Abstract
The rising energy prices and increasingly imposing regulations have drawn attention for improving the energy efficiency of manufacturing processes. Over the past decade, different approaches have been developed to target from individual unit processes to entire manufacturing systems. However, each model or approach has limitations in terms of the efficiency improvement. Thus, an integrated approach is proposed to overcome these limitations combining unit process energy consumption models with production system simulation. The outcome potentially leads to a more energy-efficient production and process planning, which considers the dynamics of individual processes as well as the entire system. Two case studies are presented to demonstrate the approach and its benefits.
1. Introduction
Energy plays an indispensable role in manufacturing and provides the power needed by the system for the purpose of production. Also, it accounts for a great portion of production cost and greenhouse gases emissions. This is particularly true for the electrical energy consumption. Over the past three decades, the electricity price has increased steadily, meanwhile the global consumption by the manufacturing sector has also trebled (IEA Citation2012). In addition, governmental initiatives and regulations have further urged manufacturing industries to reduce their energy consumption as well as to improve their environmental performance. As a result, manufacturers no longer treat energy as an overhead cost source but as a valuable and manageable resource. Correspondingly, research efforts have been attracted worldwide to improve the energy efficiency in manufacturing (Duflou et al. Citation2012).
According to the review by Duflou et al. (Citation2012), existing energy modelling studies in manufacturing were focused on two main areas. On the one hand, an individual unit process is targeted for energy consumption prediction. A great number of studies are conducted aiming to develop detailed and accurate models. The existing models contribute to a better understanding of different unit processes in terms of the energy efficiency as well as improvement potentials. However, they are significantly restricted to be applied for modelling of a whole manufacturing system, since they lack consideration of the effects of parameters in upper levels, such as batch size (Kara and Li Citation2011). On the other hand, several researchers have taken into account the consumption of energy at system level. Predominantly, discrete event simulation is applied aiming to find systematically the right answers to the questions of how much energy is needed at which time and place (Herrmann et al. Citation2011). A comprehensive energy-oriented simulation model helps to evaluate the system performance regarding dynamic changes in production parameters (e.g. schedules) and other factors (e.g. energy prices) (Weinert, Chiotellis, and Seliger Citation2011). However, the effects of changing process parameters at the unit process level are excluded in the simulation. As a result, the existing works on both areas cannot integrally improve the energy efficiency from the unit process throughout to the system level.
Therefore, this paper proposes an integrated modelling approach to simulate the energy consumption of single unit processes and production systems as well as to investigate the effects of machine tool parameterization on the energy efficiency of production systems.
2. Theoretical background
Depending on the scale of the intended system, different modelling approaches have been proposed to improve production and energy efficiency of manufacturing systems. Duflou et al. (Citation2012) presented a comprehensive review of the related manufacturing research, and classified them according to organizations, i.e. from supply chain to unit process. In this paper, the research focus is within a factory. Thus, a three-level structure is introduced as shown in Figure .
Machine tool: that is defined as where operational activities occur. It is the unit of which process chains or production systems are composed.
Process chain: that is a distinctive logical combination of machine tools, which are organized into lines or cells, in parallel or series to execute specific sequences of operations.
Factory: that is a group of process chains known as production system, which is supported by a collection of technical building services (TBSs) such as steam generation unit, compressed air system and heating, ventilating and air conditioning system.
From the unit process point of view, a machine tool consists of several energy consuming components that consume energy dynamically over time depending on process parameters and the actual state of the machine (Thiede, Posselt, and Herrmann Citation2013). According to the literature, the existing models for modelling the energy consumption of the unit process include theoretical exergy models, state-based models and empirical models. To be more specific, exergy models are introduced to theoretically describe energy requirements of various manufacturing processes during production (Gutowski, Dahmus, and Thiriez Citation2006). The results indicated that specific energy consumption (SEC) is not constant, and is a strong function of process rate. However, the relevant coefficients lack clear definition and quantification, so the estimation of total energy consumption is still infeasible. In fact, the energy consumption of a machine tool is dependent on its operational states and process parameters (Devoldere et al. Citation2007). Dietmair and Verl (Citation2009) developed a state-based modelling approach to characterize the energy consumption of a machine tool in relation to the predetermined operational states. However, using average operation time and power can lead to a significant error margin of the total energy consumption, particularly for the case of a multi-product environment or highly dynamic machining processes. Due to these limitations, Kara and Li (Citation2011) presented an empirical approach to model the energy consumption of the unit process. The approach has been proven to be effective for characterizing the relationship between energy consumption and process parameters such as turning, milling, grinding (Li et al. Citation2012) and injection moulding (Li, Kara, and Qureshi Citation2014). The derived models provide machine-specific values for predicting energy consumption. However, the energy consumption due to other operational states (apart from the production state) is suggested to be considered from a system point of view. Furthermore, although energy-efficient design and control of a unit process is necessary for minimizing energy consumption, it is not sufficient if the process is operated inefficiently, for instance, keeping a machine tool at stand-by mode without valuable outcome (Posselt et al. Citation2013).
From a system point of view, the material flow is the key factor with great impact on energy consumption of the whole system as it defines how machine tools interact with each other. Also interactions between process chains and TBSs are of importance (Li et al. Citation2014). TBSs refer to those processes which are responsible to supply necessary resources such as heat and compressed air to support production process chains. In this paper, simulation is the only suitable method to model dynamic material and energy flows in a manufacturing system. This is due to the complexity existing in these systems in terms of large number of variables, dynamic energy consumptions, interactions among processes and so on. In comparison, the objective function regarding the energy and material efficiency is not expressible with mathematical functions. Researchers (Solding and Thollander Citation2006; Solding, Petku, and Mardan Citation2009) presented a simple simulation model to calculate the energy consumption of an iron foundry, considering the constant rate for energy consumption. Consequently, the accuracy of the model is restricted significantly since the dynamic behaviour of a single machine is disregarded. He et al. (Citation2012) have applied the petri net technique and developed an event graph-based simulation approach to characterize the energy consumption according to the task flow of production processes. This model only focuses on the energy consumption during the production state. Similarly, Seow, Rahimifard, and Woolley (Citation2013) have presented a framework based on the embodied product energy concept, to formulate total energy required to produce a unit product. Their work is limited as it considers only the production state of the machine tool and lacks considering other operational states. Herrmann et al. (Citation2011) have applied a holistic view to consider both process chains and TBS. An energy-oriented simulation platform has been developed to predict energy consumption as well as the impact of production parameters (such as lot size). Similar to other approaches, a general state-based model was deployed in which average power values are used to reflect each operational state. Thus, the impacts of process parameters from a unit process perspective are excluded.
Also, there are a number of researchers who consider energy efficiency in production planning problems. Mouzon, Yildirim, and Twomey (Citation2007) studied the effectiveness of using different dispatching rules on machine energy consumption and showed that energy saving is achievable when non-bottleneck processes are turned off after being in idle mode for a certain amount of time. Mouzon and Yildirim (Citation2008) investigate scheduling problems with two objective functions: minimization of total tardiness and energy consumption. These methods are restricted in application as they lack the ability to combine single processes and to form process chains.
As discussed above, manufacturing processes are highly dynamic in terms of energy consumption. Meanwhile, each individual process in a production process chain interacts with others in a complex manner. However, the studies of unit processes cannot cope with the interactions in a process chain, while current system approaches lack the ability of handling the dynamics of individual manufacturing processes. In fact, the most energy-efficient configurations of a process chain can be achieved when both unit process and system level are considered simultaneously. Therefore, the research question in this paper is how to cope with dynamic unit processes and complex interactions among processes within a process chain in order to achieve an energy-efficient manufacturing system.
3. Conceptual framework description
An integrated conceptual framework is developed to answer the above questions. The framework takes into account parameters from both unit process (e.g. material removal rate (MRR)) and system (e.g. lot size) point of view. The relevance of those parameters is dependent on the characteristics of the unit process, as discussed in Section 3.1. Developing the integrated framework follows a bottom-up approach from unit process to a system view and has been discussed in Section 3.2. The selected approach for modelling energy consumption of unit processes is presented in Section 3.3. In order to tackle the interaction within process chains, an energy-oriented simulation model of the manufacturing systems is then introduced in Section 3.4.
3.1 Characteristics of manufacturing processes
A manufacturing factory can be considered as an integration of process chains, TBSs, administrative offices and others. Process chains are the core part of the factory as they are directly related to product manufacture. From the system perspective, production planning and control (PPC) in particular plays a significant role on overall energy efficiency. There are a number of parameters in the PPC such as process layout, production scheduling, lot size and machine selection. Their variations may influence system performance such as production time, inventory level and/or total energy consumption. Apostolos et al. (Citation2013) showed that a great portion of energy is consumed by non-bottleneck machines. The reduction of this inefficiency may be achieved through energy-efficient load management with the combination of better lot sizing and scheduling.
Every process chain consists of a number of production steps in which single process units are configured purposefully to complete production activities. The energy profile of the unit process shows a dynamic trend from two perspectives: operational states and production loads. Each process unit goes through a number of states such as ramp-up, stand-by and production, and each state requires different amounts of energy. This results in a distinctive power consumption profile for each process unit.
For some processes such as curing ovens, the power and duration of being at each operational state does not fluctuate significantly and can be considered constant. In this case, the screening approach is adequate to cope with the dynamics of a unit process in an acceptable level of accuracy. On the contrary, for some processes such as turning or milling, they are highly sensitive to the process parameters. This requires a means to characterize the relationship between energy consumption and process parameters in order to improve the accuracy of the energy efficiency evaluation. Therefore, the empirical models are introduced in conjunction with the screening approach.
3.2 Integrated conceptual framework
Having considered the above-mentioned characteristics, the proposed conceptual framework is illustrated in Figure . Overall, this framework comprises two main modelling layers including unit process and process chain. At the first layer, the unit process model predicts the energy consumption according to specific process parameters and machine characteristics. The required information for the unit process model is supported by a machine library as well as the product and process design. To be specific, the machine library does not only provide the power level and duration of each operational state, but also offers the coefficients for the empirical model to determine the energy consumption at the production state with various production loads. Meanwhile, the production load is defined according to the product design, such as product geometries, surface requirements and so on, which can be further transferred into process-related parameters such as processing volume, MRR and so on. At the second layer, the unit processes are connected on the platform of energy-oriented manufacturing system simulation. As mentioned before, the connection is based on the material flow which is configured by the factory layout and PPC. Also, the material flow triggers the change of operational states for each unit process. In particular, this framework integrates the unit process model into the system simulation to model the dynamic production loads instead of using an average figure as the previous system simulation. Thus, this integration ensures that the dynamics of each unit process were fully considered. As a result, a time-dependent energy profile as well as the traditional production performance indicators (e.g. lead time) can be obtained, which leads to further performance analysis and improvements.
3.3 Unit process model
Under the conceptual framework, the unit process model for predicting energy consumption forms the basic component in the simulation platform to improve the overall energy efficiency of a manufacturing system. There are multiple parameters related to each unit process, which determine the behaviour of machine tools in terms of energy consumption. Various combinations of these parameters produce different energy profiles, as they may change the sequence and/or timing of activated components within a machine tool as well as the production loads on the components. As mentioned before, these combinations need to be analysed in detail to reflect the dynamics of a unit process from two perspectives, namely operational state and production loads. Therefore, a hybrid structure is used here to fulfil the requirements, which combines the state-based model and the empirical model as illustrated in Figure (a).
Moreover, each transition decides either how long the machine tool stays at each operational state or under which circumstances the machine tool can switch from one operational state to another. In this paper, there are six operational states defined as below:
Idle: refers to the state when there is no order or material to be processed and only the necessary components (e.g. control panel) remain activated.
Change over: refers to the state when the machine is setting up for producing a different product, e.g. tool changing.
Ramp up: refers to the relatively short period after switching on the machine tool, or the acceleration of the main motors before the production, normally resulting in a power peak.
Preproduction: refers to the period when the process (e.g. extrusion) needs to be adjusted or to be stabilized until the process output meets the desired quality.
Production: refers to the state when the machine is processing the material and producing desirable products, normally considered as the value-adding period.
Stand-by: refers to the state when the machine remains at operational readiness for production.
Failure: refers to the abnormal state that the machine fails to produce products and requires repairs or maintenance.
For each operational state, the duration and power can be configured with three options: a constant value, a predetermined probability distribution function and a mathematical equation which is based on the existing empirical models of unit processes. The first two options are commonly used in previous simulations, and they are sufficient to configure operational states with relatively constant trends such as stand-by, idle and so on. However, using a constant value for the production state is inadequate for processes featuring dynamic production loads. As a result, empirical models are introduced here to characterize the energy consumption and process parameters. A wide range of manufacturing processes (e.g. turning, milling, grinding and injection moulding) have been studied and can be directly used here. Taking material removal processes as an example, the SEC, the total energy consumption of a machine tool for removing one unit material, shows an inverse relationship with the MRR as shown in Equation (1). The coefficients (C0 and C1) are derived empirically, which is machine specific.
Equation (1) can be converted into Equation (2):
The duration of the production state is dependent on the product and process design. Continuing the case of a turning process, the geometries of a product determine the removal volume (Q). Meanwhile, the process design selects the suitable cutting parameters according to the work piece material, tool type, surface roughness and so on. These cutting parameters can be easily interpreted into MRR as a single compound process parameter. Thus, the duration (t) can be calculated according to Equation (3).
Therefore, the dynamic energy profile of a unit process in terms of operational states and production loads as presented in Figure (a) can be fully simulated in this case, as exemplarily shown in Figure (b).
3.4 Process chain model
In addition to parameters related to unit processes, there are multiple parameters related to process chains and the whole production system which influence the energy profile of the whole system. Parameters such as batch size, the capacity of work in process (WIP) inventories, bottleneck processes or even the process layout can have significant impacts in this regard. The impacts of these parameters require to be investigated in detail to reflect the dynamics of the process chains. The material flow is fundamental to the modelling of manufacturing processes. It determines the timing of the unit processes, the pattern of the energy profile, peak time and total energy consumption. Furthermore, other parameters such as batch size and production schedule are of interest for improving the energy efficiency of the whole system. For example, a larger batch size could reduce the frequency of machine reconfiguration and set-up time, resulting in energy savings. Also the production parameters are relevant to the energy cost from the billing perspective. For instance, the start time of different machines can be scheduled in order to reduce energy peak load and the associated charges. The energy-intensive processes can also be scheduled during the off-peak period to reduce the energy cost (Heilala et al. Citation2008).
Figure illustrates the resource and data flow between the production system, TBSs and PPC centre as well as influencing factors.
The development of process chains is quite straightforward. Having considered the proposed framework, each process chain and the whole production process as a consequence could be established by using the unit process energy model. Each process chain could be created simply by dragging the unit process model for each machine tool. Then these unit processes require to be connected to each other considering the process layout and operational sequences. Eventually, each single unit process needs to be configured separately. It is important to note that the process chain requires to be powered by different types of resources such as compressed air or heat, which mainly are provided by TBSs. These services can be considered as another aspect which is placed on the top of process chains. The behaviour of TBSs is determined with process chains as they intend to meet the demand of process chains. Therefore, in this study, the details of this aspect are excluded from the scope of the paper as it has less effect on process chain energy consumption.
4. Application
Two distinctive case studies have been conducted to show the validity and applicability of the proposed approach on a number of short-term planning problems. The first case features a flexible job-shop including a number of different turning and milling machine tools. It was selected to demonstrate the ability of the proposed approach for a highly dynamic environment. The second case consists of one purpose-built process and one dynamic process with single adjustable process parameters. In contrast to the first case, this mass production system was chosen to test the applicability of the proposed approach in a highly constrained environment. At each case, a set of experiments were designed and the effects of different parameters from both unit process and system perspective were analysed. Objective functions in both cases are energy consumption and total production (completion) time. These two were selected in order to measure the economic and environmental aspects of system performance under different operating conditions. More precisely, total production time and throughput are related to economic aspects while energy consumption aims to characterize the environmental impacts of decisions.
4.1 First case overview
In this case, the simulated job-shop includes five different turning machine tools and four milling machine tools as shown in Figure . One hundred mild steel shafts need to be produced, which require two process steps: external turning and slot milling. All machines in both process steps are interchangeable to produce the same part. The parts are handled manually in between the processes. The case study aims to determine the most energy-efficient combination of turning and milling machines, the effects of process parameters and the role of lot-sizing problems on system performance. In this case, the performance was evaluated based on energy consumption, total production time and productivity. In order to investigate the main effect of a single process/system parameter, the blocking method was selected which varies one parameter and keeps the others constant in a set of experiments.
4.1.1 Selection of the most energy-efficient machine combination
The machines in the job-shop feature different capacities in terms of size, spindle speed, horsepower, number of axes, etc. According to the previous empirical studies, those machines require different amounts of energy to remove a unit volume of material (Kara and Li Citation2011). For the tested case, all the machines are capable of producing the desired parts. Thus, it is of first interest to determine which machine combination is most efficient regarding energy consumption and production time. Correspondingly, all possible combinations of turning and milling machines were simulated and analysed while the cutting parameters were kept identical. The total electricity consumption (in kWh) and production time (in seconds) as objective functions are measured and shown in Figure (a).
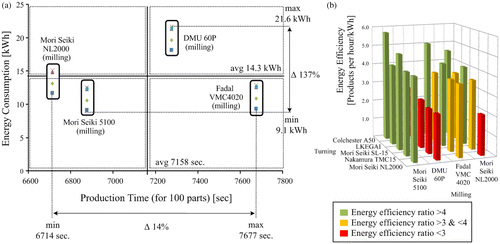
As shown in Figure (a), the total energy consumption of different machine combinations varied in a considerable range from 9.11 to 21.64 kWh. The difference among milling machining centres was mainly due to the base load consumption. For instance, Mori Seiki 5100 demands 1.01 kW at standby mode while DMU 60P requires 5.45 kW. Since DMU 60P is a five-axis machining centre, more components and larger motors are kept active to ensure operational readiness in comparison with other three-axis milling machines (Li et al. Citation2011). The same trend can be also observed among the turning machines as Mori Seiki NL2000 (with two spindles) consumed more energy than Colchester Tornado A50 (with one spindle). In addition, the old machine tools consume more energy, for example IKEIGAI AX20 was manufactured in the 1970s and showed the highest energy consumption for completing the turning processes. Compared to the difference of energy consumption, the total production time varied in a relatively insignificant range from 6714 to 7677 s. The difference was mainly due to the tool travel speed of the milling machining centres, ranging from 1.8 to 3 s per tool path for Mori Seiki NL2000 and Fadal VMC 4020, respectively. In comparison, the variation among turning machines was negligible (from 1.4 to 1.5 s). Considering both objectives (energy consumption and production time) simultaneously, DMU 60P is clearly inefficient and Colchester is the most efficient turning machine for this job. However, the selection of the milling machine is less obvious, because Mori Seiki 5100 consumed the lowest energy consumption but Mori Seiki NL 2000 took the shortest time. Thus, another indicator, energy efficiency, is introduced as the ratio of production rate per hour to total hourly energy consumption as shown in Figure (b).
In Figure (b), the combinations are classified based-on their energy efficiency value into three distinctive categories: greater than 4 product per hour/kWh, less than 3 products per hour/kWh and other values between these two numbers. As can be seen, the best combination is Colchester A50 with Mori Seiki 5100 with the highest energy efficiency about 5.75 products per hour/kWh. Notably, Mori Seiki NL2000 is a versatile machine to perform both turning and milling operations. From the single product point of view, this may lead to a time saving as no transportation and material handling is required between turning and milling. However, from the system point of view, it was more efficient to perform the turning and milling operation separately as the two machines were processing concurrently. As a result, the use of Mori Seiki NL2000 for both tasks leads to the lowest energy efficiency among all machine combinations. In addition to the best machine combination, Figure also provides a ranked list of alternative machine combinations.
4.1.2 Investigation of process parameter effects
This section aims to investigate into the impact of changing process parameters to the overall energy efficiency of the simulated system. According to the previous results, the three most efficient combinations of turning and milling machines were further analysed. The cutting parameters used in the last set of experiments were considered as the standard setting, and then they were modified by increasing or decreasing the cutting speed, feed speed and depth of cut. As a result, the MRR has been varied from 0.25 to 3.75 and 0.15 to 0.90 cm3/s for turning and milling operations, respectively. Figure compares all the cutting scenarios and machine combinations in terms of the energy efficiency and throughput rate.
As Figure suggests, the process parameters at the unit process level have a considerable influence on the overall efficiency of the system. By increasing the MRR, the energy efficiency can be improved more than twice. On the contrary, a slower process can worsen the energy efficiency more than fivefold. It should be noted that the process parameters have also an impact on the product quality (e.g. surface roughness) and WIP inventory, which need to be further studied. Nevertheless, these results provide a general guideline that a fast process rate is favourable from the energy efficiency point of view. In addition, the variance among different machine combinations was more significant when a high MRR was applied. There are two reasons that contribute to this trend. A higher MRR leads to a shorter production time but a higher share of time for non-value-adding activities (e.g. tool path without removing material), resulting in a lower throughput rate for using Fadal VMC 4020. The other reason is that a more powerful machine performs better when higher load is applied, so Mori Seiki 5100 has the highest energy efficiency compared to others. In comparison, all the tested machines are equally inefficient when a shallow cut is performed. In conclusion, a slow MRR should be avoided if possible during the product and process design stage.
4.1.3 Investigation of lot-sizing effects
As mentioned before, production system-related parameters also have an influence on the energy and production performance indicators at the system level. It is common that the processing time is different at each process step. In this case, the lot size is an important parameter to synchronize the processes or to reduce the idle time. Hence, this section deals with the effects of changing the lot size on the energy efficiency of the overall system. To do that, the turning process was assumed to be a slow process with a low MRR while the milling process remained the standard configuration. The most efficient machine combination (Colchester A50 and Mori Seiki 5100) was tested by increasing the lot size from 1 to 50 with an interval of 5. The machine tool was assumed to be switched off after 60 s idling. Figure presents the simulation result in terms of total production time, energy consumption and energy efficiency compared with the lot size 1.
Figure suggests that the total production time increased when bigger lot sizes were selected. This linear trend was because the milling machine started to operate only after the turning machine finished the first lot. Compared to lot size 1, the production time was increased by more than 17% when lot size was 50. On the contrary, the energy consumption decreased when increasing the lot size. The energy reduction was due to the milling machine remaining switched-off during the first lot production at the turning process. The energy saving became insignificant when the lot size was greater than 15, since the lot size was large enough to allow the milling machine to be switched off in between two lots. It should be noted that the larger lot size could also reduce the number of machine switch on/off and power peak during the ramp-up stage. Overall, the energy consumption can be reduced by 9% when increasing the lot size. Since the energy efficiency considers both energy consumption and production time, it suggests the best lot size of five for the simulated case.
4.2 Second case overview
The second case was chosen from a pharmaceutical company in Australia. The company has strategic targets for substantially reducing energy and resource consumption as well as reducing its carbon footprint. To achieve that goal, the company has invested on improving the transparency of its energy flows by developing energy consumption models of their processes. In this case study, an energy-intensive department was selected which produces plastic bags for medication delivery, including two different processes: extrusion and plastic welding.
In extrusion processes, PVC as raw material is fed from a top mounted hopper into the barrel of the extruder into contact with the screw. Then, the rotating screw forces raw material into the barrel which is heated to the desired melt temperature of the molten plastic. There is a flat die at the end of the screw which forms melted plastic into sheet. Then the sheets are pulled and stored in 250-m rolls at the end. The Kiefel centre is a purpose-built plastic welding machine for bag production. There are two Kiefel units within the centre simply separated as Kiefel 1 and Kiefel 2. These units use rolls of plastic sheets from extrusion and apply high frequency welding to join plastic sheets. A capacitor field with a high-frequency supply point is used to provide the necessary welding temperature. Electricity is the only energy carrier used by these two processes for production. The schematic presentation of these processes is shown in Figure (a).
4.2.1 Experiment details
In extrusion processes, throughput is measured as the mass of produced plastic sheets per time unit. It changes according to the screw RPM. In addition, the RPM is important as electricity consumption of the process during extrusion time depends on the selected RPM (Li, Kara, and Kornfeld Citation2013). Therefore, the study aimed to investigate the impact of changing RPM on the system performance and to determine the best value of RPM. A hybrid unit energy consumption model was developed for the extrusion process. The average value derived from empirical measurements was used to depict the power demand at operational states such as idle, stand-by, preproduction and so on. According to the previous studies by Li, Kara, and Kornfeld (Citation2013), the empirical model was used to configure the power demand at the production state as shown in Equation (4). In this case, the extrusion process produced one type of roll which was approximately 62 kg per roll. The previous empirical study also suggests the relationship between RPM and throughput rate, as shown in Equation (5). Hence, the production time for each roll can be calculated with Equation (6). Since the RPM can be varied from 40 to 100, the experiments tested 13 different screw RPM settings with an interval of 5.
Since the Kiefel machines are purpose-built and almost all the parameters are kept constant, the state-base unit process models were configured according to the measured energy profile. Based on the tested weekly schedule of the department, the required number of products from each Kiefel machine was extracted and shown in Figure (b). Two small size bags (A and B) are produced by Kiefel 1, while Kiefel 2 is assigned for producing three large size bags (C, D and E). Considering the bag size, each roll can make different number of bags, resulting in different processing times per roll. From the system point of view, production planning plays a significant role on the energy efficiency of a factory (Pechmann, Scholer, and Hackmann Citation2012). The load management of the line can offer great savings from an energy point of view. The reduction of the idle time through energy-efficient process planning with the combination of better batch and orders organization may lead to a better prediction of the workflow. Therefore, the scheduling problem (orders sequencing) was tested with a total combination of 12 (2! × 3! = 2 × 6 = 12), as listed in Figure (c).
4.2.2. Experiment and discussion
The developed simulation model has run with a random selection of RPM and schedule. All possible combinations were tested at least five times. For each run, the total amount of consumed electricity and the completion time for all products were measured as objective functions. Figure (a),(b) presents the average results of each tested combination of RPM and schedule.
Figure (a) suggests a general trend that the higher the RPM the shorter the completion time. For each scheduling scenario, the shortest completion time occurred when RPM researched 100. According to Equation (6), the maximum RPM resulted in the shortest processing time for the extrusion process, so the first roll could be delivered to Kiefel machines in the quickest manner. When the RPM was low, the scheduling has no impact on the total completion time, as the extrusion line was the bottleneck and pacemaker process. Once the RPM was greater than 80, there was a significant difference among the tested schedules. For example, when the RPM was 100, the extrusion process delivered one roll in every 12.39 min based on Equation (6). Having considered that the rolls were distributed equally between Kiefel machines, each Kiefel machine waited 2 × 12.39 = 24.78 min to receive the next roll. Schedules 2 and 3 prioritized the production of products C and D in Kiefel 2, respectively. According to Figure (b), Kiefel 2 spent 36.391 min to consume one roll to produce product C and spent 17.628 min for product D. Thus, schedule 2 allowed Kiefel 2 to build up the buffer, while schedule 3 starved the process and wasted time on waiting for the next roll unit finishing the production of product D.
Figure (b) shows a similar trend for the electricity consumption. The minimal electricity consumption occurred when the RPM was 90 and schedule 7 was chosen. Increasing the RPM from 40 to 90 reduced the processing time in the extrusion process. As a result, the Kiefel machine started operation sooner with less amount of time being in stand-by state. When the RPM was 90, the utilization of Kiefel machines was maximized with minimum time being wasted on waiting for rolls from the extrusion process. However, further increasing the RPM over 90 could increase the stand-by time and energy consumption of Kiefel machines with certain schedules as explained before. Thus, both completion time and energy consumption were taken into account to determine the best combination of the RPM and schedule, as shown in Figure (c). The energy efficiency, the ratio between production rate per hour and total hourly electricity consumption, suggested that the best combination was schedule 7 with RPM at 95.
5. Summary and outlook
This research developed an integrated conceptual framework to model energy consumption of a production system, and demonstrated the ability to obtain practical comprehension about potential energy efficiency improvements through experimentations. A hybrid model structure has been structured to project energy consumption of unit processes, which combines the advantages of both state-base models and empirical models. A flexible platform was constructed to accommodate different options for configuring each operation state, including constant values, predetermined probability distribution functions and mathematical equations which are based on the existing empirical models. Then the whole production system can be further developed by linking the unit process models, and controlled by the PPC. Owing to the flexibility of simulation, the developed framework enables to study a number of operational planning problems. These problems usually belong to a short-term planning horizon and mainly include scheduling, product routing and lot sizing.
Two distinct industrial cases are presented to demonstrate the potential of the proposed framework. The studies investigated into the energy efficiency of the system through machine selection, process parameter definition and PPC. As the results suggested, all these elements strongly interact with each other. In particular, the unit process parameters have significant influence on the system performance. Thus it is beneficial to combine the unit process model and system simulation together. The actual performance of the system as a whole depends on the specific case while considering machine characteristics, process and production parameters. The performance and ease of implementation suggests its applicability to more complex manufacturing environments, enabling management to make decisions timely and strategically.
Due to the scope of this paper, the interaction between unit process and TBS systems needs to be further studied. Other energy carriers, such as gas, compressed air or steam, should also be investigated in an integrated manner. Considering the enormous combinations of parameters in a manufacturing system, it is beneficial to apply optimization techniques in conjunction with energy-oriented simulation models as recommended for future research.
Disclosure statement
No potential conflict of interest was reported by the authors.
Notes
1. Email: [email protected]
2. Email: [email protected]
3. Email: [email protected]
4. Email: [email protected]
References
- Apostolos, Fysikopoulos, PapacharalampopoulosAlexios, PastrasGeorgios, StavropoulosPanagiotis, and ChryssolourisGeorge. 2013. “Energy Efficiency of Manufacturing Processes: A Critical Review.” Procedia CIRP7: 628–633. 10.1016/j.procir.2013.06.044.
- Devoldere, Tom, WimDewulf, WimDeprez, BarbaraWillems, and Joost R.Duflou. 2007. “Improvement Potential for Energy Consumption in Discrete Part Production Machines.” In Advances in Life Cycle Engineering for Sustainable Manufacturing Businesses, edited by ShozoTakata and YasushiUmeda, 311–316. London: Springer.
- Dietmair, A., and A.Verl. 2009. “A Generic Energy Consumption Model for Decision Making and Energy Efficiency Optimisation in Manufacturing.” International Journal of Sustainable Engineering2 (2): 123–133.
- Duflou, Joost R., John W.Sutherland, DavidDornfeld, ChristophHerrmann, JackJeswiet, SamiKara, MichaelHauschild, and KarelKellens. 2012. “Towards Energy and Resource Efficient Manufacturing: A Processes and Systems Approach.” CIRP Annals – Manufacturing Technology61 (2): 587–609. 10.1016/j.cirp.2012.05.002.
- Gutowski, Timothy, Jeffrey Dahmus, and Alex Thiriez. 2006. “Electrical Energy Requirements for Manufacturing Processes.” Paper presented at the 13th CIRP International Conference on Life Cycle Engineering.
- He, Yan, BoLiu, XiaodongZhang, HuaiGao, and XuehuiLiu. 2012. “A Modeling Method of Task-Oriented Energy Consumption for Machining Manufacturing System.” Journal of Cleaner Production23 (1): 167–174. 10.1016/j.jclepro.2011.10.033.
- Heilala, J., S. Vatanen, H. Tonteri, J. Montonen, S. Lind, B. Johansson, and J. Stahre. 2008. “Simulation-based Sustainable Manufacturing System Design.” Paper presented at the Proceeding of the 2008 Winter Simulation Conference, December 7–10.
- Herrmann, C., S.Thiede, S.Kara, and J.Hesselbach. 2011. “Energy Oriented Simulation of Manufacturing Systems – Concept and Application.” CIRP Annals – Manufacturing Technology60 (1): 45–48. 10.1016/j.cirp.2011.03.127.
- IEA. 2012. “Key World Energy Statistics.” Paris: IEA.
- Kara, S., and W.Li. 2011. “Unit Process Energy Consumption Models for Material Removal Processes.” CIRP Annals – Manufacturing Technology60 (1): 37–40. 10.1016/j.cirp.2011.03.018.
- Li, Yufeng, YanHe, YanWang, PingYan, and XuehuiLiu. 2014. “A Framework for Characterising Energy Consumption of Machining Manufacturing Systems.” International Journal of Production Research52 (2): 314–325. 10.1080/00207543.2013.813983.
- Li, Wen, SamiKara, and BernardKornfeld. 2013. “Developing Unit Process Models for Predicting Energy Consumption in Industry: A Case of Extrusion Line.” In Re-engineering Manufacturing for Sustainability, edited by Andrew Y. C.Nee, BinSong, and Soh-KhimOng, 147–152. Singapore: Springer.
- Li, W., S. Kara, and F. Qureshi. 2014. “Characterising Energy and Eco-efficiency of Injection Moulding Processes.” International Journal of Sustainable Engineering. https://doi.org/doi:10.1080/19397038.2014.895067.
- Li, W., M.Winter, S.Kara, and C.Herrmann. 2012. “Eco-efficiency of Manufacturing Processes: A Grinding Case.” CIRP Annals – Manufacturing Technology61 (1): 59–62.
- Li, Wen, AndréZein, SamiKara, and ChristophHerrmann. 2011. “An Investigation into Fixed Energy Consumption of Machine Tools.” In Glocalized Solutions for Sustainability in Manufacturing, edited by JürgenHesselbach and ChristophHerrmann, 268–273. Berlin: Springer.
- Mouzon, Gilles, and Mehmet B.Yildirim. 2008. “A Framework to Minimise Total Energy Consumption and Total Tardiness on a Single Machine.” International Journal of Sustainable Engineering1 (2): 105–116. 10.1080/19397030802257236.
- Mouzon, Gilles, Mehmet B.Yildirim, and JanetTwomey. 2007. “Operational Methods for Minimization of Energy Consumption of Manufacturing Equipment.” International Journal of Production Research45 (18–19): 4247–4271. 10.1080/00207540701450013.
- Pechmann, Agnes, Ilka Scholer, and Rene Hackmann. 2012. “Energy Efficient and Intelligent Production Scheduling – Evaluation of a New Production Planning and Scheduling Software.” Paper presented at the 19th CIRP International Conferencwe on Life Cycle Engineering.
- Posselt, G., K. Kellens, S. Thiede, Renaldi, C. Hermann, W. dewulf, and J. duflou. 2013. “Combining Machine Tool Builder and Operator Perspective towards Energy and Resource Efficiency in Manufacturing.” 20th CRIP International Conference on Life Cycle Engineering, Singapore.
- Seow, Yingying, ShahinRahimifard, and ElliotWoolley. 2013. “Simulation of Energy Consumption in the Manufacture of a Product.” International Journal of Computer Integrated Manufacturing1–18. 10.1080/0951192x.2012.749533.
- Solding, P., D.Petku, and N.Mardan. 2009. “Using simulation for More Sustainable Production Systems – Methodologies and Case Studies.” International Journal of Sustainable Engineering2 (2): 111–122.
- Solding, P., and P. Thollander. 2006. “Increased Energy Efficiency in a Swedish Iron Foundry Through Use of Discrete Event Simulation.” Paper presented at the 2006 Winter Simulation Conference.
- Thiede, S., G.Posselt, and C.Herrmann. 2013. “SME Appropriate Concept for Continuously Improving the Energy and Resource Efficiency in Manufacturing Companies.” CIRP Journal of Manufacturing Science and Technology6 (3): 204–211. 10.1016/j.cirpj.2013.02.006.
- Weinert, N., S.Chiotellis, and G.Seliger. 2011. “Methodology for planning and operating energy-efficient production systems.” CIRP Annals – Manufacturing Technology60 (1): 41–44.