Abstract
A novel decision support framework has been proposed herein to solve supplier selection problems by considering green as well as resiliency criteria, simultaneously. An expert team involving a finite number of Decision-Makers (DMs) is to be employed to obtain degree of importance of each of the evaluation criteria and also to assign an appropriate rating for each supplier alternative with respect to the criteria. Since subjective human judgment often bears some sort of ambiguity as well as vagueness, application of fuzzy set theory can be recommended in this context. The present work represents a dominance based decision support system in the light of fuzzy set theory which is basically a simplified version of TODIM and PROMETHEE. It explores dominance between two alternatives with respect to a particular criterion, based on which a global dominance measure is computed to facilitate ranking order of candidate suppliers. The approach proposed in this research seems straightforward which can exclude complex and tedious computational steps of TODIM as well as PROMETHEE. Application potential of the proposed dominance based fuzzy decision-making approach has been compared to that of Fuzzy-TOPSIS and Fuzzy-VIKOR. Good agreement has been observed. In addition to this, the concept of a unique performance index i.e. ‘g-resilient’ (also called ‘ecosilient’) index has been introduced to help in assessing suppliers’ overall performance extent. The work has also been extended to identify the ill (poor)-performing areas in which suppliers are lagging and seek further improvement towards enhancing g-resilient performance level.
1. Introduction
Managing the movement of goods or products from one point to another subjected to certain constraints is well acknowledged as Supply Chain Management (SCM). In a broader sense, ensuring the synchronisation between various network activities from the beginning to the destination is referred to as SCM. In traditional supply chain activities, huge industrial wastes resulted in high level of environmental pollution. In order to save environment and also the Earth, green concepts were introduced; traditional supply chain was reoriented as Green Supply Chain Management (GSCM). The primary motivation for consideration of GSCM is to diminish environmental deterioration throughout the product life cycle. GSCM intends to eliminate various industrial wastes including hazardous chemical, emissions, energy and solid waste along every network activities such as product design, material resourcing and selection, manufacturing process, delivery of final product and end-of-life management of the product (Chin, Tat, and Sulaiman Citation2015; Rao Citation2006; Srivastava Citation2007).
Supply chain performance can be enhanced by adopting green practices which in turn result in better cost saving and profitability. Adding the ‘green’ practices to SCM involves addressing the influence and relationships between SCM and natural environment (Srivastava Citation2007). It is well understood that a firm cannot survive in long run without supplier’s contribution as they are the dealer who supply necessary goods and services that the firm can’t self-produce (Kuo, Wang, and Tien Citation2010). Selection and management of appropriate supplier is the key success factor to acquire desired level of quality products at the reasonable price with on-time delivery. Thus, to support GSCM, supplier selection should emphasise on supplier’s ability to adopt green concepts like green image, green competencies, green packaging, environmental management and capability of preventing pollution. However, (Zhu, Sarkis, and Lai Citation2008) proved that the green paradigm is concerned with environmental risks and environmental impact reduction only and does not consider the effects of disturbances on the system. Afterward to handle the disturbances on the system (Christopher and Peck Citation2004) introduced the concept of resilient supply chain and highlighted resilient paradigm which focuses on the supply chain ability to recover to the desired state after a disruption occurs. Disruption is a Low Probability High Intensity Event which may cause system unbalance (turbulence) for a long term. Therefore, preparation for sustaining in disruption situations should also be considered as a critical strategic issue in the supplier selection process. Thus, proactive arrangement for these sorts of happenings should be a priority for supply chain managers (Haldar et al. Citation2014). Resiliency is an adaptive control term, where firms prepare themselves to cope up with any unexpected event or demand by assuring the continuity of the operation at the best possible rate. It is also described as the capacity of a system to attain its original state after disruption is incurred. According to (Fiksel Citation2006), resiliency refers to a firm’s capacity to survive, adapt and grow in the face of change and uncertainty. Tierney and Bruneau (Citation2007) explored the concept of a resilience triangle (shown in Figure ) that emerged from the disaster research and characterised the loss of functionality from damage and risk.
Figure 1. Resilience triangle as described by (Tierney and Bruneau Citation2007).
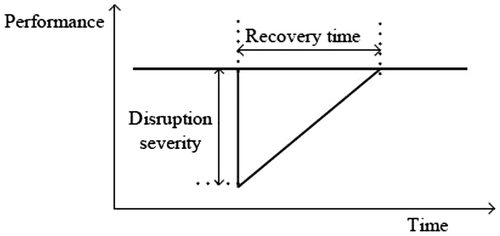
The depth of the triangle represents the disruption severity; more specifically it is the severity or magnitude of loss or damage. The length of the triangle shows the recovery time that is the time taken for the restoration; it is also known as the damping time. Reduced size of triangle shows a strong resiliency in the company’s supply chain. Therefore, the resilience triangle should be minimised at the best possible way. Actions, Behaviour and properties of companies and networks can contribute to reducing the area of the resilience triangle. Haimes (Citation2006) reported that resilience approaches are having two broad intentions (i) to provide a recovery tool for the system that has been previously disturbed, within an acceptable time range and at a standard cost and (ii) to provide control for the disturbances on the system by reducing the adverse effect which will cause a possible interruption.
It is evident from the literature that resiliency provides a strong recovery tool and a better control on disruption, whereas adaptation to green practices offers reduced waste thereby protecting the environment. A firm’s supplier selection should take care of suppliers’ resiliency strategy along with green consciousness.
2. Sate of art and problem definition
Handfield et al. (Citation2002) demonstrated the use of Analytic Hierarchy Process (AHP) to evaluate relative importance of various environmental traits and to assess the relative performance of several suppliers along these traits. The authors examined how AHP could be incorporated into a comprehensive information system supporting Environmentally Conscious Purchasing. (Humphreys, McIvor, and Chan Citation2003) outlined the development of a knowledge-based system which could integrate environmental factors into the supplier selection process. The system employed both case-based reasoning and decision support components including multi-attribute analysis. (Lu, Wu, and Kuo Citation2007) presented a methodology to evaluate the effectiveness of projects supplying GSC concept. Specifically, a multi-objective decision-making (MODM) process for GSC management was presented to help the supply chain manager in measuring and evaluating suppliers’ performance based on AHP. In order to reduce subjective bias in designing a weighting system, a fuzzy logic process was used to modify the AHP. Kannan et al. (Citation2008) analysed the interaction of criteria used to select green suppliers addressing environmental performance using Interpretive Structural Modelling (ISM) and AHP. The effectiveness of the ISM and AHP model was illustrated using an automobile company in the southern part of India. Lee et al. (Citation2009) proposed a model for evaluating green suppliers for high-tech industry. The Delphi method was applied first to differentiate the criteria for evaluating traditional suppliers and green suppliers. A hierarchy was constructed next to evaluate the importance of the selected criteria and the performance of green suppliers. In order to consider vagueness of experts’ opinions, the fuzzy extended AHP was exploited in this work.
Awasthi, Chauhan, and Goyal (Citation2010) applied Fuzzy-TOPSIS for evaluating environmental performance of suppliers. Shaik and Abdul-Kader (Citation2011) presented a generic framework integrating environmental and social criteria leading to a comprehensive selection process of green suppliers. To cater to the multi-criteria decision-making (MCDM) approach with both quantitative and qualitative attributes, the authors applied multiple attribute utility theory that could help managers formulating viable sourcing strategies. Çifçi and Büyüközkan (Citation2011) proposed a green supplier evaluation model and presented a decision support framework based on group decision-making (GDM) and fuzzy analytic hierarchy process (FAHP) for evaluating and selecting green suppliers. Datta et al. (Citation2012) reported a methodology for the evaluation of suppliers’ environmental performances (green supplier selection) in order to evaluate and select the best candidate supplier. An interval-valued fuzzy set theory coupled with VIKOR method was utilised in this work. Büyüközkan and Çifçi (Citation2012) examined the components and elements of GSCM and suggested a GSCM evaluation framework. The authors also provided a real case study of Ford Otosan, in Turkey. Moreover, to cope with ambiguity and vagueness of the decision-maker’s evaluations, the fuzzy extension of the ANP method was recommended in this paper. Büyüközkan (Citation2012) proposed a decision model for supplier performance evaluation by considering various environmental performance criteria. A FAHP was applied to determine the relative weights of the evaluation criteria and an axiomatic design (AD)-based fuzzy GDM approach was applied to rank candidate green suppliers. Bakeshlou et al. (Citation2014) developed a multi-objective fuzzy linear programming model for a GSS problem, including 17 criteria, formed into 5 clusters; while a hybrid fuzzy MODM was employed to solve it. The authors aimed to select the best set of suppliers regarding optimal allocation of order quantities while demand and supplier’s capacity were restricted. In the proposed hybrid solution algorithm, fuzzy decision-making trial and evaluation laboratory approach was used to understand the interrelation among criteria, and fuzzy analytical network process provided the criteria weights with respect to their dependencies. Then, a hybrid of fuzzy ANP and fuzzy multi-objective linear programming presented optimal order allocation among the selected suppliers. Dobos and Vörösmarty (Citation2014) examined the extension of the vendor evaluation methods with environmental, green issues. In this paper, the method composite indicator (CI) was used to study the extension of traditional supplier selection methods in consideration with environmental factors. The authors divided the criteria in two categories: the traditional (managerial) and environmental (green) factors. Then with the help of CI, the authors searched a weight system with which the environmental criteria could influence the decision with a representation of the green factors. In order to choose the aforementioned weight system, Data Envelopment Analysis (DEA) was applied with the common weights analysis method. Banaeian et al. (Citation2015) formulated an integrated framework for deciding about the green supplier selection criteria in food supply chain. Fuzzy based Grey Relational Analysis (GRA) was proposed towards ranking of suppliers in presence of qualitative and imprecise data. Also linear programming was used to present a model which could select the best set of suppliers and allocate the orders among them optimally. Freeman and Chen (Citation2015) focused on development of a green supplier selection model using an index system based on a combination of traditional supplier and environmental supplier selection criteria for the case company – a Chinese-based electronic machinery manufacturer. The decision model explored AHP and the Technique for Order Preference by Similarity to Ideal Solution (TOPSIS). (Hashemi, Karimi, and Tavana Citation2015) considered both economic and environmental criteria and thereby proposed a comprehensive green supplier selection model. The analytic network process was used to deal with the interdependencies among the criteria, and the modified GRA was applied to better address the uncertainties inherent in supplier selection decisions.
Aforesaid section represents outlines of past research on green supplier selection. The following section highlights few researches carried out so far on various issues of resilient supplier selection.
Haldar et al. (Citation2012) developed a quantitative approach for supplier selection under a disaster environment. In the four tier process presented herein, the supplier’s weights were initially determined using TOPSIS and AHP methodology for general selection criteria. A cut-off value for the supplier weight was assigned and the suppliers which were above this cut-off value were selected for the primary selection process. Using AHP-QFD methodology the manufacturer’s critical criteria and resiliency criteria were integrated into the selection process to determine the subjective factor measures for each of the primarily selected suppliers. Different cost factors were unified using a normalising technique to determine the objective factor measure for each of the suppliers. Finally, a supplier selection index was calculated in which the decision-maker’s attitude played an important role. In another report, (Haldar et al. Citation2014) provided an approach for strategic supplier selection, under a fuzzy environment, in a disaster scenario. This paper presented an integrated fuzzy GDM approach based on a fuzzy technique for TOPSIS to rank the suppliers of a manufacturing system. Suppliers’ weights for a general strategy and a resilient strategy were combined in course of sensitivity analysis, where decision-makers’ attitude played an important role. Using this approach, organisations could devise resiliency plans to alleviate the vulnerability of a supply chain system. (Chen, Hsieh, and Wee, Citation2016) sought to verify the criteria for selecting suppliers using global performance measurements to identify optimal supply resources and locations in an uncertain disaster environment. The study focused on an automotive company case study and evaluated the results through weighted goal programming and pre-emptive goal programming methods.
Owing to the increased necessity of green and resilient supply chain philosophies in recent times, the efficient supplier selection to support g-resilient (ecosilient) SCM appears to be a challenging research agenda in supply chain literature. Apart from considering traditional supplier selection criteria (cost, quality, delivery and service), green and resiliency criteria need to be assessed simultaneously for evaluating suppliers’ performance. Supplier selection was attempted in past literature considering green as well as resiliency criteria, separately. Rare attempt was made in integrating and analysing green and resiliency criteria both, to select a potential supplier.
Literature is very limited in applying integrated decision support tools on the deployment of green and resilient strategies simultaneously, particularly for the supplier selection problem. Variety of decision-making tools and techniques have been highlighted in past literature. The following section reviews the exiting studies about supplier selection methods that have been recently published.
Deng et al. (Citation2014) applied an AHP methodology extended by D numbers (D-AHP) for supplier selection problem. (Rezaei, Fahim, and Tavasszy Citation2014) conducted a case research of supplier selection in the airline retail industry using a funnel methodology. The supplier selection problem was solved by means of a two-phased methodology. In the first phase, a conjunctive screening method was used, which aimed to reduce the initial set of potential suppliers prior to the comprehensive final choice phase. In the second phase, a fuzzy AHP was used, in which suppliers were evaluated against the main criteria and sub-criteria. Rajesh and Ravi (Citation2015) considered the electronic supply chain as a case study; grey possibility values for supplier selection were calculated and the suppliers were prioritised. (Hashemi, Karimi, and Tavana Citation2015) developed an integrated green supplier selection approach with analytic network process and improved GRA. You et al. (Citation2015) extended the VIKOR method with interval 2-tuple linguistic information for group multi-criteria supplier selection problem. (Memon, Lee, and Mari Citation2015) developed an efficient approach by combining grey systems theory and uncertainty theory for group multi-criteria supplier selection. The proposed supplier selection method not only selected the most appropriate supplier(s) but also allocated optimal purchase quantity under stochastic and re-cognitive uncertainties. (Igoulalene, Benyoucef, and Tiwari Citation2015) explored fuzzy hybrid multi-criteria GDM approaches in order to choose the best strategic supplier. For each approach, the correlation coefficient and standard deviation-based objective weight determination model was used to compute the criteria weights. Chai and Ngai (Citation2015) conducted a multi-perspective strategic supplier selection research in uncertain environments and proposed a soft decision model involving multiple stakeholders and multiple perspectives. Arikan (Citation2015) suggested an interactive solution method for multiple objective supplier selection problems to work under fuzzy parameters and considered a multiple sourcing supplier selection problem as a multiple objective linear programming problem with fuzzy demand level and/or fuzzy aspiration levels of objectives. (Kanagaraj, Ponnambalam, and Jawahar Citation2016) founded a reliability-based total cost of ownership methodology for supplier selection using cuckoo-inspired hybrid algorithm. Awasthi and Kannan (Citation2016) recommended a Nominal Group Technique (NGT) and VIKOR method to work under fuzzy environment for green supplier development program, where NGT was used to identify criteria for evaluating green supplier development programs. Fuzzy theory was used to address qualitative (linguistic) ratings for the alternatives and the selected criteria used under lack of quantitative information. VIKOR was used to generate green supplier development program rankings and recommend the best program(s) for implementation. Fallahpour et al. (Citation2016) introduced an improved DEA–AI (data envelopment analysis–artificial intelligence) model for green supplier selection under fuzzy environment. Ulutas et al. (Citation2016) developed a utility-driven approach to supplier evaluation and selection; the authors conducted an empirical validation of an integrated solution framework by considering Turkish Textile Company as a case study. Wood (Citation2016) identified a set of 30 criteria for assessing supplier selection for facilities and field development projects across a case petroleum industry. The authors considered fuzzy and intuitionistic Fuzzy-TOPSIS with flexible entropy weighting for effective supplier selection in order to fulfill the petroleum industry facilities.
Supplier selection process may include quantitative/qualitative information (or combination of both); to handle the situation, past researcher developed the numerous decision-making tools and techniques seemed helpful to provide realistic solutions. Quantitative information or criteria can be evaluated by applying traditional MCDM methodologies, whereas qualitative criteria information was analysed in fuzzy/grey environment. In this context, a novel decision support framework has been delineated herein to facilitate g-resilient (ecosilient) supplier selection in fuzzy environment. The concept of dominance (between two alternatives with respect to a particular criteria) adapted from TODIM and PROMETHEE has been articulated in the proposed approach. However, compared to TODIM and PROMETHEE, attempt has been made to eliminate/exclude complex computational steps of TODIM and PROMETHEE.
The application potential of the proposed decision support module has been compared to that of Fuzzy-TOPSIS as well as Fuzzy-VIKOR. A unique g-resilient (ecosilient) index has been computed for individual supplier alternatives, based on which suppliers have been ranked and the best supplier has been selected. In addition to this, work has been extended to identify ill (poor)-performing areas in which suppliers should pay further attention to boost up their g-resilient performance up to the desired level.
The objectives of the current research have been highlighted below.
(1) | To propose a systematic and logical decision support framework to facilitate g-resilient supplier selection. The proposed framework is basically a simplified version of TODIM and PROMETHEE. The novelty of this approach is to eliminate complex procedural steps of TODIM as well as PROMETHEE. | ||||
(2) | To cope up with ill-defined and vague evaluation criteria in regard to green as well as resiliency performance, the proposed decision support framework has been formulated to work under fuzzy environment. | ||||
(3) | To validate the ranking order obtained herewith by comparing the same with that of Fuzzy-TOPSIS as well as Fuzzy-VIKOR. | ||||
(4) | To evaluate a unique performance index called ‘g-resilient’/ ‘ecosilient’ index for individual supplier alternatives; and thereby obtain the preference ranking order. | ||||
(5) | To identify ill (poor)-performing areas of individual supplier alternatives which are required to be improved in future to boost up supplier’s overall g-resilient performance extent. |
3. Preliminaries of fuzzy set theory: trapezoidal and triangular fuzzy numbers
Fuzzy logic carries a general name of ‘fuzzy set analysis’ and ‘possibility theory’, which can deal with uncertainty, vagueness and impression of subjective judgment; and is an effective tool for the application where no sharp boundaries (problem definitions) are possible (Markowski, Mannan, and Bigoszewska Citation2009). In classical set theory, a specific object is either a member or non-member of the set. However, in real life situations, due to lack of knowledge or existence of imprecise data, it is not always obvious to say whether an object belongs to a set or not. Therefore, fuzzy sets deal with an uncertainty in an approximate way. Conceptually, fuzzy set theory permits an object belonging to multiple exclusive sets in the cognitive context. Each set incorporates a degree of truth that an object belongs to a fuzzy set. Fuzzy set theory is being enormously used in industrial engineering, decision and information sciences. In this section, we review some basic notions of fuzzy sets as follows:
Definition 1: A fuzzy set in a universe of discourse X is characterised by a membership function
is termed the grade of membership of x in
Definition 2: Chen (Citation1985) proposed the concept of generalised fuzzy numbers. Let be a generalised trapezoidal fuzzy number (TrFN),
as shown in Figure , where a1, a2, a3, a4 are real values,
denotes the height of the generalised TrFN
and
If
then
becomes a TrFN and can be represented as
If a2 = a3, then
is a triangular fuzzy number. If a1 = a2 = a3 = a4, then
is a crisp value.
A TrFN denoted by is defined as
where the membership function is represented as:
(1)
In the following, we briefly review some arithmetic operations between fuzzy numbers (Chen Citation1985; Chen and Chen Citation2007; Hwang and Lin Citation1987; Kaufmann and Gupta Citation1991).
Assume that and
are two generalised TrFNs, where
and
a1, a2, a3, a4, b1, b2, b3, b4 are real values,
and
Some arithmetic operations between the generalised fuzzy numbers
and
are shown as follows:
(1) | Generalised fuzzy numbers addition ⊕: |
(2)
(2) | Generalised fuzzy numbers multiplication ⊗: |
(3)
(3) | Generalised fuzzy numbers subtraction |
(4)
(4) | Generalised fuzzy numbers division Ø: |
(5)
where b1 ≠ 0, b2 ≠ 0, b3 ≠ 0, b4 ≠ 0.
Definition 3: A linguistic variable is a variable whose values are expressed in linguistic terms. The concept of a linguistic variable is very useful in dealing with situations, which are too complex or not well defined to be reasonably described in conventional quantitative expressions (Zimmermann Citation1991). For example, ‘weight’ is a linguistic variable whose values are very low, low, medium, high, very high, etc. Fuzzy numbers can also represent these linguistic values.
Definition 4: If and
be two TrFNs. Then the distance between them can be calculated through using vertex method as (Chen Citation2000):
(6)
The vertex method is an effective and simple method to calculate the distance between two trapezoidal or triangular fuzzy numbers. According to the vertex method, two TrFNs and
are identical if and only if,
Definition 5: (Chen, Hsiao, and Horng Citation1997) presented a method for defuzzifing fuzzy numbers. Assume that is a TrFN,
then the defuzzified value
of the fuzzy number
is calculated as follows:
(7)
Definition 6: A triangular fuzzy number (Figure ) is defined by
with membership function given by:
(8)
A triangular fuzzy number in which a1, a2, a3 are real values,
denotes the height of the generalised triangular fuzzy number
and
If
then
becomes a triangular fuzzy number and can be represented as
Definition 7: Let a triangular fuzzy number then the defuzzified value
is calculated by:
(9)
Definition 8: Let two triangular fuzzy numbers and
, then the operation with these fuzzy numbers are defined as follows:
(1) | Addition of fuzzy numbers (+) |
(10)
(2) | Subtraction of fuzzy numbers (−) |
(11)
(3) | Multiplication of fuzzy numbers ⊗ |
(12)
(4) | Division of fuzzy numbers |
(13)
(5) | Multiplication by a scalar number k |
(14)
Definition 9: Let two triangular fuzzy numbers and
, then the distance between them is computed as (vertex method):
(15)
Also,(16)
Definition 10: A triangular fuzzy numbers can also be converted into TrFN like
≅
Hence, all formulations of TrFNs mathematics; can be applicable for triangular fuzzy numbers also.
4. Proposed decision support framework
The decision support system proposed herein is the simplified version of TODIM, and PROMETHEE. TODIM (an acronym in Portuguese of Interactive and Multi-criteria Decision Making) method makes use of a global measurement of value calculable by the application of the paradigm of Prospect Theory (Kahneman and Tversky Citation1979). The method is based on a description, proved by empirical evidence, of how people effectively make decisions in the face of risk. The shape of the value function of the TODIM method appears the same as the gain/loss function of Prospect Theory (Gomes and Rangel Citation2009; Tosun and Akyüz Citation2015).
The concept of introducing expressions of losses and gains in the same multi-attribute function, present in the formulation of TODIM, gives this method some similarity to the PROMETHEE (Preference Ranking Organization Method for Enrichment Evaluations) methods, which make use of the notion of net outranking flow (Avikal, Mishra, and Jain Citation2014; Bouyssou Citation1986; Brans and Mareschal Citation1990; Brans and Vincke Citation1985; Brans, Vincke, and Mareschal Citation1986; Chen Citation2014; Chen, Wang, and Wu Citation2011; Elevli Citation2014; Goumas and Lygerou Citation2000; Gupta, Sachdeva, and Bhardwaj Citation2012; Kabir and Sumi Citation2014; Li and Li Citation2010; Motlagh et al. Citation2015; Peng, Kou, and Li Citation2014; Tversky Citation1969).
In TODIM, the dominance between two alternatives with respect to a particular criterion is transformed into gain/loss function by the formulation of prospect theory; wherein PROMETHEE, the extent of dominance is interpreted in terms of outranking flow and net outranking flow by means of preference function. Hence, in PROMETHEE, the prospect function of TODIM is replaced by the preference function.
In the proposed decision support system, the dominance is measured but not transformed into gain/loss function (as in TODIM) or preference function (as in PROMETHEE). In this approach, whilst two alternatives are compared with respect to a particular criterion; if the difference between the evaluation measures becomes positive; that means the first alternative is dominating the second one and hence the dominance is positive. In the reverse case, if the difference between evaluation measures appears negative; it means the first alternative is dominated by the second one. Therefore, the dominance extent for the first alternative assumes a negative value. The dominance between two alternatives (with respect to a particular criterion) is computed to obtain partial matrices of dominance. The global matrices of dominance are then computed for the candidate alternatives, based on which a global index measure is obtained to facilitate final ranking. The proposed decision-making pathways outlined herein avoid computational complexity of TODIM as well as PROMETHEE.
The procedural steps of the decision support system proposed herein have been summarised below.
Step 1: Arrange the decision-making group, set of alternatives and evaluation criteria.
Assume m possible alternatives: K decision-makers:
Also,
The problem encompasses a total of m number of alternatives and each alternative is evaluated by a total n number of criteria.
Step 2: Construct a decision matrix for each of the decision-makers in relation to appropriateness rating of alternatives with respect to criteria i.e.
; and also obtain decision-makers’ judgment in regard to weight of the criteria
(17)
Step 3: Aggregate decision-makers’ pulled opinion to compute aggregated rating of alternatives and aggregated weight of criteria.
Aggregate fuzzy ratings of alternatives with respect to reach criterion and fuzzy weights of each criterion
.
(18)
(19)
(20)
(21)
Also (22)
(23)
(24)
(25)
Step 4: Establish initial decision-making matrix.(26)
Step 5: Normalise the decision-making data and thereby establishing the normalised decision matrix.(27)
(28)
Step 6: Construct weighted normalised decision matrix.(29)
Step 7: Calculate the partial matrices of dominance using Equation Equation(30)
(30) . The term
represents the contribution of the criterion c to the function
i.e. global dominance when comparing alternative p with alternative q.
(30)
In this expression (Equation Equation30(30) ),
and
stands for the defuzzified values of the fuzzy number
and
, respectively. Here,
and
represent weighted normalised rating of alternative p and q respectively, for a particular criterion c as obtained in Step 6. The term
designates the distance between the two fuzzy numbers
and
that can be computed using Equation Equation(6)
(6) . Three cases can occur in (Equation Equation30
(30) ): (i) if the value
is positive, it represents a dominance (alternative p is dominating alternative q); (ii) if the value
is zero, there is null dominance; (iii) if the value
is negative, it represents negative dominance (alternative q is dominating alternative p).
A separate formulation can be well attributed if fuzzy subtraction formula is employed instead of fuzzy distance measure between
and
. The dominance of alternative p over q (for a particular criterion c) can also be computed as in (Equation Equation31
(31) ) as follows:
(31)
Here represents fuzzy subtraction operator.
and
represent weighted normalised rating of alternatives p and q, respectively for a particular criterion c. In this case, partial dominance measure i.e.
also becomes a fuzzy number. Whilst, in (Equation Equation30
(30) ), the distance measure between two fuzzy numbers i.e.
being a positive value; appropriate sign has to be considered separately to indicate whether alternative p is dominating alternative q (positive dominance); or alternative p is dominated by alternative q (negative dominance).
Step 8: The final matrix of dominance is obtained by summing up the partial matrices of dominance of each criterion.(32)
If, (Equation Equation32(32) ) is applied to compute partial matrices of dominance; the global dominance measure i.e.
becomes a fuzzy number; and hence, it is to be defuzzified again to proceed for computing ξ (refer to Step 9).
Step 9: Calculate the global value of the alternative ξ by normalising the final matrix of dominance according to the following expression.(33)
Step 10: Ordering the values ξ provides the rank of each alternative. The best alternative is one that has the highest value of ξ.
5. Case empirical illustration
In this work an empirical study has been presented to demonstrate application potential of the proposed dominance based fuzzy decision support system whilst comparing with Fuzzy-TOPSIS and Fuzzy-VIKOR. Empirical research is a kind of research using empirical evidence. It is a way of gaining knowledge by means of direct and indirect observation or experience. The decision-making data explored herein are truly empirical in nature; not collected from a particular case company. In practice, the top managerial body of the company should form the particular decision-making group, consisting of a finite number of decision-makers (DMs)/ experts. Experts may be from the members associated with company management, management consultants hired from outside, and/or even personnel from academia who possess expertise and vast experience to take part such industrial decision-making tasks. Apart from conducting a real case study, empirical illustration has been provided in this paper just to make authors understand about the application procedural steps of the proposed decision support system.
The present study articulates a supplier selection problem in consideration with green and resiliency criteria. It has been assumed that all of the candidate suppliers have achieved the requirements of traditional selection criteria (product price, delivery time, quality and service) of equal extent and hence, the best supplier has to be chosen in view of green as well as resiliency criteria. Pertinent attributes (criteria) relevant to g-resilient supplier selection have been listed in Table . The following criteria: Use of environment-friendly technology (C1), Use of environment-friendly materials (C2), Green market share (C3), Partnership with green organisations (C4), Management commitment (C5), Adherence to environmental policies (C6), Green R & D projects (C7), Staff Training (C8), Lean process planning (C9), Design for environment (C10), Environmental certification (C11), and Pollution control initiatives (C12), etc. have been considered as green criteria. Similarly, the following criteria: Investment in capacity buffers (C13), Responsiveness (C14), Capacity for holding strategic inventory stocks for crises (C15), etc. have been considered as resiliency criteria. The definitions of criteria (from both green and resilient dimensions) in relation to g-resilient supplier selection have been described in Table .
Table 1.1. Pertinent attributes relevant to g-resilient supplier selection.
Table 1.2. Definitions of criteria in relation to g-resilient supplier selection.
Assuming a group of five decision-makers (DMs) [DM1, DM2, DM3, DM4, DM5] have been employed to evaluate four candidate suppliers (S1, S2, S3, S4) in view of aforementioned green as well as resiliency criteria (C1 to C15); a 7-point fuzzy linguistic scale has been chosen to collect subjective judgment of the individual member of the decision-making group with regard to criteria weight as well as rating of alternative suppliers with respect to evaluation criteria. The following linguistic terms set: Very Low (VL), Low (L), Medium Low (ML), Medium/ Moderate (M), Medium High (MH), High (H) and Very High (VH) have been explored towards assigning criteria weight and the linguistic terms set: Very Poor (VP), Poor (P), Medium Poor (MP), Fair (F), Medium Good (MG), Good (G) and Very Good (VG) have been used to assess rating of alternative suppliers with respect to various criteria. The aforesaid two linguistic terms sets along with their fuzzy representation (trapezoidal membership functions) have been depicted in Table .
Table 2. 7-point linguistic scales and corresponding fuzzy numbers representation.
The decision-making group has been instructed to utilise those linguistic scales for assigning criteria weight and rating of alternatives in terms of linguistic variables. Since all the evaluation criteria are subjective in nature such kind of linguistic assessment is well justified. However, linguistic human judgment always bears some degree of uncertainty in terms of incompleteness as well as inconsistency; therefore, ambiguity and vagueness of imprecise data can efficiently be dealt with fuzzy set theory. Hence, linguistic decision-making information as provided by the expert group has been converted into appropriate fuzzy numbers; then, by exploring fuzzy decision-making approaches, the final decision outcome is achieved. Table represents criteria weight expressed in linguistic terms as given by the Decision-Makers (DMs). The subjective ratings of alternative suppliers (S1, S2, S3, S4) with respect to criteria as assessed by the DMs have been depicted in Tables , respectively. Linguistic data have been transformed into appropriate fuzzy numbers in accordance with Table . Using fuzzy aggregation rule, aggregated fuzzy ratings of alternatives with respect to criteria have been computed using (Equations Equation18–21), and tabulated in Table . Thus, initial decision support matrix has been arrived (Table ). Similarly, using (Equations Equation22–25), aggregated fuzzy weight of criteria have been computed as shown in Table . These fuzzy decision-making matrix along with fuzzy criteria weight have been analysed to check application potential of the proposed decision support framework. In the later phase, the same data-set has been utilised through the computational steps of Fuzzy-TOPSIS and Fuzzy-VIKOR. The ranking orders of alternative suppliers, obtained thereof, have been compared as well.
Table 3. Priority weight of criteria.
Table 4.1. Appropriateness rating of supplier alternative S1 with respect to evaluation criteria.
Table 4.2. Appropriateness rating of supplier alternative S2 with respect to evaluation of criteria.
Table 4.3. Appropriateness rating of supplier alternative S3 with respect to evaluation criteria.
Table 4.4. Appropriateness rating of supplier alternative S4 with respect to evaluation criteria.
Table 5. Aggregated fuzzy rating of supplier alternatives with respect to criteria: The initial decision-making matrix.
Table 6. Aggregated fuzzy weight of criteria.
5.1. Exploration of the proposed decision support framework
The data in relation to the initial decision-making matrix (Table ) have been normalised first. Assuming all evaluation criteria as beneficial in nature, (Equation Equation27(27) ) has been used to convert aggregated fuzzy ratings of alternatives (with respect to evaluation criteria) into normalised fuzzy ratings. The normalised fuzzy decision matrix has been depicted in Table . By employing (Equation Equation29
(29) ), normalised fuzzy rating of criteria have been multiplied with corresponding criteria weight; thus to obtain the weighted normalised decision matrix (Table ).
Table 7. The normalised decision matrix.
Table 8. The weighted normalised decision matrix.
In the proposed dominance-based decision-making approach, by utilising the data from the weighted normalised decision matrix (Table ), the partial matrices of dominance between alternative pairs (with respect to individual criteria) has been computed using (Equation Equation30
(30) ) and shown in Table . In constructing Table (the partial matrices of dominance), the fuzzy distance measure formula has been utilised (Equation Equation6
(6) ). The Euclidian distance between two fuzzy numbers being a crisp value (positive), proper sign consideration should be taken care of to indicate whether an alternative is dominating the other one or it is dominated by the other one. By utilising the data from the partial matrices of dominance, the final matrices of dominance have been computed using (Equation Equation32
(32) ) and shown in Table . Finally, the global value of alternative suppliers
has been computed by normalising the final matrix of dominance according to (Equation Equation33
(33) ) and shown in Table . The ranking order of alternative suppliers appears as: S4 > S3 > S2 > S1 (Table ).
Table 9. Partial matrices of dominance: Exploration of fuzzy distance measure formula.
Table 10. Final matrices of dominance.
Table 11. Global dominance value and corresponding ranking order.
A separate computational scheme can be well articulated if fuzzy subtraction operator is utilised instead of fuzzy distance measure in order to compute the partial matrices of dominance. Using (Equation Equation31(31) ) i.e. the fuzzy subtraction operator, the partial matrices of dominance
between alternative pairs (with respect to individual criteria) has been computed and shown in Table . Since subtraction of two fuzzy numbers yields another fuzzy number, the dominance between alternative pairs with respect to criteria has been expressed in terms of fuzzy numbers as shown in Table . Now, final matrices of dominance have been constructed using (Equation Equation32
(32) ) as shown in Table . Finally, the global dominance measure (for alternative suppliers), corresponding crisp score (by utilising the defuzzification formulae as given by (Rezvani Citation2013; Rouhparvar and Panahi Citation2015), normalised crisp score have been determined; based on which candidate suppliers have been ranked accordingly (Table ). The ranking order of alternative suppliers appears as: S4 > S1 > S2 > S3 (Table ). Supplier S4 still remains the best choice to have, while there is a slight change in the ranking order of remaining supplier alternatives. This may be because of the method of defuzzification applied. Here, the method of ‘incentre of centroid’ method has been applied to deffuzzify the fuzzy values in order to get the global measure for supplier’s ranking. However, if traditional defuzzification method (Equation Equation7
(7) ) could have been applied to calculate the global measure, the revealed ranking order could have obtained as S4 > S3 > S2 > S1.
Table 12. Partial matrices of dominance: Exploration of fuzzy subtraction rule.
Table 13. Final matrices of dominance.
Table 14. Global dominance measure, corresponding crisp score, normalised crisp score and supplier ranking order.
Table 15. Separation measure of each alternative with respect to ideal
and anti-ideal solution
: Computation of closeness coefficient
and corresponding ranking order (Exploration of Fuzzy-TOPSIS).
5.2. Comparative analysis
The ranking order of alternative suppliers as obtained above has also been compared to that of Fuzzy-TOPSIS and Fuzzy-VIKOR. The procedural steps of Fuzzy-TOPSIS and Fuzzy-VIKOR could be well understood from (Junior, Osiro, and Carpinetti Citation2014; Mokhtarian Citation2015; Sang, Liu, and Qin Citation2015) and (Liu et al. Citation2015; Mehbodniya et al. Citation2013; Pourebrahim et al. Citation2014), respectively. In course of Fuzzy-TOPSIS, the ideal as well as anti-ideal solution have been assumed as (1, 1, 1, 1) and (0, 0, 0, 0), respectively; whilst in pursuing computational steps of Fuzzy-VIKOR, the fuzzy best values and the fuzzy worst values
for all criteria have been assumed as (1, 1, 1, 1) and (0, 0, 0, 0), respectively (similar to ideal and anti-ideal solution of Fuzzy-TOPSIS). In Fuzzy-TOPSIS, ranking order has been evaluated based on CCi (closeness coefficient) against individual alternatives; whilst, in case of Fuzzy-VIKOR, supplier alternatives have been ranked based on overall ranking index i.e. Qi. In both the cases, the ranking order of candidate suppliers has appeared S4 > S3 > S2 > S1; exactly matches to that of obtained in the proposed approach (Refer Tables ); in which fuzzy distance measure formula has been utilised whilst computing dominance between two alternatives.
Table 16. Ranking order of alternative suppliers based on Si, Ri and Qi (Exploration of Fuzzy-VIKOR).
Table 17. Pertinent attributes relevant to g-resilient supplier selection.
5.3. Suppliers ranking based on G-Resilient Index: identification of ill-performing areas
Aforesaid sections deal with g-resilient supplier selection in view of a consolidated list of criteria arising from two separate dimensions i.e. green and resilience. Alternative suppliers have been ranked by the proposed dominance based approach. The ranking order obtained thereof has been compared to that of obtained through Fuzzy-TOPSIS and Fuzzy-VIKOR. However, these approaches cannot estimate an overall performance index (g-resilient index i.e. GRI) of individual suppliers. Computation of a unique g-resilient index is felt necessary to ascertain overall g-resilience performance index of supplier alternatives. Alternative suppliers can also be ranked based on their g-resilient index.
In order to compute, a unique g-resilient index of candidate suppliers, a different nomenclature of criteria-hierarch has been conceptualised (shown in Table ) to frame mathematical formulations of the procedural steps. Here, all pertinent attributes (C1 to C15 of Table ) have been divided into two broad dimensions (or main criteria/ performance indicators) i.e. green performance (PD1) and resilience performance (PD2). Each main-criterion has further been divided into some sub-criteria Cjl. Under green performance (PD1), a total of twelve sun-criteria have been assumed
. These have been denoted as: Use of environment-friendly technology (C11), Use of environment-friendly materials (C12), Green market share (C13), Partnership with green organisations (C14), Management commitment (C15), Adherence to environmental policies (C16), Green R & D projects (C17), Staff Training (C18), Lean process planning (C19), Design for environment (C1,10), Environmental certification (C1,11), Pollution control initiatives (C1,12), etc. Under resilience performance (PD2), three sun-criteria have been assumed
. These have been denoted as: Investment in capacity buffers (C21), Responsiveness (C22), Capacity for holding strategic inventory stocks for crises (C23) etc. Such a nomenclature has been adapted in this part of work to understand various computational formulae easily (Refer to Equations Equation34–36).
(34)
Table 18. Weight of g-resilient performance dimensions expressed in linguistic terms as given by the DMs: Corresponding aggregated fuzzy weight.
Here, is the aggregated fuzzy rating of ith alternative with respect to lth sub-criterion Cjl which is under jth main-criterion PDj. Also
is the aggregated fuzzy weight of lth sub-criterion Cjl which is under jth main-criterion PDj.
is the computed fuzzy rating of ith alternative with respect to jth main criterion PDj. It is also assumed that jth main criterion PDj consists of a total L number of sub-criteria.
Now, g-resilient index of alternative supplier can be computed as follows (Equation Equation35(35) ):
(35)
In this expression, is the computed fuzzy rating of ith alternative with respect to jth main criterion PDj;
being the aggregated fuzzy weight of jth main-criterion PDj. Also, GRIi be the g-resilient index of ith alternative. The total number of main-criteria is assumed as n.
Candidate suppliers can be ranked based on their g-resilient index. The suppliers’ g-resilient indices being fuzzy numbers have to be defuzzified to get the final ranking order.
Evaluation of g-resilient index not only helps in selecting appropriate suppliers adhering to green and resilient practices, but also helps individual suppliers to identify ill-performing areas involved in implementing an appropriate action requirement plan to improve the g-resilient performance.
The decision-making information in regard to weights of sub-criteria and ratings of alternatives with respect to criteria have been the same as depicted in Table and Tables , respectively; however, a diffident notation have been used herein as compared to the previous sections. The aggregated fuzzy ratings of alternatives (refer to the initial decision matrix in Table ) and the fuzzy priority weight of sub-criteria (refer to Table ) have been explored here to compute GRI of candidate suppliers. Since supplier selection decision has to be made based on two distinct performance criteria (green and resiliency), aggregating performance extents of greenness and resiliency, priority weights need to be assigned to each of the performance dimensions (PD1 and PD2). Different decision-makers have their own opinion, and hence, the expert group has been requested to assign priority weights for PD1 and PD2 in a subjective manner (linguistic judgment) as depicted in Table . Linguistic judgement has been transformed into appropriate fuzzy numbers as per Table . Next, aggregated fuzzy weight of main performance dimensions viz. PD1 and PD2 have been computed (using Equations Equation22–25) and shown in Table . Now, by exploring aggregated fuzzy ratings of alternatives with respect to sub-criteria (refer to Table ) and aggregated fuzzy weights of sub-criteria (refer to Table ), ratings of alternative suppliers with respect to main performance dimensions PD1 and PD2 have been computed (using Equation Equation34(34) ) and furnished in Table . Finally, computed fuzzy ratings of PD1 and PD2 (for individual supplier alternatives) and aggregated fuzzy weights of PD1 and PD2 have been combined using (Equation Equation35
(35) ) to compute the g-resilient index
of individual suppliers. Suppliers’ g-resilient indices being fuzzy numbers; have been defuzzified (by utilising the defuzzification formulae as given by (Rezvani Citation2013; Rouhparvar and Panahi Citation2015) to obtain the final ranking order (Table ). The ranking order appears as: S4 > S3 > S2 > S1; same as obtained before.
Table 19. Computed fuzzy rating of g-resilient performance dimensions.
Table 20. Computed fuzzy g-resilient index of alternative suppliers and corresponding ranking order.
In order to identify suppliers’ ill-performing areas to improve g-resilient performance, a Fuzzy Performance-Importance Index (FPII) has been computed herein, which combines the rating and weight of each sub-criterion. FPII represents an effect which influences suppliers’ g-resilient performance level. The degree of contribution of g-resilient performance for a sub-criterion decreases with decreasing FPII. Thus, the score of the FPII of a sub-criterion is used for identifying the ill-performing areas for achievement of satisfactory g-resilient performance level. The concept of FPII has been well articulated in Lin, Chiu, and Chu Citation(2006); Lin, Chiu, and Tseng Citation(2006); Samantra et al. (Citation2013).
If is used directly to calculate the FPIIijl (for ith supplier) in relation to sub-lth criterion Cjl (which is under jth main-criterion PDj), the importance weights
will neutralise the performance ratings in calculating FPIIijl; in this case, it will become impossible to identify the actual ill-performing areas (low performance rating and high importance). If
is high, then the transformation
is low. Consequently, to elicit a sub-criterion with low performance rating and high importance, the fuzzy performance-importance index FPIIijl indicating the effect of each sub-criterion that contributes to suppliers’ g-resilient performance, is defined as
(36)
Since fuzzy numbers do not always yield a totally ordered set in the manner of real numbers, the FPIIijls must be ranked. Suppliers can easily identify ill-performing sub-criteria by ranking FPIIs of individual sub-criteria.
The FPII for alternative suppliers with respect to individual sub-criteria have been computed (using Equation Equation36(36) ) and tabulated in Table . FPII values against each criterion with respect to individual supplier alternatives have been defuzzified again to compute a crisp score; based on which criteria have been ranked accordingly (Table ). Such a performance ranking scheme of supplier alternatives with respect to green as well as resiliency criteria helps in identifying ill (poor) performing areas which require future improvement to boost up overall g-resilience performance extent. For defuzzification of FPIIs, the recently developed method of ranking generalised TrFNs with Euclidean distance by the ‘incentre of centroids’ has been utilised herein (Rezvani Citation2013; Rouhparvar and Panahi Citation2015).
Table 21. Determination of FPII; performance ranking order of criteria (for individual suppliers).
5.4. Results and discussion
A consolidated criteria hierarchy has been conceptualised (Table ) by integrating green and resiliency criteria in order to find suppliers’ g-resilient performance. In this study, a total 15 criteria and 4 supplier alternatives have been considered. An initial decision-making matrix has been developed based on the alternative’s rating and criteria weightage by utilising the basics of fuzzy set theory (Tables ). The newly developed dominance based approach has been applied in two ways (i) Exploration of partial matrices of dominance through fuzzy distance formula (Equation Equation6(6) , Table ) and (ii) Exploration of partial matrices of dominance through fuzzy subtraction rule (Equation Equation4
(4) , Table ). The results obtained using this method in two ways show that the alternative/supplier S4 must be the first choice (Tables and ). For further authentication of the proposed method, a g-resilient performance index for each alternative supplier has been calculated; based on which the ranking order thus obtained (Table ) shows that supplier S4 has appeared the best. The most appropriate choice obtained herein perfectly matches to that determined in exploring the newly proposed dominance based approach, Fuzzy-TOPSIS (Table ), Fuzzy-VIKOR (Table ).
Apart from this, determination of criteria ranking order has also been attempted by determining the FPII; it has become evident from Tables and that supplier S1 is strong enough for criteria C16 (Adherence to environmental policies) but need a huge improvement for criteria C11 (Use of environment friendly technology). Supplier S2 is fair enough for resiliency performance criteria C22 (Responsiveness) and need valuable enhancement for criteria C17, (Green R & D projects). Similarly, supplier S3 is having good control over the criteria C22 (Responsiveness) while criteria C21 (Investment in capacity buffers) needs to be add more value. For the supplier S4 the performance criteria C22 (Responsiveness) is the most preferable one while criteria C1, 12 (Pollution control initiatives) is required necessary improvements in future.
5.5. Comparative analysis: exploration of a different fuzzy scale
In Section 4, generalised TrFNs have been used to transform linguistic human judgment into appropriate fuzzy representation; following which, fuzzy operational rules along with fuzzy based decision-making modules have been utilised to derive final ranking order of alternative suppliers. It is well known that apart from TrFN (membership function), triangular fuzzy numbers do exist in fuzzy set theory. Hence it seems worth of investigating that instead of TrFNs, if triangular fuzzy numbers are used, will there be any alternation of ranking order to candidate alternatives? In order to clarify this issue, the same problem has been resolved by considering 7-point linguistic scales for representing appropriateness rating of alternative suppliers with respect to criteria and priority weight of various criteria; which have been fuzzified further by means of (Fuzzy representation 2) i.e. a fuzzy scale with a combination of triangular as well as TrFNs (Refer to Table ). It has been observed that in all cases, ranking order of candidate suppliers appeared similar as obtained through exploration of TrFNs i.e. (Fuzzy representation 1) (Refer to Tables , , ). However, while performing computations of dominance based fuzzy decision-making approach (exploring fuzzy subtraction operator); it has been observed that ranking order appears S4 > S1 > S2 > S3 in both the cases in which Fuzzy representation 1 & 2 have been used, respectively (Refer to Table ). The most appropriate supplier has appeared S4 in all the cases. Therefore, it can be concluded that in the domain along real axis i.e. [0, 1] (Refer to Figure and Figure ), if total number of fuzzy membership functions remain same; change in the type of fuzzy membership function (for example, triangular; trapezoidal) does not alter the final ranking order and consequently the best choice of candidate alternatives.
6. Managerial implication
Efficient decision support system has always been a requirement for the supply chain managers to solve a variety of industrial decision-making problems in different decision environments. Application potential of the proposed fuzzy decision support framework based on dominance theory for solving MCDM problems has possibly got a positive signal in the foregoing study. Managers from various industries are hereby advised to adopt the guidelines for solving complex decision-making problems. The superiority of the proposed approach has been summarised below.
(1) | The obtained results are accurate and present a uniform ranking order even in comparison with other well-known MCDM approaches like Fuzzy-TOPSIS and Fuzzy-VIKOR. | ||||
(2) | The procedural steps of the decision support module based on dominance theory are quite simple which avoid multifaceted calculation part. | ||||
(3) | This method is flexible enough and can be solved using either fuzzy distance operator or fuzzy subtraction operator without noticeable navigation on the results. | ||||
(4) | This study would likely help supply chain manager to find out suppliers’ ill-performing areas so that the necessary action can be taken to improve that particular area. | ||||
(5) | Apart from supplier selection problem this methods can be applied in many industrial decision-making scenario where linguistic information is expected to create a barrier for effective decision-making. |
7. Conclusions and future scope
In the foregoing work, a g-resilient supplier selection framework has been anticipated in view of a decision-making scenario aiming to select the best possible g-resilient (ecosilient) supplier by considering green as well as resilient criteria. Subjectivity of suppliers evaluation criteria have been undertaken by means of fuzzy set theory.
The work exhibits application potential of a novel decision support framework based on dominance theory in the context of g-resilient supplier selection. The ranking order of candidate g-resilient suppliers has been compared to that of Fuzzy-TOPSIS and Fuzzy-VIKOR. Apart from this, a unified attempt has also been made to determine a unique g-resilient (ecosilient) performance index with respect to individual suppliers. The ranking order obtained by considering aforementioned g-resilient performance index has been compared with those obtained in the proposed dominance based decision support approach; also compared with Fuzzy-TOPSIS and Fuzzy-VIKOR. It has been noticed that for the current supplier selection problem, the best g-resilient supplier has appeared the same in all aforementioned approaches.
Limitations of the present work have also been pointed out below.
• | The decision support framework thus proposed in this study is based on Decision-Makers’ subjective judgment expressed in terms of linguistic variables. Linguistic information is transformed into appropriate fuzzy numbers with reference to a predefined fuzzy linguistic scale for establishing a logical mathematic base in order to quantify suppliers’ overall ranking index and thereby to go for suppliers’ ranking. The fuzzy based linguistic assessment scales used in this study have been adopted from the past literature. However, sensitivity of the proposed fuzzy decision support system has not been verified. | ||||
• | This study has attempted to identify ill-performing areas against individual supplier alternatives which require future attention to improve further so as to boost up overall g-resilient performance index; however, the ways to incur such improvements have not been prescribed here. | ||||
• | In this research, it has been assumed that the decision-making attitude of individual decision-makers’ is ‘neutral/ moderate’. However, it is worth investigating whether variation in decision-making attitude (i.e. optimistic/ neutral and pessimistic) of the DMs affects the final ranking order of candidate suppliers. Work may be extended to consider aforesaid issues in future research. |
Notes on contributors
Dilip Kumar Sen is a PhD research scholar in the Department of Mechanical Engineering, National Institute of Technology, Rourkela, India. His area of interest includes Industrial Management, Decision Making.
Saurav Datta is an assistant professor in the Department of Mechanical Engineering, National Institute of Technology, Rourkela, India. His current area of research includes weld quality optimisation, modelling and simulation of production processes, and multi-criteria decision-making. He has published a number of journal papers in national/ international repute and presented a number of papers in various conferences/ symposia in India and abroad. He is presently guiding a number of research scholars for MTech / PhD.
Siba Sankar Mahapatra is a professor in the Department of Mechanical Engineering, National Institute of Technology, Rourkela, India. He has more than 20 years of experience in teaching and research. His current area of research includes multi-criteria decision-making, quality engineering, assembly line balancing, group technology, neural networks and non-traditional optimisation and simulation. He has published more than 40 journal papers. He has written few books related to his research work. He is currently dealing with few sponsored projects.
Disclosure statement
No potential conflict of interest was reported by the authors.
Acknowledgement
Authors gratefully acknowledge the support rendered by the Editor-In-Chief of the International Journal of Sustainable Engineering. Special thanks to the anonymous reviewers for their valuable constructive comments and suggestions to prepare the paper a good contributor.
References
- Akili, E. A. 2009. “Green Supplier Selection Criteria. Supply Chain Systems”. Accessed October 22, 2010: facstaff. www.edu/prasads/761Green/Select.Pdf
- Amindoust, A., S. Ahmed, A. Saghafinia, and A. Bahreininejad. 2012. “Sustainable Supplier Selection: A Ranking Model Based on Fuzzy Inference System.” Applied Soft Computing 12 (6): 1668–1677.10.1016/j.asoc.2012.01.023
- Arikan, F. 2015. “An Interactive Solution Approach for Multiple Objective Supplier Selection Problem with Fuzzy Parameters.” Journal of Intelligent Manufacturing 26 (5): 989–998.10.1007/s10845-013-0782-6
- Avikal, S., P. K. Mishra, and R. Jain. 2014. “A Fuzzy AHP and PROMETHEE Method-based Heuristic for Disassembly Line Balancing Problems.” International Journal of Production Research 52 (5): 1306–1317.10.1080/00207543.2013.831999
- Awasthi, A., and G. Kannan. 2016. “Green Supplier Development Program Selection Using NGT and VIKOR Under Fuzzy Environment.” Computers and Industrial Engineering 91: 100–108.10.1016/j.cie.2015.11.011
- Awasthi, A., S. S. Chauhan, and S. K. Goyal. 2010. “A Fuzzy Multicriteria Approach for Evaluating Environmental Performance of Suppliers.” International Journal of Production Economics 126 (2): 370–378.10.1016/j.ijpe.2010.04.029
- Azadnia, A. H., M. Z. M. Saman, K. Y. Wong, P. Ghadimi, and N. Zakuan. 2012. “Sustainable Supplier Selection based on Self-organizing Map Neural Network and Multi Criteria Decision Making Approaches.” Procedia-Social and Behavioral Sciences 65: 879–884.10.1016/j.sbspro.2012.11.214
- Bai, C., and J. Sarkis. 2010. “Integrating Sustainability into Supplier Selection with Grey System and Rough Set Methodologies.” International Journal of Production Economics 124 (1): 252–264.10.1016/j.ijpe.2009.11.023
- Bakeshlou, E. A., A. A. Khamseh, M. A. G. Asl, J. Sadeghi, and M. Abbaszadeh. 2014. “Evaluating a Green Supplier Selection Problem Using a Hybrid MODM Algorithm.” Journal of Intelligent Manufacturing: 1–15. doi:10.1007/s10845-014-1028-y.
- Banaeian, N., H. Mobli, I. E. Nielsen, and M. Omid. 2015. “Criteria Definition and Approaches in Green Supplier Selection – A Case Study for Raw Material and Packaging of Food Industry.” Production and Manufacturing Research 3 (1): 149–168.10.1080/21693277.2015.1016632
- Barbarosoglu, G., and T. Yazgac. 1997. “An Application of the Analytic Hierarchy Process to the Supplier Selection Problem.” Production and Inventory Management Journal 38 (1): 14–21.
- Bouyssou, D. 1986. “Some Remarks on the Notion of Compensation in MCDM.” European Journal of Operational Research 26 (1): 150–160.10.1016/0377-2217(86)90167-0
- Brans, J. P., and B. Mareschal. 1990. “The PROMETHEE Methods for MCDM; The PROMCALC, GAIA and BANKADVISER Software.” Readings in Multiple Criteria Decision Aid, Chapter 2, 216–252. Berlin: Springer.
- Brans, J. P., and P. Vincke. 1985. “Note – A Preference Ranking Organisation Method: The PROMETHEE Method for Multiple Criteria Decision-making.” Management Science 31 (6): 647–656.10.1287/mnsc.31.6.647
- Brans, J. P., P. Vincke, and B. Mareschal. 1986. “How to Select and How to Rank Projects: The Promethee Method.” European Journal of Operational Research 24 (2): 228–238.10.1016/0377-2217(86)90044-5
- Büyüközkan, G. 2012. “An Integrated Fuzzy Multi-Criteria Group Decision-making Approach for Green Supplier Evaluation.” International Journal of Production Research 50 (11): 2892–2909.10.1080/00207543.2011.564668
- Büyüközkan, G., and G. Çifçi. 2012. “Evaluation of the Green Supply Chain Management Practices: A Fuzzy ANP Approach.” Production Planning and Control: The Management of Operations 23 (6): 405–418.10.1080/09537287.2011.561814
- Cao, H. J., F. Liu, Y. He, and H. Zhang. 2002. “Study on Model Set Based Process Planning Strategy for Green Manufacturing.” Computer Integrated Manufacturing System 8 (12): 978–982.
- Chai, J., and E. W. Ngai. 2015. “Multi-perspective Strategic Supplier Selection in Uncertain Environments.” International Journal of Production Economics 166: 215–225.10.1016/j.ijpe.2014.09.035
- Chen, S. H. 1985. “Ranking Fuzzy Numbers with Maximizing Set and Minimizing Set.” Fuzzy Sets and Systems 17 (2): 113–129.10.1016/0165-0114(85)90050-8
- Chen, C. T. 2000. “Extensions of the TOPSIS for Group Decision-making Under Fuzzy Environment.” Fuzzy Sets and Systems 114 (1): 1–9.10.1016/S0165-0114(97)00377-1
- Chen, T. Y. 2014. “A PROMETHEE-based Outranking Method for Multiple Criteria Decision Analysis with Interval Type-2 Fuzzy Sets.” Soft Computing 18 (5): 923–940.
- Chen, S. J., and S. M. Chen. 2007. “Fuzzy Risk Analysis Based on the Ranking of Generalized Trapezoidal Fuzzy Numbers.” Applied Intelligence 26 (1): 1–11.10.1007/s10489-006-0003-5
- Chen, S. M., W. H. Hsiao, and Y. J. Horng. 1997. “A Knowledge-based Method for Fuzzy Query Processing for Document Retrieval.” Cybernetics and Systems 28 (1): 99–119.10.1080/019697297126272
- Chen, C. T., C. T. Lin, and S. F. Huang. 2006. “A Fuzzy Approach for Supplier Evaluation and Selection in Supply Chain Management.” International Journal of Production Economics 102 (2): 289–301.10.1016/j.ijpe.2005.03.009
- Chen, Y. H., T. C. Wang, and C. Y. Wu. 2011. “Strategic Decisions Using the Fuzzy PROMETHEE for IS Outsourcing.” Expert Systems with Applications 38 (10): 13216–13222.10.1016/j.eswa.2011.04.137
- Chen, A., C. Y. Hsieh, and H. M. Wee. 2016. “A resilient Global Supplier Selection Strategy – A Case Study of an Automotive Company”. International Journal of Advanced Manufacturing Technology 87 (5–8): 1475–1490.
- Chin, T. A., H. H. Tat, and Z. Sulaiman. 2015. “Green Supply Chain Management’, Environmental Collaboration and Sustainability Performance.” Procedia CIRP 26: 695–699.10.1016/j.procir.2014.07.035
- Chopra, S., and M. S. Sodhi. 2004. “Managing Risk to Avoid Supply-chain Breakdown.” Sloan Management Review 46 (1): 53–62.
- Chou, S. Y., and Y. H. Chang. 2008. “A Decision Support System for Supplier Selection Based on a Strategy-aligned Fuzzy SMART Approach.” Expert Systems with Applications 34 (4): 2241–2253.10.1016/j.eswa.2007.03.001
- Christopher, M., and H. Peck. 2004. “Building the Resilient Supply Chain.” The International Journal of Logistics Management 15 (2): 1–14.10.1108/09574090410700275
- Çifçi, G., and G. Büyüközkan. 2011. “A Fuzzy MCDM Approach to Evaluate Green Suppliers.” International Journal of Computational Intelligence Systems 4 (5): 894–909.
- Datta, S., C. Samantra, S. S. Mahapatra, S. Banerjee, and A. Bandyopadhyay. 2012. “Green Supplier Evaluation and Selection Using VIKOR Method Embedded in Fuzzy Expert System with Interval-valued Fuzzy Numbers.” International Journal of Procurement Management 5 (5): 647–678.10.1504/IJPM.2012.048880
- Deng, X., Y. Hu, Y. Deng, and S. Mahadevan. 2014. “Supplier Selection Using AHP Methodology Extended by D Numbers.” Expert Systems with Applications 41 (1): 156–167.10.1016/j.eswa.2013.07.018
- Dobos, I., and G. Vörösmarty. 2014. “Green Supplier Selection and Evaluation Using DEA-type Composite Indicators.” International Journal of Production Economics 157: 273–278.10.1016/j.ijpe.2014.09.026
- Elevli, B. 2014. “Logistics Freight Center Locations Decision by Using Fuzzy-PROMETHEE.” Transport 29 (4): 412–418.10.3846/16484142.2014.983966
- Epstein, M., and P. Wisner. 2001. “Good Neighbours: Implementing Social and Environmental Strategies with the BSC.” Balanced Scorecard Report 3 (3): 8–11.
- Fallahpour, A., E. U. Olugu, S. N. Musa, D. Khezrimotlagh, and K. Y. Wong. 2016. “An Integrated Model for Green Supplier Selection Under Fuzzy Environment: Application of Data Envelopment Analysis and Genetic Programming Approach.” Neural Computing and Applications 27 (3): 707–725.10.1007/s00521-015-1890-3
- Fiksel, J. 2006. “Sustainability and Resilience: Toward a Systems Approach”. Sustainability: Science Practice and Policy 2 (2): 14–21.
- Freeman, J., and T. Chen. 2015. “Green Supplier Selection Using an AHP-Entropy-TOPSIS Framework.” Supply Chain Management: An International Journal 20 (3): 327–340.10.1108/SCM-04-2014-0142
- Ghadimi, P., and C. Heavey. 2014. “Sustainable Supplier Selection in Medical Device Industry: Toward Sustainable Manufacturing.” Procedia CIRP 15: 165–170.10.1016/j.procir.2014.06.096
- Gogoi, M., and M. Hazarika. 2014. “A Green Process Planning System”. 5th International and 26th All India Manufacturing Technology, Design and Research Conference (AIMTDR 2014), IIT Guwahati, Assam, India, December 12–14.
- Gomes, L. F. A. M., and L. A. D. Rangel. 2009. “An application of the TODIM Method to the Multicriteria Rental Evaluation of Residential Properties.” European Journal of Operational Research 193 (1): 204–211.10.1016/j.ejor.2007.10.046
- Goumas, M., and V. Lygerou. 2000. “An Extension of the PROMETHEE Method for Decision Making in Fuzzy Environment: Ranking of Alternative Energy Exploitation Projects.” European Journal of Operational Research 123 (3): 606–613.10.1016/S0377-2217(99)00093-4
- Gupta, R., A. Sachdeva, and A. Bhardwaj. 2012. “Selection of Logistic Service Provider Using Fuzzy PROMETHEE for a Cement Industry.” Journal of Manufacturing Technology and Management 23 (7): 899–921.10.1108/17410381211267727
- Haimes, Y. Y. 2006. “On the Definition of Vulnerabilities in Measuring Risks to Infrastructures.” Risk Analysis 26 (2): 293–296.10.1111/risk.2006.26.issue-2
- Haldar, A., A. Ray, D. Banerjee, and S. Ghosh. 2012. “A Hybrid MCDM Model for Resilient Supplier Selection.” International Journal of Management Science and Engineering Management 7 (4): 284–292.
- Haldar, A., A. Ray, D. Banerjee, and S. Ghosh. 2014. “Resilient Supplier Selection under a Fuzzy Environment.” International Journal of Management Science and Engineering 9 (2): 147–156.
- Handfield, R., S. V. Walton, R. Sroufe, and S. A. Melnyk. 2002. “Applying Environmental Criteria to Supplier Assessment: A Study in the Application of the Analytical Hierarchy Process.” European Journal of Operational Research 141 (1): 70–87.10.1016/S0377-2217(01)00261-2
- Handfield, R., R. Sroufe, and S. Walton. 2005. “Integrating Environmental Management and Supply Chain Strategies.” Business Strategy and the Environment 14 (1): 1–19.10.1002/(ISSN)1099-0836
- Hashemi, S. H., A. Karimi, and M. Tavana. 2015. “An Integrated Green Supplier Selection Approach with Analytic Network Process and Improved Grey Relational Analysis.” International Journal of Production Economics 159: 178–191.10.1016/j.ijpe.2014.09.027
- He, Y., F. Liu, H. Cao, and H. Zhang. 2007. “Process Planning Support System for Green Manufacturing and its application.” Frontiers of Mechanical Engineering in China 2 (1): 104–109.10.1007/s11465-007-0018-6
- Hervani, A. A., M. M. Helms, and J. Sarkis. 2005. “Performance Measurement for Green Supply Chain Management.” Benchmarking: An International Journal 12 (4): 330–353.10.1108/14635770510609015
- Humphreys, P., R. McIvor, and F. Chan. 2003. “Using Case-based Reasoning to Evaluate Supplier Environmental Management Performance.” Expert Systems with Applications 25 (2): 141–153.10.1016/S0957-4174(03)00042-3
- Hwang, C. L., and M. J. Lin. 1987. Group Decision Making Under Multiple Criteria. Berlin: Springer-Verlag.10.1007/978-3-642-61580-1
- Igoulalene, I., L. Benyoucef, and M. K. Tiwari. 2015. “Novel Fuzzy Hybrid Multi-criteria Group Decision Making Approaches for the Strategic Supplier Selection Problem.” Expert Systems with Applications 42 (7): 3342–3356.10.1016/j.eswa.2014.12.014
- Junior, F. R. L., L. Osiro, and L. C. R. Carpinetti. 2014. “A Comparison Between Fuzzy AHP and Fuzzy TOPSIS Methods to Supplier Selection.” Applied Soft Computing 21: 194–209.10.1016/j.asoc.2014.03.014
- Jüttner, U., H. Peck, and M. Christopher. 2003. “Supply Chain Risk Management: Outlining an Agenda for Future Research.” International Journal of Logistics: Research and Applications 6 (4): 197–210.10.1080/13675560310001627016
- Kabir, G., and R. S. Sumi. 2014. “Integrating Fuzzy Analytic Hierarchy Process with PROMETHEE Method for Total Quality Management Consultant Selection.” Production and Manufacturing Research 2 (1): 380–399.
- Kahneman, D., and A. Tversky. 1979. “Prospect Theory: An Analysis of Decision under Risk.” Econometrica 47 (2): 263–292.10.2307/1914185
- Kanagaraj, G., S. G. Ponnambalam, and N. Jawahar. 2016. “Reliability-based Total Cost of Ownership Approach for Supplier Selection Using Cuckoo-inspired Hybrid Algorithm.” The International Journal of Advanced Manufacturing Technology 84 (5–8): 801–816.
- Kannan, G., A. N. Haq, P. Sasikumar, and S. Arunachalam. 2008. “Analysis and Selection of Green Suppliers Using Interpretative Structural Modelling and Analytic Hierarchy Process.” International Journal of Management and Decision Making 9 (2): 163–182.10.1504/IJMDM.2008.017198
- Kaufmann, A., and M. M. Gupta. 1991. Introduction to Fuzzy Arithmetic: Theory and Applications. New York: Van Nostrand Reinhold.
- Kuo, R. J., Y. C. Wang, and F. C. Tien. 2010. “Integration of Artificial Neural Network and MADA Methods for Green Supplier Selection.” Journal of Cleaner Production 18 (12): 1161–1170.10.1016/j.jclepro.2010.03.020
- Lee, A. H., H. Y. Kang, C. F. Hsu, and H. C. Hung. 2009. “A Green Supplier Selection Model for High-tech Industry.” Expert Systems with Applications 36 (4): 7917–7927.10.1016/j.eswa.2008.11.052
- Li, C., and L. G. Debo. 2009. “Strategic Dynamic Sourcing From Competing Suppliers with Transferable Capacity Investment.” Naval Research Logistics 56 (6): 540–562.10.1002/nav.v56:6
- Li, W. X., and B. Y. Li. 2010. “An Extension of the Promethee II Method Based on Generalized Fuzzy Numbers.” Expert Systems with Applications 37 (7): 5314–5319.10.1016/j.eswa.2010.01.004
- Lin, C. T., H. Chiu, and P. Y. Chu. 2006. “Agility Index in the Supply Chain.” International Journal of Production Economics 100 (2): 285–299.10.1016/j.ijpe.2004.11.013
- Lin, C. T., H. Chiu, and Y. H. Tseng. 2006. “Agility Evaluation Using Fuzzy Logic.” International Journal of Production Economics 101 (2): 353–368.10.1016/j.ijpe.2005.01.011
- Liu, H. C., J. X. You, X. Y. You, and M. M. Shan. 2015. “A Novel Approach for Failure Mode and Effects Analysis Using Combination Weighting and Fuzzy VIKOR Method.” Applied Soft Computing 28: 579–588.10.1016/j.asoc.2014.11.036
- Lu, L. Y. Y., C. H. Wu, and T. C. Kuo. 2007. “Environmental Principles Applicable to Green Supplier Evaluation by Using Multi-objective Decision Analysis.” International Journal of Production Research 45 (18–19): 4317–4331.10.1080/00207540701472694
- Markowski, A. S., M. S. Mannan, and A. Bigoszewska. 2009. “Fuzzy Logic for Process Safety Analysis.” Journal of Loss Prevention in the Process Industries 22 (6): 695–702.10.1016/j.jlp.2008.11.011
- Mehbodniya, A., F. Kaleem, K. K. Yen, and F. Adachi. 2013. “A Fuzzy Extension of VIKOR for Target Network Selection in Heterogeneous Wireless Environments.” Physical Communication 7: 145–155.10.1016/j.phycom.2013.02.002
- Memon, M. S., Y. H. Lee, and S. I. Mari. 2015. “Group Multi-criteria Supplier Selection Using Combined Grey Systems Theory and Uncertainty Theory.” Expert Systems with Applications 42 (21): 7951–7959.10.1016/j.eswa.2015.06.018
- Mokhtarian, M. N. 2015. “A Note on ‘Extension of Fuzzy TOPSIS Method Based on Interval-valued Fuzzy Sets’.” Applied Soft Computing 26: 513–514.10.1016/j.asoc.2014.10.013
- Motlagh, S. M. H., M. Behzadian, J. Ignatius, M. Goh, M. M. Sepehri, and T. K. Hua. 2015. “Fuzzy PROMETHEE GDSS for Technical Requirements Ranking in HOQ.” The International Journal of Advanced Manufacturing Technology 76 (9–12): 1993–2002.10.1007/s00170-014-6233-5
- Nielsen, I. E., N. Banaeian, P. Golińska, H. Mobli, and M. Omid. 2014. “Green Supplier Selection Criteria: From a Literature Review to a Flexible Framework for Determination of Suitable Criteria.” Logistics Operations, Supply Chain Management and Sustainability: 79–99. Switzerland: Springer International Publishing.
- Orji, I., and S. Wei. 2014. “A Decision Support Tool for Sustainable Supplier Selection in Manufacturing Firms.” Journal of Industrial Engineering and Management 7 (5): 1293–1315.
- Özgen, D., S. Önüt, B. Gulsun, U. R. Tuzkaya, and G. Tuzkaya. 2008. “A Two-phase Possibilistic Linear Programming Methodology for Multi-objective Supplier Evaluation and Order Allocation Problems.” Information Sciences 178 (2): 485–500.
- Peng, Y., G. Kou, and J. Li. 2014. “A Fuzzy PROMETHEE Approach for Mining Customer Reviews in Chinese.” Arabian Journal for Science and Engineering 39 (6): 5245–5252.10.1007/s13369-014-1033-7
- Pourebrahim, S., M. Hadipour, M. B. Mokhtar, and S. Taghavi. 2014. “Application of VIKOR and Fuzzy AHP for Conservation Priority Assessment in Coastal Areas: Case of Khuzestan District, Iran.” Ocean and Coastal Management 98: 20–26.10.1016/j.ocecoaman.2014.05.009
- Rajesh, R., and V. Ravi. 2015. “Supplier Selection in Resilient Supply Chains: A Grey Relational Analysis Approach.” Journal of Cleaner Production 86: 343–359.10.1016/j.jclepro.2014.08.054
- Rao, P. 2006. “Greening of Suppliers/In-bound Logistics – In the South East Asian Context.” Greening the Supply Chain, 189–204. London: Springer.
- Rao, P., and D. Holt. 2005. “Do Green Supply Chains Lead to Competitiveness and Economic Performance?” International Journal of Operations and Production Management 25 (9): 898–916.10.1108/01443570510613956
- Rezaei, J., P. B. Fahim, and L. Tavasszy. 2014. “Supplier Selection in the Airline Retail Industry Using a Funnel Methodology: Conjunctive Screening Method and Fuzzy AHP.” Expert Systems with Applications 41 (18): 8165–8179.10.1016/j.eswa.2014.07.005
- Rezvani, S. 2013. “Ranking Generalized Trapezoidal Fuzzy Numbers with Euclidean Distance by the Incentre of Centroids.” Mathematica Aeterna 3 (2): 103–114.
- Rouhparvar, H., and A. Panahi. 2015. “A New Definition for Defuzzification of Generalized Fuzzy Numbers and Its Application.” Applied Soft Computing 30: 577–584.10.1016/j.asoc.2015.01.053
- Samantra, C., S. Datta, S. Mishra, and S. S. Mahapatra. 2013. “Agility Appraisal for Integrated Supply Chain Using Generalized Trapezoidal Fuzzy Numbers Set.” The International Journal of Advanced Manufacturing Technology 68 (5–8): 1491–1503.10.1007/s00170-013-4937-6
- Sang, X., X. Liu, and J. Qin. 2015. “An Analytical Solution to Fuzzy TOPSIS and Its Application in Personnel Selection for Knowledge-intensive Enterprise.” Applied Soft Computing 30: 190–204.10.1016/j.asoc.2015.01.002
- Shaik, M., and W. Abdul-Kader. 2011. “Green Supplier Selection Generic Framework: A Multi-attribute Utility Theory Approach.” International Journal of Sustainable Engineering 4 (1): 37–56.10.1080/19397038.2010.542836
- Srivastava, S. K. 2007. “Green Supply-chain Management: A State-of-the-art Literature Review.” International Journal of Management Reviews 9 (1): 53–80.10.1111/ijmr.2007.9.issue-1
- Tang, C. S. 2006. “Robust Strategies for Mitigating Supply Chain Disruptions.” International Journal of Logistics 9 (1): 33–45.10.1080/13675560500405584
- Tang, O., and S. N. Musa. 2011. “Identifying Risk Issues and Research Advancements in Supply Chain Risk Management.” International Journal of Production Economics 133 (1): 25–34.10.1016/j.ijpe.2010.06.013
- Tierney, K., and M. Bruneau. 2007. “Conceptualizing and Measuring Resilience: A Key to Disaster Loss Reduction.” TR news 17 (250): 14–15. doi:10.17226/23168.
- Tosun, Ö., and G. Akyüz. 2015. “A Fuzzy TODIM Approach for the Supplier Selection Problem.” International Journal of Computational Intelligence Systems 8 (2): 317–329.10.1080/18756891.2015.1001954
- Tseng, M. L., and A. S. Chiu. 2013. “Evaluating Firm’s Green Supply Chain Management in Linguistic Preference.” Journal of Cleaner Production 40: 22–31.10.1016/j.jclepro.2010.08.007
- Tversky, A. 1969. “Intransitivity of Preferences.” Psychological Review 76 (1): 31–48.10.1037/h0026750
- Ulutas, A., N. Shukla, S. Kiridena, and P. Gibson. 2016. “A Utility-driven Approach to Supplier Evaluation and Selection: Empirical Validation of an Integrated Solution Framework.” International Journal of Production Research 54 (5): 1554–1567.10.1080/00207543.2015.1098787
- Wood, D. A. 2016. “Supplier Selection for Development of Petroleum Industry Facilities, Applying Multi-criteria Decision Making Techniques Including Fuzzy and Intuitionistic Fuzzy TOPSIS with Flexible Entropy Weighting.” Journal of Natural Gas Science and Engineering 28: 594–612.10.1016/j.jngse.2015.12.021
- Yazlali, Ö., and F. Erhun. 2009. “Dual-supply Inventory Problem with Capacity Limits on Order Sizes and Unrestricted Ordering Costs.” IIE Transactions 41 (8): 716–729.10.1080/07408170802705768
- Yeh, W. C., and M. C. Chuang. 2011. “Using Multi-objective Genetic Algorithm for Partner Selection in Green Supply Chain Problems.” Expert Systems with Applications 38 (4): 4244–4253.10.1016/j.eswa.2010.09.091
- You, X. Y., J. X. You, H. C. Liu, and L. Zhen. 2015. “Group Multi-criteria Supplier Selection Using an Extended VIKOR Method with Interval 2-tuple Linguistic Information.” Expert Systems with Applications 42 (4): 1906–1916.10.1016/j.eswa.2014.10.004
- Zhu, Q., J. Sarkis, and K. H. Lai. 2008. “Confirmation of a Measurement Model for Green Supply Chain Management Practices Implementation.” International Journal of Production Economics 111 (2): 261–273.10.1016/j.ijpe.2006.11.029
- Zhu, Q., Y. Dou, and J. Sarkis. 2010. “A Portfolio‐based Analysis for Green Supplier Management Using the Analytical Network Process.” Supply Chain Management: An International Journal 15 (4): 306–319.10.1108/13598541011054670
- Zimmermann, H. J. 1991. Fuzzy Set Theory – And Its Applications. 2nd ed. Boston: Kluwer Academic.10.1007/978-94-015-7949-0