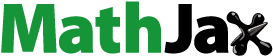
ABSTRACT
Suppliers as upstream partners play a crucial role to establish sustainability in the supply chain. For precise evaluation of the supplier’s performance on sustainability criteria researchers have mainly focused on Multi-Criteria Decision Making (MCDM) and Fuzzy Inference System (FIS) approaches. The objective of the paper is to evaluate the supplier’s sustainability performance through FIS and fuzzy MCDM approaches. Further, the results are compared and utilised to develop the Sustainable Supplier Selection (SSS) framework for the iron and steel industry of India. To calculate Suppliers Performance Index (SPI), three distinct FIS engines have been developed for each sustainability dimensions and fuzzy AHP and TOPSIS techniques are used for the MCDM environment. The results provide a deep inside of the sustainability performance of each supplier. It elicits from the results of two approaches, that ranks are interchanged among the top two suppliers.
1. Introduction
Ever-increasing judicial pressure and increasing stakeholder’s awareness about the environment and social rights have forced industries to take up initiatives to achieve sustainability objectives in their supply chain and manufacturing processes (Ahmadi et al. Citation2017; Jafarian Citation2013; Luthra, Garg, and Haleem Citation2015; Luthra et al. Citation2017). On the other hand, industries are also aware of the various benefits of sustainability attainment and have initiated the incorporation of social and environmental aspects along with economic traits (Tavana, Yazdani, and Di Caprio Citation2016; Azadnia et al. Citation2013). However, for effective integration of sustainability dimensions into supply chains, the industry needs to acquire the support and cooperation of all upstream and downstream supply chain partners (Amindoust et al. Citation2012; Roy et al. Citation2019). Suppliers are upstream partners and can contribute significantly to the attainment of the sustainability objectives in the industry (Büyüközkan and Gizem Citation2011; Luthra et al. Citation2017; Ghadimi, Dargi, and Heavey Citation2017; KhanMohammadi et al. Citation2018). Hence the sustainable supplier selection is a strategic decision for the industry (Kumar and Rahman Citation2015; Tavana, Yazdani, and Di Caprio Citation2016; Orji and Wei Citation2015; Ghadimi et al. Citation2019).
The conventional Supplier Selection (SS) process was based on economic criteria and supplier’s performance on social and environmental criteria was not considered. However, Sustainable Supplier Selection (SSS) requires supplier’s performance evaluation on economic, social and environmental dimensions i.e. Triple Bottom Line (TBL) guidelines (Shalke et al. Citation2018; Chaharsooghi and Ashrafi Citation2014; Zimmer, Fröhling, and Schultmann Citation2016; Vinodh, Mulanjur, and Thiagarajan Citation2013; Yazdani, Chatterjee, and Torkayesh Citation2020). But the consideration of economic, environmental and social dimensions in a single decision frame made decision-makers handle a large number of criteria. The handling of such a large number of sustainability criteria is a major challenge and the possible solution is criteria clustering (Jain and Singh Citation2019). Therefore for accomplishing the SSS process, DM’s require to develop clusters of evaluation criteria in three sustainability dimensions.
Saxena et al. (Citation2017) defined clustering as unsupervised learning where the objects are grouped on the basis of some similarity inherent among them. Criteria analysis encompasses various algorithms and methods for grouping objects of a similar kind into respective categories such that the degree of association between two objects in the same group is maximum and minimal otherwise. Authors have developed various criteria clustering methods such as K- means algorithm, Artificial Neural Network, self-organising Maps, etc. (Terlunen, Barreto, and Hellingrath Citation2015).
Jain and Singh (Citation2019) have considered sixty-six sustainability criteria significant for SSS in a large scale industry. Authors have applied the fuzzy Kano model for clustering the sustainability criteria into four main clusters, Must be, One dimensional, Attractive, and Indifferent. Further, the authors have applied the Type IV Kano model for three subclassifications of Must be and Attractive cluster criteria.
Sustainable supplier assessment framework in the industry requires human reasoning based input. These inputs have inherited imprecision and vagueness (Azadnia et al. Citation2013; Singh, Olugu, and Fallahpour Citation2014). Hence to cope with the vagueness, the proposed decision framework focuses on the application of FIS for SSS for a large scale industry incorporating Attractive criteria. Application of FIS provides more precise and accurate results as it incorporates the knowledge of industry experts in establishing the fuzzy rules set which enables precise evaluation of suppliers. Hence DMs need to take optimum decisions concerning the desired accuracy and the number of rules. Some researchers have used FIS for addressing the issue of SSS (Amindoust and Saghafinia Citation2016; Ghadimi, Dargi, and Heavey Citation2017; Pourjavad and Shahin Citation2018). However, the authors have not performed the criteria clustering in their models.
In literature, various researchers and academicians have also proposed Multi-Criteria Decision Making (MCDM) based techniques for SSS as MCDM techniques are well suited for structuring complex problems (Mardani et al. Citation2015; Igarashi, De Boer, and Fet Citation2013; Ho, Xiaowei, and Dey Citation2010; Chai, Liu, and Ngai Citation2013; Govindan et al. Citation2015; Agarwal et al. Citation2011). However, to perform the evaluation of potential suppliers, response from DM’s in linguistic terms are required. But linguistic response lacks the subjectivity as decision-makers express their qualitative perceptions in the quantitative form (Amindoust et al. Citation2012; Liu et al. Citation2019) To address this issue authors have integrated fuzzy logic concepts with MCDM techniques for addressing the SSS process (Ho, Xiaowei, and Dey Citation2010; Mardani et al. Citation2015). However, fuzzy logic integrated MCDM techniques fall short in the handling of a large number of sustainability criteria. The number of mathematical calculations increases in fuzzy MCDM as the number of alternatives are increased. Hence in the proposed decision framework, the fuzzy MCDM technique has been applied considering criteria clustering. However, fuzzy logic-based MCDM techniques fall short in the handling of a large number of sustainability criteria and complex & larger mathematical calculations are involved when the number of alternatives is more. Therefore to overcome these restrictions and to capture the inborn vagueness in DM’s responses, in this work a Fuzzy Inference System (FIS) has been applied. Also in the case of a sustainable supplier selection problem the input parameters need to be critically examined which can be efficiently handled by the FIS system. Further FIS system possess superiority over other fuzzy MCDM techniques (Pourjavad and Shahin Citation2018; Amindoust et al. Citation2012) because FIS make use of Rule Base Architecture (RBA) which is more capable of capturing the input parameter through membership function and rule base of FIS.
It is elicited from the literature review that fuzzy AHP and fuzzy TOPSIS are the preferred MCDM techniques (Zimmer, Fröhling, and Schultmann Citation2016). Hence, in this work, fuzzy analytic hierarchy process (FAHP) has been applied for weight assignment and fuzzy Technique for Order of Preference by Similarity to Ideal Solution (FTOPSIS) method has been used for supplier assessment and the establishment of Supplier Performance Index (SPI).
The proposed decision framework employs the same decision maker’s team to evaluate the potential suppliers on the same criteria and sub-criteria through two different approaches i.e FIS and FMCDM. SPI obtained from both the approaches are compared and a decision framework has been developed for performing the final selection of a sustainable supplier.
Our research thus contributes to the literature on sustainable supplier selection in the following ways. First, in this work, three distinct FIS engines in each sustainability dimension have been developed for which Attractive criteria and their quantified sub-criteria acts as an input. Second, the proposed methodology considers the values of SPI from FIS and fuzzy MCDM approaches on a single platform and provides robust results to decision-makers to able them to make efficient decisions. Third, in the proposed methodology, the least number of FIS rules need to be framed by DM’s in each FIS engine.
The novelty of the proposed work is its ability to incorporate the crux of MCDM as well as FIS approaches for the final selection of the suppliers. Further, the proposed work incorporates the modified structure of the FIS system which facilitates the DMs to frame the least number of rules for each FIS engine in three sustainability dimensions. The results of the present work provide a better understanding of the supplier’s performance in each sustainability dimension. Further, the results of the two approaches enable the DMs to have a comparative validation through the supplier’s evaluation. It elicits from the results that the supplier’s excelling in one dimension is not performing well in other sustainability dimensions. Hence the overall results convey the overall sustainability performance which helps the DMs to make the final selection.
Another novelty of the proposed work is, in present times when all suppliers are equipped with modern production technology and fulfill the basic criteria of selection the proposed work evaluates supplier’s sustainability on Attractive criteria for final selection. The proposed methodology has been applied to the Indian iron and steel industry as a case company.
The remainder of the paper is organised as follows. In section 2, a literature review on SSS criteria and SSS frameworks with integrated fuzzy set theory along with the research gaps is presented. Section 3 presents the proposed methodology. Section 4 presents the application of the proposed model in the case company and also covers the results of the application of the model. Discussions on the results of the work have been covered in section 5. The managerial implications of the work are discussed in section 6. Finally, a few remarks along with directions for future work have been concluded in section 7.
2. Literature review
In 1966, Dickson carried out the Pioneer research in the field of supplier selection criteria and reported quality, delivery as the criteria of extreme importance and price and technical capability as considerable importance criteria for the supplier selection process. Since Dickson’s work, many authors have reported and advocated the incorporation of new supplier selection criteria due to the emergence of new technologies and also due to the globalisation of markets. Further, due to growing awareness among customers and pressure from government agencies and stakeholders, the incorporation of environmental and social criteria has been done in the supplier selection process (Hussain and Al-Aomar Citation2018). It is elicited from the Literature review that quantum of work has been done in the field of supplier selection by various researchers. Academicians and researchers have proposed various decision frameworks incorporating different methodologies. It is also elicited from studies on traditional and sustainable supplier selection process, that there are two main issues in supplier selection process, at first establishment of appropriate sustainable criteria for selection and secondly development of a decision framework for evaluation of supplier performance based on criteria and awarding of ranks to suppliers (Govindan, Khodaverdi, and Jafarian Citation2013). Hence in this section, a brief literature review has been presented on two domains which are critical for the success of SSS.
2.1. Sustainable supplier selection criteria
The selection of efficient suppliers has been a major challenge for the procurement department of industries (Weber Citation1991) and researchers have addressed the problem of supplier selection comprehensively. Authors have proposed different supplier selection methodologies/frameworks as a possible solution to this problem (Ware, Singh, and Banwet Citation2012; Ho, Xiaowei, and Dey Citation2010; Khurrum and Bhutta Citation2003).
Traditionally the proposed methodologies incorporated only the economic dimension and the majority of the proposed methodology is based on the economic criteria reported by Dickson (Citation1966). Supplier selection was purely based on the supplier’s performance on the economic criteria (Kar and Pani Citation2014; Wilson Citation1994; Cheraghalipour and Farsad Citation2018). However, with the increasing environmental awareness among the customers, environmental criteria were incorporated along with the economic criteria in the supplier selection process (Humphreys, Wong, and Chan Citation2003; Govindan et al. Citation2015). Hence due to the inclusion of environmental criteria (Green criteria), SCM was more commonly termed as Green supply chain management (GSCM) (Kuo, Wang, and Tien Citation2010; Bai and Sarkis Citation2010; Noci Citation1997; Igarashi, De Boer, and Fet Citation2013; Banaeian et al. Citation2016). In the year 1987 Brundtland commission in its report emphasised the conservation of environment along with development and hence insisted on sustainable development. The report defined sustainable development as ‘development that meets the needs of the present without compromising the ability of future generations to meet their own needs.’ (Bruntland Citation1987). Thus with the widespread significance of social awareness among various stakeholders, economic, environmental and social criteria were considered simultaneously for supplier selection. Incorporation of all three domains leads to the development of Sustainable Supply Chain Management (SSCM). Among the various objectives of SSCM is the objective of sustainable supplier selection (Rao, Goh, and Zheng Citation2017). Academicians have addressed the topic and have proposed various MCDM tools based methodologies for supporting and structuring the decision framework (Zimmer, Fröhling, and Schultmann Citation2016; Nielsen et al. Citation2014). However, due to different social trends and traits among various cultures in the world, consideration of social characteristics of sustainability in supplier selection is more challenging as a comparison to economic and environmental aspects (Ghadimi, Dargi, and Heavey Citation2017; Winter and Lasch Citation2016).
In today’s global market, the industry needs to incorporate sustainability initiatives in its manufacturing process and its supply chains so as to withstand the competition and maintain its current position among the competitors (Cheraghalipour, Paydar, and Keshteli Citation2018). This makes the establishment of a sustainable supply chain as the prime objective of decision-makers of industries (Hassini, Surti, and Searcy Citation2012). To address this objective, many methodologies have been proposed by various authors incorporating sustainability criteria for supplier selection in different domains (Luthra et al. Citation2017; Shaw et al. Citation2012; Seuring and Muller Citation2008). However, form the literature it elicits that the authors have not considered the sustainability criteria for selecting the suppliers from the perspective of perceived satisfaction by the industry (). To attain the highest level of satisfaction from the SSS process sustainability criteria need to be clustered on the basis of criteria priority for the selection process.
Table 1. Sustainable supplier selection methodologies with integrated fuzzy set theory
Kano model is one such criteria clustering method which has been widely adopted by the authors in different research fields such as healthcare, future vehicle driving, online reviews, budget airlines, environmental quality, strategic thinking, student satisfaction, retail service industry, automated vehicles, patient satisfaction, etc. (Min-Yuan, Chen, and Chang Citation2019; Materla, Cudney, and Antony Citation2019; Jian-Wu et al. Citation2019; Wong and Ho Citation2019; Dace, Stibe, and Timma Citation2019; Watson Citation2019; Dinçer, Yüksel, and Pınarbaşı Citation2020; Stetter Citation2020; Lucadamo, Camminatiello, and D’Ambra Citation2020). Kano model has the unique feature of establishing a relationship between DM’s expectations and their satisfaction level. Criteria clustering through the Kano model facilitates DMs to clusters the sustainability criteria on the basis of perceived satisfaction into five main categories.
Attractive requirements (A): this cluster includes the requirements that are not expected by the customer in a product, therefore satisfaction level increases on fulfilment, and however nonfulfillment does not bring any dissatisfaction to the customer.
Must-be requirements (M): The requirements that are vital and which customer needs to have in the product. The dissatisfaction level significantly increases the Non-fulfilment of these requirements.
One-dimensional requirements (O): Customer satisfaction increases if these requirements are met and customer dissatisfaction increases when these requirements are not met. These requirements are performance requirements and are expected by the customer in the product or service offered.
In different requirements (I): Customer satisfaction or dissatisfaction is not affected by these requirements.
Reverse requirements (R): These requirements are not desired by the customer and the absence of these attributes increases the customer’s satisfaction level.
2.2. Sustainable supplier selection techniques incorporating fuzzy logic
Sustainable supplier selection process’s success depends much on the evaluation of supplier performance with respect to sustainability criteria in linguistic terms (Azadnia et al. Citation2013; Jain and Singh Citation2017). Decision-makers respond to supplier evaluation using linguistic terms that are simple and tangible. However, due to the subjective evaluation of qualitative and quantitative criteria, the response of DM’s are encompassing vagueness and uncertainty (Yu et al. Citation2019). To capture this ambiguity in the response to DM’s, authors have incorporated fuzzy set theory integrated with other decision tools (Jafarian Citation2013; Amindoust et al. Citation2012; Orji and Wei Citation2015).
Fuzzy set theory was developed by Zadeh (Citation1965) for capturing uncertainty and imprecision inherited in human judgement using linguistic terms and degree of membership. Further decision framework in a sustainable environment demands to mesh quantitative and qualitative criteria that traditional approaches fail to achieve (Zimmer, Fröhling, and Schultmann Citation2016). Thus authors have incorporated fuzzy set theory in their decision frameworks as a natural choice over other approaches. The fuzzy set theory has the capability of dealing with the concepts which are hard to quantify and it also provides crisp outputs for problems having subjectivity (Ghadimi, Dargi, and Heavey Citation2017). Owing to these benefits, authors have integrated fuzzy set theory with decision tools such as Analytic Hierarchy Process (AHP), Data Envelopment Analysis (DEA), Weighted Aggregated Sum Product Assessment (WASPAS), Technique for Order of Preference by Similarity to Ideal Solution (TOPSIS), etc. (Zimmer, Fröhling, and Schultmann Citation2016; Ho, Xiaowei, and Dey Citation2010; Govindan et al. Citation2015).
2.3. Research gaps and research objectives formulation
An effective SSS process depends on the identification and establishment of appropriate sustainability criteria (Rashidi and Cullinane Citation2019; Memari et al. Citation2019; Giannakis et al. Citation2020; Naveen Jain and Singh Citation2020). From the literature review, it is elicited that economic criteria have been mainly focused by authors for establishing the SSS process. Further, few authors have considered environmental criteria along with economic criteria (Bai and Sarkis Citation2010; Büyüközkan and Gizem Citation2011; Shen et al. Citation2013). however, the TripleBottom Line(TBL) approach os sustainability emphasis the consideration of criteria in three i.e social, environmental and economic domains. Hence the first objective of the present work is framed as
Objective 1: Selection of appropriate sustainability criteria cluster in economic, environmental and social dimensions for SSS.
Today, almost all the suppliers have state of the art technology and equipment and hence can provide the industry with quality products at most economical cost. In such a scenario where all suppliers are at par with each other, the industry needs to have a set of criteria that helps to evaluate and distinguish the most potential sustainable suppliers and also to achieve the highest level of satisfaction from the SSS process. Thus the second objective of the work is
Objective 2: Identification of Attractive criteria for better and distinguished supplier evaluation in the SSS process.
The SSS process involves the evaluation of suppliers against a large number of sustainability criteria. Handling such a large number of sustainability criteria is a major challenge for the DMs. Multi-Criteria Decision Making (MCDM) techniques facilitate DMs in choosing the most potential supplier considering several qualitative and quantitative criteria that are often conflicting in nature. However, the MCDM techniques suffer from the drawback that they depend on human judgement pairwise comparison for selection which is biased. Hence, the supplier evaluation needs to be done through the FIS system also, considering the same set of criteria and final selection to be made considering the results of both the approaches.
Objective 3: Development of SSS decision framework with architecture based on MCDM and FIS respectively and considering results of both approaches for final selection.
SSS process encompasses supplier evaluation through human judgement in terms of linguistic responses. As these linguistic terms inherit ambiguity and uncertainty there is a need for capturing the vagueness in the responses. Hence the last objective of the work is framed as
Objective 4: Integrating fuzzy set theory in both MCDM and FIS approaches for capturing the vagueness of DMs in the SSS decision framework.”
3. Proposed methodology
The proposed methodology has been developed for sustainable supplier selection in the iron and steel industry of India. The methodology comprises of six steps as
Step 1: Formation of Decision-makers’ team:
In the initial stage of the methodology, a team of decision-makers is to be formed. The decision-makers should have adequate experience in the industry and should be conversant with the supplier selection process of their organisation. The primary responsibility of the team comprises of formation of a pool potential suppliers from which the final selection can be made.
Step 2: Review of Literature:
A comprehensive literature survey is to be carried out for identification of the industry-specific Sustainable Supplier Selection (SSS) criteria.
Step 3: Sustainability criteria clustering
An efficient SSS framework necessitates the evaluation of suppliers to be performed against a larger number of criteria. However, consideration and handling of a larger number of sustainability criteria present problems for the decision-makers. Hence to facilitate the decision-makers there is a need for clustering of sustainability criteria into meaningful categories based on the priority of criteria form SSS perspective.
Kano model philosophy is a suitable tool for criteria clustering (Jain and Singh Citation2017). Further, the fuzzification of the Kano model helps in better capturing of the real perception of the DM’s. Application of Fuzzy Kano model, clusters sustainability criteria into four clusters (i) Must be, (ii) One dimensional, (iii) Attractive and (iv) Indifferent cluster. Further, subclassification of the sustainability criteria under the Attractive cluster can be done by using the Type IV Kano model as proposed by Shahin et al. (Citation2013) and applied by Jain and Singh (Citation2019) for clustering of sustainability criteria. Application of Type IV Kano model further subclassify the Attractive cluster into three sub-clusters: Less attractive (A1), Attractive (A2) and Highly attractive (A3).
Step 4: Identification of sub-criteria of the selected cluster
Further, after the subclassification of attractive sustainability criteria, each criterion is individually addressed by DM’s and relevant sub-criteria of attractive sustainability criteria in three dimensions are established for further analysis.
Step 5: Sustainability Performance Index (SPI) calculation through FIS
In this step sub-criteria for three sustainability dimensions are quantified and based on quantified values appropriate range for sub-criteria are established. Further, membership functions are developed which acts as an input for the Fuzzy Inference System (FIS). In this work, the FIS model has been prepared by applying the Mamdani’s compositional rule of inference (Mamdani Citation1974). Choice of Mamdani FIS over Sugeno inference system is made based on the following points
It predicts the results reasonably and comprises of a simple structure along with intuitive and interpretable rule base nature (Jassbi et al. Citation2006).
In Mamdani FIS a crisp result is obtained by defuzzification of rules’ consequent whereas in Sugeno FIS crisp result is obtained using a weighted average of the rules’ consequent.
Mamdani FIS is more suitable for human inputs, has expressive power and interpretable rule consequents whereas Sugeno FIS is suitable for mathematical analysis and lacks interpretability.
In this work, three distinct FIS engine has been developed for each sustainability dimension for evaluation of the supplier’s performance on sustainability criteria. The proposed FIS model development consists of three major steps:
3.1. Membership function and operational operators
In this step, DMs consider every sub-criterion and based on their experience and current practices of industry establish the evaluation levels and further quantify these evaluation levels. This quantification provides robustness and reliability to the proposed approach and thus helps in attaining more precise results. However, the evaluation levels established by DMs are subjective and imprecise and hence they need to be converted into the grade of membership. In this FIS model, the membership function for the input variables is developed by considering a triangular form. A triangular form of fuzzy number can be represented as = (a, b, c) as shown in and defined as EquationEq. (1)
(1)
(1) .
In this FIS model, quantified levels of sub-criteria of each sustainability dimension act as input and three fuzzy sets have been applied on these inputs. The linguistic terms used by DMs for evaluating supplier’s performance, assigned to each fuzzy sets are ‘Worst’, ‘Average’ and ‘Best’ as tabulated in .
Table 2. Linguistic terms for supplier’s evaluation
The basic mathematical operations performed on fuzzy numbers are fuzzy addition, fuzzy subtraction, fuzzy division, and fuzzy multiplication. To understand the fuzzy mathematical operations lets us consider two fuzzy numbers as Z1 = (l1, m1, u1) where and Z2 = (l2, m2, u2), where
. The basic mathematical operations considering two fuzzy numbers Z1 and Z2 are represented as shown in .
Table 3. Fuzzy mathematical operations
3.2. Fuzzy rules (KM based) and modified architecture of FIS engine
In this step, the expert’s knowledge is utilised to frame the fuzzy rule sets for the development of the FIS model. The number of fuzzy rules in a FIS model is determined by using EquationEquation (2)(2)
(2) .
Where
N: represents the number of fuzzy rules
M: represents the number of membership functions and
: represents the number of input variables.
For understanding the utility of EquationEq (2)(2)
(2) in determining the number of rules let us consider an example with values of M as 3 and the value of
as 2. As per EquationEq. 2
(2)
(2) , the total number of rules to be framed for FIS are 9. However, if the value of
is increased to 3, and keeping the value of M same, then the number of rules to be framed increases to 27 and which further increases to 81 for
= 4. Hence it can be observed from the example that, for the same number of membership functions, the number of rules increases exponentially for a higher number of input variables. As such handling of lager number of rules in FIS is complex and presents a challenge for DMs. Therefore to handle such situations, in the proposed methodology the modified architecture of FIS has applied which facilitates the DMs to handle the least possible number of rules without affecting the accuracy of the results.
3.3. Defuzzification
The fuzzified results of each rule are given as input to the FIS and the output of FIS is used as an input for the defuzzification process for obtaining the results in real numbers. In literature, authors have proposed various types of defuzzification methods such as (i) Centre of Sums Method (COS), (ii) Centre of gravity (COG) or Centroid of Area Method (COA), (iii) Bisector of Area Method (BOA) and (iv) Weighted Average Method. In the proposed FIS, COA method has been used for the defuzzification of the fuzzified results and can be expressed mathematically as EquationEquation (3)(3)
(3)
The final output of the defuzzification process is the Performance Index in Economic Dimension (PIED), Performance Index in Social Dimension (PISD), and the Performance Index in Environmental Dimension (PIEND) of each supplier. Further, DM’s assign the weight to each sustainability dimension and based on these three weighted performance indexes the final Sustainability Performance Index (SPI) for each supplier is then calculated as
Where SPI is the Sustainability Performance Index of the supplier evaluated, is the importance weight of PIED,
is the importance weight of PISD and
is the importance weight of PIEND as decided by the DM’s. The proposed methodology facilitates the decision-makers to decide the weights according to the industry needs and the global scenario. Decision-makers have the liberty to choose different weights by considering any value ranging from 0 to 1. Value 1 represents the highest priority and value 0 signifies no priority to the sustainability dimension.
Step 6: Sustainability Performance Index (SPI) calculation through an integrated fuzzy MCDM method.
In this step, a pairwise comparison of sub-criteria is performed by using the fuzzy AHP technique for the establishment of fuzzy weights of sub-criteria. Further, utilising these fuzzy weights, performance assessment of the suppliers against each sub-criteria is performed by applying the fuzzy TOPSIS method. The performance of each supplier is assessed and Sustainability Performance Index (SPI) for each supplier is determined.
3.4. Fuzzy AHP
AHP is the most preferred MCDM technique for decision modelling (Zimmer, Fröhling, and Schultmann Citation2016). AHP is a structured decision technique for dealing with complex decision making. Following are the steps of FAHP technique
3.4.1. Establish the pair-wise comparison matrix
Based on the expert opinion the pairwise comparison of factors is being prepared. The experts are required to compare each factor in pairs in a matrix form with size n x n, where n is the number of criteria.
3.4.2. Check for the consistency
To control the consistency of subjective opinions of the decision-makers and to check the accuracy of the weight factors, the consistency factor is calculated as
Where the maximum eigenvalue of the matrix and n is the number of alternatives. If the CF is less than 0.1 then pairwise comparisons are acceptable.
The values of the pairwise comparison matrix are converted into triangular fuzzy numbers
For the construction of fuzzy judgement matrix comprising of n criteria through pairwise comparison using Triangular Fuzzy Numbers (TFN) as
Where is a fuzzy triangular number represented as
= (
and
. The membership function
for TFN is a continuous mapping of real number
to the closed interval [0, 1] and can be represented by Equationequation 1
(1)
(1) .
Calculate the fuzzy geometric mean value
In the fuzzy comparison matrix, is calculated by multiplying the fuzzy numbers in a row as per the fuzzy multiplication rule and the nth root is calculated for the establishment of the mean value.
3.4.3. Develop fuzzy weights of criteria
In this step fuzzy weights for every criterion is calculated by applying the equation
3.5. Fuzzy TOPSIS
TOPSIS (technique for order preference by similarity to an ideal solution) method has the fundamental principle of establishment of a positive ideal solution (PIS) and a negative ideal solution (NIS). Further, the geometric distance of each alternative form PIS and NIS is determined and the alternative with the shortest distance from PIS and farthest distance from NIS is selected.
3.5.1. Establish a decision matrix
In this step DM’s evaluate the supplier’s sustainability performance on sustainability criteria and mark their response using linguistic variables. DM’s mark their responses and corresponding fuzzy equivalent TFN are utilised for establishing the fuzzy decision matrix. As per EquationEquation (8)(8)
(8)
Where: represents the fuzzy value Performance of ith alternative for jth criteria.
3.5.2. Establish the combined fuzzy decision matrix
In this step, the fuzzy decision matrix of all the DM’s are combined to reach the final combined fuzzy decision matrix by
Where ,
,
3.5.3. Establish the normalised fuzzy decision matrix
The values of the combined decision matrix are normalised using the EquationEquations (10)(10)
(10) and (Equation11
(11)
(11) ). EquationEquation (10)
(10)
(10) is used when the criteria are beneficial criteria i.e. the higher values are preferred and EquationEquation (11)
(11)
(11) is utilised for normalisation of non-beneficial criteria for which the lesser values are preferred.
3.5.4. Establish the weighted normalised fuzzy decision matrix
In this step, the fuzzy weights of sub-criteria calculated using FAHP are multiplied with the normalised values using EquationEquation (12)(12)
(12) according to the fuzzy multiplication rule shown in .
where represents the weight of the criteria
3.5.5. Calculate FPIS and FNIS
The fuzzy positive ideal solution (FPIS) and fuzzy negative ideal solution (FNIS)
are calculated using EquationEquation (13)
(13)
(13) and EquationEquation (14)
(14)
(14) respectively.
3.5.6. Establish the distance from each alternative to FPIS and FNIS
In this step, the fuzzy distance of each alternative from FPIS and FNIS solutions are calculated using EquationEquation (15)(15)
(15) and EquationEquation (16)
(16)
(16) respectively
3.5.7. Determine the relative closeness to the ideal solution
In this step, the fuzzy closeness distances to the FPIS and FNIS are considered simultaneously for calculating the relative closeness as per Equation.
Step 7: Ranking of alternatives and selection:
In this step, SPI’s calculated through FIS and integrated fuzzy MCDM method are considered for awarding of final ranks to the potential suppliers. Appropriate weights as decided by DMs are given to FIS and MCDM approach and Cumulative SPI (CSPI) for each supplier is calculated. Based on the values of CSPI final ranks are awarded to all potential suppliers and the final selection of a sustainable supplier for awarding the contract can be made by the industry.
The flowchart of the proposed methodology has been shown in ).
4. Application of the proposed methodology in case company
India is a fast developing country and stands at the fourth position in the world in terms of GDP. The iron and steel sector of India has contributed significantly to achieving this strong GDP position. Development in the railway sector, infrastructure, airports, oil &gas and steel demand in other sectors have resulted in the steel industry as the backbone of national growth. In 2005 India overtook the US to become the third-largest producer of crude steel. In a major achievement in January 2019 India, crude steel production reached 9.2 million tonnes (MT) and overtook Japan to become the second-largest producer in the world as reported by the World Steel Association. The Indian government is focusing much on the steel industry as it contributes 2% of the net GDP of the country and aims to increase its global share to 7.7 % by 2021.
Indian iron and steel industry is manufacturing rails, plates, bloom, billets, wires, rods, wheel, and axels, etc., and cater to the need for national and many international customers. India has the vision to become the global leader in crude steel production by increasing its steel production but at the same time is aware of the significance and necessity of achieving the sustainability objectives. Increased production will result in the enhancement of various steel making activities such as excavation of minerals from mines, search for new areas for raw materials, more emission of greenhouse gases, and disposal of wastes in water and land resulting in large scale impact over the environment. Hence the industry needs to expand its sustainability actions and in this regard need its suppliers to take up initiatives for achieving sustainability. Therefore the proposed methodology is implemented as a case study for an Indian iron and steel industry of central India.
The details of the application of the six steps of the proposed research methodology are:
Step 1: Formation of Decision-makers’ team:
At the initial stage, a team of decision-makers consisting of eight persons is formed. The team members selected, represents the top and middle management of the industry. The team members are selected from the departments which are associated with the supplier selection process in the industry. Hence, personnel from materials management, store management, finance, and quality control are considered for team formation and they have adequate experience in the industry have a thorough knowledge of the supplier selection process of the industry. Team members were personally contacted and prior consent of management and team members was taken for participating in the work. A meeting was held with all the decision-makers and a brief description was given to them about the role and responsibilities. The details of all the decision-makers of the team are presented in . The primary responsibility of the team comprises of identifying the potential suppliers and forming a pool from which the final selection can be made for contract allocation. Apart from this, the team is responsible for the finalisation of sustainability criteria specific for the industry and also contribute by providing timely responses in the decision-making process.
Table 4. Details of Decision maker’s team
Step 2: Review of Literature:
In the proposed framework the sustainability criteria proposed by Jain and Singh (Citation2019) have been considered for developing the decision framework. In their work authors have performed a comprehensive literature survey for identification of sustainability criteria for SSS for a large scale industry. Authors have reported thirty-two criteria in the economic dimension and seventeen criteria in social and environmental dimensions respectively and have applied the fuzzy Kano model for criteria clustering.
Step 3: Sustainability criteria clustering:
Jain and Singh (Citation2019) have clustered sustainability criteria into four main clusters: Must be, One dimensional, Indifferent and Attractive clusters. Further, authors have applied type IV Kano model for sub classifying sustainability criteria in the Attractive cluster into less attractive (A1), attractive (A2) and highly attractive (A3) criteria. Less Attractive (A1) subcluster have been not considered for further analysis as being in ‘Less’ subclassification, it has low significance on SSS as compared to other two subclusters of Must be and Attractive clusters.
In this work sustainability criteria of Attractive and Highly Attractive clusters have been considered for further analysis (). In the next section, the sub-criteria classification has been carried out for the criteria under the Attractive cluster.
Table 5. Subclassified Attractive cluster sustainability criteria
Step 4: Identification of sub-criteria of the selected cluster
The characteristic of Attractive criteria has been captured by establishing their sub-criteria based on current industrial practices, knowledge of DM’s, manufacturers, standards laid by national & international agencies. Further subclassification of these attractive sustainability criteria provides two-fold advantages to DM’s. Firstly the DM’s have deep insight knowledge of the criteria significance in SSS, prior to the establishment of sub-criteria. Secondly, DMs have to establish sub-criteria for lesser sustainability criteria and hence results are obtained in lesser time. For establishing the sub-criteria, a brainstorming session with decision-makers have been conducted and the Attractive sustainability criteria were presented to the DM’s with the objective of identification of sub-criteria and establishment of their evaluation levels. The team of decision-makers identified the sub-criteria based on the current supplier evaluation practices in the iron and steel industry.
For example, an Attractive cluster in economic dimension contains Warranties and claim policies (CA1) which Blischke (Citation1995) defined as ‘A Warranty is a seller’s assurance to a buyer that a product or service is or shall be as represented. It may be considered to be a contractual agreement between the buyer and seller (or manufacturer) which is entered into a sale of the product or service.’ To capture the different dimensions of Warranties and claim policies criteria DM’s have established two sub-criteria and their evaluation levels as:
Supplier recovery percentage (CA11): This refers to the Supplier recovery/total warranty cost:-Supplier recovery claims are claims made by the manufacturer to the supplier for reimbursement for the warranty-related costs that are associated with a warranty claim or work order when the cause of the defect was a supplied part. As companies source, most of the components from suppliers, percentages of the warranty recovered from suppliers is a very important part of managing warranty costs and supplier quality. Supplier warranty and recovery amounts can help measure the supplier performance and help with discussions on parts pricing and warranty terms & conditions with suppliers.
Claim frequency (CA12): It refers to the Number of Claims/Number of units. The industry can calculate the claim frequency by a number of claims or failures per thousand or million parts. A higher value of claim frequency is a matter of concern for the industry and should discuss it with the supplier.
Similarly, the Amount of past business (CA2) is another sustainability criteria in the economic dimension which refers to the amount of past business that has been done with each vendor (Weber, Current, and Benton Citation1991). However, DM’s agreed upon evaluating the performance of the supplier by considering three sub-criteria.
The growth of net worth, the average of the last 3 years (CA21): it is the increase in the value of the supplier’s net worth. It is calculated by taking the arithmetic mean of a series of net worth for a period of 3 consecutive years.
Profitability (before tax) (CA22): Profit before tax (PBT) is a profitability measure that looks at a company’s profits before the company has to pay income tax. PBT is calculated by deducting all expenses from revenue which includes interest expenses and operating expenses except for income tax.
The average amount of business in the last 3 years (CA23): It is defined as the average of the total amount of business that the industry has done with the supplier over the time span of the last three years. The higher value indicates much higher business transactions between the two parties and is considered as beneficial from supplier and industry perspective.
In the same manner, every criterion has been discussed by DM’s team and sub-criteria has been established for sustainability criteria in three dimensions (.). However, as sub-criteria may have a quantitative or qualitative nature hence it is difficult to fix a predefined range for the input variable. Therefore, depending on the nature of sub-criteria a range in the form of a minimum and maximum values are set for each evaluation level for each sub-criterion in the next step.
Table 6. Criteria, sub-criteria and quantification levels in three sustainability dimensions
Step 5: Sustainability Performance Index (SPI) calculation through FIS
In this work three discrete FIS, each for economic, environmental and social dimensions has been developed for evaluating the supplier’s performance in each sustainability dimension. For each FIS, quantified evaluation levels of the sub-criteria act as the inputs for the FIS. Further, in FIS for economic and environmental dimension Attractive and Highly attractive sub-criteria have been handled separately for an in-depth evaluation of suppliers (). The outputs of the three FIS are the performance index of the supplier in three sustainability dimensions.
Membership function and operational operators
In the proposed FIS model triangular membership function has been used in all the three FIS engines. Further, three fuzzy sets have been applied for the input and output variables and the linguistic rating variables used are ‘Best’, ‘Average’, and ‘Worst’. However, for the evaluation of Performance Index in Economic Dimension (PIED), Performance Index in Social Dimension (PISD), and the Performance Index in Environmental Dimension (PIEND) of each supplier, five fuzzy sets of membership function has been considered and linguistic variables used are ‘extremely poor’, ‘poor’, ‘average’, ‘good’, and ‘very good’. In membership function with the associated linguistic rating for the evaluation of suppliers on sub-criteria has been presented. The membership function incorporated for the evaluation of PIED, PISD, and the PIEND with respective linguistic ratings have been shown in .
(b) Fuzzy rules (KM based) and Modified Architecture of FIS engine
In this step, fuzzy rules are developed for all FIS using DM’s knowledge. A sample of rules extracted from the rule base of FIS for evaluation of supplier performance on Warranties and claim policies (CA1) criteria has been presented in . Warranties and claim policy criteria have two sub-criteria (variables) (i) Supplier recovery percentage (CA11) and (ii) Claim frequency (CA12) having three membership functions, hence the number of rules to be framed are 9. Whereas criteria Amount of past business (CA2) has three sub-criteria with three membership functions hence DM’s need to the frame (33) 27 rules. However, in this work, re-architecting of the FIS engine has been done so as to reduce the number of rules to 9 (least possible rules). Similarly, FIS engines and fuzzy rules are established for all the criteria in the economic dimension. After framing of rules, the evaluation process of suppliers has been done by DM’s by judging on each sub-criteria ().
(c) Defuzzification
Table 7. Rules examples from rule base for Warranties and claim policies criteria
Table 8. Suppliers assessment values for criteria and sub-criteria by decision makers
The output of the three distinct FIS is the fuzzified values of performance index in the respective sustainability dimension. Defuzzification of these values has been done by the Centre of Area (COM) method (EquationEq 3)(3)
(3) and corresponding crisp values have been obtained. The defuzzified values of PIED, PISD and the PIEND have been tabulated in . Based on the values of PIED, it elicits that for the economic dimension, supplier 2 has the highest performance level followed by supplier 3 and the lowest performance is marked by supplier 1. PIEND is highest for supplier 2 and has the best performance level and supplier 1 has the lowest performance level for the environmental dimension. PISD of supplier 2 is highest and supplier 1 has the least PISD indicating the best and lowest performance levels of the suppliers respectively. After carrying out discussions, DMs assigned weights as 0.5, 0.3 and 0.2 to economic, environmental and social dimensions respectively. Further using EquationEq. 4
(4)
(4) SPI of each supplier is calculated and based on SPI ranks are awarded to suppliers. FIS methodology yields, top rank to supplier 2 followed by supplier4 and supplier 3 and last rank is awarded to supplier 1.
Table 9. Performance index values in three susinability dimensions through FIS
Step 6: Sustainability Performance Index (SPI) calculation through an integrated fuzzy MCDM method.
In this step, fuzzy integrated MCDM methods have been applied for finding the SPI of suppliers. Fuzzy AHP method has been utilised for finding the weights of the criteria and their respective sub-criteria. Further, utilising these weights, the Fuzzy TOPSIS method has been applied for the assessment of the potential suppliers and determination of SPI.
4.1. Fuzzy AHP
Foremost, DM’s perform the pairwise comparison of the criteria and sub-criteria in all sustainability dimensions and assign the priority weights to each dimension. The linguistic response and its corresponding fuzzified scale used by DM’s is shown in . The results of the comparison are established in a pairwise comparison matrix. Further, consistency ratio (CR) for all the criteria in economic, environmental and social dimensions have been calculated using EquationEquation (5)(5)
(5) and has been verified as less than 0.01. The linguistic response of DM’s are converted into the corresponding TFN equivalent as shown in and a fuzzy judgement matrix has been established in this step using EquationEquation (6)
(6)
(6) . After establishing the fuzzy judgement matrix, applying the fuzzy multiplication rule as per calculations have been performed and the geometric mean values for all sustainability criteria and sub-criteria are established. Thereafter, fuzzy weights have been computed for all sustainability criteria and sub-criteria using EquationEquation (7)
(7)
(7) . Applying EquationEquation (7)
(7)
(7) the priority weights of the Attractive subcluster have been established as 0.33337 and for the Highly attractive subcluster is established as 0.66667. The CR, priority weights and fuzzified weights for Attractive subcluster in economic dimension have been shown in . Similarly, priority weights and fuzzified weights for criteria and sub-criteria under environmental and social dimensions have been established. The local and global weights of sustainability criteria in each dimension has been presented in .
Table 10. Fuzzy scale for AHP and TOPSIS
Table 11. Priority weighs for attractive economic criteria
Table 12. Global weights of sustainability criteria and sub-criteria by FAHP method
4.2. FTOPSIS
At the beginning of FTOPSIS technique, DM’s mark their responses using linguistic variables available as per .nthe response of DM’s are changed to corresponding fuzzy equivalent TFN and the fuzzy decision matrix is established as per EquationEquation (8)(8)
(8) . Further, incorporating the responses of all decision-makers a final combined fuzzy decision matrix is established using EquationEquation (9)
(9)
(9) . After the establishment of the combined fuzzy decision matrix, the values of the matrix are normalised using the EquationEquations (10)
(10)
(10) and (Equation11
(11)
(11) ). All the sub-criteria except Claim frequency and Time of supplier integration are the non-beneficial sub-criteria. As a next step, the fuzzy weights of sub-criteria calculated using FAHP are multiplied with the normalised values using EquationEquation (12)
(12)
(12) according to the fuzzy multiplication rule shown in . Further, (FPIS)
and (FNIS)
are calculated using EquationEquation (13)
(13)
(13) and EquationEquation (14)
(14)
(14) respectively for sub-criteria in each sustainability dimensions separately. Then the fuzzy distance of each alternative from FPIS and FNIS solutions are calculated using EquationEquation (15)
(15)
(15) and EquationEquation (16)
(16)
(16) respectively in each sustainability dimension and results have been presented in . Applying EquationEquation (17)
(17)
(17) the relative closeness for all suppliers has been calculated in each sustainability dimension and has been shown in . The closeness coefficient in the economic dimension represents the supplier’s performance index in the economic dimension (PIED). Similarly, closeness coefficient values of the environmental and social domains are a representation of the performance index in the environmental dimension (PIEND) and social dimension (PISD) respectively. For establishing SPI of each supplier through an integrated FMCDM approach, DMs have decided the weight of the economic dimension as 0.5, for the environmental dimension as 0.3 and for the social dimension as 0.2. Considering these weights SPI of each supplier has been calculated using EquationEquation 4
(4)
(4) . Calculated SPI values and normalised SPI values are presented in .
Table 13. SPI values of sustainability dimension through fuzzy TOPSIS
Step 7: Ranking of alternatives and selection:
In this step, the SPI of each supplier from FIS and integrated FMCDM approaches are compared for determining the final ranks of suppliers. After holding a discussion with DM’s it was decided to award equal weights (0.5) to SPI established by FIS and integrated FMCDM approaches respectively. Calculations were performed accordingly and the final SPI is calculated and based on the normalised SPI values, final ranks are awarded to the potential suppliers ().
Table 14. Final ranks of the suppliers
The top rank is attained by supplier 2 followed by supplier 4 and the last rank has been awarded to supplier 1.
5. Discussions
5.1. Sustainability criteria and sub-criteria
In the economic dimension, Warranties and claim policy criteria have been addressed by two sub-criteria. Iron and steel sector is a large scale industry and employs expensive, automatic and high-end machinery in its manufacturing process. Hence industry is delighted to have an apt warranty attached to machinery and material supplied by the supplier. Further, the efficient, simple, transparent, automated and fast claim process is desired by industry and if any supplier is offering such a claim process to the industry then the satisfaction level of the industry is enhanced. Large Amount of past business by the supplier adds to the image building of the supplier. The high amount of business also helps the supplier in developing a favourable feeling of mutual trust between supplier and industry which makes the supplier more reliable. Trusted suppliers enable organisations to outsource their non-critical activities, thus reducing internal workloads and increasing efficiencies. The industry tends to establish a strong, mutually beneficial long-term relationship with suppliers as it helps in improving sustainability performance across the supply chain, generating greater cost efficiency and also helps in the growth of the business. Further in large scale industries initial set up costs are substantial and time-consuming. But suppliers with a long association can help to offset these costs by eliminating re-tendering and renegotiation costs. Moreover, long term relationship with suppliers also provides an opportunity to industry for engaging the engage suppliers in the process of product development, development of new processes.
In Highly attractive subcluster, product development sub-criteria are of vital importance for industries. iron and steel industries have to cater to the need of new projects for which they have to develop new products. As supplier’s participation in product development projects helps to reduce the cost, improve the quality, and also provide innovative technologies (Handfield et al. Citation1999). Petersen, Handfield, and Ragatz (Citation2005) have also reported that suppliers may be involved at different stages of the new product development process. Early supplier involvement is a key coordinating process in supply chain design, product design and process design (Gentry and Savitskie Citation2008).
To compete in the global market and to withstand tough competition from its counterparts steel sector must maintain its competitive advantage. The essence of establishing a permanent competitive advantage demands the creation of value between customers through providing quality products at an acceptable cost. For this industry needs to eliminate in valued activities and maintain the least possible inventories. Therefore just in time (JIT) production system is highly desired criteria for the industry.
In the environmental dimension, ‘green warehousing’ is clustered as attractive sub-classification as supplier’s ability to maintain green warehouses helps the industry to achieve its sustainability objectives. However ‘Carbon footprint tax’ is under a highly attractive sub-cluster because the lesser carbon tax is economically beneficial and also significant in conserving the environment. Adoption of ‘Green transportation’ means by suppliers helps the industry to reduce carbon footprint tax hence is a highly attractive criterion.
In the social dimension ‘Wages’ has been subclassified as Attractive criteria and ’Human resource policy’ as Highly attractive criteria, Industry desire that the suppliers should pay salary to the employees as per the norms of government because Non-Compliance to the salary norms by suppliers can adversely affect the sustainability image of the parent organisation. Further, the industry is delighted to associate with suppliers who have a provision for providing training on sustainability issues to all employees, encourages its employees for participation in quality and sustainability improvement initiatives.
5.2. FIS and fuzzy MCDM approach
The SPI values established through the FIS approach () elicit that supplier 2 has good performance in economic and environmental dimensions but in social dimension supplier 4 has better performance. However, supplier 2 has the highest SPI and secure overall top rank among the four suppliers. Supplier 3 has performed well in economic and environmental dimension but has poor performance in social dimension and have secured the third rank. On the other hand supplier, 2 has lower performance in economic and environmental dimensions but has superior performance in the social dimension and manages to reach the second rank. Suppliers ranking based on the SPI values through FIS are S2 > S4>S3>S1.
The SPI values through the fuzzy MCDM approach () tells that supplier 2 is having the best performance in economic dimension and supplier 1 performance is lowest. However, in environmental and social dimension supplier 1 outperforms and supplier 4 has again the poorest performance in both the sustainability dimensions. Based on the SPI values through the fuzzy MCDM approach the final ranking of suppliers is S4 >S2>S3>S1. It is elicited from the results of both approaches that first and second ranks have been changed however, the third and fourth ranks are the same.
The evaluated ranks of suppliers by the FIS and fuzzy MCDM approach in each sustainability dimension are presented in . It elicits that the ranks of suppliers as established by both approaches are varying due to their inherent characteristics. In economic dimension ranks of supplier 1 and supplier 2 are the same but the ranks of supplier 3 and supplier 4 are reversed. In the environmental dimension except supplier 4, the ranks of all suppliers are different. Similarly, in social dimension supplier, 1 and supplier 3 ranks are reversed and supplier 4 and supplier 2 have no change in their ranks. The overall ranks established on SPI values of FIS and fuzzy MCDM approaches have a significant variation in the ranks of suppliers. FIS approach awards first rank to supplier 2 whereas as per the fuzzy MCDM approach supplier 4 is top-ranked. Further, As per the FIS approach supplier, 4 is having the second rank whereas the fuzzy MCDM approach awards supplier 2 with this rank. In the final awarding of ranks, supplier 2 tops the list with first rank and supplier 4 is having the second rank, supplier 3 has the third rank and supplier 1 is having the last ranking.
Table 15. Ranks position for suppliers
6. Managerial implications
The proposed SSS framework has many managerial practical implications for supply chain managers. Firstly, the proposed decision model for SSS provides managers with a framework for the SSS by evaluating the supplier’s performance on Attractive and Highly attractive criteria in three sustainability dimensions. Secondly, the proposed framework provides a common platform to consider the results of both, FIS and the fuzzy MCDM approach in a sustainability environment for evaluating the sustainability performance of potential suppliers. Thirdly, the methodology also provides deep insight into the level of perceived satisfaction by industry through the SSS process by considering sub-classified Attractive criteria. The practical application of the proposed framework in the industry helps DMs in achieving the sustainability objectives of the industry and also helps in attaining the highest level of satisfaction from the SSS process. Further, the proposed model incorporates the knowledge of managers and experts of the industry to improve the effectiveness of the SSS process and allows them to have a detailed insight into the sustainability performance of suppliers. The proposed framework considers the results of FIS and fuzzy MCDM techniques for supplier evaluation, providing more efficient and reliable results that allow the DMs to develop an effective and efficient selection process. Further, sharing and discussing the SPI values with suppliers, managers can provide the necessary inputs to help the suppliers to improve their sustainability performance. The proposed model incorporates twelve sustainability criteria and nine sub-criteria of a large scale industry. However, the framework is adaptable to incorporate different criteria and sub-criteria of other industries as per the need.
7. Conclusions and future work
Sustainable suppliers are key players in facilitating industries to attain their sustainability objectives of the supply chain. Therefore the selection of sustainable suppliers is a vital decision for industries. The present methodology provides a supplier selection framework by integrating the FIS and fuzzy MCDM approaches in the sustainability horizon. The framework incorporates Attractive and Highly Attractive sustainability criteria for sustainability performance evaluation and enhancement of perceived satisfaction by industry. The attractive cluster encompasses nine criteria in the economic dimension, five criteria under the environmental dimension and three criteria under the social dimension. Further, eight sub-criteria in economic dimension and two sub-criteria in the environmental dimension have been established and these act as an input for the FIS system and integrated fuzzy MCDM techniques respectively. SPI obtained from both approaches in the sustainability paradigm is utilised to develop a decision framework for selecting sustainable suppliers for contract allocation. The proposed methodology integrates FIS and fuzzy MCDM approaches on the sustainability platform, however, the supply chain managers can apply these approaches distinctively depending on the nature of the sustainability criteria. Managers can apply the fuzzy MCDM approach when the quantified criteria and sub-criteria are available for decision making and can utilise the FIS approach when decision framework incorporates criteria and sub-criteria of qualitative nature.
This approach has been validated by the iron and steel industry as a case company. The results of the work provide a deep insight into the performance of every supplier in each sustainability dimension. Further, the SPI of the supplier provides a means to SCM managers for taking the decision of contract allocation. SCM managers can share the results with suppliers and suppliers can self-assess their performance on sustainability dimensions and can focus on improving their sustainability performance.
The proposed model for SSS has some limitations also. Firstly, the proposed model is based on Mamdani FIS and the results significantly depend on a set of rules framed by DM’s based on their personal experience and knowledge. The number of rules increases drastically in increasing the number of inputs to FIS. Another limitation of the present work is that it incorporates the sustainability criteria relevant to the iron and steel industry of India only. Hence as future proposed work, the supply chain managers representing other industries are encouraged to develop a more comprehensive list of sustainability criteria. Further, researchers are encouraged to examine the interrelationship between sustainability criteria and their sub-criteria using ANP. As a proposed future research work, academicians and researchers can take up the task of developing a mathematical model for order allocation to selected suppliers considering the constraints in each sustainability dimension.
Disclosure Statement
No potential conflict of interest was reported by the authors.
Additional information
Notes on contributors
Naveen Jain
Naveen Jain is a research scholar at Mechanical Engineering department of National Institute of Technology, Raipur, India. He has completed his Bachelor of Engineering in Mechanical Engineering from Barkatullah University; Bhopal, India. He holds Masters in Production Engineering. His research areas are sustainability, sustainable supply chain management, multi criteria decision making and optimization. He has published papers in International and national journals and has presented papers in International conferences.
A. R. Singh
Dr. A. R. Singh is Asssistant Professor in the Mechanical Engineering department of National Institute of Technology, Raipur, India. He completed his Bachelor of Engineering degree in Mechanical engineering from U.P.T.U, University. He has Master of Technology degree in Production Engineering from Motilal Nehru National Institute of Technology, Allahabad, India. He holds a PhD in Mechanical Engineering from Motilal Nehru National Institute of Technology, Allahabad, India. His areas of specialization are operation research, supply chain management, optimization techniques and multi criteria decision making. He has published many papers in International journals. Some of the international journals in which papers are published include – International Journal of Advanced Manufacturing Technology, International journal of multi criteria decision making, Journal of intelligent manufacturing, International Journal of Design Engineering, International journal of manufacturing systems, Applied Mathematics and Computation.
R. K. Upadhyay
Dr. R. K. Upadhyay has served as a Professor in Mechanical Engg Department at Anand Engineering College Agra and as Faculty Member in Department of Mechanical Engg, DEI, Dayalbagh, Agra. He is an Academic Veteran, Technocrat cum Researcher, Mentor and Practicing Entrepreneurship Facilitator with demonstrated history of working actively in the area of Subconscious and Emotional Intelligence, Peace of Mind, Motivation and Effective problem solving at workplaces. He has conducted many interesting and thought provoking sessions for students, parents, educational institutes and corporate sectors. Additionally, he has delivered brainstorming session on cutting-edge technologies Industry 4.0 which comprises of smart manufacturing, mixed reality and automotive manufacturing. Dr. Upadhyay is also a renowned author, His books on Workshop Practice (2001) and Manufacturing Science (2004) were highly appreciated by student’s community of U.P.T.U. (now A.K.T.U.) Lucknow.
References
- Agarwal, P., M. Sahai, V. Mishra, M. Bag, and V. Singh. 2011. “A Review of Multi-Criteria Decision Making Techniques for Supplier Evaluation and Selection.” International Journal of Industrial Engineering Computations 2 (4): 801–810. doi:10.5267/j.ijiec.
- Ahmadi, H. B., S. Hamid, H. Petrudi, and X. Wang. 2017. “Integrating Sustainability into Supplier Selection with Analytical Hierarchy Process and Improved Grey Relational Analysis: A Case of Telecom Industry.” The International Journal of Advanced Manufacturing Technology 90 (9–12): 2413–2427. doi:10.1007/s00170-016-9518-z.
- Amindoust, A., and A. Saghafinia. 2016. “Textile Supplier Selection in Sustainable Supply Chain Using a Modular Fuzzy Inference System Model.” The Journal of the Textile Institute, October. 1–9. doi:10.1080/00405000.2016.1238130.
- Amindoust, A., S. Ahmed, A. Saghafinia, and A. Bahreininejad. 2012. “Sustainable Supplier Selection: A Ranking Model Based on Fuzzy Inference System.” Applied Soft Computing 12 (6). doi:10.1016/j.asoc.2012.01.023.
- Awasthi, A., K. Govindan, and S. Gold. 2018. “Multi-tier Sustainable Global Supplier Selection Using a Fuzzy Ahp-vikor Based Approach.” International Journal of Production Economics 195: 106–117. doi:10.1016/j.ijpe.2017.10.013.
- Azadnia, A. H., P. Ghadimi, M. Z. M. Saman, K. Y. Wong, and C. Heavey. 2013. “An Integrated Approach for Sustainable Supplier Selection Using Fuzzy Logic and Fuzzy AHP.” Applied Mechanics and Materials 315 (April): 206–210. doi:10.4028/www.scientific.net/AMM.315.206.
- Azadnia, A.H., M.Z.M. Saman, and K.Y. Wong. 2015. “Sustainable Supplier Selection and order Lot-sizing: an Integrated Multi-objective Decision-making Process.” International Journal of Production Research 53 (2): 383–408. doi:10.1080/00207543.2014.935827.
- Bai, C., and J. Sarkis. 2010. “Green Supplier Development : Analytical Evaluation Using Rough Set Theory.” Journal of Cleaner Production 18 (12). doi:10.1016/j.jclepro.2010.01.016.
- Banaeian, N., H. Mobli, B. Fahimnia, I. E. Nielsen, and M. Omid. 2016. “Green Supplier Selection Using Fuzzy Group Decision Making Methods: A Case Study from the Agri-Food Industry.” Computers & Operations Research 89: 337–347. doi:10.1016/j.cor.2016.02.015.
- Blischke, W. 1995. Product Warranty Handbook. New York, NY: CRC Press.
- Bruntland, G. 1987. “World Commission on Environment and Development (WCED).” In Our Common Future 17, pp.1–91.
- Büyüközkan, G., and Ç. Gizem. 2011. “A Novel Fuzzy Multi-Criteria Decision Framework for Sustainable Supplier Selection with Incomplete Information.” Computers in Industry 62 (2): 164–174. doi:10.1016/j.compind.2010.10.009.
- Chaharsooghi, S. K., and M. Ashrafi. 2014. “Sustainable Supplier Performance Evaluation and Selection with Neofuzzy TOPSIS Method.” International Scholarly Research Notices 2014: 1–10. doi:10.1155/2014/434168.
- Chai, J., J. N. K. Liu, and E. W. T. Ngai. 2013. “Application of Decision-Making Techniques in Supplier Selection: A Systematic Review of Literature.” Expert Systems with Applications 40 (10): 3872–3885. doi:10.1016/j.eswa.2012.12.040.
- Cheraghalipour, A., M. M. Paydar, and M. H. Keshteli. 2018. “Applying A Hybrid BWM-VIKOR Approach to Supplier Selection: A Case Study in the Iranian Agricultural Implements Industry.” International Journal of Applied Decision Sciences 11 (3): 274. doi:10.1504/IJADS.2018.092796.
- Cheraghalipour, A., and S. Farsad. 2018. “A Bi-Objective Sustainable Supplier Selection and Order Allocation considering Quantity Discounts under Disruption Risks: A Case Study in Plastic Industry.” Computers and Industrial Engineering 118: 237–250. doi:10.1016/j.cie.2018.02.041.
- Dace, E., A. Stibe, and L. Timma. 2019. “A Holistic Approach to Manage Environmental Quality by Using the Kano Model and Social Cognitive Theory.” In Corporate Social Responsibility and Environmental Management 2020;27: 430–443. Wiley Online Library. https://doi.org/10.1002/csr.1828
- Dickson, G. W. 1966. “An Analysis of Vendor Selection and the Buying Process.” Journal of Purchasing 2 (1): 5–17. doi:10.1111/j.1745-493X.1966.tb00818.x.
- Dinçer, H., S. Yüksel, and F. Pınarbaşı. 2020. “Kano-Based Measurement of Customer Expectations in Retail Service Industry Using IT2 DEMATEL-QUALIFLEX.” In Handbook of Research on Positive Organizational Behavior for Improved Workplace Performance, edited by Elif Baykal (Istanbul Medipol University, Turkey). 349–370. IGI Global. doi:10.4018/978-1-7998-0058-3.ch020
- Fallahpour, A., E. U. Olugu, S. N. Musa, K. Y. Wong, and S. Noori. 2017. “A Decision Support Model for Sustainable Supplier Selection in Sustainable Supply Chain Management.” Computers and Industrial Engineering 105: 391–410. doi:10.1016/j.cie.2017.01.005.
- Gentry, L., and K. Savitskie. 2008. “Understanding the Supplier’s Role in New Product Development: How to Achieve Competitive Advantage in the Supply Chain.” Academy of Taiwan Business Management Review 4 (1): 17–27.
- Ghadimi, P., A. Dargi, and C. Heavey. 2017. “Sustainable Supplier Performance Scoring Using Audition Check-List Based Fuzzy Inference System: A Case Application in Automotive Spare Part Industry.” Computers & Industrial Engineering 105 (March): 12–27. doi:10.1016/j.cie.2017.01.002.
- Ghadimi, P., C. Wang, M. K. Lim, and C. Heavey. 2019. “Intelligent Sustainable Supplier Selection Using Multi-Agent Technology: Theory and Application for Industry 4.0 Supply Chains.” Computers and Industrial Engineering 127 (October): 588–600. doi:10.1016/j.cie.2018.10.050.
- Giannakis, M., R. Dubey, I. Vlachos, and J. Yanbing. 2020. “Supplier Sustainability Performance Evaluation Using the Analytic Network Process.” Journal of Cleaner Production 247 (February): 119439. doi:10.1016/j.jclepro.2019.119439.
- Govindan, K., R. Khodaverdi, and A. Jafarian. 2013. “A Fuzzy Multi Criteria Approach for Measuring Sustainability Performance of A Supplie.” Journal of Cleaner Production 47: 345–354. doi:10.1016/j.jclepro.2012.04.014.
- Govindan, K., S. Rajendran, J. Sarkis, and P. Murugesan. 2015. “Multi Criteria Decision Making Approaches for Green Supplier Evaluation and Selection: A Literature Review.” Journal of Cleaner Production 98 (July): 66–83. doi:10.1016/j.jclepro.2013.06.046.
- Handfield, R. B., G. L. Ragatz, K. J. Petersen, and R. M. Monczka. 1999. “Involving Suppliers in New Product Development.” California Management Review 42 (1): 59–82. SAGE Publications Sage CA: Los Angeles, CA. doi: 10.2307/41166019.
- Hassini, E., C. Surti, and C. Searcy. 2012. “A Literature Review and A Case Study of Sustainable Supply Chains with A Focus on Metrics.” International Journal of Production Economics 140 (1): 69–82. doi:10.1016/j.ijpe.2012.01.042.
- Ho, W., X. Xiaowei, and P. K. Dey. 2010. “Multi-Criteria Decision Making Approaches for Supplier Evaluation and Selection: A Literature Review.” European Journal of Operational Research 202 (1): 16–24. doi:10.1016/j.ejor.2009.05.009.
- Humphreys, P. K., Y. K. Wong, and F. T. S. Chan. 2003. “Integrating Environmental Criteria into the Supplier Selection Process.” Journal of Materials Processing Technology 138 (1–3): 349–356. doi:10.1016/S0924-0136(03)00097-9.
- Hussain, M., and R. Al-Aomar. 2018. “A Model for Assessing the Impact of Sustainable Supplier Selection on the Performance of Service Supply Chains.” International Journal of Sustainable Engineering 11: 366–381. doi:10.1080/19397038.2017.1414898.
- Igarashi, M., L. De Boer, and A. M. Fet. 2013. “What Is Required for Greener Supplier Selection? A Literature Review and Conceptual Model Development.” Journal of Purchasing and Supply Management 19 (4): 1–17. doi:10.1016/j.pursup.2013.06.001.
- Jafarian, A. 2013. “A Fuzzy Multi Criteria Approach for Measuring Sustainability Performance of A Supplier Based on Triple Bottom Line Approach.” Journal of Cleaner Production 47 (November 2014): 345–354. doi:10.1016/j.jclepro.2012.04.014.
- Jain, N., and A. R. Singh. 2017. “Fuzzy Kano Integrated MCDM Approach for Supplier Selection Based on Must Be Criteria.” International Journal of Supply Chain Management 6 (2): 49–59. https://www.scopus.com/inward/record.uri?eid=2-s2.0-85021774128&partnerID=40&md5=22c4c2e5e90b89267ea39ea5e15c9b52.
- Jain, N., and A. R. Singh. 2019. “Sustainable Supplier Selection Criteria Classification for Indian Iron and Steel Industry: A Fuzzy Modified Kano Model Approach.” International Journal of Sustainable Engineering: 13 (1): 1–16. Taylor & Francis. doi:10.1080/19397038.2019.1566413.
- Jain, N., and A. R. Singh. 2020. “Sustainable Supplier Selection under Must-Be Criteria through Fuzzy Inference System.” Journal of Cleaner Production 248 (March): 119275. doi:10.1016/j.jclepro.2019.119275.
- Jassbi, J. J., P. J. A. Serra, R. A. Ribeiro, and A. Donati. 2006. “A Comparison of Mandani and Sugeno Inference Systems for A Space Fault Detection Application.” In 2006 World Automation Congress, Budapest, 2006, 1–8. IEEE. doi:10.1109/WAC.2006.376033.
- Jian-Wu, B., Y. Liu, Z.-P. Fan, and E. Cambria. 2019. “Modelling Customer Satisfaction from Online Reviews Using Ensemble Neural Network and Effect-Based Kano Model.” International Journal of Production Research 57 (22): 7068–7088. doi:10.1080/00207543.2019.1574989.
- Kannan, D. 2018. “Role of Multiple Stakeholders and the Critical Success Factor Theory for the Sustainable Supplier Selection Process.”International Journal of Production Economics 195: 391–418. doi:10.1016/j.ijpe.2017.02.020.
- Kar, A. K., and A. K. Pani. 2014. “Exploring the Importance of Different Supplier Selection Criteria.” Management Research Review 37 (1): 89–105. doi:10.1108/MRR-10-2012-0230.
- Khan, S.A., S. Kusi-Sarpong, F.K. Arhin, and H. Kusi-Sarpong. 2018. “Supplier Sustainability Performance Evaluation and Selection.” A Framework and Methodology.” Journal of Cleaner Production 205: 964–979. doi:10.1016/j.jclepro.2018.09.144.
- KhanMohammadi, E., H. Talaie, H. Safari, and R. Salehzadeh. 2018. “Supplier Evaluation and Selection for Sustainable Supply Chain Management under Uncertainty Conditions.” International Journal of Sustainable Engineering 11 (6): 382–396. doi:10.1080/19397038.2017.1421277.
- Khurrum, M., and S. Bhutta. 2003. “Supplier Selection Problem: Methodology Literature Review.” Journal of International Information Management 12 (2). http://scholarworks.lib.csusb.edu/jiim%0Ahttp://scholarworks.lib.csusb.edu/jiim/vol12/iss2/5.
- Kumar, D., and Z. Rahman. 2015. Sustainability Adoption through Buyer Supplier Relationship across Supply Chain: A Literature Review and Conceptual Framework. International Strategic Management Review. Vol. 3(1-2): 110-127. Holy Spirit University of Kaslik. doi:10.1016/j.ism.2015.04.002.
- Kuo, R. J., Y. C. Wang, and F. C. Tien. 2010. “Integration of Artificial Neural Network and MADA Methods for Green Supplier Selection.” Journal of Cleaner Production 18 (12): 1161–1170. doi:10.1016/j.jclepro.2010.03.020.
- Liu, A., Y. Xiao, L. Hui, S.-B. Tsai, and W. Song. 2019. “A Fuzzy Three-Stage Multi-Attribute Decision-Making Approach Based on Customer Needs for Sustainable Supplier Selection.” Journal of Cleaner Production 239 (December): 118043. doi:10.1016/j.jclepro.2019.118043.
- Lucadamo, A., I. Camminatiello, and A. D’Ambra. 2020. “A Statistical Model for Evaluating the Patient Satisfaction.” Socio-economic Planning Sciences: 100797. Elsevier. doi:10.1016/j.seps.2020.100797
- Luthra, S., D. Garg, and A. Haleem. 2015. “An Analysis of Interactions among Critical Success Factors to Implement Green Supply Chain Management Towards Sustainability: An Indian Perspective.” Resources Policy 46: 37–50. doi:10.1016/j.resourpol.2014.12.006.
- Luthra, S., K. Govindan, D. Kannan, S. K. Mangla, and C. P. Garg. 2017. “An Integrated Framework for Sustainable Supplier Selection and Evaluation in Supply Chains.” Journal of Cleaner Production 140 (January): 1686–1698. doi:10.1016/j.jclepro.2016.09.078.
- Mamdani, E. H. 1974. “Application of Fuzzy Algorithms for Control of Simple Dynamic Plant.” Proceedings of the Institution of Electrical Engineers 121 (12): 1585. doi:10.1049/piee.1974.0328.
- Mardani, A., A. Jusoh, K. M. D. Nor, Z. Khalifah, N. Zakwan, and A. Valipour. 2015. “Multiple Criteria Decision-Making Techniques and Their Applications–a Review of the Literature from 2000 to 2014.” Economic Research-Ekonomska Istraživanja 28 (1): 516–571. doi:10.1080/1331677X.2015.1075139.
- Materla, T., E. A. Cudney, and J. Antony. 2019. “The Application of Kano Model in the Healthcare Industry: A Systematic Literature Review.” Total Quality Management & Business Excellence 30 (5–6): 660–681. doi:10.1080/14783363.2017.1328980.
- Mavi, R. K., M. Goh, and N. Zarbakhshnia. 2017. “Sustainable Third-Party Reverse Logistic Provider Selection with Fuzzy SWARA and Fuzzy MOORA in Plastic Industry.” The International Journal of Advanced Manufacturing Technology 91 (5–8): 2401–2418. doi:10.1007/s00170-016-9880-x.
- Memari, A., A. Dargi, M. R. A. Jokar, R. Ahmad, and A. R. A. Rahim. 2019. “Sustainable Supplier Selection: A Multi-Criteria Intuitionistic Fuzzy TOPSIS Method.” Journal of Manufacturing Systems 50: 9–24. (April 2018). doi:10.1016/j.jmsy.2018.11.002.
- Min-Yuan, M., C.-W. Chen, and Y.-M. Chang. 2019. “Using Kano Model to Differentiate between Future Vehicle-Driving Services.” International Journal of Industrial Ergonomics 69: 142–152. doi:10.1016/j.ergon.2018.11.003.
- Mohammed, A., I. Harris, and K. Govindan. 2019. “A Hybrid MCDM-FMOO Approach for Sustainable Supplier Selection and order Allocation.” International Journal of Production Economics 217: 171–184. doi:10.1016/j.ijpe.2019.02.003.
- Nielsen, I. E., N. Banaeian, P. Golin, H. Mobli, and M. Omid. 2014. “Logistics Operations, Supply Chain Management and Sustainability.” In Logistics Operations, Supply Chain Management and Sustainability, edited by P. Golinska. Cham: EcoProduction: (79–99). doi:10.1007/978-3-319-07287-6.
- Noci, G. 1997. “Designing ‘Green’ Vendor Rating Systems for the Assessment of a Supplier’s Environmental Performance.” European Journal of Purchasing & Supply Management 3 (2): 103–114. doi:10.1016/S0969-7012(96)00021-4.
- Orji, I. J., and S. Wei. 2015. “An Innovative Integration of Fuzzy-Logic and Systems Dynamics in Sustainable Supplier Selection: A Case on Manufacturing Industry.” Computers and Industrial Engineering 88: 1–12. doi:10.1016/j.cie.2015.06.019.
- Petersen, K. J., R. B. Handfield, and G. L. Ragatz. 2005. “Supplier Integration into New Product Development: Coordinating Product, Process and Supply Chain Design.” Journal of Operations Management 23 (3–4): 371–388. doi:10.1016/j.jom.2004.07.009.
- Pishchulov, G., A. Trautrims, T. Chesney, S. Gold, and L. Schwab. 2019. “The Voting Analytic Hierarchy Process Revisited: a Revised Method with Application to Sustainable Supplier Selection.” International Journal of Production Economics 211 (May): 166–179. doi:10.1016/j.ijpe.2019.01.025.
- Pourjavad, E., and A. Shahin. 2018. “The Application of Mamdani Fuzzy Inference System in Evaluating Green Supply Chain Management Performance.” International Journal of Fuzzy Systems 20 (3): 901–912. doi:10.1007/s40815-017-0378-y.
- Rao, C., M. Goh, and J. Zheng. 2017. “Decision Mechanism for Supplier Selection under Sustainability.” International Journal of Information Technology & Decision Making 16 (1): 87–115. doi:10.1142/S0219622016500450.
- Rashidi, K., and K. Cullinane. 2019. “A Comparison of Fuzzy DEA and Fuzzy TOPSIS in Sustainable Supplier Selection: Implications for Sourcing Strategy.” Expert Systems with Applications 121: 266–281. doi:10.1016/j.eswa.2018.12.025.
- Roy, S. A., S. M. Ali, G. Kabir, R. Enayet, S. A. Suhi, T. Haque, and R. Hasan. 2019. “A Framework for Sustainable Supplier Selection with Transportation Criteria.” International Journal of Sustainable Engineering, June. 1–16. doi:10.1080/19397038.2019.1625983.
- Saxena, A., M. Prasad, A. Gupta, N. Bharill, O. P. Patel, A. Tiwari, M. J. Er, W. Ding, and C.-T. Lin. 2017. “A Review of Clustering Techniques and Developments.” Neurocomputing 267: 664–681. doi:10.1016/j.neucom.2017.06.053.
- Seuring, S., and M. Muller. 2008. “From a Literature Review to a Conceptual Framework for Sustainable Supply Chain Management.” Journal of Cleaner Production 16 (15): 1699–1710. doi:10.1016/j.jclepro.2008.04.020.
- Shahin, A., M. Pourhamidi, J. Antony, and S. H. Park. 2013. “Typology of Kano Models: A Critical Review of Literature and Proposition of A Revised Model.” International Journal of Quality and Reliability Management 30 (3): 341–358. doi:10.1108/02656711311299863.
- Shalke, N., M. Parvin, M. Paydar, and M. Hajiaghaei-Keshteli. 2018. “Sustainable Supplier Selection and Order Allocation through Quantity Discounts.” International Journal of Management Science and Engineering Management 13 (1): 20–32. doi:10.1080/17509653.2016.1269246.
- Shaw, K., R. Shankar, S. S. Yadav, and L. S. Thakur. 2012. “Supplier Selection Using Fuzzy AHP and Fuzzy Multi-Objective Linear Programming for Developing Low Carbon Supply Chain.” Expert Systems with Applications 39 (9): 8182–8192. doi:10.1016/j.eswa.2012.01.149.
- Shen, L., L. Olfat, K. Govindan, R. Khodaverdi, and A. Diabat. 2013. “A Fuzzy Multi Criteria Approach for Evaluating Green Supplier’s Performance in Green Supply Chain with Linguistic Preferences.” Resources, Conservation and Recycling 74: 170–179. Elsevier B.V. doi:10.1016/j.resconrec.2012.09.006.
- Singh, S., E. U. Olugu, and A. Fallahpour. 2014. “Fuzzy-Based Sustainable Manufacturing Assessment Model for SMEs.” Clean Technologies and Environmental Policy 16 (5): 847–860. doi:10.1007/s10098-013-0676-5.
- Stetter, R. 2020. “Methodical and Model-Based Design of Automated Vehicles.” In Fault-Tolerant Design and Control of Automated Vehicles and Processes, 59–91. Switzerland: Springer Nature.
- Tavana, M., M. Yazdani, and D. Di Caprio. 2016. “An Application of an Integrated ANP – QFD Framework for Sustainable Supplier Selection.” International Journal of Logistics Research and Applications 20 (3): 254–275. doi:10.1080/13675567.2016.1219702.
- Terlunen, S., G. Barreto, and B. Hellingrath. 2015. “Application and Evaluation of Multi-Criteria Clustering Algorithms for Customer-Oriented Supply Chain Segmentation.” In Logistics Management, 121–133. Switzerland: Springer International Publishing.
- Vinodh, S., G. Mulanjur, and A. Thiagarajan. 2013. “Sustainable Concept Selection Using Modified Fuzzy TOPSIS: A Case Study.” International Journal of Sustainable Engineering 6 (2): 109–116. doi:10.1080/19397038.2012.682100.
- Ware, N. R., S. P. Singh, and D. K. Banwet. 2012. “Supplier Selection Problem: A State-of-the-Art Review.” Management Science Letters 2 (5): 1465–1490. doi:10.5267/j.msl.2012.05.007.
- Watson, G. 2019. “Using the Kano Model as a Basis for Strategic Thinking.” The Journal for Quality and Participation 42 (3): 8–14. American Society for Quality
- Weber, C. A. 1991. A Decision Support System Using Multi-Criteria Techniques for Vendor Selection. Ohio, Columbus: Ohio State University.
- Weber, C. A., J. R. Current, and W. C. Benton. 1991. “Vendor Selection Criteria and Methods.” European Journal of Operational Research 50 (1): 2–18. doi:10.1016/0377-2217(91)90033-R.
- Wilson, E. J. 1994. “The Relative Importance of Supplier Selection Criteria: A Review and Update.” International Journal of Purchasing and Materials Management 30 (2): 34–41. doi:10.1111/j.1745-493X.1994.tb00195.x.
- Winter, S., and R. Lasch. 2016. “Environmental and Social Criteria in Supplier Evaluation – Lessons from the Fashion and Apparel Industry.” Journal of Cleaner Production 139: 175–190. doi:10.1016/j.jclepro.2016.07.201.
- Wong, A., T. Tsuen, and M. W-M. Ho. 2019. “Service Quality and Customer Satisfaction on Budget Airlines: Kano Model Approach.” Journal of Economics, Management and Trade 24 (5): 1–16. doi: 10.9734/jemt/2019/v24i530178.
- Yazdani, M., P. Chatterjee, and A. E. Torkayesh. 2020. “An Integrated AHP-QFD-Based Compromise Ranking Model for Sustainable Supplier Selection.” In Handbook of Research on Interdisciplinary Approaches to Decision Making for Sustainable Supply Chains, edited by Anjali Awasthi (Concordia University, Canada) and Katarzyna Grzybowska (Poznan University of Technology, Poland), 32–54. Chocolate Ave.Hershey, PA: IGI Global.
- Yu, C., Y. Shao, K. Wang, and L. Zhang. 2019. “A Group Decision Making Sustainable Supplier Selection Approach Using Extended TOPSIS under Interval-Valued Pythagorean Fuzzy Environment.” Expert Systems with Applications 121: 1–17. doi:10.1016/j.eswa.2018.12.010.
- Zadeh, L. A. 1965. Fuzzy Set Theory and Its Applications. Dordrecht: Kluwer Acad. Publ.
- Zimmer, K., M. Fröhling, and F. Schultmann. 2016. “Sustainable Supplier Management – A Review of Models Supporting Sustainable Supplier Selection, Monitoring and Development.” International Journal of Production Research 54 (5): 1412–1442. doi:10.1080/00207543.2015.1079340.