreds of colleges in the USA and Canada. It emerged in 2007 as an initiative of the Sustainability Endowments Institute. It identifies colleges and universities that are leading by example in their commitment to sustainability and endowment practices by considering nine criteria: administration, climate change, food and recycling, green building, student involvement and transportation, endowment transparency and shareholder engagement. Its weakness is that it does not consider efforts towards education or research in sustainability and/or water-related initiatives. Therefore, the second aim of this paper is to demonstrate that uD-SiM can be effectively used as a ranking chart for evaluating the performance of universities towards sustainability.
This paper unfolds as follows: Section 2 explains the uD-SiM model in detail and Section 3 presents an analysis on Green report card. This is followed by data verification and application of uD-SiM in Section 4. The insight into the model and its use for ranking along with improvement through actions are discussed in Section 5. The conclusions and recommendations are presented in Section 6.
2. Uncertainty-based DPSEEA sustainability index model
Waheed et al. (Citation2011) developed a unique decision-making model called uD-SiM that assesses the performance of a HEI by calculating the SI. This model is based on DPSEEA, which is a causal framework (Figure ). These indicator-reporting frameworks have been extensively used by WRI (Citation2005), OECD (Citation1999), EEA (Citation2001) and UN (Citation1996). The DPSEEA provides a deeper insight into causality by subdividing more steps or continuums as compared to other causality frameworks such as Pressure-State-Response (PSR) and Driving force-Pressure-State-Impact-Response (DPSIR) (Briggs et al.
Citation1996, Brulming Citation1997, Corvalán et al.
Citation1999, Dalal-Clayton and Bass Citation2002, WHO Citation2010). It also brings out the important distinction between state and impact. The DPSEEA framework illustrates the cause − effect relationships for various driving forces, pressures and states of sustainability, the impacts in the form of exposure and the effects of these causes in a hierarchical fashion. For example, changes in society, such as population growth or income increase, may exert different and variable pressures on the environment as driving forces, which can be positive or negative. The actions to mitigate the adverse effects can be taken at various stages of DPSEEA – driving forces (preventive action), pressures (hazard management), states (environmental improvements), exposures (protective) or effects (corrective). Figure illustrates DPSEEA for HEIs. Driving forces are the socio-economic and socio-cultural forces driving anthropogenic activities, which increase or mitigate pressures on the environment. This provides a secondary level of analysis mainly for policy-makers or decision-makers. This framework is explained in various reports by the UN Commission on Sustainable Development (CSD Citation1995). The uD-SiM can help to identify and evaluate fuzzy-based effects, single and multiple effects of a driving force or policy on sustainability index () (Figure ).
Figure 1 Driving force, pressure, state, exposure, effect and action (after Waheed et al. Citation2011).
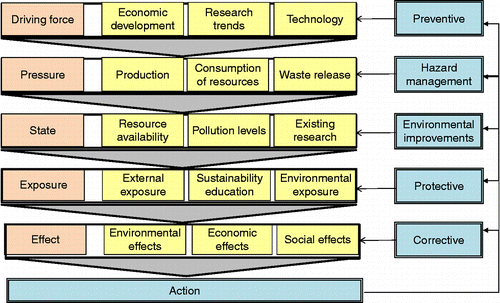
Figure 2 Proposed model uD-SiM (modified from Waheed et al. Citation2011).
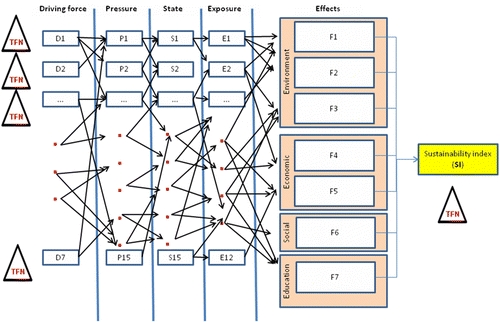
The uD-SiM is a linkage-based framework in which the final value of sustainability index () is an outcome of nonlinear effects of sustainability indicators. The information about uncertainty fuzzy-based approach is provided in Appendix A. The primary objective of this model is to develop a meaningful sustainability assessment tool for HEIs to make informed decisions. The seven procedural steps of uD-SiM are explained in the following subsections and graphically represented in Figure .
2.1 Identification of indicators
The quantitative assessment of sustainability requires various tiers of information that may include objectives, assessment criteria, indices, indicators and performance data/variables/parameters. Objectives describe the broad goals set by the decision-makers and by the public or by the user of the service. The major sustainability objectives are generally set by the TBL (i.e. environment, social and economic performance) approach. Assessment criteria, sometimes also referred to as ‘indices’ or ‘indicators’, provide principles to establish that specified objectives have been met. Assessment criteria provide yardsticks against which sustainability objectives are measured. Indicators could be leading and lagging and measurable and could be based on various methods and frameworks. The main focus should be on the outcome of performance as required by the global reporting initiative (GRI). In this paper, the indicators are selected after thorough study and are broken down under environment, economic, social and educational categories. In addition, an informal consultation with faculty members at various universities was performed.
Table provides a comprehensive list of indicators for education, environment, social and economic dimensions for driving forces, pressures, state, exposure and effect. A number of key factors that broadly affect the environmental, economic, social and educational processes for a typical HEI were selected after an extensive literature search, WHO (Citation2010), UBC (Citation2007a, Citation2007b), Rodriguez et al. (Citation2002), Lozano (Citation2006b), Cole (Citation2003), Shriberg (Citation2002), Viebahn (Citation2002), Clarke and Kouri (Citation2009), Lukman et al. (Citation2010), Goognough et al. (Citation2009), Evangelinos et al. (Citation2009), GRI (Citation2006), STAR (AASHE Citation2010) and Green Report Card (Citation2010).
Table 1 Proposed indicators for sustainability assessment of HEIs (taken from Waheed et al. Citation2011).
For this model, seven indicators were identified as driving forces: D 1– global/local and research and development trends, D 2 – institutional enhancement, D 3– annual energy consumption rate, D 4 – financial and economic growth rate, D 5– health and safety index, D 6– social equity index and D 7– education in sustainability trends. These seven indicators belong to four major categories of sustainability, i.e. environment, social, economic and education. Similarly, 15, 15, 12 and 7 indicators have been identified for pressure, state, exposure and effects, respectively.
The indicators identified in Table are connected hierarchically through causal relationships that finally lead to the quantitative assessment of sustainability. Finally, the indicators of effects are used to estimate the SI (Figure ).
2.2 Establishing causality
Causality links are established as positive and negative, which can be explained by connection between sustainability and quality or sustainability and pollution parameters, respectively. For example, a pressure indicator (P 1) production of greenhouse gases is affected by a set of driving forces {D 1 − , D 2+, D 3+, D 4+, D 7 − }, where increases in international research and development trends or advancement (D 1) and sustainability education (D 7) decrease the production of greenhouse gases. Similarly, driving forces D 2, D 3 and D 4 positively impact P 1; therefore, the increase in these indicators increases P 1, and vice versa. Using the same principles, connections are established between pressures and states, states and exposures and exposures and effects.
2.3 Assigning weights (strength) of causality
Many methods are available for determining the weights in multi-criteria decision-making (MCDM), such as direct assignment, Delphi survey, pair-wise comparison, eigenvector method and linear programming. In this paper, the direct assignment method is used to assign crisp causality weights (w i ) to input indicators based on their relative contribution to a receiving (dependent or effect) sustainability indicator in the next phase. For example, a P 1 is impacted by a set of driving force indicators {D 1, D 2, D 3, D 4 and D 7}. Therefore, causality weights are assigned to these five input indicators. The values of these weights may vary in an interval [0, 1] and are assigned in each stage of the DPSEEA framework from driving force to the final effects and finally for the environment, economics, social and education categories for the SI (Table ). These weights can be assigned by a team of decision-makers or measured. In the present study, the weights are assigned based on the relative importance of the indicators and causal link between the indicators.
Table 2 Causality weights in uD-SiM (taken from Waheed et al. Citation2011).
2.4 Activating driving force based on fuzzy input values
In uD-SiM, the input values defined for driving force indicators are triangular fuzzy numbers (TFNs) (Zadeh Citation1965, Cullen and Frey Citation1999). These input indicators can be ‘measured’ or heuristically defined values by a decision-maker. The activation level of driving forces can be based on numerous factors identified by a specific university. The seven broad driving forces are defined in this study. For example, ‘Global/local research and development trends’ is a broad term that can be a function of numerous factors that are measurable or observable, such as zero carbon policy, Leadership in Energy and Environmental Design (LEED) certified buildings and sustainability curriculum. These factors can be aggregated through some scoring methods to obtain activation levels for driving forces. Once the input values are activated, the uD-SiM estimates the intermediate indicators at various stages of the DPSEEA framework using fuzzy arithmetic operations. These fuzzy numbers will be able to propagate uncertainties throughout the structure of the uD-SiM.
2.5 Aggregation (inferencing)
After assigning weights and activating input indicators, an inference to estimate activation for any dependent indicator can be made using Equation (Equation1):
2.6 Sub-classification and SI
At the effects stage, indicators are sub-classified under environment, economic, social and education categories. The sustainability of a HEI quantitatively, that is the fuzzy sustainability index (), is calculated using the following formulation:
2.7 Defuzzification
Fuzzy defuzzification methods can be used for ranking or obtaining crisp values of fuzzy numbers. In defuzzification, the final fuzzy value is converted into a crisp value (SIo). Various techniques are used for defuzzification. Each technique extracts different levels of information from the fuzzy numbers (Tesfamariam and Sadiq Citation2006). In this paper, Yager's (Citation1980) centroid index method is used, where the centroid index is a geometric centre (SIo) of the fuzzy
, in which the geometric centre corresponds to a crisp (representative) value of SI on its universe of discourse. For a given TFN (a, b, c), Yager (Citation1980) proposed a centroid index as follows:
3. Green report card
The Green report card is currently the most comprehensive ranking method available and applied to North American universities. As the Green report card is originated by the endowment institute, it emphasises more on the impacts of endowment practices and operations of the university on sustainability (Green Report Card Citation2010). It identifies the colleges and universities that are leading by example on sustainability. It focuses on nine main criteria: administration, climate change and energy, food and recycling, green building, student involvement, transportation, endowment transparency, investment priorities and shareholders engagement. The methodology includes selection of universities, composition of four surveys (campus operations, dining services, endowment investment practices and student activities), data collection and verification (survey conducted through students and administrators), assessment and recognition. A school's overall grade is calculated from the grades received in nine equally weighted criteria. A total of 48 indicators are used to evaluate the performance within the criteria. The Overall College Sustainability Leaders award is given to universities that have made notable achievements in sustainability by earning an overall grade of ‘A-’.
The major drawback of this ranking is that the main criteria do not encompass all sustainability efforts in a university, such as teaching research and other academic aspects that are recommended as core components in assessing the sustainability of a campus by Lozano (Citation2006a, Citation2006b, Citation2009) and Lukman et al. (Citation2010). Moreover, water consumption/wastewater initiatives are not considered and some of the indicators are based on qualitative definitions and are difficult to evaluate.
Therefore, in this paper, we propose uD-SiM as a ranking chart by modifying the data obtained from the Green report card and identify the driving forces behind implementation of sustainability in universities. For the past two decades, the commitment of Canadian universities towards sustainability-related issues has been growing. Many universities and colleges are in various stages of implementing sustainability initiatives. A series of national and international declarations on sustainability in education have been developed, and many Canadian universities have committed themselves for implementing the declarations' objectives on their own campuses (Cole Citation2003). The most common of these declarations includes the Talloires Declaration (ULSF Citation1990), the Kyoto Declaration, the Halifax Declaration and the Swansea Declaration (UNESCO Citation1990, Citation1991, Citation1993). Therefore, the application of uD-SiM will be verified by application to Canadian universities only.
4. Application of uD-SiM
In uD-SiM, the driving forces inputs activate the whole model systematically. In this study, it is assumed that the cause and effect move sequentially from driving force to the effect, which means that the driving forces activate pressures, each state is activated by one or more pressures, an exposure is activated by one or more states and likewise effects are activated by one or more exposures. The uD-SiM calculates the activation for each dependent indicator based on defined weights and fuzzy-based values of activation of input indicators. It means that once the input values are activated, the uD-SiM estimates the intermediate indicators at various stages of the DPSEEA framework using fuzzy arithmetic operations (see Appendix A for details). These fuzzy numbers will be able to propagate uncertainties throughout the structure of the uD-SiM.
After aggregation of effects indicators, SI is calculated using Equation (Equation3) from the sustainability categories – environmental, economic, social and education – by assuming the weights of these categories. To consolidate various input factors (D
k
, driving forces) and their effects on SI, this paper focuses on establishing the driving forces for various universities by preparing input from known data for selected Canadian universities and the Green report card for 2010. This process is used to rank these universities on the basis of SI.
4.1 Preparation of data
After extensive literature review and from various auditing reports, ranking charts and assessment frameworks (Barth et al. Citation2007, Lozano Citation2009, AASHE 2010, Baboulet and Lenzen Citation2010, Green Report Card Citation2010, Lukman et al. Citation2010), the following major decision categories for HEIs or universities are identified:
i. | increasing the focus of research and curriculum on sustainability, | ||||
ii. | selecting environment-friendly construction and procurement, | ||||
iii. | increasing community outreach and | ||||
iv. | defining assessment measures for environmental, economic, social and educational efficiency and benefits. |
Table 3 Data preparation for driving forces.
4.1.1 D 1 – global/local research and development trends
The criteria policy declaration (X 1) and education and research (X 2) are considered for deriving input values for D 1. Policy declaration, the lead criterion, entails demonstration of commitment to sustainability of a university by the president (Vice Chancellor) and senior administrators through a sustainability policy, adoption of sustainability-related mission statements, strategic plans and local/national/international agreements such as the Talloires Declaration. It shows the commitment of the university administration towards sustainability initiatives by integrating sustainability efforts from all stakeholders into an advisory council. The education and research (X 2) factor focuses on the following key areas:
i. | Research: it is further divided into research, publications and funding for sustainability. Research includes research related to sustainability, identification and involvement of faculty, departments doing research related to sustainability, research incentives and interdisciplinary research in tenure and promotion. | ||||
ii. | Curriculum: consists of courses or programmes available for students related to sustainability. | ||||
iii. | Co-curriculum activities: it includes student sustainability educators' programme, sustainability in new student's orientation, sustainability material and publications and student sustainability outreach programme. |
4.1.2 D 2 – institutional enhancement
Institutional enhancement depends on investment priorities such as shareholder advocacy, positive sustainable investments, endowment transparency, and shareholders' engagement. The emphasis on green building investments, adoption of LEED building standards, tracking of greenhouse gas emissions inventory, plans for reduction and energy efficiency are also important. Therefore, the criteria that contribute significantly to D 2 are categorised as investment priorities (X 3); buildings, operations and maintenance (X 4) and climate change and energy (X 5). The weights assigned to X 3, X 4 and X 5 are 1, 0.5 and 0.8, respectively, where X 1 is assigned a maximum value because of its direct relation with institutional enhancement.
4.1.3 D 3 – annual energy consumption rate
The criteria considered for obtaining inputs for D 3 include:
• | Transportation (X 6) is defined as campus motor fleet based on clean-burning fuels or electricity, local transportation alternatives, bicycle programmes, car-pooling and planning of policies to discourage single-occupancy vehicles and encourage use of alternative modes of transportation. | ||||
• | Waste reduction and recycling (X 7) incorporates food purchase of organic, fair trade or other sustainable food products, recycling of food, other traditional materials, electronic wastes and source reduction. | ||||
• | Water conservation (X 8) entails initiatives for water consumption and storm water management and efforts towards drinking water and bottled water. | ||||
• | Building, operations and maintenance (X 4) relates to green building criteria for all construction and renovations on a campus, such as LEED building standards. |
4.1.4 D 4 – financial/economic growth rate
The lead criterion X 3 is assigned the full weight of 1, while X 4 is weighted as 0.7, followed by X 5 at 0.6 and X 8 at 0.5.
4.1.5 D 5 – Health and safety
The lead criterion for this driving force is Planning, administration and engagement (X 9) because it includes coordination and planning, diversity and affordability, human resources, public engagement and student engagement. Sustainability coordination and facilitating student participation in institutional decision-making are also related to sustainability. The weight assigned to this criterion is 1. The lagging criteria for D 5 are X 3, X 4 and X 6.
4.1.6 D 6 – social activity index
The lead criterion for D 6 is X 9. The remaining input is received from X 7 and X 6.
4.1.7 D 7 – trends of education in sustainability
The lead contributor for D 7 is X 2 and the remaining input comes from X 9.
4.2 Activation
After the weights are established for various factors, the next step is to develop input activation values for driving forces for five universities in Canada, namely Memorial University (MUN), the University of British Columbia (UBC), the University of Toronto (UoT), the University of Alberta (UoA) and the University of McGill. Allowing for the fact that universities do not post data on the Internet every year, the latest available data were taken into consideration during the research. The input activation values defined for driving force indicators are TFNs instead of deterministic values. As shown in Section 4.1, the TFN driving forces for the five universities are obtained from the Green report card for these universities and also through extensive web-search (McGill Citation2010, MUN Citation2010, UBC Citation2010, UoA Citation2010, UoT Citation2010). It is found that the sustainability initiative at MUN is relatively new and it did not participate in the Green report card ranking for 2010; therefore, the data obtained for MUN for this research were obtained through the website and by informal discussion with the faculty. The conversion of Green report card rankings into numerical TFNs is shown in Table .
Table 4 Conversion of Green report card ranking into numerical triangular fuzzy-based numbers.
For X 2, the information was not found in the Green report card for 2010; therefore, the sustainability office website of the five selected universities was thoroughly reviewed. The information related to the above three main items (research, curriculum and co-curriculum) was investigated and it was found that UBC's sustainability website provides thorough information to all stakeholders including students, faculty, staff and social groups about the academic programmes in education and research related to sustainability and also the link to all relevant courses. Therefore, it was assigned a value of 0.9, 1 and 1. The information related to research and courses available for sustainability was not comprehensive for the remaining four universities in comparison to the UBC. Therefore, for UoA, UoF and McGill, the values were assigned as 0.7, 0.8 and 0.9. The MUN, on the other hand, has a comparatively recent sustainability initiative and there is not enough information available; therefore, the activation value was assigned as 0.3, 0.4 and 0.5. The information available for water-related initiatives for all five universities was limited, so the activation values were assumed as 0.5, 0.6 and 0.7 for all universities. Similarly, the numerical averages were taken when two or three different ranks were transferred from Green report card to a TFN used in uD-SiM.
4.3 Aggregation
Aggregation is the process by which fuzzy sets that represent the input indicators are combined or inferred as a single fuzzy set. Using a simple weighted average method, an input activation level for driving forces is obtained by using the following equation:
Table 5 Fuzzy-based activation input.
4.4 Application of uD-SiM to Canadian universities
Using input activation levels obtained through data preparation, uD-SiM was simulated following the steps explained in Section 4 for MUN, UBC, UoA, UoT and McGill. The resulting SI obtained is presented in Figure . It is found that the defuzzified SIo was 0.90 for UBC and the smallest base width for UBC indicates lowest uncertainty for this university. Therefore, SIo was highest for UBC at 0.90, followed by McGill at 0.87, UoA at 0.87, UoT at 0.84 and MUN at 0.57 (Table ).
Table 6 Comparison of Green report card rankings (2010) with uD-SiM.
It can be seen that the overall rankings of UBC, UoA and McGill were similar (B+) under the Green report card ranking. The difference between uD-SiM ranking and Green report card could be attributed to the fact that the Green report card did not consider the water use and education in sustainability (Table ). The uD-SiM ranking provides a quantitative evaluation of sustainability as compared to the Green report card ranking. Moreover, the inclusion of initiatives in education and water in this study has provided a more comprehensive sustainability-based ranking.
5. Discussion
This paper ranks universities and demonstrates that uD-SiM is not an effective decision-making tool as proved by Waheed et al. (Citation2011) but a unique ranking tool for universities. The uD-SiM examines universities based on educational, environmental, social and economic categories. The proposed model allows better understanding of the efforts of HEIs towards sustainability in a hierarchical causal linkage system and further provides opportunities for improvement or control strategies at any level of the model.
The uD-SiM is primarily a decision-making tool that enables control strategies and decision actions to be taken at any stage of the DPSEEA framework to improve the overall SI. If the estimated SI is lower than the desired value, proper ‘actions’ are selected (e.g. least cost and most effective) to avoid any serious adverse effect on the public and environment. As the linkages between the different levels in the DPSEEA framework are the focus of quantitative research and assessing the sustainability for an institution (University campus in this case), various actions can be implemented at different stages of the framework and may take a variety of forms, including prevention (policy development and standard setting), hazard management (reduction in emissions), improvement (technical control measures), protective (pollution monitoring) and corrective (such as treatment and rehabilitation) (Figure ).
Generally, environmental research focuses on linking pressure and state levels, human health research focuses on the links between state and exposure and environmental epidemiology deals primarily with the exposure to effect linkages (Corvalán et al. Citation1999). The traditional way of analysing data and taking action at the immediate or end levels does not encourage a broader analysis of the consequences for policy and prevention. The meaningful interpretation of any indicator in the framework in relation to decision-making about policies or actions should be based on an understanding of these linkages.
In a short term, actions are often corrective or remedial at the ‘effect’ stage, such as providing health care for individuals affected by poor air quality or treating waste. Actions for the long term can be various protective measures to reduce exposure, water-saving strategies and waste minimisation. The most effective long-term interventions aim at eliminating or reducing the effects of the driving forces or the environmental pressures that cause the hazards. Interventions at the level of driving forces often have multiple implications, because the major driving forces exert influence through several causal pathways. Sometimes this can multiply benefits, but care must be taken that the overall impact is beneficial.
It can be observed from the ranking of universities through uD-SiM that the SI of MUN needs improvement. To increase the SIo from 0.57 to 0.90 (Figure ), various actions at different stages of the framework (Table ) are required. An action such as developing a policy at the highest administration level for more sustainability-related courses and initiatives will enhance the SI for the university. Similarly, the commitment of university senior management, as well as energy-saving initiatives, will increase the SI. If the financial accounting is integrated with the uD-SiM, the model will guide informed decision-making and help in selecting effective and timely interventions. Actions like reduction in energy usage can only be effective in the long term for the economic growth though they take a relatively long time to implement and even longer time to produce results. The basic rule for the selection of a specific ‘action’ A i will be to maximise index change (IC i ):
Table 7 Example of actions for the MUN at various stages of uD-SiM.
Table 8 Result of various actions on SI for the MUN.
6. Conclusions and recommendations
The decision-making tool uD-SiM, based on DPSEEA coupled with MCDM and fuzzy logic, is proposed as a solution to establishing a SI for HEIs. In this paper, it is established that this model can provide objective perspective in ranking universities because it allows the decision-makers to better understand the hierarchical perspective of various levels on the final index. Moreover, this fuzzy-based model can be effectively applied to foster improvement by promoting action at any level of DSPSEEA. Better policies can definitely lead towards longer-term, broad-spectrum interventions and long-term solutions by evaluating the driving forces operating in an institution. To implement proactive preventive approaches, development policies and planning need a long-time horizon. It can be observed that those universities where sustainability is an integral part of the planning and decision-making, and where initiatives related to sustainability started decades ago have higher sustainability indices.
Clearly, further studies are needed to improve the uD-SiM by better and more comprehensive selection of indicators and assignment of weights. For demonstration, the input values for driving forces and weights were used through direct assignment by the authors; however, actual real-time values can be used for real applications. The application of uncertainty to the weights of indicators at various levels of DPSEEA and financial accounting will enhance the model and its capability to evaluate the alternatives and decision actions in terms of cost and benefits.
Acknowledgements
The authors thankfully acknowledge the financial support provided by NSERC under the Discovery Grant Program. The authors also greatly appreciate the help of Dr Rehan Sadiq for his technical advice and support in the development and application of uD-SiM.
Notes
References
- AASHE, 2010. Sustainability tracking, assessment and rating system (STARS). Available from: https://stars.aashe.org/ [Accessed 1 November 2010]
- Ahammed , M. and Melchers , R.E. 1994 . Reliability of underground pipelines subject to corrosion . Journal of Transportation Engineering , 120 ( 6 ) : 989 – 1002 .
- Baboulet , O. and Lenzen , M. 2010 . Evaluating the environmental performance of a university . Journal of Cleaner Production , 18 : 1134 – 1141 .
- Barth , M. , Godemann , J. , Rieckmann , M. and Stoltenberg , U. 2007 . Developing key competemcies for sustainable development in higher education . International Journal of Sustainability for Higher Education , 8 ( 4 ) : 416 – 430 .
- Briggs , D. , Corvalán , C. and Nurminen , M. 1996 . Linkage methods for environment and health analysis: general guidelines , Geneva : World Health Organization .
- Brulming , E. 1997 . “ Injuries and deaths on the roads: an international perspective ” . In Health at the crossroads: transport policy and urban health , Edited by: Fletcher , T. and McMichael , A. 109 – 121 . Chichester : John Wiley & Sons .
- Clarke , A. and Kouri , R. 2009 . Choosing an appropriate university or college environmental management system . Journal of Cleaner Production , 17 : 971 – 984 .
- Cole , L. 2003 . Assessing sustainability on Canadian University campuses: development of a campus sustainability assessment framework , Victoria, Canada : Royal Roads University .
- Corvalán , C.F. , Kjellström , T. and Smith , K.R. 1999 . Health, environment and sustainable development. Identifying links and indicators to promote action . Epidemiology , 10 ( 5 ) : 656 – 670 .
- CSD . 1995 . Indicators of sustainable development – guidelines and methodologies (http://www.un.org/esa/sustdev/) , New York : United Nations .
- Cullen , A.C. and Frey , H.C. 1999 . Probabilistic techniques in exposure assessment: a handbook for dealing with variability and uncertainty in models and inputs , 352 New York : Plenum Press .
- Dalal-Clayton , B. and Bass , S. 2002 . Sustainable development strategies , 1st ed. , 358 London : Earthscan Publications Ltd. .
- Dempster , A. 1967 . Upper and lower probabilities induced by a multi-valued mapping . The Annals of Statistics , 28 : 325 – 339 .
- Dubois , F. and Parade , H. 1988 . Possibility theory: an approach to computerized processing of uncertainty , New York : Plenum Press .
- Ferson , S. , Joslyn , C.A. , Helton , J.C. , Oberkampf , W.L. and Sentz , K. 2004 . Summary from the epistemic uncertainty workshop: consensus amid diversity . Reliability Engineering & System Safety , 85 : 355 – 369 .
- Guyonnet , D. , Côme , B. , Perrochet , P. and Parriaux , A. 1999 . Comparing two methods for addressing uncertainty in risk assessments . Journal of Environmental Engineering , 125 ( 7 ) : 660 – 666 .
- EEA . 2001 . Environmental signals , Copenhagen : European Environment Agency . Report 8
- Evangelinos , K.I. , Jones , N. and Panoriou , E.U. 2009 . Challenges and opportunities for sustainability in regional universities: a case study in Mytilene, Greece . Journal of Cleaner Production , 17 : 1154 – 1161 .
- Goognough , T. , Kildegaard , A. , Kuchenreuther , M. , Rasmussen , L. and Wyckoff , P. 2009 . Leveraging assets: a case study of the sustainability initiative at the University of Minnesota, Morris . Journal of Cleaner Production , 17 : 1138 – 1142 .
- Green Report Card, 2010. Green report card for 2010. Available from: http://www.greenreportcard.org [Accessed 1 November 2010]
- GRI, 2006. Sustainability reporting guidelines. Global Reporting Initiative, Amsterdam, The Netherlands
- Klir , G.J. 1995 . Principles of uncertainty: what are they? Why do we need them? . Fuzzy Sets and Systems , 74 : 15 – 31 .
- Klir , G.J. and Yuan , B. 1995 . Fuzzy sets and fuzzy logic- theory and applications , New Jersey : Prentice-Hall, Inc .
- Lee , H.-M. 1996 . Applying fuzzy set theory to evaluate the rate of aggregative risk in software development . Fuzzy Sets and Systems , 79 : 323 – 336 .
- Lozano , R. 2006a . Incorporation and institutionalization of SD into universities: breaking through barriers to change . Journal of Cleaner Production , 14 ( 3 ) : 786 – 796 .
- Lozano , R. 2006b . A tool for a graphical assessment of sustainability in universities (GASU) . Journal of Cleaner Production , 14 ( 2 ) : 963 – 972 .
- Lozano , R. 2008 . Envisioning sustainability three-dimensionally . Journal of Cleaner Production , 18 : 1838 – 1846 .
- Lozano , R. 2009 . Diffusion of sustainable development in universities' curricula: an empirical example from Cardiff University . Journal of Cleaner Production , 18 : 637 – 644 .
- Lukman , R. , Krajnc , D. and Glavič , P. 2010 . University ranking using research, educational and environmental indicators . Journal of Cleaner Production , 18 : 619 – 628 .
- McGill, 2010. Available from: http://www.mcgill.ca/sustainability/ [Accessed 1 November 2010]
- Mebratu , D. 1998 . Sustainability and sustainable development: historical and conceptual review . Environmental Impact Assessment Review , 18 : 493 – 520 .
- MUN, 2010. Available from: http://www.mun.ca/sustain/sustainability_office/ [Accessed 1 November 2010]
- OECD, 1999. Towards sustainable development: indicators to measure progress, organisation for economic co-operation and development (OECD) conference. Rome, 15–17 December 1999
- Rodriguez, S.I., Roman, M.S., Sturhahn, S.C., and Terry, E.H., 2002. Sustainability assessment and reporting for the University of Michigan's Ann Arbor Campus. Master's thesis. University of Michigan, Ann Arbor, MI
- Savitz , A.W. and Weber , K. 2006 . The triple bottom line: how today's best-run companies are achieving economic, social and environmental success – and how you can too , 320 San Francisco, CA : John Wiley & Sons, Inc .
- Shafer , G. 1976 . A mathematical theory of evidence , Princeton, NJ : Princeton University Press .
- Shriberg , M. 2002 . Towards sustainable management: the University of Michigan housing division's approach . Journal of Cleaner Production , 10 : 41 – 45 .
- Tesfamariam , S. and Sadiq , R. 2006 . Risk-based environmental decision-making using fuzzy analytic hierarchy process (F-AHP) . Stochastic Environmental Research and Risk Assessment , 21 ( 1 ) : 35 – 50 .
- UBC . 2007a . The sustainability strategy , Vancouver, Canada : The University of British Columbia .
- UBC . 2007b . The UBC sustainability report 2006–2007 , Vancouver, Canada : The University of British Columbia .
- UBC, 2010. Available from: http://www.sustain.ubc.ca/ [Accessed 1 November 2010]
- ULSF, 1990. Talloires declaration. Association of University Leaders for a Sustainable Future. Available from: http://www.ulsf.org [accessed 11 January 2010]
- UN . 1996 . Indicators of sustainable development- framework and methodologies , New York : United Nations .
- UNESCO, 1990. Kyoto declaration. Ninth international association of universities round table
- UNESCO . 1991 . The Halifax Declaration , Paris, France : United Nations Educational, scientific and cultural organization .
- UNESCO . 1993 . The Swansea Declaration , Paris, France : UNESCO .
- UoA, 2010. Available from: http://www.sustainability.ualberta.ca/ [Accessed 1 November 2010]
- UoT, 2010. Available from: http://www.sustainability.utoronto.ca/Page4.aspx [Accessed 1 November 2010]
- Viebahn , P. 2002 . An environmental management model for universities: from environmental guidelines to staff involvement . Journal of Cleaner Production , 10 ( 4 ) : 3 – 12 .
- Waheed , B. , Khan , F. , Veitch , B. and Hawboldt , K. 2011 . Uncertainty-based quantitative assessment of sustainability for higher education institutions . Journal of Cleaner Production , 19 ( 7 ) : 720 – 732 .
- WCED . 1987 . Our common future (The Brundtland Report) , Oxford, UK : Oxford University Press .
- WHO, 2010. Available from: http://www.who.int/mediacentre/events/IndicatorsChapter7.pdf
- WRI . 2005 . World Resources 2005: the wealth of the poor, managing ecosystems to fight poverty , Washington, DC : World Resources Institute .
- Yager , R.R. 1980 . On choosing between fuzzy subsets . Kybernetes , 9 : 151 – 154 .
- Zadeh , L.A. 1965 . Fuzzy sets . Information and Control , 8 ( 3 ) : 338 – 353 .
Appendix A
Uncertainty modelling
There are two kinds of uncertainties: the first arises as variability resulting from heterogeneity or stochasticity, and the second arises from partial ignorance, systematic measurement error or subjectivity (epistemic uncertainty). Epistemic uncertainty (incomplete knowledge) dominates the decision analysis problems, such as the health effects by exposure to unknown contaminants and the economical risks associated with climate change. It plays an important role when the evidence base is small, such as the case of sustainability assessment of higher education institutes. These uncertainties are critical to analyse because of associated high consequence due to failures (Ferson et al. Citation2004).
Traditionally, probabilistic methods have been used to quantify and display uncertainties. The probabilistic methods are designed and refined over time (using Bayesian approach) to propagate uncertainties. Major probabilistic risk analysis applications have been in the fields of industrial, aeronautical, environmental, petroleum, nuclear and chemical engineering. In civil engineering, the probabilistic methods handling risk and uncertainties were developed for the analysis of structural reliability using analytical or numerical integration, simulation, moment-based methods, or first- and second-order methods (FORM/SORM) of approximation of the limit state of a system (Ahammed and Melchers Citation1994). They are now the basis for the design codes for common structures.
Both set theory and probability theory are the classical mathematical frameworks for characterising uncertainty. Since 1960s, a number of generalisations of these frameworks became available for formalising various types of uncertainties. Klir (Citation1995) reported that well-justified measures of uncertainty of relevant types are now available not only in the classical set theory and probability theory but also in the fuzzy set theory (Zadeh Citation1965), possibility theory (Dubois and Parade Citation1988), and the Dempster–Shafer theory (Dempster Citation1967, Shafer Citation1976). In 1965, Zadeh introduced fuzzy logic and fuzzy set theory, which is widely used in representing uncertain knowledge. The parameters of uncertainty model can be treated as fuzzy numbers that can be manipulated by specially designed operators. Later, Klir (Citation1995) proposed a comprehensive general information theory to encapsulate these concepts into a single framework.
Fuzzy set theory
Fuzzy set theory effectively deals with uncertainties encompassing vagueness to approximate reasoning and help in representing and propagating the uncertainties throughout the decision process. Fuzzy-based techniques are a generalised form of interval analysis used to address uncertain or imprecise information. To qualify as a fuzzy number, a fuzzy set must be normal, convex and bounded (Klir and Yuan Citation1995). Any shape of a fuzzy number is possible, but generally because of simplicity triangular or trapezoidal fuzzy numbers are used (Lee Citation1996). A fuzzy set is an extension of the classical set theory ( is either a member of set A or not) in which an
can be a member of set A with a certain membership function μ
x
. A fuzzy number describes the relationship between an uncertain quantity x and a membership function, which ranges between 0 and 1, μ: R → [0, 1] ⊆ R. Figure shows a TFN. The membership function μ determines the imprecision through the shape of the fuzzy number. Values x ∈ R for which μ(x) = 1 are said to have full membership, values x ∈ R for which 0 < μ(x) < 1 are said to have partial membership, and values x ∈ R for which μ(x) = 0 are said to have no membership to the fuzzy number. TFN is represented by three points (a, b, c) on the universe of discourse, representing the minimum, most likely and maximum value, respectively. The wider the support of the membership function, the higher the uncertainty. In this work, to simplify the implementation, a TFN is selected. Although any fuzzy number shape is possible, the selected shapes are justified by available information (Guyonnet et al.
Citation1999).
Fuzzy arithmetic
One important feature of fuzzy numbers (sets) is the concept of α-cut (Figure A.). The α-cut of a fuzzy set is a crisp set A
α that contains all the elements of the universal set X whose membership grades in A are greater than or equal to the specified value of an α-cut, i.e. (Klir and Yuan Citation1995). Fuzzy operations are carried out on fuzzy numbers using fuzzy arithmetic. Fuzzy arithmetic is based on two properties (Table A.):
1. | each fuzzy number can fully and uniquely be represented by its α-cut, and
Figure A.1 Triangular fuzzy number (TFN) (adapted from Waheed et al. Citation2011). ![]()
Table A.1 Fuzzy arithmetic operators using two TFNs. | ||||
2. | α-cuts of each fuzzy number are closed intervals of real numbers for all |
Fuzzy numbers can represent vagueness or imprecision in the parameter(s). The linguistic input values (driving forces) in D-SiM can be easily described using TFNs. The uncertainties can be propagated through the D-SiM using fuzzy arithmetic operations.