Abstract
Many developing countries such as Turkey are still making an effort on building an infrastructure for waste of electrical and electronic equipment (WEEE) reverse logistic network design (RLND) processes. It is obvious that policies/laws/regulations related to WEEE management provide a sustainable framework for implementation in the RLND. The question is here: Does the implementation of WEEE directives make sense in terms of reducing the total cost of the network in the long term? This study aims to compare regulatory and non-regulatory situations of WEEE RLND in developing countries by formulating two models named as ‘regulatory’ and ‘non-regulatory’. Model 1 is considered as sustainable with economic, environmental and social goals, and the quotas imposed by the environmental directive are taken into consideration as the data of product return amount. In Model 2, only economic goal is considered, and product return amount is forecasted using Artificial Neural Network (ANN). A case study is conducted in a recycling company in order to evaluate performance of the proposed models. This study contributes to the relevant literature by (1) comparing the regulatory and non-regulatory situations RL models explicitly and (2) proposing ANN model to forecast EEE product return or WEEE quantity for non-regulatory situation.
1. Introduction
In the past years, firms, society and governments have increased their attention towards sustainable development. One of the most popular definitions of sustainable development is stated by the World Commission on Environment and Development (WCED) as ‘sustainability is a development that meets the needs of the present without compromising the ability of future generations to meet their needs’. In general, it includes two important key points: ‘the concept of “needs”, in particular the essential needs of the world’s poor, to which overriding priority should be given’; and ‘the idea of limitations imposed by the state of technology and social organisation on the environment’s ability to meet present and future needs’. (Citation1987). As an industrial viewpoint, Shrivastava and Hart (Citation1995) believe that the structures of industrial economies should be changed using energy and resources efficiently, reducing the wastes, emissions and technological dangerous effects. Sustainable development activities have been adopted in the practice of many companies. For instance, IBM, Hewlett Packard, Xerox, ReCellular promote the take-back of their products in order to recover them (Bleiwas Citation2001; Fleischmann, Nunen, and Gräve Citation2002). Recently, sustainability has begun to address the integration of not only environmental and economic responsibilities, but also social responsibilities. Compared with industrial viewpoint, the engineering viewpoint defines sustainability explicitly by incorporating social dimensions such as social equilibrium. Gończ et al. (Citation2007) state that economic stability, ecological compatibility and social equilibrium have the same equality for sustainable development.
The definitions address that there are three main components of sustainability: economic, environmental and social. The integration of three dimensions of sustainability is also known as ‘triple bottom line approach’ which is developed by (Elkington Citation2004). The main focus of triple bottom line approach is to handle economic, environmental and social dimensions simultaneously and to consider environmental and social performances while measuring financial performance (Elkington Citation2004). The relationship between sustainability and triple bottom line is shown in Figure (Carter and Rogers Citation2008). As Figure specifies, the intersection area of three dimensions addresses sustainability.
In the last decades, one of the important managerial and engineering sustainability issues is ‘sustainable supply chain design’. It is a fairly new subject that integrates economic, environmental and societal goals in supply chain design process. The descriptions of the goals in terms of sustainable supply chain approach are discussed below.
(1) | Economic goals in supply chain are maximising the economic efficiency by providing financial benefits to owners, employees and community. In micro-economic environments, economic goals are mainly incorporated into the mathematical models as minimisation of total costs or maximisation of profit. | ||||
(2) | Environmental goals are attached more importance especially after environmental regulations come into force. Recovery activities result in environmental risks such as dangerous gas emission into the atmosphere (Chaabane et al. Citation2012b). The evaluation of trade-offs between economic advantages and dangerous gas emission is necessary in order to make strategic, tactical and operational sustainable decisions. The environmental regulations aim to conserve the natural resources, for instance by limiting carbon dioxide emission that is from vehicles used in logistics activities. Carbon dioxide is the most important greenhouse gas emitted through human operations, and with the impact of the Industrial Revolution which began around 1750, climate has been increasingly changed as a consequence of increase in carbon dioxide caused from human operations (EPA Citation2014). The main mobile sources of carbon dioxide emission are cars, vehicles, trucks, buses, aircrafts, trains and ships, construction vehicles and military vehicles and devices (Song et al. Citation2002). It indicates that the logistics activities have an important role in carbon dioxide emission amount. Besides decrease in carbon dioxide emission, the reduction of global warming and consumption of non-renewable energy can be achieved by recycling activities that allow obtaining high value materials from end of life products (Giacchetta, Leporini, and Marchetti Citation2013). | ||||
(3) | Social goals include quality-based issues, ethical-based issues, health safety issues and employment issues. Similarly, Carter and Jennings (Citation2002) specify that social aspects consist of issues related with ethics, diversity, working conditions, human rights, safety, philanthropy and community involvement. Because social aspects are affected from governmental rules and cultural characteristics (Chaabane et al. Citation2012b) and they are in intangible form, it is not so easy to incorporate them into the mathematical model. |
In many countries, the most important reason of growing interest in RL is environmental regulations. All around the world, many regulations have been developed that impose certain responsibilities on the actors of network, such as manufacturers, logistics service providers and municipalities. For instance, European Union (EU) Directives 2002/96/EC and 2002/95/EC are two of the most stringent regulations regarding waste electrical and electronic equipment (WEEE) (European Parliament and of the Council, Directive 2002/96/EC and 2002/95/EC Citation2002). WEEE is the fastest growing waste group in the EU and produced 8.3–9.1 million tons in 2005. It is forecasted that it will grow to 12.3 million tons by 2020. The main objective of the Directive is, (1) to prevent WEEE, (2) to impose on the recovery activities and (3) to develop the environmental performance of all actors in the chain (REC Citation2012). The WEEE Directive includes all electrical and electronic equipment that are commonly used in society and industry such as large household appliances; small household appliances; IT and telecommunications equipment; consumer equipment; lighting equipment; electrical and electronic tools; toys, leisure and sports equipment; medical devices; monitoring and control instruments and automatic dispensers (European Parliament and of the Council, Directive 2002/96/EC, Citation2002). The Directive includes strict obligations to decrease dangerous material usage and increase the recycling of electrical and electronic equipment by enforcing manufacturers to be responsible for taking back the products and reprocessing them. It also forces some operational goals such as collecting at least 4 kg of WEEE annually, ensuring the best technologies and organising and financing WEEE process.
For countries whose efforts are changing to sustainable processes, it is very prominent to figure out the results of sustainability with directives. Otherwise, it will not be possible for them to safeguard their systems against new outputs of the new sustainable systems. Especially budget constraints can limit their efforts; therefore, it is necessary to reveal the economic and strategic needs of sustainable systems. In order to declare the outputs of the sustainable WEEE RLND, regulatory-based sustainable network design and non-regulative network design should be compared. These models are general, flexible, multi-echelon (collection points, collection centres, recycling centres and disposal centres/refineries/material suppliers), multi-product, capacity constrained and one particular time period-based models. A case study is carried out in a recycling company in order to evaluate the performance of the proposed models. The proposed approaches can be used by companies to redesign their RL networks in order to balance their needs and required resources under the goals of regulatory and non-regulatory situations.
The paper is organised as follows: Section 2 reviews the relevant literature comprehensively. Section 3 reviews the main methodology of two models, and the implementation. We illustrate our method by applying it to the RL network of WEEE in a recycling company in Turkey. Section 4 presents sensitivity analysis and Section 5 presents the results and discussion.
2. Reverse logistics network design literature
In the relevant literature, it is seen that the RL topics range from strategic decisions to tactical and operational decisions (De Brito and Dekker Citation2004). One of the most important research topics in RL problem area is the RL network design (RLND) which has a strategic role in an effective supply chain improvement (Ramezani, Bashiri, and Tavakkoli-Moghaddam Citation2013). The main goal of environmental directives is to decrease the dangerous effects of products to the environment and society by designing sustainable RL networks. The strategic role of sustainable RLND comes from the ability to address costly, critical and risky decisions such as determination of quantity, location, capacity of the specified facilities and transportation modes under the dimensions of sustainability.
Based on sustainable issues, RLND studies also have become more important in recent years. In order to reveal the sustainability performance of the RLND studies, we execute a literature review on the studies relating to the period 1988–2016. The search consists of 237 papers. The results show that there are many years between 1998 and 2000 that no paper had been published in. Until 2006, the number of papers published each year was low. This result in itself shows that the importance of this research area has been increasing especially after 2006. Unfortunately, RLND models mentioned by previous studies are mostly using only the lowest cost or highest profit as its single evaluation objective. It is revealed that nearly 74% of papers use a single objective model and consider only economic goal. 21.5% of total takes into account environmental objectives besides economic ones. The comparison of number of studies with environmental objective and social objective can be seen in Figure . The results show that only Harraz and Galal (Citation2011), Dehghanian and Mansour (Citation2009), Darbari, Agarwal, and Chaudhary (Citation2015), Kafa, Hani, and Mhamedi (Citation2015), Govindan, Paam, and Abtahi (Citation2016) and Zhalechian et al. (Citation2016) state that they incorporate the social dimension into the RLND model. Harraz and Galal (Citation2011) consider the refund amount which represents the interest of ELV owner and a major concern of network operator as a social dimension. Dehghanian and Mansour (Citation2009) utilise Analytical Hierarchical Process (AHP) that considers four factors of social dimensions in order to assign different scores to each location alternatives. Darbari, Agarwal, and Chaudhary (Citation2015) utilise Analytical Hierarchy Process (AHP) and the Fuzzy Technique for Order Preference by Similarity to Ideal Solutions (TOPSIS) to measure the weights for the three objectives: minimisation of the total cost, the environmental impact (in terms of CO2 emissions) and maximisation of the social impact of the network. Kafa, Hani, and Mhamedi (Citation2015) MILP model is used to configure closed loop network by minimising the total cost of sourcing, the total greenhouse gas emissions and maximising job opportunities. Govindan, Paam, and Abtahi (Citation2016) simultaneously consider the environmental, social and economic aspects successfully. In order to meet the needs of sustainability, they develop a mathematical model that minimises the present value of costs, as well as environmental impacts, and optimises social responsibility. Zhalechian et al. (Citation2016) consider environmental impacts of CO2 emissions, fuel consumption, wasted energy and the social impacts of created job opportunities and economic development under uncertainty. It is clear that the existing literature is still far from combining three sustainability aspects simultaneously in RLND. Although social factors are taken into consideration as emerging subjects for the successful application of many reverse logistics practices (Sarkis Citation2001, Nikolaou, Konstantinos, and Allan Citation2012) state that recent RL models have a limited number of social dimensions that is a critical factor in the success of a firm to sustainable development. The distribution of objectives is shown in triple bottom line at Appendix 1.
Furthermore, we evaluate the papers within each solution method by examining two main methodologies: exact based and heuristic. There are 148 papers using the exact methods and 89 papers using the heuristic methods (seen in Figure ). One of the major differences between the two streams is that heuristic solution-based studies have lack of industrial case study-based implementations (as seen in Figure ). They are mostly limited with numerical examples. This result can be caused from conservativeness of companies on sharing large amount of real data and the hardness of gathering large amount of real data in a correct and trustful manner. The distribution shows that the interest on heuristics methods has occurred 10 years later than the first exact method-based study; Gottinger (Citation1988). Moreover, between 1998 and 2003, there was no study published using heuristic methods. After 2006, the number of papers using heuristics has grown exponentially and it has reached the highest point at 2010. In both exact- and heuristic solution-based RLND studies, mixed integer linear programming (MILP) is the most commonly used modelling type. However, in heuristics, the number of papers having non-linear programming model is more than exact method-based studies.
The taxonomy of the literature also shows that RLND is an immature research area especially for developing and undeveloped countries. In further years, by the effect of environmental regulations’ pressures, the motivation for researches on RLND will be expected to increase in these countries.
3. The methodology and implementation
This study proposes two quantitative models: Model 1 (Regulatory approach) and Model 2 (Non-Regulatory approach).
Model 1 refers to the sustainable situation; therefore, it is built by excluding situations resulting from complying with an environmental directive. It is assumed that the environmental directive comes into force in practical successfully, and responsibilities are fulfilled by all actors in the chain. Model 1 decides on opening the collection and recycling centres, and allocation of products/materials between centres, but it does not only aim at the minimisation of the total cost of the selected network, but also minimisation of environmental risk and maximisation of social benefit. Model 1 is proposed as a multi-objective mixed integer linear programming (MILP) model which takes requirements of sustainable economy into account. Product return amount is obtained from the quotas determined by the environmental directive.
Model 2 does not consider WEEE directives and sustainable issues. It decides on opening the collection and recycling centres, and allocation of products/materials between centres by aiming at minimising the total cost of the network. In order to forecast the product return amount, a decision-making approach using Artificial Neural Network (ANN) systems is improved. The results are used in the optimisation model as return amount data.
The methodological steps and details can be seen in Figure .
Both the proposed models are built for a general RL network in which the returned products are gathered in collection points (CP) and then collected in existing collection centres (ECC) or potential collection centres (CC) where inspection operations are conducted. The recoverable products are shipped to the existing recovery centres (ERC) or alternative recovery centres (RC); the rest of them are sent to the disposal centres (DC). After the recoverable products are recovered at recovery centres, most of them are recycled and sold to material suppliers (MS); some of them cannot be recovered because of license permissions and sent to the refinery centres (R); the rest of them are sent to disposal centres (DC). The network is given as Figure .
Various assumptions are involved in order to decrease the complexity of the problem structures in both the models. They are shown below.
• | Locations and capacities of current and alternative centres and containers in collection points are known. | ||||
• | Costs and allocation rates of products and materials are known. | ||||
• | The changing of costs in a selected term and inventory costs are negligible. | ||||
• | Material amount rates obtained from recycling are gathered from historical data. | ||||
• | Allocation rates between centres are obtained from historical data. |
In Turkey, with the results of the 2001 economic crisis, the unemployment rate increased to 8.4%. In further years, it increased to 10.3 and did not decline until 2005. As World Bank’s Report stated, in order to achieve EU average employment rate in 2010, Turkey has to increase job creation quantity to 10 million. Consequently, job creation has become the most significant and challenge topics of Turkish economy (Bilgin and Kilicarslan Citation2008). Therefore, considering the labour force increase as a job creation performance indicator is suitable for Turkey-based case in this study.
We take into consideration a recycling facility as an example to study some research questions and make some strategic decisions under triple bottom line approach necessities. Recycling centres have an important role at RL networks. They are responsible for taking out legal licenses from ministry and using appropriate technologies and techniques for recycling operations. Unless appropriate technologies and techniques are used, hazards for environment and health could increase and recycling of wastes could be done mostly by unskilled labour. Moreover, most of OEMs operate RL activities by collaborating with recycling firms. It is clear that the role of recycling centres is significant for fulfilment of an effective sustainable RL network. Therefore, in this study, case study is carried out with the data of the WEEE collection and recycling facility which operates in İzmit-Turkey.
The selected facility is the first licensed recycling firm built in Turkey. It has the highest market share as a recycling firm in this sector. The common recycled WEEE are monitors, televisions, IT and telecommunication equipment. The facility is in collaboration with municipalities, distributors and OEMs for collection of used or scrapped electrical and electronic equipment. In the network of the recycling firm, there are collection points (municipalities, OEMs and distributors), existing and alternative collection and recycling centres and disposal centres, material suppliers and refinery centres.
Strategically, the managers of the firm believe that after the WEEE Directive comes into force successfully in the following time, the product return volumes will increase rapidly. Therefore, they plan to extend its recycling operations to different locations in Turkey. Currently, it has one collection centre at İzmit () and one recovery centre at İzmit (
). In order to decide on alternative centre locations, potential locations are discussed in negotiations with related entities of municipalities. The managers determined some alternative locations for collection and recycling centres. The alternative locations for collection centres determined by managers are: Tekirdağ (
), Erzurum (
), Antalya (
), Kayseri (
), Diyarbakır (
) and Zonguldak (
). The alternative locations for recycling centres determined by managers are Ankara (
), İzmir (
), Adana (
) and Samsun (
). The products of the firm can be grouped into four types: large home appliances (product group 1), IT equipment and monitors (product group 2), lighting equipment (product group 3) and small home appliances (product group 4). The products are recycled into five different materials. They can be grouped into five categories: iron (raw material 1), metals except iron (raw material 2), glass (raw material 3), plastic (raw material 4) and others (raw material 5). There are two disposal centres (DC1 and DC2), one refinery centre (R) and three material suppliers (MS) in the existing network. Currently, transportation is achieved using highways. But WEEE Directive forces firms to use more environmentally friendly transportation modes. In this case, it is believed that the railway is going to be considered as a second transportation mode. The summary of case study characteristics is shown in Table . The location selection decisions are made in the collection and recovery centres which are shown in Figure .
Table 1. Case study characteristics.
Figure 7. The locations of the collection and recovery centres.
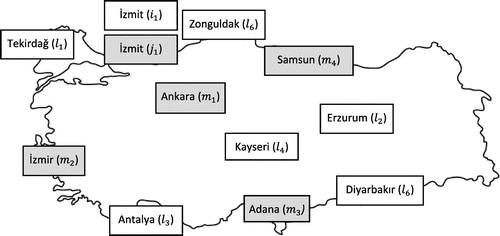
The resources of the data are illustrated in the following list.
(1) | The data of carbon dioxide emission amount are retrieved from United Nations Framework Convention on Climate Exchange (UNFCC) institution and carbon dioxide emission calculators for buildings. | ||||
(2) | The changing data regarding transportation mode are retrieved from Turkish State Railways reports. | ||||
(3) | The labour force data are retrieved from Turkish Statistical Institute’s databases. | ||||
(4) | The rest of data related to parameters and constraints are retrieved from the current and historical data of the recycling centre. |
3.1. The implementation of model 1
Model 1 proposes an optimisation model considering all sustainable dimensions in the same design procedure in accordance with triple bottom line approach. The model includes three main objectives:
(1) | minimising total costs as an economic goal, | ||||
(2) | minimising carbon dioxide emission as an environmental goal, | ||||
(3) | increasing job creation as a social goal. |
As Melo, Nickel, and Saldanha-da-Gama (Citation2009) state, although the choice of transportation mode is related to location decisions, researchers have not drawn much attention to the transportation mode selection in the relevant literature. Therefore, Model 1 also decides on the transportation mode which differs in terms of carbon dioxide emission amount.
The proposed model is general and flexible to solve larger problems. The mathematical formulation of the model is given in Appendix 2 [Equations (7)–(47)]. The following three objectives are addressed in the mathematical formulation:
(1) | Economic objective ( | ||||
(2) | Environmental objective ( | ||||
(3) | Social Objective ( |
(1) | Decision variables for opening a set of centres or closing existing ones. | ||||
(2) | Logical decisions that refer to the connections between centres. | ||||
(3) | Flow quantities between centres. |
(1) | Balance constraints which determine the equality between inputs and outputs of the centres. These constraints make assignment of products to the centres in proportion to their known rate amounts [shown in Equations (19)–(30)]. | ||||
(2) | Capacity constraints which prevent to transport more products to a centre than its capacity [shown in Equations (31)–(34)]. | ||||
(3) | Logic constraints which eliminate the possibility of irrational decisions such as sending a container to more than one centre. Furthermore, logical constraints guarantee to send products and/or materials to the centre if it is open. The minimum distribution amounts that are shown by |
The following model should be solved.(3)
(4)
For various parameters of a, many trials can be done. Boychuk and Ovchinnikov (Citation1973) propose ‘1’ for the value of a (Citation1971). If all objective functions and all constraints are linear and the value of a is 1, then the model will be a classical linear programming model. If a is chosen as 2, problem will have a quadratic form. The basic form of the model is:
Objective function
Minimum Cost (
Minimum Carbon Dioxide Emission (
Maximum Social Benefit (
Constraints
Balance constraints
Capacity constraints
Logic constraints
First of all, the multi-objective model is solved by considering each objective separately. The optimum results of each objective function ( are placed into the other objective function as known parameters. That retrieves other objective functions’ optimum values when optimal values of one objective function are considered as known parameters in the other model. The results can be shown as:
All alternatives of objective functions (
are shown in the following trade-off matrix:
(5)
The maximum values of each objective function are shown as ,
,
and the minimum values of each objective function are shown as
,
,
. The normalised model for minimisation of relative deviation of objective function values from feasible values can be shown as:
Objective function:(6)
Constraints
Balance constraints
Capacity constraints
Logic constraints
In this case, the value of a is given as ‘1’ because of the linearity of the proposed model.
The proposed model is optimised with mixed integer linear programming (MILP). It is coded in GAMS (general algebraic modelling system) CPLEX solver. As stated before, it is assumed that the data of rkp which refers to annual returns of products from collection points (containers) to collection centres are retrieved from WEEE Directive Regulation. The regulation forces all actors collect 4 kg e-wastes per person with the proportion of their market share. Therefore, returns of 81 collection points are calculated by multiplying the total population by 4 and getting the proportion of 10% that indicates the market share of the company. The results of the multi-objective model indicate that the optimum value of the network is 206,691,800 monetary units under the given conditions. The carbon dioxide emission amount will be 270,132,000,000 grams and the relative increase in labour force rate of the selected locations will be 0.000246. There exist capacity excess problems in the centres. That increases penalty costs and it causes high total cost of the network.
According to the optimum results, the existing collection and recovery centres at İzmit are not closed. Additionally, collection centres at Kayseri, Tekirdağ, Antalya and Diyarbakır and recovery centres at Ankara, İzmir, Adana and Samsun are opened. In this case, there are two different transportation modes between collection centres and recovery centres: highways and railways. The allocations between centres with various transportation modes are shown in Figure .
Due to limited space, the details cannot be shown in the figure. The detailed information about allocation results can be summarised as:
(1) | The optimum results address that product group 1 and product group 3 should be sent from the existing collection centre at İzmit ( | ||||
(2) | The unrecoverable products at collection centres should be sent to disposal centre DC 2. | ||||
(3) | The raw material 2 and raw material 5 which are retrieved from the existing recovery centre İzmit ( | ||||
(4) | The retrieved raw materials from Ankara ( | ||||
(5) | All raw materials that are retrieved from İzmir ( | ||||
(6) | The retrieved raw materials from Adana ( | ||||
(7) | The retrieved raw materials from Samsun ( | ||||
(8) | The unrecoverable and disposed products at Ankara ( | ||||
(9) | The unrecoverable and disposed products at İzmir ( | ||||
(10) | The unrecoverable and disposed products at Samsun ( |
3.2. The implementation of model 2
The studies utilising neural network approaches give more satisfactory results compared to traditional statistical methods in many cases (Mukta & Kumar, Citation2009). Therefore, an ANN approach which is a system that simulates biological neural networks to get solution for hard, mathematically ill-defined, non-linear or stochastic problems (Graupe Citation2007) is used for forecasting product return amount of Model 2. A general ANN structure includes three kinds of layer: input layer, hidden layer and output layer. The principle of ANN depends on carrying out many trials in order to find the best layer and processing element quantity for the relevant problem. The value of mean square error (MSE), as a performance indicator, shows the reasonability of each trial. If a trial gives MSE value equal or less than 0.01, it is mostly considered as highly satisfactory.
For Model 2, it is assumed that the factors such as take-back price (Klausner and Hendrickson Citation2000; Guide and Van Wassenhove Citation2001), population density (WEEE Directive; Hanafi, Kara, and Kaebernick Citation2007), income (WEEE Directive), product category (Hess and Mayhew Citation1997; Rogers and Tibben-Lembke Citation1999), investment on environmental protection, number of households (Ikhlayel Citation2016) and electricity consumptions have an impact on product return amount. That means the input layer consists of these seven factors. The general structure of the proposed ANN model is given in Figure .
It is necessary to train many networks with different hidden layers and neuron combinations to find the best structure which has the minimum MSE value. It is assumed that existing 200 collection points’ return amount is known. The ANN system is run only for forecasting product return of 19 alternative cities which have high potential to collect high amount of end of life products after the directive comes into force. Therefore, in total there will be 219 collection points in Model 2.
Experiment results show that in the network structure with minimum MSE values (MSE = 0.01) and maximum correlation value on test results (0.74), there are two hidden layers, and four neurons take place at first hidden layer and four neurons at second one. Neuro Solutions for Excel Software is utilised for ANN development process. The product amounts are given in Table .
Table 2. ANN results: return amount of alternative locations (in kg).
The mathematical model is a one-objective version of Model 1 that is given in Appendix 2. The environmental and social parameters and environmental and social objectives are excluded. The objective is to minimise total cost of the network as sum of annualised fixed costs, information costs, transportation costs, processing costs, disposal and refinery costs, collection costs and penalty costs (that is unit variable cost of non-used capacity of collection centres to each product). Balance constraints make sure that incoming and outgoing flows of the centres are balanced. Capacity equations make the incoming flow to each centre not exceed the maximum capacity of the centre, ensure that one container is sent to only one collection centre and reflect the limitations in minimum product amount required for moving into profit. Others represent the binary variables and the non-negativity of decision variables. Data of (product return amount) are gathered at the end of ANN development process.
The results indicate that the cost of the network is 55.434.247 monetary units under the given conditions. The existing collection and recovery centres at İzmit are not closed. Additionally, one collection centre at Kayseri and recovery centre at Ankara should be opened. The allocations between centres with various transportation modes are shown in Figure .
The detailed information about allocation results can be summarised as:
(1) | At the existing collection recovery centre (İzmit ( | ||||
(2) | At the existing recovery centre (İzmit ( | ||||
(3) | At the newly opened recovery centre (Ankara ( | ||||
(4) | The raw materials which are retrieved from the existing recovery centre İzmit ( |
Table 3. Results of Model 1 and Model 2.
4. Sensitivity analysis
In order to explore the effect of changing capacities and product return amount, two sensitivity analyses are proposed for both the models.
4.1. Sensitivity analysis for model 1
4.1.1. The effect of capacity changing
In the first sensitivity analysis, it is aimed to explore if the optimum centres are opened and their capacities are decreased, they will be successful to meet the product return amount or not. Because of that reason, the model is run for decreasing capacities of all collection and recovery centres by keeping the rest of the parameters constant. It is evident that the total cost converges with decreasing volume. It is seen that if their capacities are decreased more than 40%, the solution becomes infeasible. That means the capacity amounts can be reviewed again by managers because there is a capacity excess and that results in high penalty costs. It is necessary to look over the initial capacities for potential centres. The impact of decrease in capacities on the total cost can be seen in Figure . Moreover, it is worth mentioning that the amount of qualified and non-qualified workers linearly changes according to increase or decrease in capacity. Therefore, the effect of capacity on labour force is equal to the percentage change in capacity. But in actual, as a social responsibility, rather than laying off, the company should attach more importance on training the workers to increase their multi-functionalities, and thus new job opportunities can be created for excess labour within the company, such as automation-oriented operations and organising awareness raising activities.
The impact of decrease in capacities on the total carbon dioxide emission can be seen in Figure . As Figure specifies, if the capacities are decreased by 40%, besides increase in cost by 25%, the carbon dioxide emission will be decreased by 3.5%.
4.1.2. The effect of product returns amount changing
In the future, it is expected that the return quantity of WEEE will increase when the consciousness of consumer increases. In order to explore the effect of return amounts on the results, a sensitivity analysis is proposed by keeping the rest of the parameters constant. It is evident that the total cost converges with increasing volume. Also, when the amounts are increased more than 70% for each collection point, the model gives infeasible solution. That means if the return amount is increased by 70%, new centres should be open for recycling of total products. Increase in total cost with increasing return amounts can be seen in Figure .
The impact of increase in product return amount on the total carbon dioxide emission can be seen in Figure . As Figure specifies, if the product return amount is increased by 70%, the carbon dioxide emission will be increased by 15%.
4.2. Sensitivity analysis for model 2
4.2.1. The effect of capacity changing
The model is performed for decreasing capacities of all collection and recovery centres from 1% to 7% by keeping the rest of the parameters constant. As seen in Figure , the total cost converges with decreasing volume. Also, when the capacities decreased more than 7%, the model gives infeasible solution. That means if the capacities decreased to 7%, it is advised to open new centres for recycling of existing products.
4.2.2. The effect of product returns amount changing
The model is performed for increasing return amounts of all collection points from 5 to 10% by keeping the rest of the parameters constant. As seen in Figure , it is explicit that the total cost converges with increasing volume. Also, when the amounts increased more than 10%, the model gives infeasible solution. That means if the return amount is increased to 10%, it advised to open new centres for recycling of total products.
Table 4. Yearly product return amount retrieved from trend analysis.
According to sensitivity analysis results, it is clear that the given capacity values are high for existing conditions of the firm. That means firms should analyse capacity requirements with decision-making tools before making strategic decisions and high investments. Moreover, firms should follow yearly changing on return amounts in order to see their requirements on opening extra facilities (Figure ).
5. Results and discussion
This study presents a methodology that gives efficient solutions for all important actors of the system: companies, environment and society. The proposed models enable governments and managers to evaluate their strategic decisions from both sustainable regulative and non-regulative perspectives. There are some important discussion points and managerial insights that should be considered by the company:
• | In the sustainable network, it is revealed that it is not easily possible for a company in a developing country to have profit from RL activities. As Srivastava (Citation2008b) figures out, in developing countries in which directives comes into force, new, recovery options cannot give economic advantages in a short time. | ||||
• | In the sensitivity analysis, it is figured out that if the return amount is increased by 70%, new centres should be open for recycling of total products. By considering the Ministry of Environment and Urban Planning’s quotas between 2012 and 2018 (Ministry of Environment and Urban Planning Citation2008), we conducted a trend analysis in order to forecast how long the capacities will be appropriate for the company. As Table specifies, if the Directive comes into force successfully, in 2024, the company will have 7.09 kg e-wastes that refers to 70% increase on 4 kg e-waste quotas. These results indicate that in 2024, the company will have to review their capacities. | ||||
• | In order to clarify the total profit gained by RL activities in Model 2, break-even analysis is conducted. The revenue obtained from each material that is taken by recovery options is approximately 2.121 monetary units/ton. The 64% of total product returns are transformed to the materials such as plastic, glass, iron and aluminium. Under the light of these, looking up the relationship between marginal costs and revenue shows that the profit relatively decreases across time (as seen in Figure ). That shows although companies believe that establishment of a sustainable structure is highly expensive, non-regulative network design also offers no profitable advantage in the length of time. | ||||
• | In a sustainable environment, the given capacity values are high for existing conditions of the firm. That means firms should review capacity requirements by utilising decision-making tools before making strategic decisions that need high investments. | ||||
• | In a non-regulatory situation, if the return amount is increased to 10%, it advised to open new centres for recycling of total products. Therefore, managers should carefully track the increases in the product return amount in order to safeguard themselves against enlargement needs. | ||||
• | Unfavourable results indicate that it is not realistic to make the amount of product return goal as 4 kg for the year of 2018. Turkish Ministry of Environment and Urban Planning should review the product return quotas again, and rather than accepting the EU’s environmental regulation’s quotas directly, they should improve a unique system that considers Turkey’s conditions and characteristics. |
6. Conclusions and further researches
In the uncertain and complex nature of RL networks of developing countries, developing a methodology that compares the RL design model with regulative and non-regulative dimensions becomes a significant task. Although developing countries are familiar with non-regulative RL networks, due to the difficulty in incorporating environmental and social benefit into RLND models, there is a lack of RLND studies that fully achieves sustainability by taking into account triple bottom line approach necessities. In the light of these, this study aims to reveal the regulatory and non-regulatory situations of WEEE RLND in developing countries in which individual sustainable WEEE RLND efforts are still unorganised and informal. With this aim, we present two MILP models; one refers to the current conditions of a developing country including economical goal, and one refers to the future conditions of a developing country including three objectives: minimising total cost, minimising environmental risk and maximising labour force. Both the models are multi-echelon, multi-product, capacity constrained, particular time period facility location allocation models. Model 1 contributes to the relevant literature by generating multi-objective sustainable RL model for WEEE industry of developing countries. Model 2 contributes by proposing an ANN-based process for forecasting product returns. A case study is conducted in a recycling company in order to evaluate and validate performance of the proposed models. This comparative methodology can help the managers take decisions on facility selection by contributing to the industrial sustainable development and allow governments review their regulatory initiatives in terms of return amount expectations. The results reveal that in the adoption process managers should be aware of enormous investment costs that they have been charged at the beginning of the RL network design processes because in the beginning of the adoption process, product return amount is not enough to satisfy and meet the quotas identified by regulations. That also indicates that the ministry should immediately review the lower limits of WEEE collection amounts because it is not possible for a developing system to make society aware of the benefits of WEEE collection in a short time. Profit analysis indicates that even if managers are not likely to invest on sustainable networks because of its high costs, non-regulative systems offer no satisfactory profit advantage across time as it is expected. Therefore, they should not ignore the effect of economies of scale in WEEE collection and make effort on collecting more and more e-wastes that will also increase the profits after a while.
Directions for further researches include: (1) new issues can also be added into the methodology, such as lot sizing, vehicle routing and inventory management, (2) generalising the model to a multi-period programming model that can handle time-based changing on capacities, product returns, etc. and (3) allowing some parameters such as product returns, costs, capacities etc. uncertain. Furthermore, considering the lack of recorded data for the recycling facility employment, labour productivity and compensation costs, it should be highlighted that investigation of capacity and product return quantity impacts on job creation is a comprehensive evaluation focusing on recruiting decisions for future studies.
Notes on contributors
Gül Tekin Temur is an associate professor of Management Engineering at Bahçeşehir University, Turkey. Her main research interests are in supply chain management, reverse logistics, decision-making and artificial ıntelligence. Previous publications have appeared in Applied Soft Computing, International Journal of Computational Intelligence Systems, and Enterprise Information Management.
Bersam Bolat is an associate professor of Management Engineering at İstanbul Technical University, Turkey. Her main research interests are in production and operations management, production planning and control and project management. Previous publications have appeared in Resources, Conservation and Recycling, Enterprise Information Management, Computers & Industrial Engineering.
Disclosure statement
No potential conflict of interest was reported by the authors.
Funding
This study was supported by the Scientific and Technological Research Council of Turkey (TÜBİTAK) (Project No: 115M551).
Acknowledgement
The authors are thankful to TÜBİTAK for supporting their project numbered as 115M551.
References
- Accorsi, R., R. Manzini, C. Pini, and S. Penazzi. 2015. “On the Design of Closed-Loop Networks for Product Life Cycle Management: Economic, Environmental and Geography Considerations.” Journal of Transport Geography 48: 121–134.10.1016/j.jtrangeo.2015.09.005
- Achillas, C., D. Aidonis, C. Vlachokostas, N. Moussiopoulos, G. Banias, and D. Triantafillou. 2012. “A Multi-Objective Decision-Making Model to Select Waste Electrical and Electronic Equipment Transportation Media.” Resources, Conservation and Recycling 66: 76–84.10.1016/j.resconrec.2012.01.004
- Achillas, C., C. Vlachokostas, D. Aidonis, Ν. Moussiopoulos, E. Iakovou, and G. Banias. 2010. “Optimising Reverse Logistics Network to Support Policy-Making in the Case of Electrical and Electronic Equipment.” Waste Management 30: 2592–2600.10.1016/j.wasman.2010.06.022
- Ahluwalia, P. K., and A. K. Nema. 2006. “Multi-Objective Reverse Logistics Model for Integrated Computer Waste Management.” Waste Management and Research 24: 514–527.10.1177/0734242X06067252
- Alfonso, E. H., J. R. Montoya-Torres, and E. Gutiérrez-Franco. 2013. “Modeling Reverse Logistics Process in the Agro-Industrial Sector: The Case of the Palm Oil Supply Chain.” Applied Mathematical Modelling 37: 9652–9664.10.1016/j.apm.2013.05.015
- Alshamsi, A., and A. Diabat. 2015. “A Reverse Logistics Network Design.” Journal of Manufacturing Systems 37: 589–598.10.1016/j.jmsy.2015.02.006
- Alumur, S. A., S. Nickel, F. C. Saldanha-da-Gama, and V. Verter. 2012. “Multi-Period Reverse Logistics Network Design.” European Journal of Operational Research 220: 67–78.10.1016/j.ejor.2011.12.045
- Amin, S. H., and G. Zhang. 2012. “An Integrated Model for Closed-Loop Supply Chain Configuration and Supplier Selection: Multi-Objective Approach.” Expert Systems with Applications 39: 6782–6791.10.1016/j.eswa.2011.12.056
- Amin, S. H., and G. Zhang. 2013. “A Multi-Objective Facility Location Model for Closed-Loop Supply Chain network Under Uncertain Demand and Return.” Applied Mathematical Modelling 37: 4165–4176.10.1016/j.apm.2012.09.039
- Amin, S. H., and F. Baki. 2016. “A Facility Location Model for Global Closed-Loop Supply Chain Network Design.” Applied Mathematical Modelling 41: 316–330. doi:10.1016/j.apm.2016.08.030.
- Aras, N., D. Aksen, and A. G. Gönül Tanuğur. 2008. “Locating Collection Centers for Incentive-Dependent Returns Under a Pick-Up Policy with Capacitated Vehicles.” European Journal of Operational Research 191: 1223–1240.10.1016/j.ejor.2007.08.002
- Aras, N., Aybek Korugan, G. Büyüközkan, F. Şerifoğlu, İ. Erol, and M. N. Velioğlu. 2015. “Locating Recycling Facilities for IT-Based Electronic Waste in Turkey.” Journal of Cleaner Production 105: 324–336.10.1016/j.jclepro.2015.02.046
- Ashayeri, J., N. Ma, and R. Sotirov. 2015. “The Redesign of a Warranty Distribution Network with Recovery Processes.” Transportation Research Part E: Logistics and Transportation Review 77: 184–197.10.1016/j.tre.2015.02.017
- Assavapokee, T., and W. Wongthatsanekorn. 2012. “Reverse Production System Infrastructure Design for Electronic Products in the State of Texas.” Computers and Industrial Engineering 62: 129–140.10.1016/j.cie.2011.09.001
- Ayvaz, B., B. Bolat, and N. Aydın. 2015. “Stochastic Reverse Logistics Network Design for Waste of Electrical and Electronic Equipment.” Resources, Conservation and Recycling 104: 391–404.10.1016/j.resconrec.2015.07.006
- Bai, M. G. 2011. “Research on the Network Optimization for Reuse Reverse Logistics Under Grey Environment.” In Proceedings of 30th Chinese Control Conference (CCC), China, 5377–5381.
- Baptista, S., Gomes, M. I., and Barbosa-Povoa, A. P. 2012. “A Two-Stage Stochastic Model for the Design and Planning of A Multi-Product Closed Loop Supply Chain.” In Proceedings of the 22nd European Symposium on Computer Aided Process Engineering, 17–20 June 2012. London.
- Barros, A.I., R. Dekker, and V. Scholten. 1998. “A Two-Level Network for Recycling Sand: A Case Study.” European Journal of Operational Research 110: 199–214.10.1016/S0377-2217(98)00093-9
- Baykasoglu, A., and K. Subulan. 2015. “An Analysis of Fully Fuzzy Linear Programming with Fuzzy Decision Variables Through Logistics Network Design Problem.” Knowledge-Based Systems 90: 165–184.10.1016/j.knosys.2015.09.020
- Beamon, B. M., and C. Fernandes. 2004. “Supply Chain Network Configuration for Product Recovery.” Production Planning and Control 15: 270–281.10.1080/09537280410001697701
- Behmanesh, E., and J. Pannek. 2016. “Modeling and Random Path-Based Direct Encoding for a Closed Loop Supply Chain Model with Flexible Delivery Paths.” IFAC-PapersOnLine 49: 78–83.10.1016/j.ifacol.2016.03.014
- Bennekrouf, M., F. Boudahri, and Z. Sari 2011. “Optimal Design of Two Levels Reverse Logistic Supply Chain by Considering The Uncertain Quantity of Collected Multi-Products.” In Proceedings of 4th International Conference on Logistics (LOGISTIQUA), Tunusia, 397–404.
- Bilgin, H. B., and I. N. Kilicarslan. 2008. “An Analysis of the Unemployment in Selected Mena Countries and Turkey.” Journal of Third World Studies 189–205.
- Bing, X., J. Bloemhof-Ruwaard, A. Chaabane, and J. van der Vorst. 2015. “Global Reverse Supply Chain Redesign for Household Plastic Waste Under the Emission Trading Scheme.” Journal of Cleaner Production 103: 28–39.10.1016/j.jclepro.2015.02.019
- Bing, X., J. J. Groot, J. M. Bloemhof-Ruwaard, and J. G. A. J. van der Vorst 2013. “Multimodal Network Design for Sustainable Household Plastic Recycling.” International Journal of Physical Distribution and Logistics Management, 43 (5/6): 452–477.10.1108/IJPDLM-04-2012-01134
- Bleiwas, D. 2001. “Obsolete Computers, ‘Gold Mine,’ or High-Tech Trash? Resource Recovery from Recycling.” U.S. Department of the Interior. USGS Fact Sheet FS-060-01, 2001.
- Boychuk, L., and V. O. Ovchinnikov 1973. “Principal Methods for Solution of Multicriterial Optimization Problems.” Soviet Automatic Control 6 (3): 1–4.
- Cardoso, S. R., A. P. F. D. Barbosa-Póvoa, and S. Relvas. 2013. “Design and Planning of Supply Chains with Integration of Reverse Logistics Activities Under Demand Uncertainty.” European Journal of Operational Research 226: 436–451.10.1016/j.ejor.2012.11.035
- Carter, C. R., and M. M. Jennings. 2002. “Logistics Social Responsibility: An Integrative Framework.” Journal of Business Logistics 23: 145–180.10.1002/jbl.2002.23.issue-1
- Carter, C. R., and D. S. Rogers. 2008. “A Framework of Sustainable Supply Chain Management, Moving Toward New Theory.” International Journal of Physical Distribution and Logistics Management 38: 360–387.10.1108/09600030810882816
- Chaabane, A., A. Ramudhin, and M. Paquet. 2012a. “Design of Sustainable Supply Chains Under the Emission Trading Scheme.” International Journal of Production Economics 135: 37–49.10.1016/j.ijpe.2010.10.025
- Chaabane, A., A. Ramudhin, and M. Paquet. 2012b. “Designing Supply Chains with Sustainability Considerations.” Production Planning and Control 22: 727–741.
- Chang, X. Y., T. J. Fan, and Y. Xia 2009. “A Goal Programming Model for Reverse Logistics Network Planning.” In Proceedings of International Conference on Information Management, Innovation Management and Industrial Engineering, China, 451–456.
- Chen, Y. Y., and H. F. Wang 2012. “A Flexible Scheme for the Bi-Directional Logistics.” In Proceedings of PICMET ‘12, Technology Management for Emerging Technologies, 2817–2823.
- Chen, Y., D. Liu, H. Mao, and Y. Tian 2008. “Uncertain Programming of the Distribution/Recovery Center in Forward/Reverse Hybrid Logistics System.” In Proceedings of IEEE International Conference on Service Operations and Logistics and Informatics, China, 3057–3062.
- Chen,Q., and Q. Gu 2007. “Analysis of Quantity Uncertainty of Returns on Remanufacturing Logistic Network Using a Stochastic Programming Model.” In Proceedings of IEEE International Conference on Control and Automation, China, 3073–3077.
- Cheng, L. 2009. “Design for the Reverse Logistics Network Based on the Reverse Logistics Center Location.” In Proceedings of International Forum on Information Technology and Application, China, 457–459.
- Chen, Y. W., L. C. Wang, A. Wang, and T. L. Chen. 2016. “A Particle Swarm Approach for Optimizing a Multi-Stage Closed Loop Supply Chain for the Solar Cell Industry.” Robotics and Computer-Integrated Manufacturing 43: 111–123.
- Choudhary, A., S. Sarkar, S. Settur, and M. K. Tiwari. 2015. “A Carbon Market Sensitive Optimization Model for Integrated Forward-Reverse Logistics.” International Journal of Production Economics 164: 433–444.10.1016/j.ijpe.2014.08.015
- Chu, L. K., Y. Shi, S. Lin, D. Sculli, and J. Ni. 2010. “Fuzzy Chance-Constrained Programming Model for a Multi-Echelon Reverse Logistics Network for Household Appliances.” Journal of the Operational Research Society 61: 551–560.10.1057/jors.2008.162
- Costa, A. M., P. M. França, and C. L. Lyra Filho. 2011. “Two-Level Network Design with Intermediate Facilities: An Application to Electrical Distribution Systems.” Omega 39: 3–13.10.1016/j.omega.2010.01.005
- Costantino, N., M. Dotoli, M. Falagario, and F. Sciancalepore 2012. “Fuzzy Network Design of Sustainable Supply Chains.” In Proceedings of the 14th IFAC Symposium on Information Control Problems in Manufacturing Bucharest, 23–25 May 2012, Romania.
- Cruz-Rivera, R., and J. Ertel. 2009. “Reverse Logistics Network Design for the Collection of End-of-Life Vehicles in Mexico.” European Journal of Operational Research 196: 930–939.10.1016/j.ejor.2008.04.041
- Dai, Z., and X. Zheng. 2015. “Design of Close-Loop Supply Chain Network Under Uncertainty Using Hybrid Genetic Algorithm: A Fuzzy And Chance-Constrained Programming Model.” Computers and Industrial Engineering 88: 444–457.10.1016/j.cie.2015.08.004
- Darbari, J. D., V. Agarwal, and K. Chaudhary 2015. “Multi-Criteria Decision Approach for a Sustainable Reverse Logistics Network Under Fuzzy Environment.” P.C.Jha 978-1-4799-6065-1/15, IEEE.
- Das, K., and A. Chowdhury. 2012. “Designing a Reverse Logistics Network for Optimal Collection, Recovery and Quality-Based Product-Mix Planning.” International Journal of Production Economics 135: 209–221.10.1016/j.ijpe.2011.07.010
- Dat, L. K., Doan Thi Truc Linh, S. Y. Chou, and V. F. Yu. 2012. “Optimizing Reverse Logistic Costs for Recycling End-of-Life Electrical and Electronic Products.” Expert Systems with Applications 39: 6380–6387.10.1016/j.eswa.2011.12.031
- De Brito, M. P., and R. Dekker. 2004. “A Framework for Reverse Logistics.” In Reverse Logistics, Quantitative Models for Closed-Loop Supply Chains, edited by R. Dekker, K. Inderfurth, L. N. van Wassenhove, and M. Fleischmann. Berlin Heidelberg: Springer-Verlag.
- De Rosa, V., M. Gebhard, E. Hartmann, and J. Wollenweber. 2013. “Robust Sustainable Bi-Directional Logistics Network Design Under Uncertainty.” International Journal of Production Economics 145: 184–198.10.1016/j.ijpe.2013.04.033
- Dehghanian, F., and S. Mansour. 2009. “Designing Sustainable Recovery Network of End-of-Life Products Using Genetic Algorithm.” Resources, Conservation and Recycling 53: 559–570.10.1016/j.resconrec.2009.04.007
- Demirel, N. Ö., and H. Gökçen. 2008. “A Mixed Integer Programming Model for Remanufacturing in Reverse Logistics Environment.” International Journal of Advanced Manufacturing Technology 39: 197–206.
- Demirel, E., N. Demirel, and H. Gökçen. 2016. “A Mixed Integer Linear Programming Model to Optimize Reverse Logistics Activities of End-of-Life Vehicles in Turkey.” Journal of Cleaner Production 112: 2101–2113.10.1016/j.jclepro.2014.10.079
- Di, W. M., and M. J. Wang 2009. “Optimal Design of Manufacturing/Remanufacturing Logistics Network Based on Uncertain Programming.” In Proceedings of International Conference on Management Science and Engineering, China, 867–873.
- Diabat, A., T. Abdallah, A. Al-Refaie, D. Svetinovis, and K. Govindan 2013. “Strategic Closed-Loop Facility Location Problem with Carbon Market Trading.” IEEE Transactıons on Engineering Management, 60.
- Diabat, A., D. Kannan, M. Kaliyan, and D. Svetinovic. 2013. “An Optimization Model for Product Returns Using Genetic Algorithms and Artificial Immune System.” Resources, Conservation and Recycling 74: 156–169.10.1016/j.resconrec.2012.12.010
- Ding, S. 2010a. “Logistics Network Design and Optimization Based on Fuzzy Adaptive Differential Evolution Algorithm.” In Proceedings of International Conference on Biomedical Engineering and Computer Science (ICBECS), China, 1064–1068.
- Ding, S. 2010b. “Logistics Network Design Optimization Based on Differential Evolution Algorithm.” In Proceedings of International Conference on Logistics Systems and Intelligent Management. China: 1064–1068.
- Dongai, W., W. Guo, and K. Chen 2009. “The Design of Reverse Logistics Network Based on Remanufacturing for a Diesel Engine Enterprise.” In Proceedings of International Conference on Management and Service Science, China, 1–4.
- Dowlatshahi, S. 2010. “A Cost-Benefit Analysis for the Design and Implementation of Reverse Logistics Systems, Case Studies Approach.” International Journal of Production Research 48: 1361–1380.10.1080/00207540802552642
- Du, F., and G. Evans. 2008. “A Bi-Objective Reverse Logistics Network Analysis for Post-Sale Service.” Computers and Operations Research 35: 2617–2634.10.1016/j.cor.2006.12.020
- El Sayed, M., N. Afia, and A. El-Kharbotly. 2010. “A Stochastic Model for Forward-Reverse Logistics Network Design Under Risk.” Computers and Industrial Engineering 58: 423–431.10.1016/j.cie.2008.09.040
- Elbadrawy, R., A. F. A. Moneim, and M. N. Fors. 2015. “E-Waste Reverse Logistic Optimization in Egypt.” 978-1-4799-6065-1/15, IEEE.
- Elkington, J. 2004. Enter the Triple Bottom Line, Does It All Add Up?. Routledge: London.
- Ellili, W., M. Samet, and A. Kachouri. 2013. “Neural Networks for Optimized Reverse Logistics Network: If Collection is End of Life.” In 14th international conference on Sciences and Techniques of Automatic control and computer engineering- STA’2013, Sousse, Tunisia, December 20–22, 2013.
- Ene, S., and N. Öztürk. 2014. “Open Loop Reverse Supply Chain Network Design.” 2nd World Conference On Business, Economics and Management- WCBEM 2013.
- Ene, S., and N. Öztürk. 2015. “Network Modeling for Reverse Flows of End-of-Life Vehicles.” Waste Management 38: 284–296.10.1016/j.wasman.2015.01.007
- Entezaminia, A., M. Heydari, and D. Rahmani. 2016. “A Multi-Objective Model for Multi-Product Multi-Site Aggregate Production Planning in a Green Supply Chain: Considering Collection and Recycling Centers.” Journal of Manufacturing Systems 40: 63–75.10.1016/j.jmsy.2016.06.004
- EPA. 2014. Overview of Greenhouse Gases. Accessed March 10. http://www.epa.gov/climatechange/ghgemissions/gases/co2.html
- Eskandarpour, M., N. Nikbakhsh, and H. Zegordi. 2013. “Variable Neighborhood Search for the Bi Objective Post-Sales Network Design Problem, a Fitness Landscape Analysis Approach.” Computers and OperationsResearch 52: 300–314.
- Eskandarpour, M., Seyed Hessameddin Zegordi, and Ehsan Nikbakhsh. 2013. “A Parallel Variable Neighborhood Search for the Multi-Objective Sustainable Post-Sales Network Design Problem.” International Journal of Production Economics 145: 117–131.10.1016/j.ijpe.2012.10.013
- European Parliament and of the Council. (2002). Directive 2002/95/EC of the European Parliament and of the Council of 27 January 2003 on the Restriction of the Use of Certain Hazardous Substances in Electrical and Electronic Equipment (RoHS), Official Journal L 037, 13/02/2003, 19–23, 2003.
- European Parliament and of the Council, (2002). Directive 2002/96/EC of the European Parliament and of the Council of 27 January 2003 on Waste Electrical and Electronic Equipment (WEEE). Joint Declaration of the European Parliament, the Council and the Commission Relating to Article 9, Official Journal L 037, 13/02/2003, 24–39, 2003.
- Evren, R., and F. Ülengin. 1992. Yönetimde Çok Amaçlı Karar Verme. İstanbul: İTÜ Yayınları.
- Fandel, G., and M. Stammen. 2004. “A General Model for Extended Strategic Supply Chain Management with Emphasis on Product Life Cycles Including Development and Recycling.” International Journal of Production Economics 89: 293–308.10.1016/S0925-5273(03)00198-1
- Fathi, M., A. Hasanzadeh, A. Raad, F. Dehghanian, and S. Mansour. 2009. “Using Compromised Method in Design of Reverse Logistics Network with Eco-Efficiency Consideration.” In Proceedings of IEEE International Conference on Industrial Engineering and Engineering Management, China, 1454–1458.
- Ferri, G. L., G. L. D. Diniz Chaves, and G. M. Ribeiro. 2015. “Reverse Logistics Network for Municipal Solid Waste Management: The Inclusion of Waste Pickers as a Brazilian Legal Requirement.” Waste Management 40: 173–191.10.1016/j.wasman.2015.02.036
- Fleischmann, M., J. Nunen, and B. Gräve. 2002. Integrating Closed-Loop Supply Chains and Spare Parts Management at IBM. Erim Report Series Research in Management. The Netherlands: Erasmus Research Institute of Management.
- Galveza, D., A. Rakotondranaivo, L. Morel, M. Camargo, and M. Fick. 2015. “Reverse Logistics Network Design for a Biogas Plant: An Approach Based on MILP Optimization and Analytical Hierarchical Process (AHP).” Journal of Manufacturing Systems 37: 616–623.10.1016/j.jmsy.2014.12.005
- Gao, Y., B. Chen, and J. Wang. 2013. “Product Recycling Network Design with Recovery Rate Taken into Consideration Under Fuzzy Environment.” In Proceedings of Control and Decision Conference (CCDC), China, 414–418.
- Ge, X. Q., Z. Y. Tian, and J. Zhang. 2009. “Designing Three-Stage Reverse Logistics Network with Incentive.” In Proceedings of International Conference on Electronic Commerce and Business Intelligence, China, 213–216.
- Ghadge, A., Q. Yang, N. Caldwell, C. König, and M. K. Tiwari. 2016. “Facility Location for a Closed-Loop Distribution Network: A Hybrid Approach.” International Journal of Retail and Distribution Management 44: 9.
- Giacchetta, G., M. Leporini, and B. Marchetti. 2013. “Evaluation of the Environmental Benefits of New High Value Process for the Management of the End of Life of Thin Film Photovoltaic Modules.” Journal of Cleaner Production 51: 214–224.10.1016/j.jclepro.2013.01.022
- Gobebiewski, Bronisław, J. Trajer, M. Jaros, and R. Winiczenko. 2013. “Modelling of the Location of Vehicle Recycling Facilities: A Case Study in Poland.” Resources, Conservation and Recycling 80: 10–20.10.1016/j.resconrec.2013.07.005
- Gończ, E., U. Skirke, H. Kleizen, and M. Barber. 2007. “Increasing the Rate of Sustainable Change, a Call for a Redefinition of the Concept and the Model for its Implementation.” Journal of Cleaner Production 15: 525–537.
- Gomes, M. I., A. P. B. Barbosa-Povoa, and A. Q. Novais. 2011. “Modelling a Recovery Network for WEEE: A Case Study in Portugal.” Waste Management 31: 1645–1660.10.1016/j.wasman.2011.02.023
- Gottinger, H. W. 1988. “A Computational Model for Solid Waste Management with Application.” European Journal of Operational Research 35: 350–364.10.1016/0377-2217(88)90225-1
- Govindan, K., P. Paam, and A. R. Abtahi. 2016. “A Fuzzy Multi-Objective Optimization Model for Sustainable Reverse Logistics Network Design.” Ecological Indicators 67: 753–768.10.1016/j.ecolind.2016.03.017
- Graupe, D. 2007. Principles of Artificial Neural Networks. 2nd ed. Singapore: World Scientific Publishing.10.1142/ASCAS
- Guide, V. D. R., and L. N. Van Wassenhove. 2001. “Managing Product Returns for Remanufacturing.” Production and Operations Management 10 (2): 142–155.
- Guo, H., and W. Dai. 2011. “Research on Remanufacturing Reverse Logistics Network Optimization Under Uncertain Recycling Number.” Advances in Intelligent and Soft Computing 106: 691–695.10.1007/978-3-642-23753-9
- Guranowska, A. M. 2011. “End of Life Vehicles Recycling Network Design.” Journal of KONES Powertrain and Transport 18: 261–268.
- Hanafi, J., S. Kara, and H. Kaebernick. 2007. “Generating Fuzzy Coloured Petri Net Forecasting Model to Predict the Return of Products.” IEEE International Symposium on Electronics and the Environment: 245–250).
- Harraz, N. A., and N. M. Galal. 2011. “Design of Sustainable End-of-Life Vehicle Recovery Network in Egypt.” Ain Shams Engineering Journal 2: 211–219.10.1016/j.asej.2011.09.006
- Harris, I., M. Naim, A. Palmer, A. Potter, and C. Mumford. 2011. “Assessing the Impact of Cost Optimization Based on Infrastructure Modelling on CO2 Emissions.” International Journal of Production Economics 131: 313–321.10.1016/j.ijpe.2010.03.005
- Hatefi, S. M., and F. Jolai. 2014. “Robust and Reliable Forward–Reverse Logistics Network Design Under Demand Uncertainty and Facility Disruptions.” Applied Mathematical Modelling 38: 2630–2647.10.1016/j.apm.2013.11.002
- He, B. 2011. “Bi-Objective Programming Model and Algorithm for Reverse Logistics Network Design Problem.” In Proceedings of Fourth International Joint Conference on Computational Sciences and Optimization, China, 1245–1249.10.1109/CSO.2011.20
- He, B., C. Yang, and M. M. Ren. 2007. “A Fuzzy Multi-Objective Programming for Optimization of Reverse Logistics for Solid Waste Through Genetic Algorithms.” In Proceedings of Fourth International Conference on Fuzzy Systems and Knowledge Discovery, China, 416–420.10.1109/FSKD.2007.37
- Hernández-Hernández, H. J., J. R. Montoya-Torres, and F. Niebles-Atencio. 2014. “Design of Multi-Product/Multi-Period Closed-Loop Reverse Logistics Network Using a Genetic Algorithm.” In Computational Intelligence in Production and Logistics Systems (CIPLS), 2014 IEEE Symposium.
- Hess, J. D., and G. E. Mayhew. 1997. “Modeling Merchandise Returns in Direct Marketing.” Journal of Direct Marketing 11 (2): 20–35.10.1002/(ISSN)1522-7138
- Huang, M., P. Yi, L. Guo, and T. Shi. 2016. “A Modal Interval Based Genetic Algorithm for Closed-loop Supply Chain Network Design under Uncertainty.” IFAC-PapersOnLine 49: 616–621.10.1016/j.ifacol.2016.07.743
- Huo, H., and J. Wang. 2011. “Study on Network Model of Reverse Logistics Based on Used Appliances.” In Proceedings of International Conference on Management Science and Industrial Engineering (MSIE), China, 624–628.
- Ikhlayel, M. 2016. “Differences of Methods to Estimate Generation of Waste Electrical and Electronic Equipment for Developing Countries: Jordan as a Case Study.” Resources, Conservation and Recycling 108: 134–139.10.1016/j.resconrec.2016.01.015
- Jayaraman, V., R. A. Patterson, and E. Rolland. 2003. “The Design of Reverse Distribution Networks: Models and Solution Procedures.” European Journal of Operational Research 150: 128–149.10.1016/S0377-2217(02)00497-6
- Jeihoonian, M., M. K. Zanjani, and M. Gendreau. 2016a. “Closed-Loop Supply Chain Network Design Under Uncertain Quality Status: Case of Durable Products.” International Journal of Production Economics 183: 470–486.
- Jeihoonian, M., M. K. Kazemi Zanjani, and M. Gendreau. 2016b. “Accelerating Benders Decomposition for Closed-Loop Supply Chain Network Design: Case of Used Durable Products with Different Quality Levels.” European Journal of Operational Research 251: 830–845.10.1016/j.ejor.2015.12.052
- Jia, H., J. Xu, and Z. Sun 2010. “An Optimization Model for Closed-Loop Logistic System with Uncertain Demands.” In Proceedings of 29th Chinese Control Conference, China: 1201–1206.
- Jianhua, J., and G. Qiaolun 2006. “An Operational Model of Closed-Loop Logistics Based on the End-Customer’s Willingness.” In Proceedings of IEEE International Conference on Service Operations and Logistics and Informatics, China, 603–608.
- Jiawang, X., Z. Yunlong and J. Bo. 2010. “A Dynamic Operation Model for a Class of Closed-Loop Logistic System.” International Conference on Intelligent Computation Technology and Automation, China, 80–83.
- Jindal, A., K. S. Sangwan, and S. Saxena. 2015. “Network Design and Optimization for Multi-product, Multi-time, Multi-echelon Closed-loop Supply Chain under Uncertainty.” Procedia CIRP 29: 656–661.10.1016/j.procir.2015.01.024
- John, S. T., and R. Sridharan. 2015. “Modelling and Analysis of Network Design for a Reverse Supply Chain.” Journal of Manufacturing Technology Management 26 (6): 853–867.10.1108/JMTM-03-2014-0035
- Kadambala, D. K., N. Subramanian, M. K. Tiwari, M. Abdulrahman, and C. Liu. 2016. “Closed Loop Supply Chain Networks: Designs for Energy and Time Value Efficiency.” International Journal of Production Economics 183: 382–393.
- Kafa, N., Y. Hani, and A. Mhamedi. 2015. “An Integrated Sustainable Partner Selection Approach with Closed-Loop Supply Chain Network Configuration.” IFAC-PapersOnLine 48: 1840–1845.10.1016/j.ifacol.2015.06.354
- Kalaitzidou, M. A., P. Longinidis, and M. C. Georgiadis. 2015. “Optimal Design of Closed-Loop Supply Chain Networks with Multifunctional Nodes.” Computers and Chemical Engineering 80: 73–91.10.1016/j.compchemeng.2015.05.009
- Kammoun, A., M. Benaissa, and H. Chabchoub. 2013. “Planning Model in Reverse logistic.” Proceedings of International Conference on Advanced Logistics and Transport: 446–451.
- Kannan, D., A. Diabat, M. Alrefaei, K. Govindan, and G. Yong. 2012. “A Carbon Footprint Based Reverse Logistics Network Design Model.” Resources, Conservation and Recycling 67: 75–79.10.1016/j.resconrec.2012.03.005
- Kara, S. S., and S. Onut. 2010. “A Two-Stage Stochastic and Robust Programming Approach to Strategic Planning of a Reverse Supply Network: The Case of Paper Recycling.” Expert Systems with Applications 37: 6129–6137.10.1016/j.eswa.2010.02.116
- Kaya, O., and B. Urek. 2016. “A Mixed Integer Nonlinear Programming Model and Heuristic Solutions for Location, Inventory and Pricing Decisions in a Closed Loop Supply Chain.” Computers and Operations Research 65: 93–103.10.1016/j.cor.2015.07.005
- Keyvanshokooh, E., M. Fattahi, S. M. Seyed-Hosseini, and R. Tavakkoli-Moghaddam. 2013. “A Dynamic Pricing Approach for Returned Products in Integrated Forward/Reverse Logistics Network Design.” Applied Mathematical Modelling: 10182–10202.10.1016/j.apm.2013.05.042
- Keyvanshokooh, E., M. Fattahi, S. M. Seyed-Hosseini, and R. Tavakkoli-Moghaddam. 2015. “A Dynamic Pricing Approach for Returned Products in Integrated Forward/Reverse Logistics Network Design.” Applied Mathematical Modelling 37: 10182–10202.
- Keyvanshokooh, E., S. M. Ryan, and E. Kabir. 2016. “Hybrid Robust and Stochastic Optimization for Closed-Loop Supply Chain Network Design Using Accelerated Benders Decomposition.” European Journal of Operational Research 249: 76–92.10.1016/j.ejor.2015.08.028
- Khatami, M., M. Mahootchi, and R. Z. Farahani. 2015. “Benders’ Decomposition for Concurrent Redesign of Forward and Closed-Loop Supply Chain Network with Demand and Return Uncertainties.” Transportation Research Part E: Logistics and Transportation Review 79: 1–21.10.1016/j.tre.2015.03.003
- Kilic, H. S., U. Cebeci, and M. B. Ayhan. 2015. “Reverse Logistics System Design for the Waste of Electrical and Electronic Equipment (WEEE) in Turkey.” Resources, Conservation and Recycling 95: 120–132.10.1016/j.resconrec.2014.12.010
- Kim, J. S., and D. H. Lee. 2013. “A Restricted Dynamic Model for Refuse Collection Network Design in Reverse Logistics.” Computers and Industrial Engineering 66: 1131–1137.10.1016/j.cie.2013.08.001
- Kisomi, M. S., M. Solimanpur, and A. Doniavi. 2016. “An Integrated Supply Chain Configuration Model and Procurement Management Under Uncertainty: A Set-Based Robust Optimization Methodology.” Applied Mathematical Modelling 40: 7928–7947.10.1016/j.apm.2016.03.047
- Kizilboga, G., G. Mandil, M. E. Genevois, and P. Zwolinski. 2013. “Remanufacturing Network Design Modeling, a Case of Diesel Particulate Filter.” In Proceedings of 2nd International Through-life Engineering Services Conference, Procedia CIRP 11, 163–168.
- Klausner, M., and C. Hendrickson. 2000. “Reverse-Logistics Strategy for Product Take-Back.” Interfaces 30 (3): 156–165.10.1287/inte.30.3.156.11657
- Klein, M. W., S. Schuh, and R. K. Triest. 2002. “Job Creation, Job Destruction and the Real Exchange Rate.” Journal of International Economics 59: 239–265.
- Krikke, H. R. 2011. “Impact of Closed-Loop Network Configurations on Carbon Footprints: A Case Study in Copiers.” Resources, Conservation and Recycling 55: 1196–1205.10.1016/j.resconrec.2011.07.001
- Krikke, H. R., A. van Harten, and P. C. Schuur. 1999. “Business Case Océ: Reverse Logistic Network Re-Design for Copiers.” OR Spectrum 21: 381–409.10.1007/s002910050095
- Kuruüzüm, A. 1998. Karar Destek Sistemlerinde Çok Amaçli Yöntemler. Antalya: Akdeniz Üniversitesi Yayınları.
- Kusumastuti, R. D., R. Piplani, and G. H. Lim, 2004. “An Approach to Design Reverse Logistics Networks for Product Recovery.” In Proceedings of International Engineering Management Conference, China, 1239–1243.
- Lee, D. H., and M. Dong. 2008. “A Heuristic Approach to Logistics Network Design for End-of- Lease Computer Products Recovery.” Transportation Research Part E: Logistics and Transportation Review 44: 455–474.10.1016/j.tre.2006.11.003
- Lee, D. H., and M. Dong. 2009. “Dynamic Network Design for Reverse Logistics Operations Under Uncertainty.” Transportation Research Part E: Logistics and Transportation Review 45: 61–71.10.1016/j.tre.2008.08.002
- Lee, J. E., M. Gen, and K. G. Rhee. 2009. “Network Model and Optimization of Reverse Logistics by Hybrid Genetic Algorithm.” Computers and Industrial Engineering 56: 951–964.10.1016/j.cie.2008.09.021
- Li, N. 2011. “Selection of Re-Manufacturing Plant’s Location Based on Genetic Algorithm.” In Proceedings of International Conference on Management Science and Industrial Engineering, China, 1010–1012.
- Li, R. C., and T. J. C. Tee. 2012. “A Reverse Logistics Model for Recovery Options of E-waste Considering the Integration of the Formal and Informal Waste Sectors.” Procedia - Social and Behavioral Sciences 40: 788–816.10.1016/j.sbspro.2012.03.266
- Li, S., N. Wang, and T. Z. Jia, and H. Liang. 2016. May 2016. “Multi Objective Optimization for Multi Period Reverse Logistics Network Design.” IEEE Transactions on Engineering Management 63: 2.
- Lieckens, K., and N. Vandaele. 2007. “Reverse Logistics Network Design with Stochastic Lead Times.” Computers and Operations Research 34: 395–416.10.1016/j.cor.2005.03.006
- Lieckens, K., P. Colen, and M. Lambrecht. 2013. “Optimization of a Stochastic Remanufacturing Network with an Exchange Option.” Decision Support Systems 54: 1548–1557.10.1016/j.dss.2012.05.057
- Lili, R., and H. Yong. 2010. “Optimization of Forward/Reverse Logistics Networks for Electronic Products.” Proceedings of International Conference of Information Science and Management: 536–538.
- Lin, Z. K., L. L. Gao, and X. Guo. 2009. “The Reverse Logistics Network Design of Drugs.” In Proceedings of Ninth International Conference on Machine Learning and Cybernetics, Qingdao, 86–90.
- Ling, Z., W. Zhengxiao, P. Xiaohong, and D. Tianyang. 2010. “Optimization Model for Remanufacturing Logistics Network with Fuzzy Parameters.” In Proceedings of International Conference on Measuring Technology and Mechatronics Automation, China 2: 849–852.
- Listeş, O. 2007. “A Generic Stochastic Model for Supply-and-Return Network Design.” Computers and Operations Research 34: 417–442.10.1016/j.cor.2005.03.007
- Liu, X., J. Guo, and, C. Liang, 2015. “ Joint Design Model of Multi-Period Reverse Logistics Network with the Consideration of Carbon Emissions for E-Commerce Enterprises.” IEEE 12th International Conference on e-Business Engineering.
- Lo, C. C., H. M. Chen, and H. L. Huang, 2008. “A Multi-Objective Reverse Logistics Network for Product Returns.” In Proceedings of IEEE International Conference on Industrial Engineering and Engineering Management, China, 1634–1638.
- Louwers, D., B. J. Kip, E. Peters, F. Souren, and S. D. P. Flapper. 1999. “A Facility Location Allocation Model for Reusing Carpet Materials.” Computers and Industrial Engineering 36: 855–869.10.1016/S0360-8352(99)00168-0
- Lu, T., and X. Zhao, 2010. “Reverse Logistics Network Design with Consideration of Forward And Reverse Facility Integration.” In Proceedings of International Conference on E-Business and E-Government, China, 3271–3274.
- Lu, Y., P. Lu, and L. Liang, 2008. “Multi-Objective Optimization of Reverse Logistics Network Based on Random Weights and Genetic Algorithm.” In Proceedings of International Conference on Networking, Sensing and Control, China, 1196–1200.
- Lu, Z., and N. Bostel. 2007. “A Facility Location Model for Logistics Systems Including Reverse Flows: The Case of Remanufacturing Activities.” Computers and Operations Research 34: 299–323.10.1016/j.cor.2005.03.002
- Lundin, J. F. 2011. “Redesigning a Closed-Loop Supply Chain Exposed to Risks.” International Journal of Production Economics 140: 596–603.
- Ma, R., L. Yao, M. Jin, P. Ren, and Z. Lv. 2016. “Robust Environmental Closed-Loop Supply Chain Design Under Uncertainty.” Chaos, Solitons and Fractals 89: 195–202.10.1016/j.chaos.2015.10.028
- Mahmoudzadeh, M., S. Mansour, and B. A. Karimi, 2011. “Decentralized Reverse Logistics Network for End of Life Vehicles From Third Party Provider Perspective.” In Proceedings of 2nd International Conference on Environmental Science and Technology, China, 2338–2342.
- Marler, R. T., and J. S. Arora. 2004. “Survey of Multi-Objective Optimization Methods for Engineering.” Structural and Multidisciplinary Optimization 26: 369–395.10.1007/s00158-003-0368-6
- Melo, M. T., S. Nickel, and F. Saldanha-da-Gama. 2009. “Facility Location and Supply Chain Management – A Review.” European Journal of Operational Research 196: 401–412.10.1016/j.ejor.2008.05.007
- Min, H., and H. J. Ko. 2008. “The Dynamic Design of a Reverse Logistics Network from the Perspective of Third-Party Logistics Service Providers.” International Journal of Production Economics 113: 176–192.10.1016/j.ijpe.2007.01.017
- Min, H., H. J. Jeung Ko, and C. S. Seong Ko. 2006. “A Genetic Algorithm Approach to Developing the Multi-Echelon Reverse Logistics Network for Product Returns.” Omega 34: 56–69.10.1016/j.omega.2004.07.025
- Ministry of Environment and Urban Planning. 2008. Atık Elektrik Elektronik Eşyaların (AEEE) Kontrolü Ve Yönetimi Yönetmeliği. Çevre Yönetimi Genel Müdürlüğü Atık Yönetimi Dairesi Başkanlığı.
- Minli, X. and D. Yu, 2010. “Optimization Model for Manufacturing/Remanufacturing Logistics Network of Disassembled Products.” In Proceedings of International Conference on Logistics Systems and Intelligent Management, China, 1509–1512.
- Mounir, B., K. Afifa, and C. Habib. 2011. “Facility Location Model for Reverse Logistics.” Advances in Production Engineering and Management 6: 37–44.
- Mukta, P., and U. A. Kumar. 2009. “Neural Networks and Statistical Techniques: A Review of Applications.” Expert Systems with Applications 36: 2–17.
- Mutha, A., and S. Pokharel. 2009. “Strategic Network Design for Reverse Logistics and Remanufacturing Using New and Old Product Modules.” Computers and Industrial Engineering 56: 334–346.10.1016/j.cie.2008.06.006
- Neto, J. Q. F., J. M. Bloemhof-Ruwaard, J. A. E. E. van Nunen, and E. van Heck. 2008. “Designing and Evaluating Sustainable Logistics Networks.” International Journal of Production Economics 111: 195–208.10.1016/j.ijpe.2006.10.014
- Niknejad, A., and D. Petrovic. 2014. “Optimisation of Integrated Reverse Logistics Networks with Different Product Recovery Routes.” European Journal of Operational Research 238: 143–154.10.1016/j.ejor.2014.03.034
- Nikolaou, I. E., I. E. Konstantinos, and S. Allan. 2012. “A Reverse Logistics Social Responsibility Evaluation Framework Based on the Triple Bottom Line Approach.” Journal of Cleaner Production 56: 173–184.
- Ossenbruggen, P. J., and P. C. Ossenbruggen. 1992. “Swap: A Computer Package for Solid Waste Management.” Computers, Environment and Urban Systems 16: 83–100.10.1016/0198-9715(92)90021-I
- Özceylan, E., T. Paksoy, and T. Bektaş. 2014. “Modeling and Optimizing the Integrated Problem of Closed-Loop Supply Chain Network Design and Disassembly Line Balancing.” Transportation Research Part E: Logistics and Transportation Review 61: 142–164.10.1016/j.tre.2013.11.001
- Pati, R. K., P. Vrat, and P. Kumar. 2008. “A Goal Programming Model for Paper Recycling System.” Omega 36: 405–417.10.1016/j.omega.2006.04.014
- Pedram, A., N. B. Yusoff, O. E. Udoncy, A. B. Mahat, P. Pedram, and A. Babalola. 2016. “Integrated Forward and Reverse Supply Chain: A Tire Case Study.” Waste Management 60: 460–470.
- Peng, P., Y. Zhang, and G. Pang, 2011. “The Optimal Design Model of Logistics Network for Scrap Copper Recycling.” In Proceedings of 10th International Symposium on Distributed Computing and Applications to Business, Engineering and Science, China, 116–119.
- Piplani, R., and A. Saraswat. 2011. “Robust Optimization Approach to the Design of Service Networks for Reverse Logistics.” International Journal of Production Research 50: 1424–1437.
- Pishvaee, M. S., and, J. Razmi. 2011. “Environmental Supply Chain Network Design Using Multi-Objective Fuzzy Mathematical Programming.” Applied Mathematical Modelling 36: 3433–3446.
- Pishvaee, M. S., M. Rabbani, and S. Y. Torabi, 2011. “A Robust Optimization Approach to Closed-Loop Supply Chain Network Design Under Uncertainty.” Applied Mathematical Modelling 35: 637–649.10.1016/j.apm.2010.07.013
- Pishvaee, M. S., R. Z. Farahani, and W. Dullaert. 2010. “A Memetic Algorithm for Bi-Objective Integrated Forward/Reverse Logistics Network Design.” Computers and Operations Research 37: 1100–1112.10.1016/j.cor.2009.09.018
- Pishvaee, M. S., F. Jolai, and J. Razmi. 2010. “A Stochastic Optimization Model for Integrated Forward/Reverse Logistics Network Design.” Journal of Manufacturing Systems 28: 107–114.
- Pishvaee, M. S., K. Kianfar, and B. Karimi. 2010. “Reverse Logistics Network Design Using Simulated Annealing.” The International Journal of Advanced Manufacturing Technology 47: 269–281.10.1007/s00170-009-2194-5
- Pochampally, K. K., and S. M. Gupta. 2008. “A Multiphase Fuzzy Logic Approach to Strategic Planning of a Reverse Supply Chain Network.” IEEE Transactions on Electronics Packaging Manufacturing 31: 72–82.10.1109/TEPM.2007.914229
- Pugh, M. P. 1993. “The Use of Mathematical Models in Evaluating Resource Recovery Options.” Resources. Conservation and Recycling 8: 91–101.10.1016/0921-3449(93)90021-7
- Qi, Z., and W. Hongcheng, 2008. “Research on Construction Mode of Recycling Network of Reverse Logistics of Automobile Enterprises.” In Proceedings of International Conference on Information Management, Innovation Management and Industrial Engineering, China, 36–40.
- Qian, X., Y. Han, and Q. Da, 2011. “Reverse Logistics Network Design Model Based on E-Commence.” In Proceedings of 2nd International Conference on Artificial Intelligence, Management Science and Electronic Commerce, China, 1486–1490.
- Qin, Z., and X. Ji. 2011. “Logistics Network Design for Product Recovery in Fuzzy Environment.” European Journal of Operational Research 202: 479–490.
- Ramezani, M., M. Bashiri, and R. T. Tavakkoli-Moghaddam. 2013. “A New Multi-Objective Stochastic Model for a Forward/Reverse Logistic Network Design with Responsiveness and Quality Level.” Applied Mathematical Modelling 37: 328–344.10.1016/j.apm.2012.02.032
- Ramezani, M., A. M. Kimiagari, B. Karimi, and T. H. Hejazi. 2014b. “Closed-Loop Supply Chain Network Design Under a Fuzzy Environment.” Knowledge-Based Systems 59: 108–120.10.1016/j.knosys.2014.01.016
- Rangwani, N., P. Subramanian, N. Ramkumar, and T. T. Narendran. 2011. “Heuristic for a Single Period Single Product Closed Loop Supply Chain.” International Journal of Enterprise Network Management 4: 311–324.10.1504/IJENM.2011.041159
- Realff, M. J., J. N. Ammons, and D. Newton. 1998. “Carpet Recycling, Determining the Reverse Production System Design.” The Journal of Polymer-Plastics Technology and Engineering 38: 547–567.
- REC (Regional Environment Center-Turkey) 2012. Regulatory Impact Assessment of EU Waste Electrical and Electronic Equipment (WEEE) Directive (2002/96/EC).
- Ren, C., and J. Ye. 2011. “Volume Removed - Publisher's Disclaimer.” Energy Procedia 13: 1–10380.10.1016/S1876-6102(14)00454-8
- Rogers, D., and R. Tibben-Lembke. 1999. Going Backwards: Reverse Logistics Trends and Practices. Pittsburgh: RLEC Press.
- Roghanian, E., and P. Pazhoheshfar. 2014. “An Optimization Model for Reverse Logistics Network Under Stochastic Environment by Using Genetic Algorithm.” Journal of Manufacturing Systems 33: 348–356.10.1016/j.jmsy.2014.02.007
- Sadjadi, S. J., Roya Soltani, and A. Eskandarpour. 2014. “Location Based Treatment Activities for End of Life Products Network Design Under Uncertainty by a Robust Multi-Objective Memetic-Based Heuristic Approach.” Applied Soft Computing 23: 215–226.10.1016/j.asoc.2014.06.028
- Sahyouni, K., R. C. Savaskan, and M. S. Daskin. 2007. “A Facility Location Model for Bidirectional Flows.” Transportation Science 41: 484–499.10.1287/trsc.1070.0215
- Salema, M. I. G., A. P. Póvoa, and A. Q. Novais. 2006. “A Warehouse-Based Design Model for Reverse Logistics.” Journal of the Operational Research Society 57: 615–629.10.1057/palgrave.jors.2602035
- Salema, M. I. G., A. P. Barbosa-Povoa, and A. Q. Novais. 2007. “An Optimization Model for the Design of a Capacitated Multi-Product Reverse Logistics Network with Uncertainty.” European Journal of Operational Research 179: 1063–1077.10.1016/j.ejor.2005.05.032
- Salema, M. I. G., A. P. Barbosa-Povoa, and A. Q. Novais. 2010. “Simultaneous Design And Planning of Supply Chains With Reverse Flows: A Generic Modelling Framework.” European Journal of Operational Research 203: 336–349.10.1016/j.ejor.2009.08.002
- Santibanez-Gonzalez, E. D. R., and A. Diabat. 2013. “Solving a Reverse Supply Chain Design Problem by Improved Benders Decomposition Schemes.” Computers and Industrial Engineering 66: 889–898.10.1016/j.cie.2013.09.005
- Sarkis, J. 2001. “Manufacturing’s Role in Corporate Environmental Sustainability–Concerns for the New Millennium.” International Journal of Operations and Production Management 21: 666–686.10.1108/01443570110390390
- Sasikumar, P., and A. N. Haq. 2011. “Integration of Closed Loop Distribution Supply Chain Network and 3PRLP Selection for the Case of Battery Recycling.” International Journal of Production Research 49: 3363–3385.10.1080/00207541003794876
- Sasikumar, P., G. Kannan, and A. N. Haq. 2010. “A Multi-Echelon Reverse Logistics Network Design for Product Recovery-A Case of Truck Tire Remanufacturing.” The International Journal of Advanced Manufacturing Technology 49: 1223–1234.10.1007/s00170-009-2470-4
- Schweiger, K., and R. Sahamie. 2013. “A Hybrid Tabu Search Approach for the Design of a Paper Recycling Network.” Transportation Research Part E: Logistics and Transportation Review 50: 98–119.10.1016/j.tre.2012.10.006
- Serrano, C., W. Aggoune-Mtalaa, and N. Sauer, 2013. “Dynamic Models for Green Logistic Networks Design.” 7th IFAC Conference on Manufacturing Modelling, Management, and Control, 19–21 June 2013. Saint Petersburg, Russia.
- Shi, L. H. 2009. “A Mixed Integer Linear Programming for Medical Waste Reverse Logistics Network Design.” In Proceedings of International Conference on Management Science and Engineering (16th), China, 1971–1975.
- Shi, L., H. Fan, P. Gao, and H. Zhang, 2009. “Network Model and Optimization of Medical Waste Reverse Logistics by Improved Genetic Algorithm.” In Proceedings of International Symposium on Intelligence Computation and Applications, China, 40–52.
- Shih, L. H. 2001. “Reverse Logistics System Planning for Recycling Electrical Appliances and Computers in Taiwan.” Resources, Conservation and Recycling 32: 55–72.10.1016/S0921-3449(00)00098-7
- Shrivastava, P., and S. Hart. 1995. “Creating Sustainable Corporations.” Business Strategy and the Environment 4: 154–165.10.1002/(ISSN)1099-0836
- Siri, S., I. T. Mendis, and C. Repetto, 2015. “The Facility Location Problem in a Reverse Logistic Network: Weeenmodels Project in the City of Genoa.” IEEE 18th International Conference on Intelligent Transportation Systems.
- Soleimani, H., and K. Govindan. 2014. “Reverse Logistics Network Design and Planning Utilizing Conditional Value at Risk.” European Journal of Operational Research 237: 487–497.10.1016/j.ejor.2014.02.030
- Soleimani, H., and G. Kannan. 2015. “A Hybrid Particle Swarm Optimization and Genetic Algorithm for Closed-Loop Supply Chain Network Design in Large-Scale Networks.” Applied Mathematical Modelling 39: 3990–4012.10.1016/j.apm.2014.12.016
- Song, C., S. T. Srimat, S. Murata, W. Pan, L. Sun, A. W. Scaroni, and J. N. Armor, 2002. “Effects of Pressure on CO2 Reforming of CH4 Over Ni/Na-y and Ni/Al2O3 Catalysts.” ACS Symposium Series CO2 Conversion and Utilization 809: 258–274.10.1021/symposium
- Spengler, T., H. Püchert, T. Penkuhn, and O. Rentz. 1997. “Environmental Integrated Production and Recycling Management.” European Journal of Operational Research 97: 308–326.10.1016/S0377-2217(96)00200-7
- Srivastava, S. K., and R. K. Srivastava. 2006. “Managing Product Returns for Reverse Logistics.” International Journal of Physical Distribution and Logistics Management 36: 524–546.10.1108/09600030610684962
- Srivastava, S. K. 2008a. “Network Design for Reverse Logistics.” Omega 36: 535–548.10.1016/j.omega.2006.11.012
- Srivastava, S. K. 2008b. “Value Recovery Network Design for Product Returns.” International Journal of Physical Distribution and Logistics Management 38: 311–331.10.1108/09600030810875409
- Subramanian, P., N. Ramkumar, T. T. Narendran, and K. Ganesh. 2013. “PRISM: Priority Based Simulated Annealing for a Closed Loop Supply Chain.” Applied Soft Computing 13 (2): 1121–1135.10.1016/j.asoc.2012.10.004
- Subulan, K., A. S. Taşan¸ and A. Baykasoğlu, 2015a. “Designing an Environmentally Conscious Tire Closed-Loop Supply Chain Network with Multiple Recovery Options using Interactive Fuzzy Goal Programming.” Applied Mathematical Modelling 39: 2661–2702.10.1016/j.apm.2014.11.004
- Subulan, K., A. S. Taşan¸ and A. Baykasoğlu, 2015b. “A Fuzzy Goal Programming Model to Strategic Planning Problem of a Lead/Acid Battery Closed-Loop Supply Chain.” Journal of Manufacturing Systems 37: 243–264.10.1016/j.jmsy.2014.09.001
- Talaei, M., B. F. Farhang Moghaddam, M. S. Pishvaee, A. Bozorgi-Amiri, and S. Gholamnejad. 2016. “A Robust Fuzzy Optimization Model for Carbon-Efficient Closed-Loop Supply Chain Network Design Problem: A Numerical Illustration in Electronics Industry.” Journal of Cleaner Production 113: 662–673.10.1016/j.jclepro.2015.10.074
- Tancrez, J. S., J. C. Lange, and P. Semal. 2012. “A Location-Inventory Model for Large Three-Level Supply Chains.” Transportation Research Part E: Logistics and Transportation Review 48: 485–502.10.1016/j.tre.2011.10.005
- Tang, Q., and F. Xie. 2007. “A Genetic Algorithm for Reverse Logistics Network Design.” In Proceedings of Third International Conference on Natural Computation, China 4: 277–281.
- Tasan, A. Serdar, and M. Gen. 2012. “A Genetic Algorithm Based Approach to Vehicle Routing Problem with Simultaneous Pick-Up and Deliveries.” Computers and Industrial Engineering 62: 755–761.10.1016/j.cie.2011.11.025
- Tian, Z. Y., C. H. Jin, and X. Q. Ge, 2009. “Designing Reverse Logistics Network for End-of-Life Vehicles Under Capacity Constraints.” In Proceedings of IEEE 16th International Conference on Industrial Engineering and Engineering Management, China, 1488–1491.
- Tuzkaya, G., Bahadır Gülsün, and Ş. Önsel. 2011. “A Methodology for the Strategic Design of Reverse Logistics Networks and its Application in the Turkish White Goods Industry.” International Journal of Production Research 49: 4543–4571.10.1080/00207543.2010.492804
- Ülkü, M. A. 2012. “Dare to Care: Shipment Consolidation Reduces Not Only Costs, But Also Environmental Damage.” International Journal of Production Economics 139: 438–446.10.1016/j.ijpe.2011.09.015
- Vahdani, B., R. Tavakkoli-Moghaddam, M. Modarres, and A. Baboli. 2012. “Reliable Design of a Forward/Reverse Logistics Network Under Uncertainty: A Robust-M/M/c Queuing Model.” Transportation Research Part E: Logistics and Transportation Review 48: 1152–1168.10.1016/j.tre.2012.06.002
- Vahdani, B., and M. Mohammadi. 2015. “A Bi-Objective Interval-Stochastic Robust Optimization Model for Designing Closed Loop Supply Chain Network with Multi-Priority Queuing System.” International Journal of Production Economics 170: 67–87.10.1016/j.ijpe.2015.08.020
- Vidovic, M., B. Dimitrijevic, B. Ratkovic, and V. Simic. 2011. “A Novel Covering Approach to Positioning ELV Collection Points.” Resources, Conservation and Recycling 57: 1–9.10.1016/j.resconrec.2011.09.013
- Wan, F., and Q. Zhang, 2008. “Research on Re-Use Reverse Logistics Network of Disused Household Appliances Based on Genetic Algorithm.” In Proceedings of International Conference on Intelligent Computation Technology and Automation, China, 135–139.
- Wan, Y., X. Liu, and F. Wang, 2014. “Models of Design and Optimization of Waste Air-Materials in Reverse Logistics Network.” In Proceedings of the 33rd Chinese Control Conference July 28–30, Nanjing.
- Wang, H. F., and H. W. Hsu. 2010. “A Closed-Loop Logistic Model with a Spanning-Tree Based Genetic Algorithm.” Computers and Operations Research 37: 376–389.10.1016/j.cor.2009.06.001
- Wang, X., and L. Zhao, 2009. “Network Design of Reverse Logistics Integrated with Forward Logistics.” In Proceedings of Asia-Pacific Power and Energy Engineering Conference, China, 1–4.
- Wang, Z., J. Yin, and W. Ma, 2008. “A Reverse Logistics Optimization Model for Hazardous Waste in the Perspective of Fuzzy Multi Objective Programming Theory.” In Proceedings of IEEE Congress on Evolutionary Computations, China, 1576–1580.
- Wang, Z., Y. Zou, H. Wang, and Z. Feng, 2010. “The Optimization of Reverse Supply Chain Supernetwork.” In Proceedings of International Conference on Logistics Systems and Intelligent Management, China, 659–663.
- Wolfer, S., H. Sander, and F. Gogol, 2011. “Reverse Logistics Network Design for Waste Electrical and Electronic Equipment (WEEE) in China, A Shanghai Case Study on Eco-Innovation In Industry.” In Proceedings of the 9th GLOBELICS International Conference on Creativity, Innovation and Economic Development, China.
- Wongthatsanekorn, W. 2009. “A Goal Programming Approach for Plastic Recycling System in Thailand.” World Academy of Science, Engineering and Technology 49: 513–518.
- World Commission on Environment and Development. 1987. Report of the World Commission on Environment and Development, Our Common Future.
- Wu, M. 2009. “The Research on Modeling and Optimizing of Remanufacturing Reverse Logistics Networks.” In Proceedings of 4th International Conference on Computer Scienceand Education, China, 660–664.
- Xanthopoulos, A., and E. Iakovou. 2009. “On the Optimal Design of the Disassembly and Recovery Processes.” Waste Management 29: 1702–1711.10.1016/j.wasman.2008.11.009
- Xiangru, M., and J. Hua, 2009. “The Construction of Reverse Logistics Network About the Reusable Packages.” In Proceedings of International Conference on Measuring Technology and Mechatronics Automation, China, 698–701.
- Xiangru, M. and S. Wei, 2009a. “Network Design Based on Closed-Loop Reverse Logistics Recycling.” In Proceedings of IITA International Conference on Control, Automation and Systems Engineering, China, 533–536.
- Xiangru, M. and, S. Wei, 2009b. “Open-Loop Network Design Based on Reverse Logistics Recycling.” In International Conference on Services Science, Management and Engineering, China, 209–212.
- Xiangru, M., and M. Xin, 2010. “Remanufacturing Network Analysis of Third-Party Reverse Logistics for Waste Household Appliances.” In Proceedings of International Conference on Management and Service Science, China, 1–3.
- Xiangru, M., Z. Yongjie, and S. Wei, 2009. “Network Construction on Reverse Logistics of Discarded And Used Household Appliances.” In Proceedings of International Conference on Environmental Science and Information Application Technology, China, 108–111.
- XiaoYan, Q., H. Yong, D. Qinli, and P. Stokes. 2012. “Reverse Logistics Network Design Model Based on e-Commerce.” International Journal of Organizational Analysis 20 (2): 251–261.10.1108/19348831211227864
- Xie, F. 2009. “Reverse Logistics Network Optimization by Genetic Algorithm.” In Proceedings of Fifth International Conference on Natural Computation, China, 403–407.
- Xiwang, G., L. Shixin, W. Dazhi, and H. Chunming, 2012. “An Improved Multi-Objective Scatter Search Approach for Solving Selective Disassembly Optimization Problem.” In Proceedings of the 31th Chinese Control Conference, 7703–7708.
- Xu, H., and E. Cao. 2016. “Closed-Loop Supply Chain Network Equilibrium Model and its Newton Method.” Kybernetes 45 (3): 393–410.10.1108/K-08-2013-0179
- Xu, J., and Y. Zhu, 2010. “Modeling for the Operation of Closed-Loop Supply Chain with Remanufacturing.” In Proceedings of International Conference on Logistics Systems and Intelligent Management, China, 69–73.
- Yan, B., and D. Lee, 2008. “Application of Genetic Algorithm on Remanufacturing Reverse Logistics Network Model.” In Proceedings of International Seminar on Future BioMedical Information Engineering, China, 126–129.
- Yan, B., F. Wang, P. Shi, and B. Huang. 2013. “A Dynamic Location Model of Remanufacturing Reverse Logistics Network.” Proceedings of International Conference on Service Science: 244–249.
- Yi, P., M. Huang, L. Guo, and T. Shi. 2016. “A Retailer Oriented Closed-Loop Supply Chain Network Design for End of Life Construction Machinery Remanufacturing.” Journal of Cleaner Production 124: 191–203.10.1016/j.jclepro.2016.02.070
- Yu, Q., and Z. G. Wei. 2009. “Improved Niche Pso to The Reverse Logistics Network Design Model.” Proceedings of International Colloquium on Computing, Communication, Control, and Management, China, 513–516.
- Yu, H., and W. D. Solvang, 2013. “A Reverse Logistics Network Design Model for Sustainable Treatment Of Multi-Sourced Waste of Electrical And Electronic Equipment (WEEE).” 4th IEEE International Conference on Cognitive Infocommunications, December 2–5, Budapest, Hungary.
- Yu, H., and W. D. Solvang. 2016a. “A Stochastic Programming Approach with Improved Multi-Criteria Scenario-Based Solution Method for Sustainable Reverse Logistics Design of Waste Electrical and Electronic Equipment (WEEE).” Sustainability 8 (12): 1331.10.3390/su8121331
- Yu, H., and W. D. Solvang. 2016b. “A General Reverse Logistics Network Design Model for Product Reuse and Recycling with Environmental Considerations.” The International Journal of Advanced Manufacturing Technology 87: 2693–2711.10.1007/s00170-016-8612-6
- Yushi, G., H. Zuqing, and Y. Yuxiang, 2015. “Waste Electrical Recycling Network Optimum Design Under the Carbon Cap Constraint.” 11th International Conference on Computational Intelligence and Security.
- Zarei, M., S. Mansour, A. H. Kashan, and B. Karimi, 2010. “Designing a Reverse Logistics Network for End-of-Life Vehicles Recovery.” Hindawi Publishing Corporation Mathematical Problems in Engineering art. No. 649028.
- Zeballos, L. J., M. I. Gomes, A. P. Barbosa-Povoa, and A. Q. Novais. 2012. “Addressing the Uncertain Quality and Quantity of Returns in Closed-Loop Supply Chains.” Computers and Chemical Engineering 47: 237–247.10.1016/j.compchemeng.2012.06.034
- Zegordi, S. H., M. Eskandarpour, and E. Nikbakhsh, 2011. “A Novel Bi-Objective Multi-Product Post-Sales Reverse Logistics Network Design Model.” In Proceedings of World Congress on Engineering, China.
- Zhalechian, M., R. Tavakkoli-Moghaddam, B. Zahiri, and M. Mohammadi. 2016. “Sustainable Design of a Closed-Loop Location-Routing-Inventory Supply Chain Network Under Mixed Uncertainty.” Transportation Research Part E: Logistics and Transportation Review 89: 182–214.10.1016/j.tre.2016.02.011
- Zhang, G., and S. H. Amin, 2011. “Network Design of a Closed-Loop Supply Chain with Uncertain Demand and Return.” International Conference on Service Operations, Logistics and Informatics, 376–379.
- Zhang, G. and S. H. Amin, 2011. “Network Design of a Closed-Loop Supply Chain with Uncertain Demand and return.” In Proceedings of International Conference on Service Operations, Logistics and Informatics, China, 376–379.
- Zhang, L., Z. Wang, X. Pan, and T. Dong. 2010. “Optimization Model for Remanufacturing Logistics Network with Fuzzy Parameters.” In Proceedings of International Conference on Measuring Technology and Mechatronics Automation, China, 849–852.10.1109/ICMTMA.2010.696
- Zhang, Y., J. Wang, X. Wang, Y. Huang, and X. Wang. 2010. “Model and Algorithm of Returned Logistics Network Planning for Renewable Resources.” In Proceedings of International Conference on Logistics Systems and Intelligent Management, China, 977–980.
- Zhang, Y., J. Wang, X. Wang, Y. Huang, and X. Wang. 2010b. “Model and Algorithm of Returned Logistics Network Planning for Renewable Resources.” International Conference on Logistics Systems and Intelligent Management: 977–980.
- Zhang, Y., L. Xuhong, M. Haijun, and M. Chenglin. 2007. “Designing a Hazardous Waste Reverse Logistics Network for Third Party Logistics Supplier Under the Fuzzy Environment.” In Proceedings of IEEE International Conference on Service Operations and Logistics and Informatics, China, 493–498.
- Zhou, G., Z. Cao, J. Cao, and Z. Meng. 2005. “A Genetic Algorithm Approach on Reverse Logistics Optimization for Product Return Distribution Network.” In Lecture Notes in Computer Science including subseries Lecture Notes in Artificial Intelligence and Lecture Notes in Bioinformatics, 267–272.
- Zhou, G., Z. Cao, Z. Meng, and Q. Xu. 2008. “A GA-Based Approach on A Repair Logistics Network Design with M/M/S Model.” In Proceedings of International Conference on Computational Intelligence and Security, China, 145–149.
- Zhou, X., and M. Zhang. 2009. “Research on Reverse Logistics Network Design Methods of Household Appliance.” In Proceedings of 16th International Conference on Industrial Engineering and Engineering Management, China, 1579–1582.
- Zhou, X., X. Ma, and M. Zhang. 2010. “Research on Network Design Methods of Automobile Manufacturing Enterprises Reverse Logistics.” In Proceedings of International Conference on Advanced Management Science, China, 14–17.
- Zhou, Y., and S. Wang. 2008. “Generic Model of Reverse Logistics Network Design.” Journal of Transportation Systems Engineering and Information Technology 8: 71–78.10.1016/S1570-6672(08)60025-2
- Zhou, X., and Y. Zhou. 2015. “Designing a Multi-Echelon Reverse Logistics Operation and Network: A Case Study of Office Paper in Beijing.” Resources, Conservation and Recycling 100: 58–69.10.1016/j.resconrec.2015.04.009
- Zhou, G., Z. Cao, J. Cao, and Z. Meng. 2005. “A Genetic Algorithm Approach on Reverse Logistics Optimization for Product Return Distribution Network.” Lecture Notes in Computer Science (including subseries Lecture Notes in Artificial Intelligence and Lecture Notes in Bioinformatics): 267–272.
- Zhou, G., Z. Cao, Z. Meng, and Q. Xu. 2008. “A GA-Based Approach on a Repair Logistics Network Design with m/m/s Model.” International Conference on Computational Intelligence and Security: 145–149.
- Zhou, X., and M. Zhang. 2009. “Research on Reverse Logistics Network Design Methods of Household Appliance.” 16th International Conference on Industrial Engineering and Engineering Management, 1579–1582.
- Zhou, X., and Y. Zhou. 2015. “Designing a Multi-Echelon Reverse Logistics Operation and Network: A Case Study of Office Paper in Beijing.” Resources, Conservation and Recycling 100: 58–69.10.1016/j.resconrec.2015.04.009
- Zohal, M., and H. Soleimani. 2016. “Developing an Ant Colony Approach for Green Closed-Loop Supply Chain Network Design: A Case Study in Gold Industry.” Journal of Cleaner Production 133: 314–337.10.1016/j.jclepro.2016.05.091
Appendix 1.
Triple bottom line graph for grouping RLND studies according to their goals
Appendix 2.
Indices and parameters of model 1
The following notations are used in the formulation of the model.
Sets:
k ∈ K: | = | set of collection point (CP) locations |
l ∈ L: | = | set of potential collection centre (CC) locations |
i ∈ İ: | = | set of existing collection centre (ECC) locations |
m ∈ M: | = | set of potential recycling centre (RC) locations |
j ∈ J: | = | set of existing recycling centre (ERC) locations |
d ∈ D: | = | set of disposal centre (DC) locations |
s ∈ S: | = | set of material supplier (MS) locations |
r ∈ R: | = | set of refinery centre (RC) locations |
p ∈ P: | = | set of product type |
h ∈ H: | = | set of material type |
t ∈ T: | = | set of transportation mode |
Model parameters:
Costs:
Fixed costs (in monetary unit):
= | annualised fixed costs for opening l | |
= | annualised fixed costs for closing i | |
= | annualised fixed costs for opening m | |
= | annualised fixed costs for closing j | |
= | annual information cost at l | |
= | annual information cost at i | |
= | annual information cost at m | |
= | annual information cost at j | |
= | annual application cost at l | |
= | annual application cost at i | |
= | annual application cost at m | |
= | annual application cost at j |
Transportation costs (in monetary unit/ton-km):
= | unit variable cost serving p from k to l by transportation mode t | |
= | unit variable cost serving p from k to i by transportation mode t | |
= | unit variable cost serving p from l to d by transportation mode t | |
= | unit variable cost serving p from i to d by transportation mode t | |
= | unit variable cost serving p from l to m by transportation mode t | |
= | unit variable cost serving p from l to j by transportation mode t | |
= | unit variable cost serving p from i to m by transportation mode t | |
= | unit variable cost serving p from i to j by transportation mode t | |
= | unit disposal cost for p served from m to d by transportation mode t | |
= | unit disposal cost for p served from j to d by transportation mode t | |
= | unit variable cost serving h from m to s by transportation mode t | |
= | unit variable cost serving h from j to s by transportation mode t | |
= | unit disposal cost for h served from m to r unit disposal cost for h served from j to r by transportation mode t | |
= | unit disposal cost for h served from j to r unit disposal cost for h served from j to r by transportation mode t |
Collecting and processing costs (in monetary unit/ton):
= | unit collecting cost of p from k to l by transportation mode t | |
= | unit processing cost of p at l | |
= | unit processing cost of p at i | |
= | unit processing cost of p at m | |
= | unit processing cost of p at j |
Disposing and refinery costs (in monetary unit/ton):
= | unit disposing cost for p served from l or i to d | |
= | unit disposing cost for p served from m j to d | |
= | unit refinery cost for p served from m or j to r |
Penalty costs (in monetary unit/ton):
= | opportunity costs caused from not collecting p at l | |
= | opportunity costs caused from not collecting p at i |
Carbon dioxide emission amount (ton):
= | carbon dioxide emission amount caused from opening l | |
= | carbon dioxide emission amount caused from closing i | |
= | carbon dioxide emission amount caused from opening m | |
= | carbon dioxide emission amount caused from closing j | |
= | carbon dioxide emission amount caused serving p from k to l by transportation mode t | |
= | carbon dioxide emission amount caused serving p from k to i by transportation mode t | |
= | carbon dioxide emission amount caused serving p from l to d by transportation mode t | |
= | carbon dioxide emission amount caused serving p from i to d by transportation mode t | |
= | carbon dioxide emission amount caused serving p from l to m by transportation mode t | |
= | carbon dioxide emission amount caused serving p from l to j by transportation mode t | |
= | carbon dioxide emission amount caused serving p from i to m by transportation mode t | |
= | carbon dioxide emission amount caused serving p from i to j by transportation mode t | |
= | carbon dioxide emission amount caused serving p from m to d by transportation mode t | |
= | carbon dioxide emission amount caused serving p from j to d by transportation mode t | |
= | carbon dioxide emission amount caused serving h from m to s by transportation mode t | |
= | carbon dioxide emission amount caused serving h from j to s by transportation mode t | |
= | carbon dioxide emission amount caused serving p from m to r by transportation mode t | |
= | carbon dioxide emission amount caused serving p from j to r by transportation mode t |
Distances (km):
= | distance k to l by transportation mode t | |
= | distance k to i by transportation mode t | |
= | distance l to d by transportation mode t | |
= | distance i to d by transportation mode t | |
= | distance l to m by transportation mode t | |
= | distance l to j by transportation mode t | |
= | distance i to m by transportation mode t | |
= | distance i to j by transportation mode t | |
= | distance m to d by transportation mode t | |
= | distance j to d by transportation mode t | |
= | distance m to s by transportation mode t | |
= | distance j to s by transportation mode t |
Capacities (in ton):
= | capacity of potential collection centre l for p | |
= | capacity of existing collection centre i for p | |
= | capacity of potential recycling centre m for p | |
= | capacity of existing recycling centre j for p |
Allocation rates:
= | average rate of p sent from l to d | |
= | average rate of p sent from l to m and j | |
= | average rate of h sent from m to s | |
= | average rate of p sent from m to r | |
= | average rate of p sent from m to d | |
= | average rate of p sent from i to d | |
= | average rate of p sent from i to m and j | |
= | average rate of h sent from j to s | |
= | average rate of p sent from j to r | |
= | average rate of p sent from j to d |
Annual return amount (in ton):
= | annual returns of product p from k |
Minimum required product amount (in ton):
= | min. product for moving into profit at l | |
= | min. product for moving into profit at m | |
= | min. product for moving into profit at i | |
= | min. product for moving into profit at j |
Material rate:
= | rate of material h for each product |
Social benefit amounts:
= | increase in non-qualified labour force when l is opened | |
= | increase in qualified labour force when l is opened | |
= | increase in non-qualified labour force when m is opened | |
= | increase in qualified labour force when m is opened | |
= | decrease in non-qualified labour force when i is closed | |
= | decrease in qualified labour force when i is closed | |
= | decrease in non-qualified labour force when j is closed | |
= | decrease in qualified labour force when j is closed |
Decision variables
Decisions related to centre location:
= | Indicator opening l (1 if opened; 0 otherwise) | |
= | Indicator closing i (1 if closed; 0 otherwise) | |
= | Indicator opening m (1 if opened; 0 otherwise) | |
= | Indicator closing j (1 if closed; 0 otherwise) |
Logical decisions:
= | Indicator connecting k to l (1 if connected; 0 otherwise) | |
= | Indicator of connecting k to i (1 if connected; 0 otherwise) |
Decisions related to production units:
= | flow p from k to l by transportation mode t | |
= | flow p from k to i by transportation mode t | |
= | flow p from l to m by transportation mode t | |
= | flow p from i to m by transportation mode t | |
= | flow p from l to j by transportation mode t | |
= | flow p from i to j by transportation mode t | |
= | flow p from l to d by transportation mode t | |
= | flow p from i to d by transportation mode t | |
= | flow h from m to s by transportation mode t | |
= | flow h from j to s by transportation mode t | |
= | flow p from m to r by transportation mode t | |
= | flow p from j to r by transportation mode t | |
= | flow p from m to d by transportation mode t | |
= | flow p from j to d by transportation mode t |
Model formulation
Objective function
1. Economic objective (
Fixed costs: are the costs to open potential centres in different locations or close the existing ones.(7)
Collection costs: are the costs to collect the returned products, such as take-back payments and costs of container placement.(8)
Disposal and refinery costs: are the costs to dispose unrecoverable products and make some special products recover in licensed firms.(9)
Processing costs: are the costs to process operations to recover the recoverable products.(10)
Transportation costs: are the costs to distribute or transport the products and materials.(11)
Information costs: are the costs to increase the consciousness of the actors on RL activities in the chain. The consciousness raising studies are organised by municipalities, OEMs or logistics service provides.(12)
Application costs: are the costs to improve the technical adjustment of the RL systems into the existing systems.(13)
Penalty costs: are the costs not able to make the centres process in full capacity. Excess capacity addresses that capacity utilisation is low and that makes the firm put up with the cost of missing the opportunity for gaining more.(14)
2. Environmental objective (f2(x)):
Carbon dioxide emission amount caused from transportation:(15)
Carbon dioxide emission amount caused from opening/closing centres:(16)
3. Social objective (f3(x)):
Increase in labour force when a centre is opened:(17)
Decrease in labour force when a centre is closed:(18)
Constraints:
Balance constraints:(19)
(20)
(21)
(22)
(23)
(24)
(25)
(26)
(27)
(28)
(29)
(30)
Capacity constraints:(31)
(32)
(33)
(34)
Logic constraints:(35)
(36)
(37)
(38)
(39)
(40)
(41)
(42)
(43)
(44)
(45)
(46)
(47)
Other constraints: