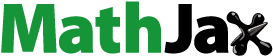
ABSTRACT
This study aims to evaluate the Green Supplier Development Programs (GSDPs) for greening a supply chain. However, this problem is threatened by restricted quantitative information, the specific context of the organisation, lack of prior experience and varying supplier backgrounds. In this paper, we propose a fuzzy integrated Multi-Criteria Decision-Making approach for investigating and prioritising GSDPs. The approach is developed by integrating fuzzy Decision-Making Trial and Evaluation Laboratory (DEMATEL), fuzzy Analytic Hierarchy Process (AHP) and Technique for Order of Preference by Similarity to Ideal Solution (TOPSIS) methods. First, fuzzy DEMATEL is applied to determine the main green factors, then the fuzzy AHP method is used to acquire the local weights of criteria, and finally, the GSDPs are prioritised based on the green factors by fuzzy TOPSIS. The proposed approach is employed to estimate GSDPs of the painting companies. The outcomes indicate that ‘requiring ISO 14,000 certification for suppliers? and ‘building top management commitment for suppliers for green supply practices’ have the highest and lowest impact on improving the environmental performance of suppliers, respectively. It is also concluded that ‘green procurement’ measure has the highest effect on prioritising the GSDPs.
1. Introduction
With the advent of a new era of business where change is regarded as one of its main features, nowadays the success and survival of organisations cannot be easily guaranteed, particularly regarding demanding environmental protection and green manufacturing issues (Pourjavad and Shahin Citation2018b). Green Supply Chain Management (GSCM) as an organisational philosophy aims to increase the market share and financial capability of companies with minimising environmental negative impacts and increasing ecological efficiency (Govindan, Soleimani, and Kannan Citation2015). Hence, most companies are looking for solutions approaches to improve the performance of GSCM. In this regard, suppliers play a significant role in developing GSCM (Hussain and Al-Aomar Citation2018). The performance of GSCM is highly improved by improving the environmental performance of suppliers (Fu, Zhu, and Sarkis Citation2012). Hence, companies improve the performance of GSCM through the selection of existing green suppliers. Regarding this matter, scholars have proposed various approaches for selecting green and sustainable suppliers, evaluating the environmental performance of suppliers, and assessing the green suppliers.
However, it is undeniable fact that all suppliers are not initially green and able to create better their environmental or sustainability performance, effectively. Therefore, companies have focused on aiding suppliers to improve their environmental performance by ‘introducing’ Green Supplier Development Programs (GSDPs). Competitive advantages can be accrued from GSDPs, but significant organisational resources are required to implement these programs. Also, all GSDPs cannot equally provide environmental improvement and supplier operational performance. The investigation and selection of the appropriate and efficient GSDPs are required by companies due to the heterogeneous performance of GSDPs and limited available resources for implementation (Dou, Zhu, and Sarkis Citation2014).
Several criteria and their inter-relationships with uncertain information should be implemented to evaluate GSDPs (Bai and Sarkis Citation2010). Multi-Criteria Decision-Making (MCDM) approaches (Sangaiah et al. Citation2017) have been commonly employed to evaluate the supplier environmental performance (Shaik and Abdul-Kader Citation2011), for instance, Analytic Hierarchy Process (AHP), Analytic Network Process (ANP), and Technique for Order of Preference by Similarity to Ideal Solution (TOPSIS). These methods have been applied to evaluate the relationships between supplier development program involvement attributes and performance outcomes, to analyse the influence relationships amongst GSDPs, and to assess the effects of green programs on supplier performance (Pourjavad and Shahin Citation2018a).
The defined criteria for evaluating green supplier development programs are divided into two categories; quantitative and vague or imprecisely defined qualitative. The vagueness intrinsic to the evaluation of qualitative criteria and vague weighing of these criteria by several decision-makers increases the uncertainty of GSDPs evaluation. Hence, the importance of a good approach which handles both quantitative and qualitative criteria is highlighted to reach the appropriate results of GSDPs evaluation. In addition, the MCDM approaches are accompanied by human judgements and preferences which are vague because of uncertainty and imprecision in the human decision-making process (Goyal, Kaushal, and Sangaiah Citation2018). Hence, these approaches are coped with subjective human preferences for most problems in real-world applications (Sangaiah et al. Citation2018). The decision-makers prefer to use linguistic terms instead of exact scales for evaluation (Pourjavad and Mayorga Citation2019). The fuzzy set theory facilitates decision-making by approximate reasoning and linguistic terms (Pourjavad and Mayorga Citation2018c), hence it is recognised as a solution to solve such uncertainty problems of MCDM models.
As mentioned earlier, the problem of GSDPs evaluation is influenced by multiple criteria with various levels of importance. The process of GSDPs evaluation would be more difficult, time-consuming and inappropriate if green criteria with low importance are being considered. The Decision-Making Trial and Evaluation Laboratory (DEMATEL) is recognised as one of the best methods to analyse the cause-and-effect relationships of criteria and find the most important ones (Sangaiah, Subramaniam, and Zheng Citation2015). Hence, in this paper, we use a fuzzy DEMATEL to define key green criteria by avoiding low influences for evaluating GSDPs.
In this paper, an integrated fuzzy MCDM approach based on the fuzzy DEAMTEL, fuzzy AHP, and fuzzy Technique for Order of Preference by Similarity to Ideal Solution (TOPSIS) is proposed to evaluate GSDPs according to green criteria. The proposed approach is composed of three main steps. In the first step, the fuzzy DEMATEL is implemented to determine critical green factors that have a high effect on decision-making. Then, the fuzzy AHP method is applied to obtain the local weights of each criterion, and finally, the programs of green supplier development are assessed and ranked based on green criteria by fuzzy TOPSIS. The proposed fuzzy-integrated approach is applied to evaluate GSDPs for greening suppliers in painting industries. In addition, a sensitivity analysis is conducted to estimate the importance of green criteria to prioritise green supplier development suppliers.
We make the following research contributions in this study:
Determining critical green criteria to investigate green supplier development programs;
Developing an integrated fuzzy MCDM approach to prioritise GSDPs under restricted quantitative information;
Conducting a sensitivity analysis to evaluating the influence of green criteria in ranking GSDPs.
The rest of this paper is organised as follows: Section 2 presents a review of the literature related to GSDPs, Section 3 reveals the proposed fuzzy MCDM approach for GSDPs evaluation, Section 4 applies the proposed approach for prioritising GSDPs of painting industries, Section 5 discusses the achieved results from the proposed approach and presents a sensitivity analysis on the effect of green criteria for evaluating GSDPs and managerial implications, finally, Section 6 provides some concluding remarks.
2. Literature review
The conducted studies in the field of green supply chain management are divided into six main categories: sustainable supplier selection, green supplier selection, green supplier evaluation, green partner selection, supplier environmental performance evaluation, and green supplier development program evaluation ().
Table 1. A summary of GSCM approaches
For the first category, sustainable supplier selection, scholars developed different approaches such as Full Consistency method (Durmić Citation2019) to evaluate suppliers based on the aspects of sustainability; environmental, social, and economy. In this regard, Zarbakhshnia et al. (Citation2020) also integrated fuzzy AHP and grey multi-objective optimisation by ratio analysis (MOORA-G) to evaluate third-party reverse logistics providers based on sustainability criteria. For the categories of green supplier selection and green partner selection, the deterministic and fuzzy MCDM methods such as ANP and DEA (Kuo and Lin Citation2012), fuzzy AHP-TOPSIS (Chatterjee and Stević Citation2019), AHP and fuzzy TOPSIS (Hamdan and Cheaitou Citation2017) have been also used for selecting suppliers based on green factors. Lo et al. (Citation2018) proposed a novel model to select green suppliers by integrating best–worst method (BWM), TOPSIS, and fuzzy multi-objective linear programming (FMOLP). Chang, Liou, and Lo (Citation2019) developed an integrated BWM and the fuzzy TOPSIS technique framework to evaluate the performance and priority rankings of strategic alliance partners. The review of conducted studies for the category of green supplier evaluation shows the MCDM methods have been applied for assessing the suppliers based on green criteria. The environmental performance of suppliers has been evaluated by the fuzzy MCDM approach such as fuzzy TOPSIS (Awasthi, Chauhan, and Goyal Citation2010), and fuzzy AHP-TOPSIS (Stević et al. Citation2016).
For all conducted studies in the first five categories, it is assumed that all suppliers are green or follow the sustainability criteria in their performance. However, as mentioned above, all suppliers are not green or improve sustainability performance. Hence, in the last category, green supplier development programmes evaluation, scholars focus on finding ways to green and improve the environmental performance of suppliers in order to improve the green supply chain management. Despite the importance of development programs to green suppliers, there are a few studies in this area. For example, Bai and Sarkis (Citation2010) presented a formal method based on rough set theory to explore the relationships between GSDPs attributes and performance outcomes. A formalised grey-based DEMATEL methodology was suggested to investigate the GSDPs by Fu, Zhu, and Sarkis (Citation2012) within a telecommunication systems provider. Blome, Hollos, and Paulraj (Citation2014) extracted the opposing theoretical views of legitimacy in exploring company performance and top management commitment as results in green supplier development. In addition, they evaluated the effects of two criteria on supplier performance, green procurement and green supplier development. Dou, Zhu, and Sarkis (Citation2014) introduced a grey Analytical Network Process-based (grey ANP-based) model to determine GSDPs that improved the performance of suppliers, effectively. They also investigated green supplier development programs, comprehensively with explicit consideration of supplier involvement propensity levels. The review of conducted studies in field of Green Supplier Development Programs evaluation show there is not a procedure to find the important green criteria for evaluating GSDPs. Hence, the above approaches are time-consuming and inappropriate when green criteria with low significance are being considered. In addition, the uncertainty issue in the process of GSDPs evaluation has been neglected by other scholars. The uncertainty is addressed by fuzzy logic in the proposed approach of this study.
3. The proposed approach
In this section, we develop an integrated fuzzy MCDM approach to investigate GSDPs. The main steps of the proposed approach are highlighted in . This approach comprises three main steps, (i) recognising the evaluation dimensions and related criteria of GSDPs using the fuzzy DEMATEL method, (ii) obtaining the local weights of green criterion by fuzzy AHP, (iii) prioritising the green supplier development programs using fuzzy TOPSIS. The main steps of approach are detailed as below:
3.1. Green criteria selection by fuzzy DEMATEL
The GSDPs evaluation is complicated by the fact that various criteria should be considered in the assessment process. These factors are generally categorised into supplier operational performance and supplier environmental performance (). The supplier operational performance metrics include cost (C1), quality (C2), time (C3), flexibility (C4), innovativeness (C5) and process management (C6) (Sarkis and Talluri Citation2002). Ho, Xu, and Dey (Citation2010) identified quality as the most popular factor, followed by delivery, cost, manufacturing capability, and service. Cost, quality, on-time delivery, and flexibility were recognised as the most common supplier performance criteria by Chuu (Citation2011). Innovativeness and process management have also been considered as important operational performance criteria for supplier performance assessment.
Table 2. GSDP Criteria
The environmental criteria are another significant player in supplier performance evaluation (Tang and Zhou Citation2012). Humphreys, Wong, and Chan (Citation2003) used environmental factors such as green design, environmental management systems, and environmental competencies to evaluate suppliers. Handfield et al. (Citation2002) introduced 10 environmental criteria such as emissions, noise, waste, green packaging, green manufacturing, green procurement and reverse logistics to evaluate supplier performance. Jabbour and Jabbour (Citation2009) identified qualitative and quantitative environmental criteria to investigate and select suppliers for Brazilian companies. They applied green warehousing, reverse logistics, waste, water recovery and energy usage criteria in their assessment.
As mentioned above, all these green criteria do not have the same level of importance for evaluating GSDPs. Hence, we use the fuzzy DEMATEL method to determine the important green criteria. A set of 10 academic experts who are working in fields of sustainability, green supply chain management, and environmental issues from Business schools and Industrial Engineering departments participated to complete pair-wise of green criteria. In this study, we used the developed fuzzy DEMATEL by Dalalah, Hayajneh, and Batieha (Citation2011) to determine the green criteria with high importance. The main steps of this approach are detailed as below:
Step one: the matrix with
dimension is built up with the linguistic assessment terms () based on the experts’ opinions. The dimension
denotes the number of green criteria. Each element of this matrix is represented with Triangular Fuzzy Numbers (TFNs) as
= (
,
,
). The
represents the degree to which the criterion
affects criterion
.
Table 3. Total relation matrix of green criteria
Table 4. Linguistic terms of green criteria for GSDPs evaluation
Step two: the normalised direct-relation fuzzy matrix () is created as:
where:
= (
,
,
) and
Step three: based on the following term the total-relation fuzzy matrix is provided.
Therefore, matrix could be displayed as:
where = (
,
,
) is identified as the overall influence rating of decision-maker for each criterion
against criterion
.
Step four: the sum of rows () and sum of columns (
) of the sub-matrices
,
,
are obtained by the following equations:
Step five: the casual diagram is provided by mapping the ordered pairs of (+
) and (
-
). Then, the following equation is used to calculate the importance of green criteria.
Step six: the final weights of green criteria are provided by normalising values as follows:
The prominence, relation and respective weights of green criteria are shown in .
Based on the provided results from fuzzy DEMATEL (), we can find the most significant green criteria for GSDPs evaluation. This method prioritises green criteria based on their importance. The () represents the importance of each green criterion in comparison with others. As seen, ‘Flexibility’ (C4) from supplier operational performance category and ‘Energy Usage’ (C17) from supplier environmental performance got the highest scores, (0.0691) and (0.0702), respectively. These results also indicate, ‘Process Management’ (C7) and ‘Noise’ (C18) has the lowest significance among green criteria for these two categories. In this study, the first eight green criteria which got the highest score based on importance (
) were selected as the main factors for GSDPs evaluation.
3.2. Obtaining the weights of green criteria using fuzzy AHP
The AHP method is a quantitative technique that organises a multi-attribute, multi-person and multi-period problem hierarchically so that solutions are facilitated (Saaty Citation1980). A fuzzy extension of AHP was developed to cover shortages of the original AHP technique and to solve the hierarchical fuzzy problems. The scholars developed several fuzzy AHP methods (Pourjavad and Peng Citation2017). We used the developed fuzzy AHP method by Ayag and Ozdemir (Citation2007) to calculate the weights of green criteria. Triangular fuzzy numbers (,
,
,
,
) are applied to show the relative strength of each pair of elements in the same hierarchy ().
3.3. Ranking GSDPs using fuzzy TOPSIS
In the third step, the fuzzy TOPSIS method is implemented to prioritise the green supplier development programs based on green criteria. This method includes the following steps:
Step 1: the linguistic rating values are defined for the GSDPs with respect to the selected green criteria ().
Step 2: the aggregated fuzzy ratings (=
) of the GSDPs are calculated as:
,
,
, (9)
when the fuzzy ratings of experts are presented by TFNs as = (
,
,
),
.
If = (
,
,
),
;
, represents the fuzzy rating of the
decision-maker, the aggregated fuzzy rating
is represented as:
,
Step 3: the fuzzy decision matrix is established.
Step 4: the normalised fuzzy decision matrix () is constructed as:
=
,
;
where:
Step 5: the weighted normalised matrix () is built up as:
where =
. The (
) represents the weights of green criteria which is calculated by fuzzy AHP, the
denotes the normalised fuzzy decision matrix.
Step 6: the Fuzzy Positive Ideal Solution (FPIS) and Fuzzy Negative Ideal Solution (FNIS) are calculated as:
= (
,
, …,
) where
= (
,
,
) and
= max {
} (15)
= (
,
, …,
) where
= (
,
,
) and
= min {
} (16)
=
and
Step 7: the distance green supplier development program from FPIS () and FNIS (
) is calculated as:
=
)
(17)
=
)
(18)
Step 8: the closeness coefficient (CCi) of each green supplier development program is provided as:
CCi =
The green supplier development programs are prioritised based on the calculated CCi .
4. Case Study
In this section, the proposed approach is applied to evaluate GSDPs of painting industries in IRAN. These programs are labelled ‘setting long-term contracts with environmental dimensions incorporated’ (P1); ‘building top management commitment for suppliers for green supply practices’ (P2); ‘requiring ISO 14,000 certification for suppliers’ (P3); ‘training suppliers on environmental issues’ (P4); and ‘providing green technological advice to suppliers’ (P5).
Ten academic experts along with 20 senior supply managers of the painting industries participated to evaluate the GSDPs according to green criteria. First, the experts make pair-wise comparisons of two main categories and eight green criteria (extracted from fuzzy DEMATEL) using linguistic variables (). The pair-wise comparisons of the green criteria are displayed in . Then, the weights of green criteria are provided by fuzzy AHP ().
Table 5. Fuzzy comparison matrix of the green criteria using TFNs
Table 6. Eigenvector for comparison matrix of the green criteria (CR = 0.075)
Then, a fuzzy evaluation matrix is formed according to experts’ opinions to compare green supplier development programs based on green criteria (). The upper and lower half of show the linguistic variables and triangular fuzzy numbers, respectively. In this section, the linguistic evaluation matrix and fuzzy evaluation matrix of one of the experts are only shown due to space limitation. In the next step, the aggregate fuzzy weights of the GSDPs are provided by EquationEq.(10)(10)
(10) and their values are normalised by EquationEq. (12)
(12)
(12) and EquationEq. (13)
(13)
(13) . Then, the weighted evaluation matrix is provided by the EquationEq. (14)
(14)
(14) .
Table 7. Linguistic scale evaluation matrix for GSDPs
The type of used green criteria in this paper is negative (cost) and positive (benefit) criteria. For this reason, the FPSI () and FNIS (
) are determined as
= (1, 1, 1) and
= (0, 0, 0) for benefit criteria, and
= (0, 0, 0) and
= (1, 1, 1) for cost criteria. The Cr1, Cr7, and Cr8 are categorised into cost criteria and the rest criteria are considered as benefit criteria. Then, the distance of each green supplier development programmes from FPIS (
) and FNIS (
) are provided by Eqs. (26) and (27). Finally, CCi are calculated for all green supplier development programs ().
Table 8. The GSDPs ranking by fuzzy TOPSIS
demonstrates the environmental performance of GSDPs in terms of eight green criteria for greening the suppliers. As seen, P3 and P4 with 0.382 and 0.378 values were ranked as the first and second, respectively. It means they have a relatively significant positive effect on improving the performance of suppliers based on green criteria. In contrast, P1 and P2 have a relatively weak positive effect on supplier performance, with values of 0.339 and 0.338, respectively. P5 got the third rank with 0.371 value.
5. Discussion
In this section, firstly the provided solutions by our approach are discussed and compared with other studies, then the impact of green criteria for prioritising the GSDPs is analysed (Section 5.1), finally, the managerial implications of this study are presented (Section 5.2).
The obtained results of investigating and ranking GSDPs based on green criteria are displayed in . These results provide analytical information for selecting those GSDPs that improve supplier environmental performance. The results show P3 and P2 are identified as the most and less effective GSDPs for suppliers of painting industries, respectively. It means, requiring ISO 14,000 certification for suppliers (P3) has the best environmental performance for the suppliers of the painting companies. In contrast, building top management commitment to using green supply practices for suppliers (P2), has the lowest importance to improve the environmental performance of suppliers. The acquired results also reveal that other GSDPs (P4, P5, and P1) are relatively useful for greening suppliers.
The obtained results can be variously interpreted by supply chain managers of the painting industries to enhance the performance of suppliers based on green criteria. The low efficient GSDPs are eliminated to manage limited resources, while still obtaining good performance results by initially implementing the GSDPs that considerably improve the green performance of suppliers. Supply chain managers initially seek to execute GSDPs more likely to be successful programs, while they understand some green programs are more likely to give larger overall benefits. Hence, it is highly recommended that companies focus on encouraging suppliers to be involved in GSDPs that got the highest scores based on green factors.
The provided results from the developed fuzzy integrated MCDM approach in this study can be compared with conducted studies in this area. The problem of GSDPs evaluation was especially reviewed by Fu, Zhu, and Sarkis (Citation2012), Dou, Zhu, and Sarkis (Citation2014), and Awashti and Kannan (Citation2016). The obtained results from our approach for painting industries show requiring ISO 14,000 certification for the supplier (P3) and training suppliers on environmental issues (P4) were recognised as the best programs for enhancing the environmental performance of suppliers. The importance of these two GSDPs for greening the suppliers has been confirmed by other studies (Fu, Zhu, and Sarkis (Citation2012); Dou, Zhu, and Sarkis (Citation2014); Awashti and Kannan (Citation2016)). In addition, the obtained results from conducted studies by Fu, Zhu, and Sarkis (Citation2012) and Dou, Zhu, and Sarkis (Citation2014) indicate that ‘setting long-term contracts with environmental dimensions incorporated’ (P1) and ‘building top management commitment for suppliers for green supply practices’ (P2) have the lowest impact for improving the environmental performance of suppliers. As can be seen from , these two GSDPs (P1 and P2) got also the lowest importance for greening suppliers in our study.
5.1. Sensitivity analysis
In this section, we conduct a sensitivity analysis to evaluate the impact of green criteria on prioritising the GSDPs. The eleven (11) experiments are designed and conducted (). For this purpose, the different weights are devoted to green criteria. For the first eight experiments, we devote the highest value (0.6) to each green criterion and give the same values (0.075) to the rest of the criteria. For example, for the experiment #1, the value of 0.6 is set for the first criterion (cost) and 0.075 for weights of other criteria (). It should be noted that the sum weights of all green criteria are equal to 1. For the experiment #9, the same weight (0.125) is considered for all green criteria. The used green criteria are divided into two categories, cost and benefit criteria. For the experiment #10, we devote 0 to weights of cost criteria (
) and 0.2 to benefit criteria (
). For the experiment #11, the values of 0 and 0.33 are considered for weights of benefit criteria (
–
) and cost criteria (
), respectively.
Table 9. Experiments for sensitivity analysis
As can be seen from and , ‘requiring ISO 14,000 certification for suppliers’ (P3) has the highest score in 8 out of the 11 experiments. In the remaining three experiments, P4 got the highest score for the two experiments and P2 for one experiment. Based on the results achieved, the ‘green procurement’ is the most significant criterion for evaluating GSDPs. The obtained results also reveal that the proposed fuzzy integrated MCDM approach for evaluating GSDPs is insensitive to the weights of criteria weights with P3 emerging as the winner in the majority of the experiments (8 out of 11).
5.2 Managerial implications
Several managerial implications for supply chain managers can be extracted from the obtained results of the proposed fuzzy MCDM approach. Employing the proposed approach help managers to effectively evaluate the performance of GSDPs or select the best program to execute. The achieved results of approach help managers to focus on GSDPs that provide greater supplier performance and higher supplier involvement. It also facilitates resource and investment allocations for managers. They could identify the poorly performing GSDPs and focus on searching for improvement opportunities for further development. The stability of managers’ decisions is tested by the sensitivity analysis. As mentioned earlier, the uncertainty is an unavoidable reality that exists in the green qualitative criteria for GSDPs evaluation. The proposed approach in this study addresses the uncertainty of GSDPs assessment by fuzzy logic.
6. Conclusions
In this study, an integrated fuzzy MCDM approach was developed to evaluate and prioritise the green supplier development programs using fuzzy DEMATEL and fuzzy AHP-TOPSIS. At first, we applied a fuzzy DEMATEL method to analyse the cause-and-effect relationships of green criteria and select the most important ones. Then, the fuzzy AHP-TOPSIS model was used to evaluate green supplier development programs based on green criteria. The proposed approach was utilised to evaluate GSDPs of painting industries. Based on the results achieved, ‘requiring ISO 14,000 certification for the supplier’ (P3) and ‘training suppliers on environmental issues’ (P4) were recognised as the best GSDPs for greening the suppliers. In addition, a sensitivity analysis was conducted to investigate the effect of green criteria on GSDPs selection. The achieved results of the analysis showed the ‘green procurement’ has the highest effect on prioritising the GSDPs.
The significant contributions and advantages of this study are summarised as (i) this approach considers the imprecise or vague judgements that lead to ambiguity in the evaluation process; (ii) the proposed approach determines the most significant green criteria to prioritise the GSDPs; (iii) the proposed approach contributes to the improvement of environmental performance of companies by selecting the best GSDPs for greening the suppliers. Several future research avenues can be recognised for other scholars. In this study, we developed an integrated fuzzy MCDM approach based on DEMATEL and AHP-TOPSIS for this problem, however there are other MCDM methods that can be used. The original TOPSIS method was used for ranking GSDPs in this study, however it would be substituted with new modified version of TOPSIS (Kuo Citation2017) for future studies. Also, applying the proposed approach in this study on freely available data for different companies is suggested for future study.
Disclosure statement
No potential conflict of interest was reported by the authors.
Additional information
Notes on contributors
Ehsan Pourjavad
Ehsan Pourjavad is a Postdoctoral researcher at Department of Mathematical and Industrial Engineering at Polytechnique Montreal, Canada. He received a Ph.D. in Industrial Engineering from the University of Regina, Canada in 2018. His research interests include operation research, operation management, supply chain management. Ehsan has published more than 24 papers in international journals and conferences since 2011.
Arash Shahin
Arash Shahin is a Full-time Professor at the Department of Management and the Director of Quality Management Research Group at the University of Isfahan, Iran. He received his Ph.D. in Industrial Engineering–Quality Engineering from the Newcastle University, UK. His research interests include quality engineering and management, supply chain management, maintenance management and innovation management. Professor Shahin has been the author of 11 books, five book chapters and 450 published papers in national and international journals and conferences since 1994.
References
- Akman, G., and H. Piskın. 2013. “Evaluating Green Performance of Suppliers via Analytic Network Process and TOPSIS.” Journal of Industrial Engineering 2013: 1–13.
- Amin, S. H., and G. Zhang. 2012. “An Integrated Model for Closed-loop Supply Chain Configuration and Supplier Selection: Multi-objective Approach.” Expert Systems with Applications 39 (8): 6782–6791.
- Awashti, A., and G. Kannan. 2016. “Green Supplier Development Program Selection Using NGT and VIKOR under Fuzzy Environment.” Computers and Industrial Engineering 91: 100–108.
- Awasthi, A., S. S. Chauhan, and S. K. Goyal. 2010. “A Fuzzy Multicriteria Approach for Evaluating Environmental Performance of Suppliers.” International Journal of Production Economics 126 (2): 370–378.
- Ayag, A., and R. G. Ozdemir. 2007. “An Analytic Network Process-based Approach to Concept Evaluation in a New Product Development Environment.” Journal of Engineering Design 18 (3): 209–226.
- Bai, C., and J. Sarkis. 2010. “Green Supplier Development: Analytical Evaluation Using Rough Set Theory.” Journal of Cleaner Production 18 (12): 1200–1210.
- Bai, C., S. Kusi-Sarpong, H. Badri Ahmadi, and J. Sarkis. 2019. “Social Sustainable Supplier Evaluation and Selection: A Group Decision-support Approach.” International Journal of Production Research 57 (22): 1–22.
- Bali, O., E. Kose, and S. Gumus. 2013. “Green Supplier Selection Based on IFS and GRA.” Grey Systems: Theory and Application 3 (2): 158–176.
- Banaeian, N., H. Mobli, B. Fahimnia, I. E. Nielsen, and M. Omid. 2018. “Green Supplier Selection Using Fuzzy Group Decision Making Methods: A Case Study from the Agri-food Industry.” Computers & Operations Research 89: 337–347.
- Blome, C., D. Hollos, and A. Paulraj. 2014. “Green Procurement and Green Supplier Development: Antecedents and Effects on Supplier Performance.” International Journal of Production Research 52 (1): 32–49.
- Büyüközkan, G. 2012. “An integrated fuzzy multi-criteria group decision-making approach for green supplier evaluation.” International Journal of Production Research 50(11): 2892–2909 doi:10.1080/00207543.2011.564668
- Büyüközkan, G, and Çifçi, G. 2012. “A novel hybrid MCDM approach based on fuzzy DEMATEL, fuzzy ANP and fuzzy TOPSIS to evaluate green suppliers.” Expert Systems with Applications 39(3) (2012): 3000–3011 doi:10.1016/j.eswa.2011.08.162
- Cakir, S. 2017. “Selecting the Best Supplier at a Steel-producing Company under Fuzzy Environment.” The International Journal of Advanced Manufacturing Technology 8 (5): 1345–1361.
- Chang, M. H., J. J. Liou, and H. W. Lo. 2019. “A Hybrid MCDM Model for Evaluating Strategic Alliance Partners in the Green Biopharmaceutical Industry.” Sustainability 11 (15): 4065.
- Chatterjee, P., and Ž. Stević. 2019. “A Two-phase Fuzzy AHP-fuzzy TOPSIS Model for Supplier Evaluation in Manufacturing Environment.” Operational Research in Engineering Sciences: Theory and Applications 2 (1): 72–90.
- Chuu, S. J. 2011. “Interactive Group Decision-making Using a Fuzzy Linguistic Approach for Evaluating the Flexibility in a Supply Chain.” European Journal of Operational Research 213 (1): 279–289.
- Dalalah, D., M. Hayajneh, and F. Batieha. 2011. “A Fuzzy Multi-criteria Decision Making Model for Supplier Selection.” Expert Systems with Applications 38 (7): 8384–8391.
- Demir, L., M. E. Akpınar, C. Araz, and M. A. Ilgın. 2018. “A Green Supplier Evaluation System Based on A New Multi-criteria Sorting Method: VIKORSORT.” Expert Systems with Applications 114: 479–487.
- Dos Santos, B. M., L. P. Godoy, and L. M. Campos. 2019. “Performance Evaluation of Green Suppliers Using entropy-TOPSIS-F.” Journal of Cleaner Production 207: 498–509.
- Dou, Y., Q. Zhu, and J. Sarkis. 2014. “Evaluating Green Supplier Development Programs with a Grey-analytical Network Process-based Methodology.” European Journal of Operational Research 233 (2): 420–431.
- Durmić, E. 2019. “Evaluation of Criteria for Sustainable Supplier Selection Using FUCOM Method.” Operational Research in Engineering Sciences: Theory and Applications 2 (1): 91–107.
- Fu, X., Q. Zhu, and J. Sarkis. 2012. “Evaluating Green Supplier Development Programs at a Telecommunications Systems Provider.” International Journal of Production Economics 140 (1): 357–367.
- Genovese, A., S. C. L. Koh, G. Bruno, and E. Esposito. 2013. “Greener Supplier Selection: State of the Art and Some Empirical Evidence.” International Journal of Production Research 51 (10): 2868–2886.
- Govindan, K., H. Soleimani, and D. Kannan. 2015. “Reverse Logistics and Closed-loop Supply Chain: A Comprehensive Review to Explore the Future.” European Journal of Operational Research 240 (3): 603–626.
- Govindan, K., R. Khodaverdi, and A. Jafarian. 2013. “A Fuzzy Multi Criteria Approach for Measuring Sustainability Performance of A Supplier Based on Triple Bottom Line Approach.” Journal of Cleaner Production 47: 345–354.
- Goyal, R. K., S. Kaushal, and A. K. Sangaiah. 2018. “The Utility Based Non-linear Fuzzy AHP Optimization Model for Network Selection in Heterogeneous Wireless Networks.” Applied Soft Computing 67: 800–811.
- Hamdan, S., and A. Cheaitou. 2017. “Supplier Selection and Order Allocation with Green Criteria: An MCDM and Multi-objective Optimization Approach.” Computers and Operation Research 81: 282–304.
- Handfield, R., S. V. Walton, R. Sroufe, and S. A. Melnyk. 2002. “Applying Environmental Criteria to Supplier Assessment: A Study in the Application of the Analytical Hierarchy Process.” European Journal of Operational Research 141 (1): 70–87.
- Ho, W., X. W. Xu, and P. K. Dey. 2010. “Multi-criteria Decision Making Approaches for Supplier Evaluation and Selection: A Literature Review.” European Journal of Operational Research 202 (1): 16–24.
- Humphreys, P. K., Y. K. Wong, and F. T. S. Chan. 2003. “Integrating Environmental Criteria into the Supplier Selection Process.” Journal of Materials Processing Technology 138 (1–3): 49–356.
- Hussain, M., and R. Al-Aomar. 2018. “A Model for Assessing the Impact of Sustainable Supplier Selection on the Performance of Service Supply Chains.” International Journal of Sustainable Engineering 11 (6): 366–381.
- Jabbour, A. B. L. S., and C. J. C. Jabbour. 2009. “Are Supplier Selection Criteria Going Green? Case Studies of Companies in Brazil.” Industrial Management and Data Systems 109 (4): 477–495.
- Kuo, R. J., Y. C. Wang, and F. C. Tien. 2010. “Integration of Artificial Neural Network and MADA Methods for Green Supplier Selection.” Journal of Cleaner Production 18 (12): 1161–1170.
- Kuo, R. J., and Y. J. Lin. 2012. “Supplier Selection Using Analytic Network Process and Data Envelopment Analysis.” International Journal of Production Research 50 (11): 2852–2863.
- Kuo, T. 2017. “A Modified TOPSIS with A Different Ranking Index.” European Journal of Operational Research 260 (1): 152–160.
- Lee, T. R., T. P. Nha Le, A. Genovese, and L. S. C. Koh. 2012. “Using FAHP to Determine the Criteria for Partner’s Selection within a Green Supply Chain: The Case of Hand Tool Industry in Taiwan.” Journal of Manufacturing Technology Management 23 (1): 25–55.
- Lo, H. W., J. J. Liou, H. S. Wang, and Y. S. Tsai. 2018. “An Integrated Model for Solving Problems in Green Supplier Selection and Order Allocation.” Journal of Cleaner Production 190: 339–352.
- Luthra, S., K. Govindan, D. Kannan, S. K. Mangla, and C. P. Garg. 2017. “An Integrated Framework for Sustainable Supplier Selection and Evaluation in Supply Chains.” Journal of Cleaner Production 140: 1686–1698.
- Memari, A., A. Dargi, M. R. A. Jokar, R. Ahmad, and A. R. A. Rahim. 2019. “Sustainable Supplier Selection: A Multi-criteria Intuitionistic Fuzzy TOPSIS Method.” Journal of Manufacturing Systems 50: 9–24.
- Pourjavad, E., and A. Shahin. 2018a. “Hybrid Performance Evaluation of Sustainable Service and Manufacturing Supply Chain Management: An Integrated Approach of Fuzzy Dematel and Fuzzy Inference System.” Intelligent Systems in Accounting, Finance and Management 25 (3): 134–147.
- Pourjavad, E., and A. Shahin. 2018b. “The Application of Mamdani Fuzzy Inference System in Evaluating Green Supply Chain Management Performance.” International Journal of Fuzzy Systems 20 (3): 901–912.
- Pourjavad, E., and R. V. Mayorga. 2018c. A fuzzy rule-based approach to prioritize third-party reverse logistics based on sustainable development pillars.“ Journal of Intelligent & Fuzzy Systems 35(3): 3125-3138.
- Pourjavad, E., and R. V. Mayorga. 2019. “A Comparative Study and Measuring Performance of Manufacturing Systems with Mamdani Fuzzy Inference System.” Journal of Intelligent Manufacturing 30 (3): 1085–1097.
- Pourjavad, E., and W. Peng. 2017. “An Integrated Fuzzy MCDM Approach for Risk Evaluation of New Product in a Pipe Industry.” International Journal of Product Development 22 (3): 165–188.
- Saaty, T. L. 1980. The Analytic Hierarchy Process: Planning Priority Setting. New York: McGraw Hill.
- Sangaiah, A. K., J. Gopal, A. Basu, and P. R. Subramaniam. 2017. “An Integrated Fuzzy DEMATEL, TOPSIS, and ELECTRE Approach for Evaluating Knowledge Transfer Effectiveness with Reference to GSD Project Outcome.” Neural Computing and Applications 28 (1): 111–123.
- Sangaiah, A. K., O. W. Samuel, X. Li, M. Abdel-Basset, and H. Wang. 2018. “Towards an Efficient Risk Assessment in Software projects–Fuzzy Reinforcement Paradigm.” Computers & Electrical Engineering 71: 833–846.
- Sangaiah, A. K., P. R. Subramaniam, and X. Zheng. 2015. “A Combined Fuzzy DEMATEL and Fuzzy TOPSIS Approach for Evaluating GSD Project Outcome Factors.” Neural Computing and Applications 26 (5): 1025–1040.
- Sarkis, J., and S. Talluri. 2002. “A Model for Strategic Supplier Selection.” Journal of Supply Chain Management 38 (1): 18–28.
- Shaik, M., and W. Abdul-Kader. 2011. “Green Supplier Selection Generic Framework: A Multi-attribute Utility Theory Approach.” International Journal of Sustainable Engineering 4 (1): 37–56.
- Shen, L., L. Olfat, K. Govindan, R. Khodaverdi, and A. Diabat. 2013. “A Fuzzy Multi Criteria Approach for Evaluating Green Supplier’s Performance in Green Supply Chain with Linguistic Preferences.” Resources, Conservation and Recycling 74: 170–179.
- Stević, Ž., I. Tanackov, M. Vasiljević, B. Novarlić, and G. Stojić. 2016. “An Integrated Fuzzy AHP and TOPSIS Model for Supplier Evaluation.” Serbian Journal of Management 11 (1): 15–27.
- Tang, C. S., and S. Zhou. 2012. “Research Advances in Environmentally and Socially Sustainable Operations.” European Journal of Operational Research 223 (3): 585–594.
- Yang, C. L., S. P. Lin, Y. H. Chan, and C. Sheu. 2010. “Mediated Effect of Environmental Management on Manufacturing Competitiveness: An Empirical Study.” International Journal of Production Economics 123 (1): 210–220.
- Yeh, W. C., and M. C. Chuang. 2011. “Using Multi Objective Genetic Algorithm for Partner Selection in Green Supply Chain Problems.” Expert Systems with Applications 38 (4): 4244–4253.
- Zarbakhshnia, N., Y. Wu, K. Govindan, and H. Soleimani. 2020. “A Novel Hybrid Multiple Attribute Decision-making Approach for Outsourcing Sustainable Reverse Logistics.” Journal of Cleaner Production 242: 118461.