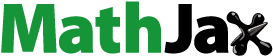
ABSTRACT
The objective of this research is to identify and rank the solutions to mitigate the sustainable remanufacturing supply chain (RSC) risks effectively. Risks and the solutions are identified based on a literature survey and discussion with the expert’s panel. A hybrid multi-criteria decision making (MCDM) framework using fuzzy step-wise weight assessment ratio analysis (SWARA) and fuzzy complex proportional assessment of alternatives (COPRAS) is applied to analyse the risks and the solutions. Fuzzy SWARA is used to obtain relative weights of each risk and fuzzy COPRAS is used to rank and select the most efficient solution to manage the risks. The proposed framework was numerically applied to a case organisation involved in manufacturing/remanufacturing activities. The results indicate that initiatives from the management and modification in organisation goals is the top-ranked solution that would mitigate the majority of risks. Government, manufacturer and distributor collaboration to develop action plans and setting up international standards are also prominent solutions to overcome the risks. Further, this research performs a sensitivity analysis to check the robustness of the proposed fuzzy SWARA-fuzzy COPRAS framework. The findings and implications of this research will help the practitioners and researchers for decision-making in RSC.
1. Introduction
The focus on the adoption of sustainable practices in the supply chain is on the rise in recent years due to increased attention from society, government and non-government institutions towards deteriorating environment (Luthra and Mangla Citation2018). Decision-makers need to focus on active consideration of environmental issues across the supply chain activities (Lin, Tseng, and Pai Citation2018). In this regard, the adoption of supply chain remanufacturing is being seen as a potential approach to move towards sustainability, as its implementation helps generate economic, social and environmental benefits (Seitz Citation2007). Sustainable advantages such as energy conservation, emission reduction (Li et al. Citation2019); increased profitability, reduced land disposal and raw material savings (Chakraborty, Mondal, and Mukherjee Citation2017) can be observed due to supply chain remanufacturing. According to Rathore, Kota, and Chakrabarti (Citation2011), a remanufactured product saves 40% energy and 30% raw material in comparison to the requirement of new product from new materials. India has huge potential to derive sustainable benefits by encouraging business organisations to integrate remanufacturing practices in their supply chain due to reasons such as the second most populated country, availability of technical skills and abundant labour (Bhatia, Rajiv, and Srivastava Citation2018). Supply chain remanufacturing has a history of more than 50 years in some developed countries; however, it is still at a nascent stage in many developing countries (Zhang, Yang, and Chen Citation2017). In India, supply chain remanufacturing related activities are still evolving, and in fact only a marginal growth has been reported in the last 15 years (Mukherjee and Mondal Citation2009; Bhatia, Rajiv, and Srivastava Citation2018). Indian organisations are facing a number of remanufacturing supply chain (RSC) risks which restrict the adoption of remanufacturing. Organisations need to develop appropriate strategies and capabilities to overcome these RSC risks. Tang and Nurmaya Musa (Citation2011) suggest that a comprehensive understanding of what risk is, where it exists and what are the ways to overcome these risks, would improve the supply chain competence in the present challenging business environment. It is therefore significant to manage and mitigate the risks involved in the implementation of sustainable RSC. Hence, it needed to focus on developing solutions and risk mitigation strategies to help the decision-makers for the effectiveness of sustainable RSC.
1.1 Research motives
Sustenance of today’s supply chain has become very difficult as it is exposed to many global factors such as uncertainties related to currency rates, crude oil prices and competition between nations to become market leaders. In addition to the global factors, RSC itself is a highly uncertain process due to issues related to the acquisition of core and its timing, labour availability, cores conditions and market demand (Wei et al. Citation2015; Zhang, Yang, and Chen Citation2017). These issues would definitely create problems in managing the supply chain effectively. A risk associated with the supply chain would significantly impact the organisational performance in terms of a process failure, product performance, supply issues, increased negative environmental impact, decreased organisational performance and even failure of business (Mangla, Kumar, and Barua Citation2015). Christopher and Lee (Citation2004) suggest that a supply chain cannot succeed with associated high risk. In this era, an organisation that only focusses on higher efficiency but disregards risk management would be a failure (Rostamzadeh et al. Citation2018). Indian remanufacturers are yet to make remarkable progress in remanufacturing in comparison to the US and are exposed to a number of challenges (Bhatia, Rajiv, and Srivastava Citation2018). For effective functioning of RSC, the practitioners/decision-makers need to understand the risks involved and their effects, to contribute towards the social-economical-environmental performance. In this context, some studies were conducted by Indian scholars as well as research scholars of other nations to explore the research field ().
Table 1. Summary of research works on remanufacturing supply chain
Majority of the Indian studies either explored the drivers/motivating factors/initiatives (Kannan, Diabat, and Madan Shankar Citation2014; Das and Chaudhari Citation2015; Sharma, Garg, and Sharma Citation2016a, Citation2016b; Chakraborty, Mondal, and Mukherjee Citation2017) or barrier/issues (Mukherjee and Mondal Citation2009; Govindan, Shankar, and Kannan Citation2016; Sinha et al. Citation2017; Bhatia, Rajiv, and Srivastava Citation2018) of RSC. The research scholars of other nations too focussed the research field for either driver analysis or barrier analysis. Hence, research that evaluates the risk issues and the solutions to overcome these risks in supply chain remanufacturing are limited. In the context of problem focus, several aspects related to supply chain remanufacturing have been analysed such as product-service system improvement, consumer’s role, EOL product management and operational or financial issues. However, the evaluation of supply chain remanufacturing risks with the main concentration on sustainability concept (economic, social and environmental dimension) is limited. Also, the majority of studies have employed a single MCDM technique for criteria analysis, i.e. either to prioritise or to derive interrelationship between the criteria. The use of a hybrid MCDM approach in RSC is limited, but recently few studies (Govindan, Shankar, and Kannan Citation2016; Chakraborty, Mondal, and Mukherjee Citation2017) were reported. Hence, the application of an integrated MCDM approach to analyse RSC can be an issue of interest among the research community. It is clear that that there is a sufficient knowledge gap in the context of sustainable RSC risk analysis and their solutions. Hence, to systematically manage the sustainable RSC risks and enhance the adoption of the remanufacturing practices a set of appropriate risk mitigation strategies (solutions) needs to be identified and ranked.
1.2 Research objectives
The objectives of the present research field are two-fold and they are: (1) To recognise and propose the solutions to manage the risks associated with sustainable RSC implementation (2) To analyse the solutions for determining their rank to effectively manage and minimise the effect of risks associated with sustainable RSC in a systematic manner.
To achieve the above-mentioned objectives, this study proposes a hybrid multi-criteria decision-making model (MCDM) framework using fuzzy step-wise weight assessment ratio analysis (SWARA) and fuzzy complex proportional assessment of alternatives (COPRAS). A fuzzy SWARA technique is used to analyse the sustainable RSC risks and determine their relative weights. Further, a fuzzy COPRAS technique is proposed to rank the solutions to overcome these risks. SWARA is an important technique that helps decision-makers to evaluate conflicting criteria of a supply chain and determine their weights, especially when most of them are conflicting in nature. In comparison to other MCDM methods, SWARA technique is simple for experts to understand and apply their knowledge and skills; and requires less computational time (Mardani et al. Citation2017). In addition, the integration of COPRAS in the framework helps to evaluate the set of alternatives against the criteria based on how significant each alternative is and determines the best alternative. SWARA and COPRAS, a new MCDM integrated approach has been used by several researchers in different fields such as green supplier selection (Rajesh and Malliga Citation2018); third-party reverse logistics provider selection (Zarbakhshnia, Soleimani, and Ghaderi Citation2018); ranking of high tech industry (Hashemkhani Zolfani and Bahrami Citation2014); ranking the best machine tool (Aghdaie et al. Citation2013), etc., for decision-making. Furthermore, an Indian remanufacturing case organisation is selected to demonstrate the applicability of the proposed hybrid fuzzy SWARA-fuzzy COPRAS framework approach.
The rest of the article is arranged as follows: Section 2 presents the literature review on supply chain remanufacturing. Section 3 presents the research methods and the proposed hybrid framework. The application of the hybrid framework to a case company is carried out in Section 4. Discussion of the results is presented in Section 5. Section 6 presents the sensitivity analysis and finally, the study is concluded in Section 7.
2. Literature review
This section reviews the published articles that are most relevant to the present research objectives. To identify these articles, this research follows a systematic process.
Database selection for article search: In the first step, SCOPUS, a large database publishing research related to scientific, engineering, social science, etc., are considered for the article search process. Additionally, major publishers such as Elsevier, Taylor and Francis, Springer, Emerald, and Wiley were also accessed.
Keywords to be searched in the database: The structured keywords were decided according to the objectives of the present research. Hence, the keywords used for review of the article are ‘Remanufacturing and India’, ‘Remanufacturing and Barrier’, ‘Remanufacturing Supply Chain and Risk’ and ‘Remanufacturing and Case study’. SCOPUS database was searched based on these keywords reflecting in ‘Title, Abstract and Keywords’.
Finalisation of the relevant articles: Articles published in conference, book chapters, short communicated articles, etc., were eliminated to maintain the quality of the paper. Then, based on article title, abstract and keywords, full paper analysis was performed. Lastly, articles relevant to the objectives of the present research were finalised. The finalisation of articles was focussed on supply chain remanufacturing, associated risk and its solution.
The finalised articles are discussed in this research to explore the work done by the researchers.
2.1 Supply chain remanufacturing
This sub-section discusses the contribution of several research scholars to analyse supply chain remanufacturing problems. Although most of the research has been conducted in the context of China and India, several other countries have also significantly contributed to analysing RSC problems.
Ijomah (Citation2009) describes key decision-making issues that impede remanufacturing operations as well as in designing the products for remanufacturing. Mukherjee and Mondal (Citation2009) analyse the inter-relationship between the key remanufacturing process management issues. They suggest the impact of the workplace environment as the most prominent enabler during the remanufacturing decision-making process. Kuo et al. (Citation2010) identify the barriers for a product-service system with a focus on maintenance and remanufacturing. The findings indicate three major challenges for the product-service system (PSS), namely lack of support from related laws and regulations, lack of awareness related to PSS and load increase of maintenance service system. Alimoradi, Yussuf, and Zulkifli (Citation2011) solve the remanufacturing facility location decision-making problem using fuzzy TOPSIS. Their evaluation is based to select best place for effective recovery. Ghazalli and Murata (Citation2011) propose an integrated approach to determine the remanufacturing strategy for EOL products. They select the best EOL option for the parts and components of the used product. Zhang et al. (Citation2011) analyse the barriers before remanufacturing, during remanufacturing and after remanufacturing. Their findings suggest that government, industry, universities and research institutes jointly working with the sector would enhance system performance. Kannan, Diabat, and Madan Shankar (Citation2014) develop a framework to analyse the drivers of end-of-life (EOL) tire management. The findings suggest that environmental conservation is the most influencing driver for EOL management.
Das and Chaudhari (Citation2015) identify the motivating factors and impeding factors to pursue reverse supply chain initiatives. The findings of the study suggest that economic benefit is the prime reason for the organisations to adopt reverse supply chain functions. Xia, Govindan, and Zhu (Citation2015) develop a framework to evaluate the internal barriers for a Chinese automotive parts remanufacturer. Based on the analysis performed, lack of funds for research in new technologies has a high influence on remanufacturing implementation. Wei et al. (Citation2015) identify the drivers and the barriers for remanufacturing in the Chinese context. Their findings reveal that environmental and ethical responsibility, customer orientation and strategic advantage are the three most important drivers, while customer recognition is the most crucial barrier. Widera and Seliger (Citation2015) aim to reduce the complexity of the original equipment manufacturer (OEM) by exploring different potentials for competitive remanufacturing. They identify case-specific challenges to overcome the complexity. Zhu, Sarkis, and Lai (Citation2015) identify the strategic and operational barriers for Chinese truck RSC. Their results reveal lack of strong financial support for remanufacturing technologies or equipment updates and innovation as the key barriers. Govindan, Shankar, and Kannan (Citation2016) analyse the inter-relationships between the main barriers for an Indian auto parts remanufacturer. The results indicate that higher cost and lack of consumer acceptance are the most prominent barriers for Indian auto parts remanufacturing sector. Matsumoto et al. (Citation2016) explore the enablers and impeding factors for remanufacturing. They identified four research and development related issues for remanufacturing, namely product design for remanufacturing, additive manufacturing for remanufacturing, operations management in remanufacturing, and business models for remanufacturing.
Sharma, Garg, and Sharma (Citation2016a) propose a set of remanufacturing initiatives to improve remanufacturing practices implementation. Chakraborty, Mondal, and Mukherjee (Citation2017) aim to improve the remanufacturability of the product by identifying the product design characteristics and analysing them. Their research analysis found that the success of the remanufacturing process largely depends on the cleaning operation. Ilgin (Citation2017) focussed on the selection of the most appropriate used product to be collected by third-party reverse logistics providers for remanufacturing. Karvonen et al. (Citation2017) identify the barriers for remanufacturing business and process. The study provides the multi-stakeholders a set of solutions to overcome the barriers. Sinha et al. (Citation2017) empirically investigate an Indian automobile industry to explore the issues that restrict remanufacturing growth. They identify key barriers that are inhibiting the growth of automotive remanufacturing business in India. Zhang, Yang, and Chen (Citation2017) investigate the remanufacturing development status of Chinese automotive parts remanufacturers and explores the crucial challenges faced by this sector. Their research analysis suggests that poor support from the government is harming the automotive remanufactured product business. Bhatia, Rajiv, and Srivastava (Citation2018) analyse the external barriers of remanufacturing. Their findings suggest lack of channels to collect used products and customer unwillingness to return the products as the major barriers. Matsumoto, Chinen, and Endo (Citation2018) investigate the factors that impact the consumer’s intention to purchase remanufactured auto parts (RAPs). The authors found that consumers’ knowledge and perceived benefits of RAPs positively affect consumers’ purchase intentions of remanufactured auto parts. Ansari, Kant, and Shankar (Citation2019) prioritised the performance outcomes due to the adoption of supply chain remanufacturing critical success factors (CSFs) using hybrid fuzzy AHP-fuzzy TOPSIS approach. Toke and Kalpande (Citation2019) prioritise the CSFs of green manufacturing using AHP to achieve sustainability. They found top management commitment as the most important factor. presents the summary of research works performed to analyse supply chain remanufacturing in the context of the main focus, methodology/technique used, sector focussed and nationality of the main author.
2.2 Identification of sustainable remanufacturing supply chain risks and their solutions
The sustainable RSC risks (criteria) were identified through literature analysis and, subsequent inputs provided by the decision-making panel. The list of sustainable RSC risks identified from the published literature was presented to the decision panel (for details refer Section 4.1) for approval. Also, the experts were asked to add any risk if not in the list. This procedure leads to the identification of total 24 sustainable RSC risks (). Further, the solutions (alternatives) to overcome these risks were also finalised based on the literature analysis and discussion with the expert panel. presents the 12 solutions identified to overcome the risks.
Table 2. List of common sustainable remanufacturing supply chain risks
Table 3. Solutions to overcome the sustainable remanufacturing supply chain risks
3. Research methods and proposed hybrid fuzzy SWARA-fuzzy COPRAS framework
A hybrid SWARA-COPRAS is a novel MCDM solution approach for complex problems involving conflicting criteria (Mardani et al. Citation2017). SWARA technique is used to determine the criteria weights of the problem, while the COPRAS technique is used to rank the alternatives. Several studies have been reported in the past and recently too, to evaluate the complex problems in different application areas. A few research contributions by various researchers in different areas are discussed. Rajesh and Malliga (Citation2018) select the strategic green supplier using the hybrid SWARA-COPRAS approach. Zarbakhshnia, Soleimani, and Ghaderi (Citation2018) integrated SWARA-COPRAS to rank and select the sustainable third-party reverse logistics provider in the presence of risk factors. Hashemkhani Zolfani and Bahrami (Citation2014) prioritise the developing high tech industries in the Iranian context by integrating SWARA and COPRAS techniques. Aghdaie et al. (Citation2013) propose a hybrid SWARA-COPRAS approach to rank the best machine tool for machining. Hashemkhani and Saparauskas (Citation2013) integrate SWARA-COPRAS for sustainable assessment of the energy system. Nakhaei et al. (Citation2016) propose a SWARA-COPRAS approach to evaluate different light supply methods in underground safe spaces. This suggests that SWARA-COPRAS has been applied by several researchers for effective decision-making. Before the explanation of integrated fuzzy SWARA-fuzzy COPRAS methodology, the four algebraic operations such as addition, subtraction, multiplication and division on the two triangular fuzzy numbers, and
are presented as follows:
3.1 Fuzzy SWARA
Several MCDM techniques exist in the literature to determine the criteria weights, e.g. Analytic Hierarchy Process (AHP), Analytic Network Process (ANP), Decision-Making Trial and Evaluation Laboratory (DEMATEL), Simple Multi-Attribute Rating Technique (SMART), Weighted Sum Method (WSM), BWM and many more (Yadav et al. Citation2018). AHP is one of the most popular approaches used to determine criteria weights. However, evaluation using the AHP technique can be only be considered reliable if the expert’s opinions are in concordance and that the degree of consistency value is within limits (Keršuliene, Zavadskas, and Turskis Citation2010). Hence, to handle the above issues, SWARA technique was developed by Violeta Keršuliene in 2010. SWARA technique addresses the above issues and presents a novel procedure to determine the criteria weights through estimating the differences of their significances. In addition, SWARA is a method where experts apply their own implicit knowledge, experiences, and information. Also, SWARA is uncomplicated and easy for experts to understand and apply (Hashemkhani and Saparauskas Citation2013). The key feature of SWARA method is its possibility to estimate experts or interest groups opinions about the significant ratio of the attributes in the process of their weights determination (Keršuliene, Zavadskas, and Turskis Citation2010). The criterion that is most significant in reference to the goal or objective is given the first rank, while the least significant criterion is given the last rank (Keršulienė and Turskis Citation2011). The popularity of SWARA technique to determine criteria weights can be demonstrated by the application of this technique by different researchers recently in areas like reverse logistics (Prajapati, Kant, and Shankar Citation2019; Zarbakhshnia, Soleimani, and Ghaderi Citation2018; Mavi, Goh, and Zarbakhshnia Citation2017); construction equipment (Ghorabaee et al. Citation2018); logistics system (Ghorabaee et al. Citation2018); and supplier selection (Jain and Singh Citation2017).
The procedure performed to compute the relative weights of criteria using fuzzy SWARA is described as follows:
Step 1. Criteria are arranged in a sequence starting from most important criteria to the least important criteria, based on the goal of decision-making. presents the fuzzy scales.
Table 4. The fuzzy scale
Step 2. This process starts from the second factor wherein the experts assigns a score to factor j relative to the previous criterion (j – 1), for each individual criterion. Keršuliene, Zavadskas, and Turskis (Citation2010) term this ratio as the comparative importance of value.
Step 3. Determine the coefficient
Step 4. Determine the recalculated fuzzy weights
Step 5. Computation of relative weighting factors of the considered criteria
where = comparative relative weights of j criterion and n = number of criteria
Step 6. Compute non-fuzzy value of , using equation,
3.2 Fuzzy COPRAS
COPRAS, an analytical MCDM technique was developed by Zavadskas, Kaklauskas, and Sarka (Citation1994). COPRAS technique is used to evaluate the various alternatives and among them select the best alternative. The COPRAS technique identifies the best alternative by determining the ratio to the ideal solution and the ratio with the ideal-worst solution (Zavadskas et al. Citation2012). The main advantages of the COPRAS technique are: it is reliable and presents an accurate assessment method; and it takes into account every aspect of each criterion and separates the positive and negative criterion (Amoozad Mahdiraji et al. Citation2018). Zavadskas and Antucheviciene (Citation2007) were the first to propose fuzzy COPRAS to handle the uncertain decision-making problems. Fuzzy COPRAS has been extensively used by researchers in different application area like sustainable transportation (Hatefi Citation2018); reverse logistics (Zarbakhshnia, Soleimani, and Ghaderi Citation2018); total productive maintenance (Bekar, Ebru, and Kahraman Citation2016); machine tool evaluation (Nguyen et al. Citation2015); boiler evaluation (Özçil et al. Citation2015); and infrastructure evaluation (Yazdani, Alidoosti, and Zavadskas Citation2011).
The following steps are performed for ranking the solutions to overcome the sustainable RSC risks.
Step 1. Select the alternatives (solutions) to overcome the identified risks.
Step 2. Prepare the fuzzy decision matrix using triangular fuzzy number as shown in .
Table 5. Alteration for fuzzy membership functions
where m = number of alternatives, n = number of criterions (factors), and = evaluation of ith alternative for factor j. The alteration of fuzzy triangular numbers is presented in .
Step 2a. Prepare the aggregated fuzzy decision matrix using the below equation.
Step 3. Normalisation of the fuzzy decision matrix . The normalised value of the fuzzy decision matrix is calculated using the procedure adopted in Kaklauskas et al. (Citation2016). This method of normalising the initial fuzzy decision matrix makes the computation process efficient and improves the accuracy of numeration (Liu and Liu Citation2010; Zarbakhshnia, Soleimani, and Ghaderi Citation2018). As
and
Step 4. Computing the weighted normalised fuzzy decision matrix. This is obtained by multiplying the weights obtained for each factor using fuzzy SWARA with the normalised fuzzy decision matrix. For multiplication of each criteria weight with the normalised fuzzy decision matrix, the equation used is .
Step 5. Sums of attributes values which larger values are more preferable (optimisation direction is maximisation) calculation for each alternative (line of the decision-making matrix):
Step 6. Sums of attributes values which smaller values are more preferable (optimisation direction is minimisation) calculation for each alternative (line of the decision-making matrix):
Step 7. Computing non-fuzzy values of and
using the following equation (Zarbakhshnia, Soleimani, and Ghaderi Citation2018)
Step 8. Determining the minimal value of
Step 9. Computation of relative weight of each alternative
Formula (17) can also be written as
Step 10. Determining the optimal alternative using the below equation
Step 11. Computing the utility degree of each alternative
where = non-fuzzy relative weight of each alternative and
= value of optimal alternative. The alternatives are ranked according to the value of
, such that the alternative having high value of
, is an ideal alternative.
Hence, the present research proposes a hybrid fuzzy SWARA-fuzzy COPRAS framework for ranking the solutions to overcome the sustainable RSC risks. This hybrid framework follows a three-step methodology.
Phase I: Identification and finalisation of sustainable RSC risk criteria, sub-criteria, and solutions.
Phase II: Fuzzy SWARA application to compute weights of sustainable RSC risks.
Phase III: Fuzzy COPRAS application to rank risk solutions.
demonstrates the flow diagram of the three-phase methodology adopted in this study. A numerical case study analysis of an Indian bearing manufacturing organisation is conducted to demonstrate the applicability of the proposed framework to identify and rank the solutions to mitigate the sustainable RSC risks.
4. Numerical application of the proposed hybrid framework to case organisation
The proposed hybrid framework is applied to a case organisation involved in bearing manufacturing/remanufacturing in India. The ‘PQR’ organisation (name not disclosed to maintain confidentiality) was established in the year 1962. The company is not only a major supplier of bearings to Indian manufacturing sectors (automotive, steel plants, electrical industries, etc.) but also exports to Europe, US and Asia. ISO 9001, TS 16,949 and ISO 14,001 certification suggests organisational sensitivity towards quality and environment. The organisation also conducts several programmes under the corporate social responsibility policy. The case organisation is a brand name in Indian as well as International market. The top executives are already aware that to sustain in a globalised and competitive market, and to maintain their brand status, simply focusing on financial performance is not sufficient enough. They also need to focus on the social and environment-related issues for overall growth. Also, integrating remanufacturing practices in the traditional manufacturing makes the supply chain complicated and exposed to risk issues such as maintaining the profitability while addressing the social and environmental dimensions of sustainability. Hence, the case organisation executives are also interested in evaluating the sustainable RSC risks and what could be the solutions to overcome them. The executives also desire to know the best solution that would best eliminate the majority of the risks involved. The recent hybrid fuzzy SWARA-fuzzy COPRAS approach would help the executives/practitioners to handle the sustainable RSC risks effectively.
4.1 Phase I: identification and finalisation of sustainable remanufacturing supply chain risk criteria, sub-criteria and solution
A decision-making panel consisting of five experts from the case organisation was formed to identify and finalise the evaluating criteria, sub-criteria and solutions (alternatives). The five case organisation experts, namely Head (Projects), Head (Manufacturing), Head (Industrial Engineering), Head (Technologistic) and Senior Manager (Logistics) are knowledgeable and possess industrial experience of more than 10 years. Based on the inputs of the decision-making panel and literature analysis, 24 sub-criteria (sub-risks) were finalised. It is extremely important to validate the considered criteria to ensure that the factors are in line with the organisation mission/vision and strategies. The finalised RSC risk factors were systematically categorised across the three main dimensions of sustainability (economic risks, social risks and environmental risks). In addition, 12 solutions (alternatives) to overcome these risks were also evaluated and finalised using the decision panel inputs. demonstrates the hierarchical structure depicting the goal, main risks, sub-risks categorised under the main risks and the solutions to these risks.
4.2 Phase II: fuzzy SWARA application to compute weight of sustainable RSC risks
This section presents the results computed using fuzzy SWARA. In this methodology, as discussed earlier, at first the finalised list of main risks and sub-risks was presented to the decision-making (experts) panel. Next, the decision-making panel assign relative weight to the main risks and sub-risks using the fuzzy scale () provided to them. Experts were provided with a questionnaire form (Appendix II) for fuzzy SWARA input data collection. The decision panel after several rounds of discussion formed a common consensus and arranged the main risks in sequence from the most important risk to the least important risk. They further assigned ratings to the risks using the linguistic terms as provided in . Next, the relative fuzzy weights are computed for the main criteria (risks) using EquationEquation (5)(5)
(5) –(Equation7
(7)
(7) ) (). The sub-criterion under the main risks are also rated by the decision-making panel in a similar manner. presents the local weight and global weight of sub-criteria under the economic risks. The global weight of sub-criteria is obtained by multiplying the weight of the main factor with the local weight of each sub-criteria. For example, the local weight of ER8 in its category is (0.396, 0.443, 0.496) () and the weight of economic risk is (0.478, 0.526, 0.584) (). The global weight for ER8 is obtained by multiplying these two weights which is (0.189, 0.233, 0.289) (). Lastly, the de-fuzzy value of each sub-criteria is computed using the equation,
. The local weight, global weights and de-fuzzy value of sub-criteria under the social and environmental risks are also computed similarly. and present the local weight, global weight and de-fuzzy weight of sub-criterion under the social and environmental risks category, respectively.
Table 6. Final weight of main risk factors
Table 7. Local weight and final weight of economic risks sub-criteria
Table 8. Local weight and final weight of social risks sub-criteria
Table 9. Local weight and final weight of environmental risks sub-criteria
4.3 Phase III: fuzzy COPRAS application to rank risk solutions
Solutions to the sustainable RSC risks are ranked in this section using fuzzy COPRAS approach. The weights computed for sub-risks using fuzzy SWARA technique is utilised in fuzzy COPRAS approach. To apply fuzzy COPRAS approach EquationEquation (8)(8)
(8) –(Equation20
(20)
(20) ) is used. Fuzzy decision matrix of the alternatives in the form of questionnaire (Appendix III) were presented to five experts for assigning the fuzzy numbers (). Due to space constraint, only one fuzzy decision matrix obtained from one of the expert is presented in . Then, aggregated fuzzy decision matrix () is formulated using EquationEquation (9)
(9)
(9) . The aggregated fuzzy decision matrix is then normalised using EquationEquations (10)
(10)
(10) –(Equation12
(12)
(12) ). The results obtained after normalisation process is presented in . After computing the normalised fuzzy decision matrix, then the weighted fuzzy decision matrix is computed. The criteria weights obtained using fuzzy SWARA technique is used with normalised fuzzy decision matrix to compute the weighted normalised fuzzy decision matrix ().
Table 10. Fuzzy decision matrix between alternative and criteria (Expert 1)
Table 11. Aggregate fuzzy decision matrix between alternatives and criteria
Table 12. Normalised fuzzy decision matrix between alternatives and criteria
Table 13. Weighted normalised fuzzy decision matrix between alternatives and criteria
Further, from the weighted normalised fuzzy decision matrix, rest of the fuzzy COPRAS calculations are performed using EquationEquations (13)(13)
(13) –(Equation20
(20)
(20) ). The final results as obtained are shown in .
Table 14. Final results and rank of the solutions (alternatives)
5. Discussion
Several solutions exist to overcome the risks involved in sustainable RSC implementation. However, it is difficult to define that implementation of which solution eradicates the majority of the risks. The proposed fuzzy SWARA–fuzzy COPRAS technique systematically helps the decision-maker by ranking the solutions to overcome the sustainable RSC risks. Ranking the solutions enables the practitioner first to select the best risk mitigation solution and so on. The solutions were ranked based on value, and the sequence of ranking is S2 > S4 > S6 > S7 > S1 > S5 > S8 > S11 > S3 > S10 > S12 > S9 (). Initiatives from management and modification in organisation goals (S2) is the highest-ranked solution to overcome sustainable RSC risks. In developing countries, the manufacturers have the fear that remanufactured products from their own returned product results in the cannibalisation of their new product (Chaowanapong, Jongwanich, and Ijomah Citation2018). Agrawal, Atasu, and Van Ittersum. (Citation2015) suggest that the cannibalise fear of the manufacturer can be overcome by identifying separate markets for new and remanufactured product. Hence, top management needs to take initiatives to identify specific market for new product and remanufactured product (Govindan, Shankar, and Kannan Citation2016). Also, the organisation should formulate innovative strategies for engaging itself in a circular economy to manage the waste (Zlamparet et al. Citation2017). Zlamparet et al. (Citation2017) suggest that the manufacturer should maintain a database which consists of information regarding the type of product, client, technical specification of the product and its location. The authors reported that the environmental impact could be reduced by 37% by maintaining such type of system. Government, manufacturer and distributor collaboration to develop action plans (S4) is the second most important solution. In India, the manufacturers do not have pressure for remanufacturing because stringent regulatory laws regarding remanufacturing from the government is still lacking (Sharma, Garg, and Sharma Citation2016b). Wang et al. (Citation2014) suggest that manufacturers and third-party remanufacturers of developing countries lack the necessary support from the government in the form of finance, subsidies, and rebates. Also, restrictive and stringent laws regarding the import of remanufactured parts in the Indian context is a significant issue for manufacturer’s to start with supply chain remanufacturing activities (Chakraborty, Mondal, and Mukherjee Citation2019). Also, the acquisition of used products is a significant risk involved in Indian context because of consumer attitude to throw away the used product rather than returning it to the collection centre. Hence, the government should come up with specific initiatives such as providing financial benefits, incentives, and rewards to the manufacturers or third-party remanufacturers and the consumers. This research work is carried out in the Indian context; however, in a similar developing country like China, Cao et al. (Citation2020) reported that government had drafted a policy wherein at least 10% discount on the market price is offered to the consumers who purchase the remanufactured product. The author also reported that the government provides subsidies to the enterprises involved in collecting scrapped vehicles. This policy increased the collection rate (backbone of remanufacturing) to 4%-6% in 2016 from 1% in 2013. Also, Giri and Sharma (Citation2015) reported a 1.24% increase in total profit in the case where the manufacturer and retailer are collectively involved in the collection of used products from the consumer. This research work suggests that the government, manufacturer and the distributor should work in collaboration and discuss the issues faced by each other, which otherwise would create a feasible environment for supply chain remanufacturing implementation.
The solution namely setting up international standards (S6) is ranked third to overcome the sustainable RSC risks. Customer recognition for the remanufactured product is a significant issue in developing countries. Wei et al. (Citation2015) suggest that this is because remanufacturing business is still evolving in developing economies, and consumer knowledge regarding the remanufactured product is very poor. The consumer is not aware of the sustainable benefits that can be realised due to supply chain remanufacturing. The manufacturer should take the initiative to integrate quality management systems to enhance the quality standards of the remanufactured products. Further, it helps to improve the confidence of the customers in the remanufactured product. Also, awareness regarding remanufacturing needs to be increased among the customers to enhance customer recognition by setting up international standards and adoption of best remanufacturing practices. Developing structured channels to collect EOL products (S7) is at the fourth rank to solve sustainable RSC risks. Designing a reverse logistics network for the collection of EOL products is very challenging, especially in the Indian context because of its large geographical area (Govindan, Shankar, and Kannan Citation2016). The consumers of India are usually unknown regarding the collection channel wherein the used product should be returned. How will the manufacturer indulge itself in remanufacturing business, if the used product is not returned to the remanufacturing centre? Hence, the manufacturers should establish suitable collection channels and adopt novel return strategies to collect the EOL products. Soleimani, Chaharlang, and Ghaderi (Citation2018) investigated a case problem by implementing a novel simultaneous collection and delivery approach. They found that a reduction in 10.5% cost was observed due to the adoption of the simultaneous collection and delivery approach in comparison to the separate collection and delivery approach. Also, Zlamparet et al. (Citation2018) implemented a novel online collection system (Internet+) in a leading Chinese third-party collection organisation. The organisation is involved in the collection, testing, and selling of second-hand mobile phones and tablets. The case organisation realised a total cost reduction by 45% due to the implementation of the new collection system.
The fifth-ranked solution is sustainable product design (S1). Improper design of the product during the product development stage poses a major risk for remanufacturing of the used product. Hatcher, Ijomah, and Windmill (Citation2011) reported that the majority of the OEMs do not integrate the ‘design for remanufacturing’ (DfRem) concept while designing their original product. Improper design of the fasteners, complexity in product design, number of parts and non-standardised parts are some product design-related issues that hinder remanufacturing operations (Chakraborty, Mondal, and Mukherjee Citation2019). The presence of these issues creates problem during disassembly, cleaning and reassembly. Due to non-standardisation, the parts/cores mismatch problem emerges as a severe concern for remanufacturing. The research and development team should consider disassembly and EOL strategies, for instance, remanufacturing at the early stage of product design to achieve a sustainable product design. Favi et al. (Citation2017) performed a case study wherein the domestic cooker hood was re-designed to enhance the remanufacturability and recyclability of the components or cores. The re-design replaced fasteners and non-removable connections with efficient joining methods; reduced number of components, materials; and modified the geometry/shape for ease in disassembly for the operators. They found that approximately 11% of components could be remanufactured while approximately 40% of components could be recycled with the help of the new design. Also, Badurdeen, Aydin, and Brown (Citation2018) found that considering EOL strategies such as remanufacturing, reuse and recycling during the product design results in a reduction in total lifecycle cost, total global warming potential and total water use by 20%. In this sense, this study suggests that sustainable product design should be designed to recover even the smallest part of the used product and mitigate the issues related to disassembly, inspection, cleaning, and reassembly and testing.
Promotional strategies (S5) is the sixth-ranked solution. In Indian scenario, the negative attitude of the consumer towards the remanufactured product is a major issue. Due to a lack of awareness, the consumers have the perception that remanufactured product is of inferior quality in comparison to the new product. Sharma, Garg, and Sharma (Citation2016b) suggest that Indian consumers consider remanufactured product as a second-hand/low-grade product which is a significant risk for the remanufacturers. The remanufacturers need to develop appropriate promotional strategies to change the negative perception of the consumer regarding the functionality of the remanufactured product (Mukherjee and Mondal Citation2009). Recently, Singhal, Tripathy, and Jena (Citation2019) in their study reported that effective strategies should be adopted to promote the remanufactured products. It reduces the risk associated with the negative attitude of the consumer towards the remanufactured product and enhances the effectiveness of sustainable RSC. Structured guidelines for product remanufacturing (S10), ease in providing financial assistance (S12) and relaxation in current legislative restrictions (S9) are low ranked solutions. The case organisation is not much affected by this criterion. The legislative restriction on imported products and higher taxes do not affect them because their involvement in such activities is limited. However, it is not true that the low ranked solutions are not relevant, but the ranking enables the practitioner to first focus on the high-rank solutions and develop strategies accordingly for effective implementation of RSC practices to minimise the risk issues.
6. Sensitivity analysis
This study performs sensitivity analysis to check the robustness in the ranking of sustainable RSC risk solutions (alternatives). A similar approach was used by Wang et al. (Citation2019) and Prakash and Barua (Citation2016) to validate the final rankings of the alternatives due to variation in criteria weights. A total of 19 experiments (sensitivity runs) were performed. During these 19 experiments, the effect on the final ranking of the solutions was investigated due to changes in the weights of sustainable RSC risk criteria. The details of the experiments are presented in . The value of each solution is calculated for each experiment. For the first three sensitivity runs, one risk criteria is substituted with a lower weight (0.015), while the weight of other criteria are substituted with higher value (0.6). In sensitivity analysis experiments 4 to 17, in each case one risk criteria is substituted with a higher weight (0.6), while weights of other risk criteria is set as constant (0.015). In the case of experiment 18, weights of risk criteria (ER1-ER4) is set 0, while the remaining risk criteria weights were set at 0.3. For experiment 19, the weight of each risk criteria weight is taken as 0.03.
Table 15. Sensitivity analysis
The final ranks obtained for the 12 solutions due to changes in risk criterion weights are presented in and . The result indicates that out of 19 experiments, S2 scored the highest rank in eight experiments (1–2, 5, 9–11, 18–19). S4 turned out to be the best solution in five experiments (3–4, 6, 8, 15). S7 secured the highest rank in five experiments (7, 12–13, 16–17), while S8 was the best solution in one experiment (14). Also, S9 is the lowest-ranked solution in eleven experiments. The findings are consistent with the results presented in , wherein S2, S4, S6 and S7 are the highly ranked solutions, while S9 is the lowest-ranked solution. This also suggests that ranking of 12 solutions to mitigate the sustainable RSC risks is relatively sensitive to the risk criteria weights.
7. Conclusion
The present study is directed towards the management of risks involved in the field of SC remanufacturing business because of the presence of uncertain risk factors. Risks have adverse effects on the functionality as well as the profitability of the RSC. The impact of associated risks can be mitigated or even nullified by the adoption of appropriate risk mitigation strategies (solutions). However, it would be difficult for the industrial practitioners to implement the risk mitigation solutions without its assessment and knowing its importance. Hence, ranking the solutions will help the practitioners to implement these solutions in a systematic manner to manage the RSC effectively. This research work presents a structural solution model to rank the solutions to mitigate the risks associated with RSC. Firstly, the several risks associated with RSC and the solutions to mitigate these risks were identified through literature analysis and experts response. Next, the risks and the solutions were analysed using a hybrid fuzzy SWARA-fuzzy COPRAS approach. The weight of common risks associated with supply chain remanufacturing was determined using fuzzy SWARA. Then, fuzzy COPRAS is utilised to determine the ranking of solutions.
The efficacy of the proposed approach is demonstrated by its application to a case organisation. The evaluation process was based on 24 risk factors categorised under three main risks (economic risks, social risks, and environmental risks); and 12 solutions. The input data required in the evaluation process is collected from five experts (decision-makers) that were experienced in the research field. Based on the expert’s panel opinion the weight of risks criterion and the ranking of solutions was determined using the fuzzy SWARA-fuzzy COPRAS approach. A sensitivity analysis is also performed in this study to demonstrate that the ranking of solutions is not only sensitive to risks criterion weight but also robust in nature.
This evaluation process helps to identify the most important risk mitigation solution from the identified solutions, and also assist the industrial practitioners to focus on high-rank solution. The findings of the study indicate ‘initiatives from management and modification in organization goals’ (S2) as the most important solution and ‘relaxation in current legislative restriction’ (S9) as the least important solution. Hence, industrial practitioners should focus on high-rank solutions to mitigate the risks and improve the effectiveness of supply chain remanufacturing practices adoption.
Based on the findings of the study, the present research work proposes several implications to the practitioners as discussed below:
This research work enables the decision-makers/policy-makers and remanufacturers to have proper understanding and analysis of the risks that hinder the effective implementation of sustainable RSC practices. This study is an initial attempt that categorises 24 risks of RSC across three main dimensions of sustainability, namely economic, social and environmental. The results of the study will help the managers to deal with these risks and develop risk management approaches.
This study also presents 12 solutions to overcome the identified 24 sustainable RSC risks. However, the penetration of all the 12 solutions in the organisation is very difficult. The present research work plays a significant role to understand the various solutions and ranks them. The ranking of the solutions will help the practitioners to implement the risk solutions in a step-by-step manner.
From the results, it can be implied that the remanufacturers should initiate to develop a sustainable culture in the organisation wherein each supply chain member is given the role and responsibility in managing the associated risks. Furthermore, the manufacturer, government and distributor should work in collaboration to create awareness among the customers to return the products after its EOL and also develop proper collection channels.
The results of the study will not only assist the practitioners of the case organisation to effectively mitigate the sustainable RSC risks for its effective implementation but also enhance the ecological-economic-social benefits.
This research proposes a hybrid framework using fuzzy SWARA-fuzzy COPRAS to evaluate the sustainable RSC risks and rank the solutions to overcome these risks arranged in a hierarchical structure (). Fuzzy SWARA was used to evaluate the sustainable RSC risks and fuzzy COPRAS was used to rank the solutions to minimise these risks. The present research work will motivate the researchers to enhance its application in supply chain remanufacturing practices implementation.
This research work has certain limitations. Sustainable RSC risks and the solutions have been identified through world-wide literature analysis including India and finalised with the expert panel. Hence, the relevance of the risks and the solutions exist world-wide. However, the findings of the study cannot be generalised because the results generated are based on the inputs of decision panel (experts) of a single case organisation in the Indian context. Development of matrix by the experts between the risks and solutions is a complex and tedious process especially when the number of risk factors and solutions is large in number. Also, the ranking of the solutions is based on human judgements and hence there may a possibility of inconsistency during decision-making. The computation process for ranking the solutions is complex and care should be taken during the evaluation process. Several research directions can be derived based on the present research. First, the proposed model could be applied to other industries or a similar type of risk evaluation problem. Second, all other stakeholders (government, customer, supplier, non-government organisations) should be involved to identify comprehensive sustainable RSC risk factors and their solutions for sustainable improvements. The interdependency between the sustainable RSC risks was suggested by the experts during this research study. Hence, analysing the interrelationships between the sub-risks is the third future opportunity that needs to be investigated. Lastly, the statistical validation of the proposed model should be conducted using structural equation modelling (SEM) as part of future research.
Disclosure statement
No potential conflict of interest was reported by the authors.
Additional information
Notes on contributors
Zulfiquar N. Ansari
Zulfiquar N. Ansari is pursuing his Ph.D. in the area of supply chain remanufacturing from the Department of Mechanical Engineering, Sardar Vallabhbhai National Institute of Technology, Surat, India. His areas of interest are supply chain management, remanufacturing supply chain, sustainable supply chain management and operations management.
Ravi Kant
Dr. Ravi Kant is Associate Professor at the Department of Mechanical Engineering, Sardar Vallabhbhai National Institute of Technology, Surat, India. His areas of research interest include Knowledge Management, Operations & Supply Chain, Lean & Six Sigma. He has about 14 years of experience in industry, teaching and research. He has co-authored more than 200 research papers in international journals and conferences and three books.
Ravi Shankar
Dr. Ravi Shankar is Professor at Department of Management Studies, Indian Institute of Technology (IIT) Delhi, India. He is also the Honorary Visiting Professor of Decision Science at School of Economics and Management, Loughborough University, UK. His areas of interest include Decision Sciences, Business Analytics and Big Data, Operations and Supply Chain Management, Sustainable Freight Transportation, Project Management, Total Quality Management and Six Sigma, Strategic Technology Management, etc. He has co-authored more than 400 research papers and six books.
References
- Abdulrahman, M., N. Dan-Asabe, C. L. Subramanian, and C. Shu. 2015. “Viability of Remanufacturing Practice: A Strategic Decision Making Framework for Chinese Auto-parts Companies.” Journal of Cleaner Production 105: 311–323. doi:10.1016/j.jclepro.2014.02.065.
- Aghdaie, H., S. Mohammad, H. Zolfani, and E. K. Zavadskas. 2013. “Decision Making in Machine Tool Selection: An Integrated Approach with SWARA and COPRAS-G Methods.” Engineering Economics 24 (1): 5–17.
- Agrawal, V. V., A. Atasu, and K. Van Ittersum. 2015. “Remanufacturing, Third-party Competition, and Consumers’ Perceived Value of New Products.” Management Science 61 (1): 60–72. doi:10.1287/mnsc.2014.2099.
- Alimoradi, A., R. M. Yussuf, and N. Zulkifli. 2011. “A Hybrid Model for Remanufacturing Facility Location Problem in A Closed-loop Supply Chain.” International Journal of Sustainable Engineering 4 (1): 16–23. doi:10.1080/19397038.2010.533793.
- Ansari, Z. N., R. Kant, and R. Shankar. 2019. “Prioritizing the Performance Outcomes Due to Adoption of Critical Success Factors of Supply Chain Remanufacturing.” Journal of Cleaner Production 212: 779–799. doi:10.1016/j.jclepro.2018.12.038.
- Badurdeen, F., R. Aydin, and A. Brown. 2018. “A Multiple Lifecycle-based Approach to Sustainable Product Configuration Design.” Journal of Cleaner Production 200: 756–769. doi:10.1016/j.jclepro.2018.07.317.
- Bekar, T., M. C. Ebru, and C. Kahraman. 2016. “Fuzzy COPRAS Method for Performance Measurement in Total Productive Maintenance: A Comparative Analysis.” Journal of Business Economics and Management 17 (5): 663–684. doi:10.3846/16111699.2016.1202314.
- Bhatia, M. S., K. Rajiv, and Srivastava. 2018. “Analysis of External Barriers to Remanufacturing Using grey-DEMATEL Approach: An Indian Perspective.” Resources, Conservation and Recycling 136: 79–87. doi:10.1016/j.resconrec.2018.03.021.
- Cao, J., X. Chen, X. Zhang, Y. Gao, X. Zhang, and S. Kumar. 2020. “Overview of Remanufacturing Industry in China: Government Policies, Enterprise, and Public Awareness.” Journal of Cleaner Production 242: 118450. doi:10.1016/j.jclepro.2019.118450.
- Chakraborty, K., S. Mondal, and K. Mukherjee. 2017. “Analysis of Product Design Characteristics for Remanufacturing Using Fuzzy AHP and Axiomatic Design.” Journal of Engineering Design 28 (5): 338–368. doi:10.1080/09544828.2017.1316014.
- Chakraborty, K., S. Mondal, and K. Mukherjee. 2019. “Critical Analysis of Enablers and Barriers in Extension of Useful Life of Automotive Products through Remanufacturing.” Journal of Cleaner Production 227: 1117–1135. doi:10.1016/j.jclepro.2019.04.265.
- Chang, D.-Y. 1996. “Applications of the Extent Analysis Method on Fuzzy AHP.” European Journal of Operational Research 95 (3): 649–655. doi:10.1016/0377-2217(95)00300-2.
- Chaowanapong, J., J. Jongwanich, and W. Ijomah. 2018. “The Determinants of Remanufacturing Practices in Developing Countries: Evidence from Thai Industries.” Journal of Cleaner Production 170: 369–378. doi:10.1016/j.jclepro.2017.09.134.
- Christopher, M., and H. Lee. 2004. “Mitigating Supply Chain Risk through Improved Confidence.” International Journal of Physical Distribution & Logistics Management 34 (5): 388–396. doi:10.1108/09600030410545436.
- Das, D., and R. Chaudhari. 2015. “Reverse Supply Chain Management in Consumer Electronics: An Indian Perspective.” International Journal of Logistics Systems and Management 16 20 (3): 348–369. doi:10.1504/IJLSM.2015.068425.
- Favi, C., M. Germani, A. Luzi, M. Mandolini, and M. Marconi. 2017. “A Design for EoL Approach and Metrics to Favour Closed-loop Scenarios for Products.” International Journal of Sustainable Engineering 10 (3): 136–146. doi:10.1080/19397038.2016.1270369.
- Ghazalli, Z., and A. Murata. 2011. “Development of an AHP–CBR Evaluation System for Remanufacturing: End-of-life Selection Strategy.” International Journal of Sustainable Engineering 4 (1): 2–15. doi:10.1080/19397038.2010.528848.
- Ghorabaee, M. K., M. Amiri, E. K. Zavadskas, and J. Antucheviciene. 2018. “A New Hybrid Fuzzy MCDM Approach for Evaluation of Construction Equipment with Sustainability Considerations.” Archives of Civil and Mechanical Engineering 18 (1): 32–49. doi:10.1016/j.acme.2017.04.011.
- Giri, B. C., and S. Sharma. 2015. “Optimizing a Closed-loop Supply Chain with Manufacturing Defects and Quality Dependent Return Rate.” Journal of Manufacturing Systems 35: 92–111. doi:10.1016/j.jmsy.2014.11.014.
- Govindan, K., and A. Chaudhuri. 2016. “Interrelationships of Risks Faced by Third Party Logistics Service Providers: A DEMATEL Based Approach.” Transportation Research Part E: Logistics and Transportation Review 90: 177–195. doi:10.1016/j.tre.2015.11.010.
- Govindan, K. K., M. Shankar, and D. Kannan. 2016. “Application of Fuzzy Analytic Network Process for Barrier Evaluation in Automotive Parts Remanufacturing Towards Cleaner Production–a Study in an Indian Scenario.” Journal of Cleaner Production 114: 199–213. doi:10.1016/j.jclepro.2015.06.092.
- Hashemkhani, Z. S., and J. Saparauskas. 2013. “New Application of SWARA Method in Prioritizing Sustainability Assessment Indicators of Energy System.” Engineering Economics 24 (5): 408–414.
- Hashemkhani Zolfani, S., and M. Bahrami. 2014. “Investment Prioritizing in High Tech Industries Based on SWARA-COPRAS Approach.” Technological and Economic Development of Economy 20 (3): 534–553. doi:10.3846/20294913.2014.881435.
- Hatcher, G. D., W. L. Ijomah, and J. F. C. Windmill. 2011. “Design for Remanufacture: A Literature Review and Future Research Needs.” Journal of Cleaner Production 19 (17–18): 2004–2014. doi:10.1016/j.jclepro.2011.06.019.
- Hatefi, S. M. 2018. “Strategic Planning of Urban Transportation System Based on Sustainable Development Dimensions Using an Integrated SWOT and Fuzzy COPRAS Approach.” Global Journal of Environmental Science and Management 4 (1): 99–112.
- Ijomah, W. L. 2009. “Addressing Decision Making for Remanufacturing Operations and Design-for-remanufacture.” International Journal of Sustainable Engineering 2 (2): 91–102. doi:10.1080/19397030902953080.
- Ilgin, M. A. 2017. “An Integrated Methodology for the Used Product Selection Problem Faced by Third-party Reverse Logistics Providers.” International Journal of Sustainable Engineering 10 (6): 399–410. doi:10.1080/19397038.2017.1317873.
- Jain, N., and A. R. Singh. 2017. “Fuzzy Kano Integrated MCDM Approach for Supplier Selection Based on Must Be Criteria.” International Journal of Supply Chain Management 6 (2): 49–59.
- Kaklauskas, A., E. K. Zavadskas, S. Raslanas, R. Ginevicius, A. Komka, and P. Malinauskas. 2016. “Selection of Low-e Windows in Retrofit of Public Buildings by Applying Multiple Criteria Method COPRAS: A Lithuanian Case.” Energy and Buildings 38 (5): 454–462. doi:10.1016/j.enbuild.2005.08.005.
- Kannan, D., A. Diabat, and K. Madan Shankar. 2014. “Analyzing the Drivers of End-of-life Tire Management Using Interpretive Structural Modeling (ISM).” The International Journal of Advanced Manufacturing Technology 72 (9–12): 1603–1614. doi:10.1007/s00170-014-5754-2.
- Karvonen, I., K. Jansson, K. Behm, S. Vatanen, and D. Parker. 2017. “Identifying Recommendations to Promote Remanufacturing in Europe.” Journal of Remanufacturing 7 (2–3): 159–179. doi:10.1007/s13243-017-0038-2.
- Keršulienė, V., and Z. Turskis. 2011. “Integrated Fuzzy Multiple Criteria Decision Making Model for Architect Selection.” Technological and Economic Development of Economy 17 (4): 645–666. doi:10.3846/20294913.2011.635718.
- Keršuliene, V., E. K. Zavadskas, and Z. Turskis. 2010. “Selection of Rational Dispute Resolution Method by Applying New Step‐wise Weight Assessment Ratio Analysis (SWARA).” Journal of Business Economics and Management 11 (2): 243–258. doi:10.3846/jbem.2010.12.
- Kuo, T. C., H.-Y. Ma, S. H. Huang, A. H. Hu, and C. S. Huang. 2010. “Barrier Analysis for Product Service System Using Interpretive Structural Model.” The International Journal of Advanced Manufacturing Technology 49 (1–4): 407–417. doi:10.1007/s00170-009-2399-7.
- Li, Z., W. Zheng, Q. Meng, and S. Jin. 2019. “The Impact of Government Subsidy and Tax Policy on the Competitive Decision-making of Remanufacturing Supply Chains.” International Journal of Sustainable Engineering 12 (1): 18–29. doi:10.1080/19397038.2018.1449031.
- Lin, K.-P., M.-L. Tseng, and P.-F. Pai. 2018. “Sustainable Supply Chain Management Using Approximate Fuzzy DEMATEL Method.” Resources, Conservation and Recycling 128: 134–142. doi:10.1016/j.resconrec.2016.11.017.
- Liu, W., and P. Liu. 2010. “Hybrid Multiple Attribute Decision Making Method Based on Relative Approach Degree of Grey Relation Projection.” African Journal of Business Management 4 (17): 3716.
- Luthra, S., and S. K. Mangla. 2018. “When Strategies Matter: Adoption of Sustainable Supply Chain Management Practices in an Emerging Economy’s Context.” Resources, Conservation and Recycling 138: 194–206. doi:10.1016/j.resconrec.2018.07.005.
- Mahdiraji, A., S. A. Hannan, G. Stauskis, and E. Zavadskas. 2018. “A Hybrid Fuzzy BWM-COPRAS Method for Analyzing Key Factors of Sustainable Architecture.” Sustainability 10 (5): 1626. doi:10.3390/su10051626.
- Mangla, S. K., P. Kumar, and M. K. Barua. 2015. “Risk Analysis in Green Supply Chain Using Fuzzy AHP Approach: A Case Study.” Resources, Conservation and Recycling 104: 375–390. doi:10.1016/j.resconrec.2015.01.001.
- Mardani, A., M. Nilashi, N. Zakuan, N. Loganathan, S. Soheilirad, M. Z. M. Saman, and O. Ibrahim. 2017. “A Systematic Review and meta-Analysis of SWARA and WASPAS Methods: Theory and Applications with Recent Fuzzy Developments.” Applied Soft Computing 57: 265–292. doi:10.1016/j.asoc.2017.03.045.
- Matsumoto, M., K. Chinen, and H. Endo. 2018. “Paving the Way for Sustainable Remanufacturing in Southeast Asia: An Analysis of Auto Parts Markets.” Journal of Cleaner Production 205: 1029–1041. doi:10.1016/j.jclepro.2018.09.074.
- Matsumoto, M., S. Yang, K. Martinsen, and Y. Kainuma. 2016. “Trends and Research Challenges in Remanufacturing.” International Journal of Precision Engineering and Manufacturing-Green Technology 3 (1): 129–142. doi:10.1007/s40684-016-0016-4.
- Mavi, R. K., M. Goh, and N. Zarbakhshnia. 2017. “Sustainable Third-party Reverse Logistic Provider Selection with Fuzzy SWARA and Fuzzy MOORA in Plastic Industry.” The International Journal of Advanced Manufacturing Technology 91 (5–8): 2401–2418. doi:10.1007/s00170-016-9880-x.
- Mukherjee, K., and S. Mondal. 2009. “Analysis of Issues Relating to Remanufacturing Technology–a Case of an Indian Company.” Technology Analysis & Strategic Management 21 (5): 639–652. doi:10.1080/09537320902969174.
- Nakhaei, J., S. L. Arefi, M. Bitarafan, and K. Simona. 2016. “Evaluation of Light Supply in the Public Underground Safe Spaces by Using of COPRAS-SWARA Methods.” International Journal of Strategic Property Management 20 (2): 198–206. doi:10.3846/1648715X.2015.1132790.
- Nguyen, H.-T., S. Z. M. Dawal, Y. Nukman, H. Aoyama, and K. Case. 2015. “An Integrated Approach of Fuzzy Linguistic Preference Based AHP and Fuzzy COPRAS for Machine Tool Evaluation.” PloS One 10 (9): e0133599. doi:10.1371/journal.pone.0133599.
- Özçil, A., İ. Ertuğrul, T. Öztaş, and G. Z. Öztaş. 2015. “Combi Boiler System Modeling with Fuzzy Inference Mechanism and Fuzzy Copras Method.” In 2015 IEEE International Conference on Fuzzy Systems (FUZZ-IEEE), Istanbul, pp. 1–8.
- Parkinson, H. J., and G. Thompson. 2004. “Systematic Approach to the Planning and Execution of Product Remanufacture.” Proceedings of the Institution of Mechanical Engineers, Part E: Journal of Process Mechanical Engineering 218 (1): 1–13. doi:10.1243/095440804322860591.
- Prajapati, H., R. Kant, and R. Shankar. 2019. “Prioritizing the Solutions of Reverse Logistics Implementation to Mitigate Its Barriers: A Hybrid Modified SWARA and WASPAS Approach.” Journal of Cleaner Production 240: 118219. doi:10.1016/j.jclepro.2019.118219.
- Prakash, C., and M. K. Barua. 2016. “An Analysis of Integrated Robust Hybrid Model for Third-party Reverse Logistics Partner Selection under Fuzzy Environment.” Resources, Conservation and Recycling 108: 63–81. doi:10.1016/j.resconrec.2015.12.011.
- Rajesh, G., and P. Malliga. 2018. “Selection of Suppliers Using Swara and Copras-G.” International Journal of Enterprise Network Management 9 (2): 169–185. doi:10.1504/IJENM.2018.093712.
- Rathore, P., S. Kota, and A. Chakrabarti. 2011. “Sustainability through Remanufacturing in India: A Case Study on Mobile Handsets.” Journal of Cleaner Production 19 (15): 1709–1722. doi:10.1016/j.jclepro.2011.06.016.
- Rostamzadeh, R., M. K. Ghorabaee, K. Govindan, A. Esmaeili, and H. B. K. Nobar. 2018. “Evaluation of Sustainable Supply Chain Risk Management Using an Integrated Fuzzy TOPSIS-CRITIC Approach.” Journal of Cleaner Production 175: 651–669. doi:10.1016/j.jclepro.2017.12.071.
- Saavedra, Y. M., A. P. Barquet, H. Rozenfeld, F. A. Forcellini, and A. R. Ometto. 2013. “Remanufacturing in Brazil: Case Studies on the Automotive Sector.” Journal of Cleaner Production 53: 267–276. doi:10.1016/j.jclepro.2013.03.038.
- Seitz, M. A. 2007. “A Critical Assessment of Motives for Product Recovery: The Case of Engine Remanufacturing.” Journal of Cleaner Production 15 (11–12): 1147–1157. doi:10.1016/j.jclepro.2006.05.029.
- Sharma, V., S. K. Garg, and P. B. Sharma. 2016b. “Identification of Major Drivers and Roadblocks for Remanufacturing in India.” Journal of Cleaner Production 112: 1882–1892. doi:10.1016/j.jclepro.2014.11.082.
- Sharma, V. S., K. Garg, and P. B. Sharma. 2016a. “Identification and Analysis of Industry Initiatives in Improving Remanufacturing in India.” International Journal of Operational Research 26 (3): 340–366.
- Singhal, D., S. Tripathy, and S. K. Jena. 2019. “Sustainability through Remanufacturing of E-waste: Examination of Critical Factors in the Indian Context.” Sustainable Production and Consumption. doi:10.1016/j.spc.2019.06.001.
- Sinha, A., S. Mondal, T. Boone, and R. Ganeshan. 2017. “Analysis of Issues Controlling the Feasibility of Automobile Remanufacturing Business in India.” International Journal of Services and Operations Management 26 (4): 459–475. doi:10.1504/IJSOM.2017.082893.
- Soleimani, H., Y. Chaharlang, and H. Ghaderi. 2018. “Collection and Distribution of Returned-remanufactured Products in a Vehicle Routing Problem with Pickup and Delivery considering Sustainable and Green Criteria.” Journal of Cleaner Production 172: 960–970. doi:10.1016/j.jclepro.2017.10.124.
- Tang, O., and S. Nurmaya Musa. 2011. “Identifying Risk Issues and Research Advancements in Supply Chain Risk Management.” International Journal of Production Economics 133 (1): 25–34. doi:10.1016/j.ijpe.2010.06.013.
- Terkar, R., H. Vasudevan, and D. V. Kalamkar. 2013. “Enhancing Productivity through Cost and Lead Time Reduction in Remanufacturing.” International Journal of Mechanical Engineering and Technology (IJMET) 4 (2): 286–297.
- Toke, L. K., and S. D. Kalpande. 2019. “Critical Success Factors of Green Manufacturing for Achieving Sustainability in Indian Context.” International Journal of Sustainable Engineering 12 (6): 415–422. doi:10.1080/19397038.2019.1660731.
- Vasanthakumar, C., S. Vinodh, and K. Ramesh. 2016. “Application of Interpretive Structural Modelling for Analysis of Factors Influencing Lean Remanufacturing Practices.” International Journal of Production Research 54 (24): 7439–7452. doi:10.1080/00207543.2016.1192300.
- Wang, B., J. Song, J. Ren, L. Kexin, H. Duan, and X. Wang. 2019. “Selecting Sustainable Energy Conversion Technologies for Agricultural Residues: A Fuzzy AHP-VIKOR Based Prioritization from Life Cycle Perspective.” Resources, Conservation and Recycling 142: 78–87. doi:10.1016/j.resconrec.2018.11.011.
- Wang, Y., X. Chang, Z. Chen, Y. Zhong, and T. Fan. 2014. “Impact of Subsidy Policies on Recycling and Remanufacturing Using System Dynamics Methodology: A Case of Auto Parts in China.” Journal of Cleaner Production 74: 161–171. doi:10.1016/j.jclepro.2014.03.023.
- Wei, S., D. Cheng, E. Sundin, and O. Tang. 2015. “Motives and Barriers of the Remanufacturing Industry in China.” Journal of Cleaner Production 94: 340–351. doi:10.1016/j.jclepro.2015.02.014.
- Widera, H., and G. Seliger. 2015. “Methodology for Exploiting Potentials of Remanufacturing by Reducing Complexity for Original Equipment Manufacturers.” CIRP Annals 64 (1): 463–466. doi:10.1016/j.cirp.2015.04.111.
- Xia, X., K. Govindan, and Q. Zhu. 2015. “Analyzing Internal Barriers for Automotive Parts Remanufacturers in China Using grey-DEMATEL Approach.” Journal of Cleaner Production 87: 811–825. doi:10.1016/j.jclepro.2014.09.044.
- Yadav, G., S. K. Mangla, S. Luthra, and S. Jakhar. 2018. “Hybrid BWM-ELECTRE-based Decision Framework for Effective Offshore Outsourcing Adoption: A Case Study.” International Journal of Production Research 56 (18): 6259–6278. doi:10.1080/00207543.2018.1472406.
- Yazdani, M., A. Alidoosti, and E. K. Zavadskas. 2011. “Risk Analysis of Critical Infrastructures Using Fuzzy COPRAS.” Economic research-Ekonomska Istraživanja 24 (4): 27–40. doi:10.1080/1331677X.2011.11517478.
- Zarbakhshnia, N., H. Soleimani, and H. Ghaderi. 2018. “Sustainable Third-party Reverse Logistics Provider Evaluation and Selection Using Fuzzy SWARA and Developed Fuzzy COPRAS in the Presence of Risk Criteria.” Applied Soft Computing 65: 307–319. doi:10.1016/j.asoc.2018.01.023.
- Zavadskas, E. K., A. Kaklauskas, and V. Sarka. 1994. “The New Method of Multicriteria Complex Proportional Assessment of Projects.” Technological and Economic Development of Economy 1 (3): 131–139.
- Zavadskas, E. K., and J. Antucheviciene. 2007. “Multiple Criteria Evaluation of Rural Building’s Regeneration Alternatives.” Building and Environment 42 (1): 436–451. doi:10.1016/j.buildenv.2005.08.001.
- Zavadskas, E. K., S. Sušinskas, A. Daniūnas, Z. Turskis, and S. Henrikas. 2012. “Multiple Criteria Selection of Pile-column Construction Technology.” Journal of Civil Engineering and Management 18 (6): 834–842. doi:10.3846/13923730.2012.744537.
- Zhang, J.-H., B. Yang, and M. Chen. 2017. “Challenges of the Development for Automotive Parts Remanufacturing in China.” Journal of Cleaner Production 140: 1087–1094. doi:10.1016/j.jclepro.2016.10.061.
- Zhang, T., J. Chu, X. Wang, X. Liu, and P. Cui. 2011. “Development Pattern and Enhancing System of Automotive Components Remanufacturing Industry in China.” Resources, Conservation and Recycling 55 (6): 613–622. doi:10.1016/j.resconrec.2010.09.015.
- Zhu, Q., J. Sarkis, and K.-H. Lai. 2015. “Reprint of “Supply Chain-based Barriers for Truck-engine Remanufacturing in China”.” Transportation Research Part E: Logistics and Transportation Review 74: 94–108. doi:10.1016/j.tre.2014.12.004.
- Zlamparet, G. I., Q. Tan, A. B. Stevels, and J. Li. 2018. “Resource Conservation Approached with an Appropriate Collection and Upgrade-remanufacturing for Used Electronic Products.” Waste Management 73 (2018): 78–86. doi:10.1016/j.wasman.2017.11.053.
- Zlamparet, G. I., W. Ijomah, Y. Miao, A. K. Awasthi, X. Zeng, and L. Jinhui. 2017. “Remanufacturing Strategies: A Solution for WEEE Problem.” Journal of Cleaner Production 149: 126–136. doi:10.1016/j.jclepro.2017.02.004.
Appendix I
Questionnaire form presented to the expert panel to determine the type of criterion (minimise criteria or maximise criteria)
Appendix II
Questionnaire form for expert panel to arrange the criteria in accordance with most important to least important and then assign the importance weight. For sub-criteria similar form is prepared but because of space constraints only one form for sub-criteria is presented.
Appendix III
Questionnaire form for comparing solution with each sub-criteria (risk criterion)