Abstract
The increasing environmental degradation, fossil fuel prices and political implications of fossil fuel dependency have increased the demand of battery electric vehicles and plug-in hybrid vehicles with lithium-ion batteries (LiBs). However, LiBs use various materials such as plastics, copper, aluminium and nickel, along with scarce metals such as cobalt and lithium. Therefore, the recycling of these batteries is important but the selection of recycling process for these batteries is a complex problem. Alternative recycling processes have to be evaluated with respect to different criteria such as energy consumption, material recovery, environmental impact, health and safety, processing time, degree of flexibility, initial investment and labour cost. This paper provides an integrated fuzzy multi-criteria decision model using fuzzy analytical hierarchical process and fuzzy technique for order preference by similarity to ideal solution for the evaluation of different recycling alternatives for LiBs. The proposed model has been validated by a case study.
Introduction
In the last two decades, rapid economic growth of India and China, the most populated countries, has increased the demand of vehicles in the market. In the major cities of these countries, an increase in the number of vehicles on the roads per day has led to huge environmental degradation in terms of air quality and emissions. It has also severely affected the quality of life in these cities. The public have also become aware of the environmental issues affecting them. This has forced the governments to introduce stringent emission regulations for the vehicles (Badami Citation2005, Pucher et al.Citation2005). Auto manufacturers are not only trying to comply with these ever-changing regulations but also working hard to beat the competition among themselves. Environment-friendly vehicles provide an edge over competitors. In addition to this, the increasing prices of fossil fuels and the political implications of fossil fuel dependency on few countries have made the governments and manufacturers to think about vehicles with alternative fuel. The number of electric vehicles in recent years has increased, and this trend will continue. For example, the German government has aimed at a market penetration of 1 million plug-in hybrid vehicles and battery electric vehicles by 2020 (Nemry and Brons Citation2010). An essential component of such vehicles is the lithium-ion battery (LiB) that has high-specific power, high-energy density and long life. The primary non-ferrous resources required for the manufacturing of these batteries are copper, cobalt, nickel and lithium. Unfortunately, these resources are also scarce and some of them such as cobalt and lithium are available only in a few countries. According to the U.S. Geological Survey (Citation2010), seven countries account for 85% of the world's production of mined cobalt and lithium. Recycling of LiB needs to be focused due to scarcity of the resources used in the production of these batteries. Recycling provides economic benefits and lessens the dependency on the countries producing cobalt and lithium.
There are few processes for recycling of portable LiBs with the primary objective of cobalt recovery. However, the chemistry in LiBs intended for vehicle applications is quite different (Ekermo Citation2009). Portable LiBs use lithium cobalt oxide, which offers high-energy density, but has well-known safety concerns, especially when damaged. However, vehicle batteries use lithium iron phosphate, lithium manganese oxide and lithium nickel manganese cobalt oxide, which offer lower energy density, but longer lives and inherent safety. Hereafter, in this paper LiB means the lithium ion batteries used in vehicles. In general, disassembly, mechanical conditioning, pyrometallurgy and hydrometallurgy are used in recycling of LiBs to separate the different non-iron metals and other materials from each other. These four processes are usually used in the combination of two or more to recycle or recover the main components from spent LiBs (Kwade Citation2010) as shown in Figure .
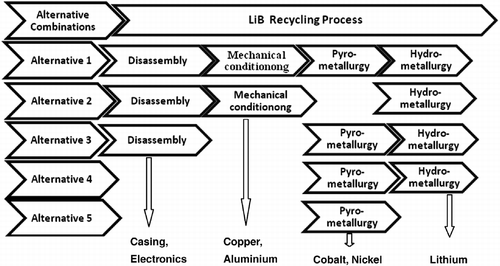
The evaluation is a major hurdle in the justification and selection of any multifaceted process/technology/system (Sangwan Citation2011). The evaluation of different battery recycling alternatives is also a multifaceted problem; the alternatives are to be evaluated on the basis of various criteria. Traditional economic evaluation techniques require hard-core quantitative data that may be difficult to retrieve; hence, it may be highly inappropriate to apply these techniques for the multifaceted problems. To overcome this dilemma, a multi-criteria decision model has been proposed for the evaluation of alternative LiB recycling processes. The selection of the best alternative combination depends on many potential criteria such as energy consumption, material recovery, environmental impact, health and safety, processing time and degree of flexibility (Hoyer et al.Citation2011). Furthermore, many uncertainties are related to input, output and processes of recycling. Input-related uncertainties can be classified into quantity, spatial distribution and specification of the battery returns. Output-related uncertainties can be related to product reuse, component reuse and material reuse opportunities. Process-related uncertainties exist with respect to optimal combination and configuration of processes (Hoyer et al.Citation2011). It is well known that fuzzy logic is the best way to deal with uncertainty and vagueness (Karimi et al.Citation2011).
Therefore, this paper presents an integrated multi-criteria decision model comprising fuzzy technique for order preference by similarity to ideal solution (TOPSIS) and fuzzy analytical hierarchical process (AHP) to evaluate and select the best recycling process for LiBs. The model has been validated by a case study with the help of four German industrial experts involved in the recycling of LiBs under the project LithoRec. It is expected that the proposed model will provide the decision makers/managers sufficient confidence for evaluation of alternative recycling processes for LiBs to select the best alternative under the given circumstances. The paper is organised as follows. Section 2 provides a brief review of various recycling processes used for LiB recycling. An overview of AHP and TOPSIS methods has been given in Section 3. The proposed research methodology and its elucidation using the case study are given in Section 4. Results are discussed in Section 5 followed by conclusions in Section 6.
Background
LiBs are rechargeable with promising properties for electric mobility due to their high-power density and specific energy. LiB cells consist of a cathode, an anode, a separator, an electrolyte and a casing. Cathode and anode conductors are usually made of aluminium and copper foils, respectively. The cathode is coated with either a mix of lithium or other metals such as cobalt, nickel, manganese and aluminium (LiMeO2) or lithium iron phosphate (LiFePO4). The anode is usually coated with either graphitic or amorphous carbon or lithium-alloying metals (Shukla and Kumar Citation2008). Recycling processes for LiBs are classified into disassembly, mechanical conditioning, hydrometallurgical conditioning and pyrometallurgical conditioning (Kwade Citation2010). These four processes are used in combination with each other as discussed later in this section.
Disassembly
In disassembly, battery systems are broken down to module and cell level, a prerequisite for remanufacturing of batteries. Reusable components and large material fractions, e.g. the battery casing and electronics, are separated before further conditioning. Being a manual process, it requires high expenditure on labour. Scarce metals such as cobalt, nickel and lithium cannot be separated and recovered by disassembly alone.
Mechanical conditioning
In mechanical conditioning, materials of the cells are separated by different comminuting, sizing and concentration processes such as crushing, screening and magnetic separation. Mechanical separation processes cannot separate all components in spent LiBs as these are composed of several metals, organic substances and inorganic substances, which penetrate into each other. These materials are present in small volumes with accurate, fine and complicated structure; therefore, these components are difficult to separate from each other by mechanical separation processes (Xu et al.Citation2008). Mechanical conditioning can recover copper and aluminium but scarce metals such as cobalt, nickel and lithium cannot be recovered. It can be a necessary preparation for subsequent recovery.
Pyrometallurgical conditioning
Pyrometallurgical conditioning is the thermal treatment of the materials at high temperatures. Pyrometallurgy of LiBs can be easily combined with the production of steel and other ferromanganese alloys. Thermal treatment has the advantage of having simple and convenient operations. However, in this technique it is not possible to recover organic compounds as these get burnt. Costly equipment for purifying the smoke and gas resulting from combustion of carbon and organic compounds needs to be installed. Because of its relative simplicity, it is the most commonly used process (Kwade Citation2010).
Hydrometallurgical conditioning
Hydrometallurgical conditioning typically combines leaching, solution concentration and purification. The hydrometallurgical process is generally used to recover non-ferrous metals from active materials after mechanical conditioning or from the slag of pyrometallurgical process. Although it separates lithium from other components, it is a complex and costly process.
As LiBs consist of a variety of different materials, a single recycling process alone can recover a part of these components. Therefore, a combination of different recycling processes is necessary to recycle or recover all the relevant materials from spent LiBs (Xu et al.Citation2008). Several recycling process chains have been developed for the recycling of portable LiBs with the primary objective of cobalt recovery (Lupi et al.Citation2005). However, the chemistry in LiBs intended for vehicle applications is quite different. The weight of a vehicle battery ranges from 30 to 300 kg, but the battery from a laptop computer weighs only about 300 g. Thus, the potential hazards of dismantling are very different. In order to be well prepared for end-of-life treatment of the batteries, new recycling process combinations must be developed for vehicle LiBs. Figure shows different process combination possibilities and the resultant recovered materials. Each combination has its advantages and disadvantages based on potential criteria such as energy consumption, material recovery, environmental impact, health and safety, processing time, degree of flexibility, initial investment and labour cost. Therefore, an integrated fuzzy multi-criteria decision model is developed to evaluate various LiB recycling process alternatives.
Overview Of Ahp And Topsis
The AHP, first introduced by Saaty (Citation1980), is an effective method for solving multi-criteria decision problems. Traditional AHP requires exact or crisp judgements. However, due to the complexity and uncertainty involved in real-world decision problems, like the uncertainties involved in the recycling of LiB, decision makers may be reluctant to provide crisp judgements. The preferences in AHP are essentially judgements based on human perceptions, so fuzzy approaches allow for a more accurate description of the decision-making process (Chen et al.Citation2008). TOPSIS method was first proposed by Hwang and Yoon (Citation1981). The basic concept of this method is that the chosen alternative should have the shortest distance from the positive ideal solution and the farthest distance from the negative ideal solution. Positive ideal solution is a solution that maximises the direct criteria and minimises the indirect criteria, whereas the negative ideal solution maximises the indirect criteria and minimises the direct criteria (Wang and Elhag Citation2006). In the classical TOPSIS method, the weights of the criteria and the ratings of alternatives are known precisely and crisp values are used in the evaluation process. However, under many conditions, crisp data are inadequate to model real-life decision problems. Therefore, the fuzzy TOPSIS method is proposed where ratings of alternatives are evaluated by linguistic variables represented by fuzzy numbers to deal with the deficiency in the traditional TOPSIS.
Both AHP and TOPSIS have their own advantages. First, AHP helps to decompose an unstructured problem into a reliable hierarchic structure that includes various criteria, sub-criteria and alternatives to determine the best choice. Second, it can elicit judgements from decision makers to determine the weights of the elements. Third, it uses an admissible consistency ratio to validate the consistency of the decision-making process. However, sometimes, a large number of pairwise comparisons made by decision makers can cause impractical usage of the AHP process, especially in fuzzy AHP. To cope with this problem, fuzzy TOPSIS method can be used to reduce the number of pairwise comparisons and to rank the alternatives (Önüt et al.Citation2008). TOPSIS method is a rational, understandable and easily programmable computation procedure. With the integrated application of AHP and TOPSIS methods, evaluation process has advantages of the two methods. Therefore, a more realistic approach may be the use of an integrated AHP and TOPSIS methodology (Perçin Citation2009). Pires et al. (Citation2011) integrated AHP with TOPSIS to help decision-making for solid waste management.
The proposed research methodology
In this paper, an integrated fuzzy AHP and fuzzy TOPSIS approach is proposed for the evaluation of recycling processes of LiBs. Fuzzy AHP is used to calculate the criteria weights and fuzzy TOPSIS is used to rank the alternatives. This section presents a case study to evaluate the LiB recycling alternatives with the help of four German experts involved in the recycling of LiBs under the project LithoRec. However, to retain confidentiality the names and organizations are not mentioned. The various steps of the proposed methodology are presented in Figure and elucidated here.
Selection of recycling alternatives
The first step is to select the various alternative process combinations for the recycling of LiBs from the literature review and discussion with recycling experts. The five selected alternatives as shown in Figure are disassembly–mechanical conditioning–pyrometallurgy–hydrometallurgy combination (A1), disassembly–mechanical conditioning–hydrometallurgy combination (A2), disassembly–pyrometallurgy–hydrometallurgy combination (A3), pyrometallurgy–hydrometallurgy combination (A4) and pyrometallurgy (A5).
Selection of recycling criteria
This step involves the selection of criteria for evaluating alternative recycling process combinations. These criteria are obtained from the literature review and discussion held with recycling experts. Eight criteria selected for the present problem are given in Table . Furthermore, these criteria were divided into direct and indirect criteria in which direct means more the better (e.g. degree of flexibility) and indirect means less the better (e.g. cost).
Table 1 Criteria influencing recycling process.
Selection of fuzzy linguistic variables and membership functions
In fuzzy set theory, conversion scales are applied to transform the linguistic terms into fuzzy numbers. In this paper, a scale of 1–9 for rating the criteria and the alternatives has been used. Table presents the linguistic variables and membership functions for the alternatives and criteria (Önüt et al.Citation2008). The membership functions are expressed via the triangular fuzzy numbers (TFNs) in order to represent the relative importance among the hierarchy's criteria (Zhu et al.Citation1999). The reason for using TFNs to capture the vagueness of the linguistic assessments is that TFN is intuitively easy to use (Liang and Wang Citation1994). A TFN is fully characterised by a triple real number (a, b, c), where parameter ‘b’ gives the maximal grade of the membership function μ(x) and parameters ‘a’ and ‘c’ are the lower and upper bounds that limit the field of the possible evaluation. The TFN membership is calculated as:
Table 2 Linguistic terms and fuzzy ratings for the alternatives and criteria.
Calculation of criteria weight with fuzzy AHP
The steps followed to calculate the criteria weight using fuzzy mathematics in conjunction with AHP are as follows (Chang Citation1996):
Each expert/decision maker (Dk) individually carries out pairwise comparison as below.
Table 3 Fuzzy evaluation matrix.
Next, the fuzzy priority weights of all criteria are determined by using extent analysis (Chang Citation1996)
In the method, the ‘extent’ is quantified by using a fuzzy number. According to the extent analysis method, each object could be taken to carry out extent analysis for each goal. On the basis of the fuzzy values for the extent analysis of each object, a fuzzy synthetic degree value can be obtained, which is defined as follows:
The fuzzy synthetic extents of the eight criteria computed as above are as follows:
Next, compare the degree of possibility of Sc2 ≥ Sc1. As Sc1 = (l1, m1, u1) and Sc2 = (l2, m2, u2) are two TFNs. The degree of possibility of Sc2 ≥ Sc1 is defined by
Table 4 Fuzzy value comparison for all criteria.
Finding of the priority weight for criteria using minimum operator
To compare Sc1 and Sc2, both the values of V (Sc1 ≥ Sc2) and V (Sc2 ≥ Sc1) are needed.
The degree of possibility for a convex fuzzy number to be greater than k convex fuzzy numbers Scj (j = 1, 2, …, k) is given by:
The normalised weight for criteria is computed as
Assessment of recycling alternatives with fuzzy TOPSIS
This section presents a fuzzy TOPSIS model, developed for the assessment of recycling alternatives for LiBs using fuzzy mathematics in conjunction with TOPSIS. The various steps of the model are as follows:
Assignment of rating to the alternatives
First, each decision maker gives linguistic rating for the recycling alternatives. Table shows the linguistic rating of each alternative by all decision makers with respect to all criteria. In this case study, there are five alternatives, eight criteria and four decision makers.
Table 5 Aggregate fuzzy rating for the alternatives.
Computation of aggregate fuzzy ratings for the alternatives
The fuzzy ratings of all decision makers are described as TFNs , k = 1, 2, …, K, then the aggregated fuzzy rating is given by
, where
Computation of the fuzzy decision matrix
The fuzzy decision matrix for the alternatives is shown in Table .
Table 6 Fuzzy decision matrix for the alternatives.
Normalisation of the fuzzy decision matrix
The decision matrix is normalised using a linear scale transformation to bring the various criteria scales to a comparable scale. The normalised fuzzy decision matrix is given by
Table 7 Normalised fuzzy ratings for the alternatives.
Computation of the weighted normalised matrix
The weighted normalised matrix for criteria is computed by multiplying the normalised fuzzy decision matrix
by the weights
of evaluation criteria. The weights of criteria were already calculated in Section 4.4.
The weighted normalised matrix is shown in Table .
Table 8 Weighted normalised alternatives.
Computation of the fuzzy positive ideal solution (FPIS) and the fuzzy negative ideal solution (FNIS)
The FPIS and FNIS of the alternatives are computed as follows and the values are shown in last two rows of Table 8.
Computation of the distance of each alternative from FPIS and FNIS
The distance of each weighted alternative () from FPIS (
) and FNIS (
) is given by
and
, respectively, and is shown in Table . This distance is calculated by vertex method, i.e. if
and
are two TFNs, then the vertex distance is given by:
Table 9 Distance of alternatives.
Computation of the closeness coefficient (CCij) and aggregate closeness coefficient (Ci) of each alternative
The closeness coefficient CCij represents the distances to the FPIS and the FNIS
simultaneously. Closeness coefficient of alternatives with respect to each criterion is calculated by the following equation and is shown in Table .
Table 10 Closeness coefficient for alternatives with respect to each criterion.
The aggregate closeness coefficient (CCi) and distance of each alternative from FPIS and FNIS are shown in Table . The closeness coefficient of alternatives with respect to each criterion and the aggregate closeness coefficient are shown in Figure for easy comparison.
Table 11 Aggregate closeness coefficient for alternatives.
Ranking of the alternatives
The alternatives are ranked according to the closeness coefficient (CCi) in decreasing order, and the alternative with the highest closeness coefficient is selected for final implementation. The best alternative is closest to the FPIS and farthest from the FNIS. Therefore, from Table the ranking of alternatives under the decreasing order of CCi is as follows:
Results and discussion
The computed weights for the eight criteria to evaluate the various recycling alternatives using fuzzy AHP method are 0.159 (health and safety), 0.156 (environmental impact), 0.155 (material recovery), 0.134 (degree of flexibility), 0.131 (labour cost), 0.122 (energy consumption), 0.09 (processing time) and 0.052 (initial investment) as given in Section 4.4. It shows that under the given circumstances, the four experts perceive health and safety aspects of the recycling process (weight 0.159) as the most important criterion to select the different recycling alternatives for LiBs. This is closely followed by the environmental impact (weight 0.156) of the process and the amount of material recovered (weight 0.155) by the process. The initial investment required for the recycling process is given the least importance (weight 0.052). The recycling time is also given a low importance (weight 0.09).
It can be observed from Tables and and Figure that the best alternative under the given circumstances is the combination of hydrometallurgy and pyrometallurgy. However, it is interesting to note that this combination is not the best on any individual criterion, it shares the best position with alternative A5 (pyrometallurgy) for health and safety criterion. This is because this combination performs consistently well on most of the criteria. Alternative A5 (Pyrometallurgy) is the best recycling process if users consider initial investment, labour cost, energy consumption and processing time criteria. However, pyrometallurgical conditioning provides poor material recovery as some of the recoverable materials get burnt. The burning of materials also leads to poor environmental impact. The degree of flexibility is also less in pyrometallurgical conditioning. Alternative A1 (disassembly, mechanical conditioning, pyrometallurgical and hydrometallurgical conditioning combination) provides the highest material recovery and degree of flexibility as all materials are separated out and recovered by different processes. Alternative A2 (disassembly, mechanical conditioning and hydrometallurgical conditioning combination) is the best recycling chain as far as environmental impact is concerned. This is because it does not include the pyrometallurgical conditioning in which the burning of parts such as casing and electronics releases high effluents to the environment. Pyrometallurgical conditioning alone (alternative A5) is a quick and cost-effective recycling process. It ranks best for five criteria but because of very poor ranking/score for environmental impact and material recovery makes this process least ranked under the given circumstances. It will be interesting to find how this process will be ranked by the users/experts from developing countries where the cost will be a dominating factor. Therefore, the results obtained in this study cannot be standardised as these depend on the knowledge, experience and input of the decision makers. As this case study has been done only with the help of German experts, one of its consequences may be that high weights were obtained for environmental impact and health and safety criteria. However, for developing and emerging countries, the initial investment may be more important resulting in different rankings. The objective of this study, was is to provide a robust process for the evaluation of different LiB recycling processes and not the final result.
Conclusion
An integrated multi-criteria decision model has been developed for the evaluation of LiB recycling processes using eight criteria of energy consumption, material recovery, environmental impact, health and safety, processing time, degree of flexibility, initial investment and labour cost. In the first step, the weights for the different criteria were computed using a fuzzy AHP. In the second step, a fuzzy TOPSIS has been used for computing the ranking of different recycling processes. The AHP has been used to ensure that the decisions of all experts are consistent. The inherent uncertainty in input, output and processes has been taken care of by the use of fuzzy logic. Moreover, fuzzy logic made it possible to treat the qualitative data to provide crisp values. Under the given circumstances and background of expert experience, it has been found that the best recycling process for LiBs is the combination of pyrometallurgy and hydrometallurgy.
REFERENCES
- Badami, M.G., 2005. Transport and urban air pollution in India. Environmental Management, 36 (2), 195–204.
- Chang, D.-Y., 1996. Applications of the extent analysis method on fuzzy AHP. European Journal of Operational Research, 95 (3), 649–655.
- Chen, M.-F., Tzeng, G.-H., and Ding, C.G., 2008. Combining fuzzy AHP with MDS in identifying the preference similarity of alternatives. Applied Soft Computing, 8 (1), 110–117.
- Ekermo, V., 2009. Recycling opportunities for Li-ion batteries from hybrid electric vehicles. Master of Science. Chalmers University of Technology, Göteborg, Sweden.
- Hoyer, C., Kieckhäfer, K., and Spengler, T.S., 2011. A strategic framework for the design of recycling networks for lithium-ion batteries from electric vehicles. In: J.Hesselbach and C.Herrmann, eds. Glocalized solutions for sustainability in manufacturing. Berlin, Heidelberg: Springer, 79–84.
- Hwang, C.L. and Yoon, K., 1981. Multiple attributes decision making methods and applications. Berlin: Springer.
- Karimi, A.R., et al., 2011. Using of the fuzzy topsis and fuzzy ahp methods for wastewater treatment process selection. International Journal of Academic Research, 3 (1), 737–745.
- Kwade, A., 2010. On the way to an “intelligent” recycling of traction batteries. In: 7thBraunschweiger Symposiumon Hybrid, Electric Vehicles and Energy Management, Braunschweigh.
- Liang, G.-S. and Wang, M.-J.J., 1994. Personnel selection using fuzzy MCDM algorithm. European Journal of Operational Research, 78 (1), 22–33.
- Lupi, C., Pasquali, M., and Dell'Era, A., 2005. Nickel and cobalt recycling from lithium-ion batteries by electrochemical processes. Waste Management, 25 (2), 215–220.
- Nemry, F. and Brons, M., 2010. Plug-in hybrid and battery electric vehicles: market penetration scenarios of electric drive vehicles [online]. Available from: http://ftp.jrc.es/EURdoc/JRC58748_TN.pdf [Accessed 1 February 2011].
- Önüt, S., Soner Kara, S., and Efendigil, T., 2008. A hybrid fuzzy MCDM approach to machine tool selection. Journal of Intelligent Manufacturing, 19 (4), 443–453.
- Perçin, S., 2009. Evaluation of third-party logistics (3PL) providers by using a two-phase AHP and TOPSIS methodology. Benchmarking: An International Journal, 16 (5), 588–604.
- Pires, A., Chang, N.-B., and Martinho, G., 2011. An AHP-based fuzzy interval TOPSIS assessment for sustainable expansion of the solid waste management system in Setúbal Peninsula, Portugal. Resources, Conservation and Recycling, 56 (1), 7–21.
- Pucher, J., et al., 2005. Urban transport crisis in India. Transport Policy, 12 (3), 185–198.
- Saaty, T.L., 1980. The analytic hierarchy process: planning, priority setting, resource allocation. New York: McGraw Hill.
- Sangwan, K.S., 2011. Development of a multi criteria decision model for justification of green manufacturing systems. International Journal of Green Economics, 5 (3), 285–305.
- Shukla, A.K. and Kumar, T.P., 2008. Materials for next-generation lithium batteries. Current Science, 94 (3), 314–331.
- U.S. Geological Survey, 2010. Mineral commodity summaries [online]. U.S. Government Printing Office. Available from: http://minerals.usgs.gov/minerals/pubs/mcs/2010/mcs2010.pdf [Accessed 4 January 2011].
- Wang, Y.-M. and Elhag, T.M.S., 2006. Fuzzy TOPSIS method based on alpha level sets with an application to bridge risk assessment. Expert Systems with Applications, 31 (2), 309–319.
- Xu, J.Q., et al., 2008. A review of processes and technologies for the recycling of lithium-ion secondary batteries. Journal of Power Sources, 177 (2), 512–527.
- Zhu, K.-J., Jing, Y., and Chang, D.-Y., 1999. A discussion on extent analysis method and applications of fuzzy AHP. European Journal of Operational Research, 116 (2), 450–456.