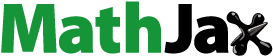
ABSTRACT
The lifetime and complexity of some IT scenarios significantly exceed the typical lifetime and complexity of a technological system. This is a source of significant economic efficiency improvement. In this paper, we propose an approach that enables us to leverage costs, reduce the space floor requirements, and lower CO2 emissions incurred by the infrastructure. By re-investing a significantly smaller financial amount compared to the initial investment an IT infrastructure with the same or better performance and with significantly higher energy efficiency can be purchased. By using a mathematical model, we can quantify the benefits of the approach based on technological advancement, electrical energy prices, and prices of data centre construction. We compare two strategies: the frozen strategy relying on a fixed IT infrastructure during the entire IT system lifecycle and the migration strategy leveraging technological advancement.
Introduction
In recent years, information technologies (IT) play a bigger role in everyday life and are also interconnected with many products and services. The lifetime as well as the complexity of some IT systems has significantly increased compared to standard operational scenarios. Examples of such systems include railway operations centres, nuclear power station control, national airspace control, spacecraft operations centre systems, missile approach warning systems, and others. The lifetime of 15–20 years or more of some of these scenarios significantly exceeds the typical lifetime of a technological system. In addition, the complexity of such systems is typically very high and it is not unusual to find operational sets of 100 and more computer systems. This leads to a lifetime and complexity gap between the standard and some of the new operational scenarios, see . This deserves special attention as it is a source of potential significant cost savings, see below.
The need for an exploration of this problem originates from the spacecraft operations domain that falls into the category of complex systems with a long life too. The findings and conclusions are, however, applicable also in other domains. At the beginning of the research, a short survey was undertaken among 45 persons from six organisations from the top 15 European satellite operators in order to discover the most important aspects and concerns of complex IT systems. The persons consisted of IT infrastructure decision-makers and spacecraft control IT operations engineers. High availability, good performance, low costs, long-term maintainability, and short repair times were identified as the most important factors to be considered. Based on this survey, we conclude that the economic and performance aspects are fundamental and should be optimised.
However, not enough attention is currently paid to the complex systems with long lifetimes although there exist clear requirements for economical efficiency improvement. Within the space domain, the increased complexity, increased costs, and the stress on reducing the costs of IT operations, with regard to initial deployment and repeated expenditures have been sought. This is, for example, also demonstrated by the finding of the Consultative Committee for Space Data Systems (CCSDS.Org Citation2016). There are also a few examples of the top-level requirement for cost reduction. The most well-known example of a top manager asking for lowered costs may be the famous call to carry out space projects ‘faster, better, cheaper’ by the 9th NASA administrator Daniel Goldin (Spear Citation1998). In 2010, Dan Ward (Ward Citation2010) stated that success-per-dollar was a more meaningful measurement of achievement than success-per-attempt. A clear need to focus on cost reduction techniques to the ground system and operations was formulated also by (Miau and Holdaway Citation2000).
Currently, a significant amount of literature describes ways to reduce costs by optimising space crafts themselves, including mission duration, flying orbits, instruments, and other parameters (Sandau Citation2006; Wertz and Larson Citation1996, Citation1999; Williams Citation2016). The techniques and methods, however, apply to scientific missions and try to change their actual parameters. For example, on a scientific planetary mission, it may be possible to reduce the amount of orbit time required by using a higher resolution camera with a wider-angle lens in order to capture the entire planet. Unfortunately, rather than changing the space-based or scientific parameters or instruments, the main cost drivers arise from ground operations during the lifetime of a long-term space mission. The space based services and instruments are typically predefined and do not normally change over time. As the goal of long-term missions is to provide services for a long duration, missions usually cannot be shortened in order to optimise the related costs without losing their scientific or commercial value.
As most missions are strongly automated, personnel costs cannot be easily lowered any further. Thus, mainly the ground segment IT infrastructure needs to be reviewed to find possible cost savings. Within the context of standard IT operations, IT originated costs are typically captured either as a total number or in a Total Cost of Ownership (TCO) or similar model. Unfortunately, such models are usually looking only at typical IT life cycles of three to five years, expecting the IT equipment to be replaced after such a period (Koomey Citation2007). Further on, the models are typically not complete and lack certain elements, like power consumption and energy efficiency (Mell Citation2003; Schäppi et al. Citation2009). As identified by (Wright, Kemp, and Williams Citation2011), the other currently most important factors to be considered are of an environmental nature. We can expect energy to be the main contributor to this measure. We therefore also focus on CO2 emissions as the largest indicator of environmental concern (United States Environmental Protection Agency Citation2018). As the research revealed, the data centre floor space usage also significantly contributes to IT costs. We, therefore, also aim at investigating how technological advancement can lead to lowering the data centre floor space usage.
At the same time, the quality, reliability, and availability of the systems must not be negatively impacted by following the proposed approach.
In this paper, we propose an approach that enables to exploit the lifetime and complexity gap existing in complex long-term operational IT infrastructures, leverage costs, reduce the space floor requirements, and lower CO2 emissions incurred by the infrastructure. It is expected that by re-investing a significantly smaller financial amount in comparison to the original IT budget after a typical duration of an IT industry equipment lifecycle (e.g., 5 years), an IT infrastructure with the same or better performance and with significantly higher energy efficiency can be purchased, which enables achieving the desired benefits. As a part of our demonstration, we compare two strategies: the frozen strategy assuming a fixed IT infrastructure during the entire IT system lifecycle and the migration strategy leveraging the technological advancement by introducing migration steps that enable the space IT infrastructure to exploit the current IT advancement. We also present a mathematical model that quantifies the benefits of the migration approach based on technological advancement, electrical energy prices, and prices of data centre construction.
Main cost drivers in IT systems
One of the methods for classification of IT-related costs can be derived from the ITIL model (Axelos Citation2018). The approach tries to achieve effective financial management of IT services resulting in cost-effective services that carry the potential for a positive Return on Investment (Persse Citation2012). The different areas of costs defined by the ITIL model include capital/operating, direct/indirect, and fixed/variable costs. While ITIL offers a way of categorising costs and generally offers a model for operating a service, it does not directly suggest any solutions and possibilities of reducing the costs. In fact, certain sources even see ITIL causing the opposite, increasing the costs (Pauli Citation2008).
There clearly exist also some generic recommendations to reduce the costs of IT systems. They include, for example, renegotiating major contracts, consolidating infrastructure and operations, reducing power and cooling needs, automating wherever possible, controlling data storage growth, or moving an IT infrastructure to the cloud (Clark Citation2013; Pultz Citation2011). While some of these recommendations are already being implemented, some of them are not acceptable for critical, long-term operations, and some of them are not realistic. A promising possibility is to achieve cost reduction via harnessing technological advancement (Gotter Citation2017).
In order to identify the potential for lowering the costs in complex IT operations, the actual real-world IT costs and their distribution has to be known. Usually, in larger installations in mission-critical systems, computers are not operated like in a normal office. In fact, highly sophisticated data centres are being used. Leading industry research organisations regularly analyse how data centre costs are distributed. A few typical IT costs distributions to be expected across data centre operations are summarised in . Of course, every operational scenario is different and has therefore different factors of influence. Here, we need to identify an average set of the cost related factors in order to reveal the factors that deserve most of the attention.
Table 1. Different sources of IT data centre costs. Some of the figures taken from the cited resources were adjusted, so they describe the reality in a better way. They, for example, accommodate price increases through inflation occurring since their publication time
From the figures from , we can derive that the greatest drivers are depreciation of hardware (43%), labour (25%), and electricity (20%). However, the numbers presented here refer to a 3-year write-off scenario of computing equipment used. In long-term, complex IT operations, the accumulated cost distribution is actually expected to be different.
In order to estimate the impact of individual cost categories, the numbers derived from need to be extrapolated towards a longer, for example, 15-year period. Unfortunately, due to the unavailability of primary data related to costs in long-term operational scenarios of 15 or more years, other ways for identifying the main cost drivers need to be explored. It is clear, that such an extrapolation can never be accurate in terms of down to a single percent. However, to identify the main research directions, the achieved accuracy is sufficient. The extrapolation of costs is made under the following preconditions:
• Labour. After the initial installation of the system, labour mainly takes place on a software level and to carry out preventive administration tasks. The software usually remains unchanged and systems are operated according to the initially designed specifications. Thus, little maintenance is required on systems during the routine operations phase. In a long-term operational scenario, labour clearly has a smaller impact on the costs in comparison to a scenario where there are a lot of equipment replacements undertaken constantly. In order to be very conservative, we assume that 50% of the labour is accumulated during the initial installation and 50% of the labour is required for ongoing operations and maintenance. Labour and its generated cost accumulate over time. Estimating 50% of labour required during long-term operations is not very satisfying, but according to the authors’ practical experience in such undertakings, the number should be approximately right or even on the high side of estimation. Unfortunately, only approximate operational data could be accessed, no exact primary data was available on such a scenario. For this reason, labour will be scaled upwards to 180 months (15 years) in terms of time by using 50% of the estimated 25% of labour as a cost-driver as found previously in .
• Electricity. Electricity usage remains constant during the entire life cycle. The cost of energy, therefore, accumulates linearly during the 15-year period. According to the European Commission (Capros et al. Citation2016), energy prices should only increase on average by 2% until 2035 in the entire period. Therefore, no additional weighting of energy prices needs to be undertaken.
• Servers, Networking, Storage, and Facilities. Here, the depreciation is set at the 15 years operational scenario and not at the typical 36 to 60 months as with standard information technologies. Under unchanged circumstances, there is no hardware replaced in long-term IT operations. The costs are thus scaled over 180 months instead of over 36 to 60 months respectively.
• Heating, Ventilation, and Air Condition Equipment (HVAC). This equipment and depreciation are treated in the same way as servers and scaled over 180 months.
• Other overheads. Other costs accumulate over time. A linear multiplier is therefore used as the factor of the relevant number of months added.
Based on the previously described considerations, new figures representing the cost distribution in long-term operations have been calculated, see . The largest portion of costs is generated by electricity consumption which can be a very interesting area of savings. Due to this reasoning, electricity usage (power consumption) will be in the main focus of our research. The second largest cost driver that is capable of being optimised include facilities. They are tightly related to floor space which is an important parameter of IT infrastructures (Turner and Seader Citation2006).
Figure 2. Distribution of costs in data centre operations extrapolated to 15 years in a scenario with no hardware changes to IT infrastructure using average data from (Gartner Citation2009; Hamilton Citation2011; Kontorinis et al. Citation2012; Koomey Citation2006; Williams Citation2016)
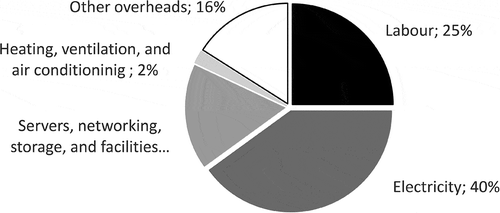
Electrical power consumption
There exist several approaches to an evaluation of electrical power consumption parameters in IT operations centres. They evaluate the efficiency of power usage with the help of various metrics. One of these widely used metrics is Power Usage Effectiveness, PUE (Belady Citation2018). The PUE value describes the relationship between the total energy used in a data centre and the power finally used for the IT equipment: PUE = Total facility power/IT equipment power.
Completely not optimised data centres with inefficient cooling achieve the values of PUE greater than 3. The values around 1.4 are considered to be better than average and were achieved, e.g., by Amazon Web Services in 2011 (Hamilton Citation2011). The values between 1 and 1.2 represent best practices, like in the case of the Portugal Telecom Datacenter in Covilhã (Villarrubia Citation2013) or the main Google datacenters (Gartner Citation2009). The values below 1 represent future trends achievable only via on-site energy generation. Unfortunately, while the usage of PUE is actually providing at least an initial perspective of the energy situation of a data centre, there are several problems with the details of gathering, measurement, interpretation, and inclusion of data. In certain cases, it is possible that only the results from a theoretical analysis are used to describe the data centre efficiency. Real operations can reveal significant differences from the mathematical model (Fortrust Citation2015). Further on, in the definition of PUE, there is no specific timeframe outlined during which the actual determination of PUE has to take place. For example, under certain circumstances, it is possible to actually use outside air-cooling only in cold times. Then, the measurement results may not be fully representative (Clark Citation2013). Finally, PUE is a measure of the overhead of data centres. The numbers thus do not provide information on how much energy is actually used.
Due to the availability of data, it is possible to demonstrate the overall volume of the IT equipment as well as the IT infrastructure composition on the scale of a country, here Germany. According to a study of the Federal Environmental Protection Agency of Germany in 2010/2011, updated with the numbers of a study of the Federal Association for Information Technology (Hintemann and Clausen Citation2014), the overall German national data centre infrastructure can be outlined in . As can be seen, the total yearly sum of the electrical power consumption corresponds to about 10 TWh. This value had been rising until 2008 and has remained almost the same since then (Hintemann Citation2014).
Table 2. The German national data centre infrastructure (Hintemann and Fichter Citation2010)
Considering the cost of electricity of approx. 26 EUR/kWh for installations up to a server room and industry prices of approx. 8.5 EUR/kWh for small, medium, and large data centres, the total monetary cost per year for electricity can be estimated to be around EUR.
Compared to the national electricity usage of Germany, which was about 45 GW in 2012 (Gründwald et al. Citation2012), the overall power consumed by data centres can be estimated to cause approximately 2.5% of the German national baseload capacity. In addition, the operation of IT in Germany generates a total of tons of CO2 per year (calculating with 0.55 kg CO2/kWh, according to the German Federal Office for Environmental Protection) so electricity consumption has also a large environmental impact.
There are a few typical power losses in computer systems. Server power supply losses are typically around 5%. For mechanical hard disks, they are around 2.5–3%, for RAM about 1–1.5% per piece or 3.5–10% per server (based on 4–8 modules), mainboards (including onboard video card, chipset, network cards, CPU voltage converters, and fans) lose about 21–34%, and CPUs at 50% usage about 50% for a two CPU server. The numbers come from own measurements or from manufacturer data (Angelini Citation2014; HP Citation2016; Intel Citation2009; Lenovo Citation2016; Schmidt and Roos Citation2009). It is evident, that CPUs are the main factor to improve power efficiency.
The cost of data centre floor space
According to the existing research, there are several models for determining data centre construction costs. The central finding of (Turner and Seader Citation2006) is that data centre construction costs can be estimated by using a tier-based cost-per-power coefficient along with a size-based cost factor. The Uptime Institute (Uptime Institute Citation2018) distinguishes four tiers of data centres. The simplest one, Tier I, uses a single, non-redundant IT equipment with non-redundant power and network connectivity with no overcapacity in any components, allowing a downtime of up to 28.8 hours per year (99.671% availability). In the highest tier, Tier IV, all components are fully fault-tolerant including uplinks, storage, chillers, cooling systems, and servers, dual power is provided to every component, and the allowable downtime is up to 0.44 hours per year (99.995% availability). The costs of server power used in data centres range from 10,000 USD/kW for Tier I systems to 22,000 USD/kW for Tier IV systems. Additional costs of 2,400 USD/m of floor space must be added (Turner and Seader Citation2006).
Technological advancement and its impact on cost
The overall IT power usage has not been increasing constantly (e.g., in Germany it stays more or less constant since 2008) although computers become more widespread and the complexity of IT systems is constantly increasing. This is enabled by the technological advancement that can be demonstrated by a few famous laws.
Dr. Gordon Moore, a co-founder of the Intel Corporation, researched into the chip complexity increases. Already in 1965, he described his observation of the chip complexity doubling approximately every two years (Moore Citation1965). As an indicator, he used the number of transistors in the integrated circuit. Even though the outline given by Moore was based initially on observation in the period between 1959 and 1965, the projection has stayed accurate until today.
The main finding of Koomey et al. (Koomey et al. Citation2009, Citation2011) is that the number of computations per unit of energy and, thereby, the chip efficiency has been doubling approximately every 19 months since 1950. It is expected that Koomey’s law will effective until 2048 (Landauer Citation1982).
Additional information is needed to infer the floor space from the models of chip efficiency. Information about the number of CPUs per machine (nowadays, typically two) enables a rough estimation of the development in processing power. Statistical data from the years 2000 to 2015 clearly demonstrate that typical maximum power dissipation figures per CPU (also called Thermal Design Power, TDP) are almost stable. The TDP was in the range of 130 W ± 25% in the years 2007 to 2015 (Rupp Citation2014). As the maximum TDP stays almost unchanged, which means that the maximum power per CPU is roughly constant, the conclusion related to the exponential growth of FLOPS/kWh in time can be directly translated into an exponential development performance per machine.
In order to analyse the memory price development, data from (Byte Magazine Citation1975–1998; McCallum Citation2002, Citation2015; Newegg Inc Citation2012; PC Magazine Citation2016; Schembri and Boisseau Citation2015; Stengel Citation2018) was researched and the development of costs per MB of RAM storage in US Dollars was derived. , using a logarithmic scale on the y-axis, clearly demonstrates that the price decrease is exponential. The price per MB of memory drops by factor 100 per decade since approximately 1955.
Figure 3. Memory (RAM) price in USD/MB vs. time (Gotter Citation2017)
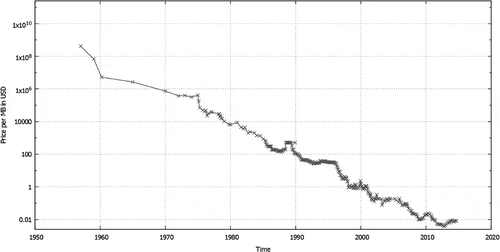
A mathematical model of technology advancement
The previously mentioned laws and regularities can be transformed into a mathematical model. The technological development of chips (the number of transistors on a chip) according to Moore’s law is described by Cavin (Cavin, Lugli, and Shirnov Citation2012) as follows:
where is the number of transistors at time
,
is the number of transistors at the beginning, and
is a time in years.
The chip efficiency development expressed in FLOPS per kWh is represented by the equation derived from the work of Koomey et al. (Koomey et al. Citation2009):
where is a number of FLOPS per kWh at time
,
is the initial FLOPS per kWh at time
,
is a growth factor, and
is a time in years. The average growth factor has been empirically found to be
(Gotter and Pfau Citation2014).
The development of the price of MB of memory can be characterised by the following equation which is derived from the data (Gotter Citation2017) represented by :
where is the memory price per MB at time
,
is the memory price per MB at time
,
is a degradation factor, and
is time in years. The average value of the degradation factor was found to be
.
The Equationequations 1(1)
(1) –Equation3
(3)
(3) can be used to build a model that enables us to quantify the increase in computer capabilities after a given number of years. This means that fewer computers are needed to achieve the same performance. The difference between the current and actually needed number of computers can be then translated to savings in terms of electric power costs, floor space, and CO2 emissions. The model is used in a real-world example in Section 4.
To verify the proposed mathematical model, real measurements of power consumption of 60 to 72 servers in six racks together with two air condition units for a duration of about 2 years were undertaken. The servers had their purchase dates between 2009 and 2013. The oldest ones were taken as the reference point and were compared to the newer ones. Voltage, current, and electric power were measured to calculate the load per system. It has been found that the actual power consumption of the servers of the same kind was quite similar. It was also confirmed that there is definitely a clear connection between the theoretical performance increase and practically measured results. For more details about the measurement, see (Gotter Citation2017).
Enabling possibilities of exploiting technological advancement
During long-term missions, upgrades are usually undertaken only when absolutely necessary. While this approach can be understood from the operational safety point of view, it also introduces some considerable disadvantages. Vendors of the equipment used in missions usually provide their support for a time shorter than the lifetime of the mission. Additional support contracts tend to be very expensive. It is also not possible to use the opportunity that technological advancement brings with regards to both IT efficiency and floor space demand.
The use of energy needed to satisfy the computing requirements is exponentially decreasing over time. This results in much fewer physical computers being necessary to achieve the same computing performance. Thus, much less physical space is needed which thereby results in much lower facility costs. In such a case, already existing data centres can be re-used and still provide enough resources for additional missions, and thereby the construction of entirely new facilities can be avoided.
Within an operational context, three- and five-year periods for considering technology-related changes are of interest. The periods of three and five years can be supported by the largest server manufacturers by the means of service being offered in standard contracts. Any support required outside of the scope of a period of five years would require specific negotiations and would lead to large additional costs. The authors, therefore, propose using the maximal default supportable period of five years. This results, according to the previously elaborated calculation model, in a 9:1 ratio in terms of energy consumption per FLOPS as well as a price drop per unit of memory storage to 1/10. The existing machines and memories could not be reused due to technical incompatibilities having arisen due to technological development in such a time frame. Thus, the replacement costs impact will be also outlined.
The software of complex missions (like the software of the ground segment in a space mission) typically remains fixed. Software patches fixing erroneous behaviour or patches to resolve significant security issues belong to a few exceptions. As we demonstrated in previous chapters, the largest financial benefits can be achieved by using newer, more efficient hardware. In order to reach this goal, it is essential to find an abstraction between software and the physical hardware and maintain the compatibility between the new hardware and original software. We suggest introducing a virtualisation technology together with middleware supporting application deployment that enables the abstraction. The suggested architecture of hardware virtualisation following standard models of virtualisation can be found in .
Figure 4. Hardware virtualisation architecture enabling exploiting the technological advancement (Gotter and Pfau Citation2014)
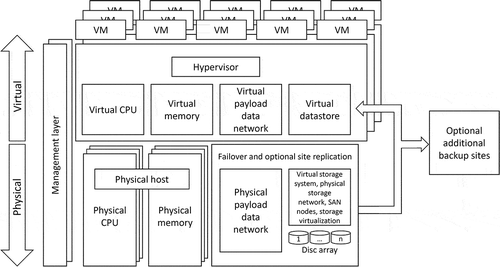
A collection of physical hosts consisting of hardware resources like CPUs and memory forms the bottom level. Physical payload data networking allows communication between the physical hosts. Disk arrays and the physical storage network provide the hardware capabilities for a virtual storage system.
A software module known as the hypervisor is run on each physical host. Its purpose is to map physical hardware into the virtual one, manage the hardware resources, control the execution of virtual machines. Each virtual machine is hosting an operating system and the software for a mission control system. Physical payload networks are mapped into virtual networks and the virtual storage system serves as a data store for the virtual machines. The virtual storage system can be optionally mirrored by a backup system and data can be synchronised to the storage system of the backup system automatically. A management layer supports the configuration, maintenance, and monitoring of the whole system (Gotter and Pfau Citation2014). A management layer has an important effect on the usability of the system since a hypervisor does not enable creating a fully-featured infrastructure for the system.
There exist several types of the hypervisor in the software market: bare-metal hypervisors and hypervisors deployed on top of existing operating systems, adding a guest operating system in parallel to the main operating system that runs the hypervisor (Graniszewski and Arciszewski Citation2016). The latter systems are relevant especially for the virtualisation of desktop and development environments. For mission-critical systems, we recommend using bare-metal hypervisors because they are resistant to side effects caused by an intermediary operating system (Graniszewski and Arciszewski Citation2016).
The products to be potentially considered include VMware ESXi, Oracle VM Server/XEN, and Microsoft Hyper-V standalone server since the market offer is rather limited. After trying the available products over several weeks we found that the most suitable alternative for the specific deployment was VMware ESXi. The product has the widest and most generic operation system support among the considered options. The provider of this system is also the only one that is independent of an in-house operating system product.
A real world example
Three sample space missions were chosen and the power and space requirement parameters were measured. The average floor space and power requirements for a larger mission required in a spacecraft operations data centre were estimated to 580 m and 431 kW. As per our previous finding in , the average electrical power needed for a German data centre is to be expected in the range of 765 W/m
. In our experiments, as an average of the data centres investigated into, a load of 743 W/m
was measured. Using the previous findings of (Turner and Seader Citation2006), and its two-fold definition using floor space and electrical power usage as input parameters in order to determine construction costs, this leads to the costs of
USD associated with floor space and
USD related to the use of electrical power. The estimated total construction cost of a data centre of such a size is then almost
USD.
Electricity savings
We are investigating a scenario of 20 years, which is a typical mission duration. To calculate the potential power and floor space savings, we work with the scenario where the migration steps occur every 5 years, which is a typical length of standard industry support contracts. contains the electrical power consumption and floor space savings achieved when applying the migration approach. After 20 years, the total energy saving potential is about 54 GWh, and the total released floor space about 97,000 m*months.
Table 3. Steps of migration and overall resource usage
To determine the cost savings on electricity, we can use the electricity costs forecast undertaken by the EU to the year 2050 Capros et al. (Citation2016). As the base price, 12.2 EUR/kWh was used as suggested by the Fraunhofer Institute for Systems and Innovation Research for energy-intensive industries (Hintemann and Fichter Citation2010). The calculations of energy savings for the frozen and migration approaches can be found in .
Table 4. Calculating the electrical power consumption, price development, and total cost in a 20-years scenario
CO2 emissions decrease
When we look at the environmental aspect, there are significant potential carbon dioxide savings. When considering CO2 emissions of 0.55 kg/kWh, which was outlined by the German Federal Office for Environmental Protection, the total CO2 emissions of 11,674 tons can be achieved in the migration approach compared to 41,531 tons of the frozen approach. This means savings of about 72% (this is equal to the CO2 emissions of about 17,000 cars creating 176 g CO2 per km, driving 10,000 km each).
Floor space savings
When considering the floor space savings, it is clear that the initial cost of a data centre construction cannot be gained back directly because once the data centre is constructed, the money has been spent. The savings will only be achieved when the freed space could be used in an alternative way and the construction of additional data centre space is avoided. In general, the requirement for more data centre space exists in most spacecraft operations control centres in Europe, sometimes constructing entirely new data centre buildings or building extensions, in order to be able to instal all required equipment.
According to the German tax regulations, data centre buildings are usually written off within a time period of 25 years (4% of the building costs are depreciated per year). The total usage timeframe of 300 months is then reached. By using the model outlined by (Turner and Seader Citation2006), we are reaching the costs of 8 USD per m per month of usage. In addition, electrical power usage must be considered. In our scenario, it is about 743 W/m
on average. As a result and outcome of this model in this specific case, we need to add costs of 16,348 USD/m
over a duration of 25 years to the simple floor space costs, or calculated per month a cost per m
of 54.49 USD. With this specific power density, the result is a total cost of 62.49 USD/m
/month which can be re-gained in case we free up floor space and re-use it in accordance with the same electrical power usage scenario. Considering the floor space reduction from , there is over
USD savings potential, which is about 70% of the floor space-related costs.
Overall benefits
In our example, the total electricity demand of a data centre is 431 kW. However, not all of this energy is available to the infrastructure. Typical investigated space operations data centres, unless they are newly built, reach approximately 2.0 as the value of PUE (Digital Realty Research Trust Citation2012). About 215.5 kW of electrical power is thus available to equipment. About 78% of the electrical load, which is about 168 kW, is generated by servers; the rest of the consumption can be attributed to the network equipment and disk arrays.
238 W of power usage at an average 50% CPU usage per a representative server as the average load was measured in our lab setup. According to these calculations, we would expect to have around 705 two-CPU servers to be in operation simultaneously. In reality, there were 804 servers deployed since not all of them contained two CPUs. This finding is, therefore, can bring even greater cost savings since we propose upgrading to the state where the maximum CPU density of each server is reached Gotter and Pfau (Citation2014).
The initial hardware acquisition costs were 3,581,796 EUR in our scenario. Using our model, we calculated that the current equipment can be replaced by about 11% of current state-of-the-art computers to provide the same performance. The expected cost of hardware replacement in the first migration step after 5 years is 369,564 EUR (the prices of state-of-the-art servers remained very similar). Additional costs related to upgrading, integrating, and migrating are estimated to be up to 50% of the hardware purchase costs. The estimated costs of replacement will be approximately 552,000 EUR (about 15.4% of the original purchase costs).
Similarly, migration steps occur every five years and additional savings are achieved. A graphical representation of the total cost in a mission lasting 20 years in three different scenarios is in . The graph is based on the calculations that quantify the impact of technology advancement on the life cycle costs as explained earlier in this paper.
We can see that the generated electrical energy cost savings between the fifth and the tenth year (after the migration after five years) are about EUR. The required reinvestment costs are only a quarter of these savings, leaving about
EUR available. Without additional migration steps, the total savings on electricity alone will be approximately
EUR during the entire lifetime.
It is evident (see ) that the first migration step brings the largest cost savings. The second migration step provides an additional potential for savings of about EUR. The decision about the second migrations step, which still brings interesting savings, will be based on the financial background and also on another detailed reliability and risk assessment.
Discussion
There exists a clear disproportion between the initial mission technological requirements and the capabilities of technology caused by technological development on the IT market in long-term space missions or other complex and mission-critical projects with strong IT support. Although the paper focuses on spacecraft operations scenarios in its example, one can transfer the findings to other areas of applicability. The preconditions for transferring the demonstrated approach without any modifications include that the reviewed IT undertaking is complex (e.g., 100+ computers) and has the requirement of operating for a long time of 10 and more years. If another scenario that is not too complex is reviewed the same principles may be applied. The limitations of reducing the number of computers may be, however, hit earlier as no further reduction of computers could be possible due to having the need to keep redundancy concepts established.
In complex and mission-critical operations, the performance and platform requirements are usually relatively static. However, computing performance makes progress exponentially during the anticipated operational lifetime. During such a timeframe, there is not only an exponential decrease in price per computation but also memory prices measured in costs per MB and computer prices in terms of cost per performance are dropping exponentially. In this paper, the mathematical model enabling the prediction of the technological development was presented, based on long-term historical data for the number of transistors per processor, calculations per power, and prices per unit of memory. The theoretical findings in terms of energy required for calculations were also confirmed experimentally in (Gotter Citation2017).
The most important finding was the necessity to exploit the efficiency gains of newer computer processor generations. Unfortunately, practically almost no missions make use of this potential during their lifetime. When long-term, complex IT undertakings (like space missions in our example) were able to exploit these technology performance improvements, considerable savings related to decreased electrical power consumption would be possible.
In addition to these primary financial savings, significant savings of data centre floor space will be achieved during the progress of a mission, which could bring additional financial savings. These can be realised especially at shared IT operations centres, like, for example, shared spacecraft control centres. When some floor space is released after the migration steps, it might be possible to use it instead of constructing new facilities. The availability expressed in terms of square meters*time achieve almost 70% during the reviewed time frame of the tax based write-off period of 25 years. Further research in this area could be undertaken, based on other specific examples of applicability.
While financial savings in terms of electricity may be the primary objective, a reduction of greenhouse gasses output based on the lowered energy consumption is an automatic result. Based on the United States Environmental Protection Agency provided (United States Environmental Protection Agency Citation2018), we found that the emissions originated from electrical power generation consists of 99% of CO2, in terms of greenhouse gasses. We consider the reduction of the emissions to be the most important environmental indicator and goal to be achieved. Such reduced CO2 emissions are definitely the gain for the protection of the environment.
In order to enable the possibility of such financial, floor space, and CO2 savings, we proposed an approach taking the advancements in technology development into consideration during a mission lifetime. If the suggested approach is followed straight from the beginning of a mission, complicated migration steps can not only be avoided, but cost savings will be directly embedded into the IT infrastructure planning.
The outcome of this research, which is mainly the ability to quantify the potential savings enabled by technology advancements, can be applied to a typical scenario of a mission operation. We analysed three larger real-world missions (operating groups of satellites with a duration of 15–20 years). We found that even with just one migration step after 5 years the electricity costs might be reduced by about 67%. There is enough evidence that the costs can be optimised in a self-paying way, even when considering the migration costs. A break-even point will be reached rather quickly and real cost savings will be generated.
Technological aspects
To facilitate the migration strategy, we suggest using systems virtualisation on all levels. Virtualisation enables decoupling the operational software from the technological infrastructure, which enables keeping the infrastructure in line with the latest improvements in the field of technology.
When it comes to CPU/instruction set and virtualisation, special virtualisation layers can be utilised to supply emulated environments, especially for older hardware solutions. A usual solution consists of combining virtual machine and hardware abstraction layers. Abstraction binds modern hardware solutions (not only a CPU instruction set) to legacy emulated hardware utilised by the guest system. An example of such a solution is Charon-AXP (emulating legacy platforms PDP-11, Vax, and AXP alpha server). Another way to handle incompatibilities on memory or storage architecture is using a containerised solution that some vendors provide for standard x86 and/or x64 platforms (HP UX containers) on top of classical virtualisation.
Current wide-spread virtualisation solutions are also bundled with utility tools that simplify tasks like virtual disk conversion. The same can be said for formats compatibility between versions where these tools enable effortless migration. Example implementations of such tools include vmware-vdiskmanager, ovftool, or qemu-img.
Much of the above mentioned is also covered in Open Virtualisation Format that defines a set of standards and metadata for packing and distributing software which is meant to be run in a virtual environment.
In terms of hypervisor hardware compatibility, the usage of newer hardware and an up-to-date hypervisor is suggested as a standard solution. As mentioned above, various emulation layers can be utilised to handle discrepancies. It is very likely that an old hypervisor will be able to work with newer hardware (if we consider reasonably new hypervisors) but won’t be able to take full advantage of the new hardware. Therefore, an up-to-date hypervisor is the best solution in most cases.
Other aspects
While our approach suggests changes towards an existing infrastructure, an additional finding was that it was important to evaluate the changes with regards to their impact on the availability of the system. In the specific researched example, due to the criticality of the infrastructure, no changes, which would decrease the availability, would be acceptable. Gotter (Gotter Citation2017) demonstrated that relevant and required changes to IT systems are possible without actually compromising the availability. The Mean Time Between Failure can be even improved, as the calculations showed.
Beyond the use in the space domain, the found principles can be also applied to a wider range of applications with similar scenarios. In the future, it is to be expected that a large variety of activities related to the public utility sector applications, like traffic flow monitoring, public water supply infrastructure, aircraft traffic control, and power grid control will be based on even more information systems with a long operational lifetime. If concentrated in fewer places they will also create very complex infrastructures. Re-applying the presented approach to such scenarios can also help reduce the cost here significantly.
Conclusion
In this paper, we focused on achieving savings in long-term IT scenarios where the lifetime and complexity significantly exceeds the typical lifetime and complexity of a technological system. We have shown that there is more than a clear possibility for cost savings created through the increase in computational efficiency through technological advancement. We propose an approach that enables us to leverage costs, reduce the space floor requirements, and lower CO2 emissions incurred by the infrastructure.
Based on data characterising technological advancement, a mathematical model that quantifies the benefits of the migration approach based on technological advancement, electrical energy prices, and prices of data centre construction can be derived.
Main cost savings can be realised through savings of electrical energy and floor space of data centres. We demonstrated that potential electricity savings can reach the range of millions of EUR for larger space missions. In addition, the CO2 emissions generated by computing infrastructure can be drastically reduced.
While this paper mainly focuses on energy as well as data centre space savings as the largest cost contributor in this specific context, it is likely that also other costs arising in the context of long-term complex IT operations, e.g., labour or cost of the hardware itself could be lowered. Such cost savings would be subject to further research in this area.
Disclosure statement
No potential conflict of interest was reported by the authors.
Additional information
Notes on contributors
František Dařena
František Dařena is an associate professor and supervisor of master and doctoral study programs at the Department of Informatics, Mendel University, Brno. He has published numerous articles in international scientific journals, conference proceedings, and monographs, and is a member of editorial boards of several international journals and a member of International Association of Engineers. His research includes text/data mining, intelligent data processing, and machine learning.
Florian Gotter
Florian Gotter earned his Ph.D. degree from Mendel University in Brno. He is involved in the field of delivering complex, mission-critical software systems in his professional work.
References
- Angelini, C. 2014. Intel Core i7-5960X, −5930K and −5820K CPU Review. Haswell-E Rises.
- Axelos 2018. “What Is ITIL Best Practice?”
- Belady, C. 2018. “Green Grid Data Center Power Efficiency Metrics: PUE and DCIE.” Technical Report 6, green grid.
- Byte Magazine (1975–1998). “Byte Magazine.” https://archive.org/details/byte-magazine
- Capros, P. De Vita, A, Tasios, N, Siskos, P, Kannavou, M, Petropoulos, A, Evangelopoulou, S, Zampara, M, Papadopoulos, D, Nakos, C, et al. 2016. “Trends to 2050.”
- Cavin, R. K., P. Lugli, and V. V. Shirnov 2012. “Science and Engineering beyond Moore’s Law.” In Proceedings of the IEEE 100 (Special Centennial Issue), 1720–1749.
- CCSDS.Org. 2016. “Consultative Committee for Space Data Systems.”
- Clark, J. 2013. “Is PUE Still Useful?”
- Digital Realty Research Trust. 2012. “Data Center Survey.”
- Fortrust. 2015. “PUE: Is It a Meaningful Measurement of Data Center Efficiency?”
- Gartner. 2009. “Data Center Cost Portfolio.”
- Gotter, F. 2017. “Analysing and Exploiting Technology Driven Cost Factors in Long Term IT Operations in Complex, Mission Critical Operations Centres.” PhD thesis., Mendel University, Brno.
- Gotter, F., and J. Pfau 2014. “Retaining the Operational Status of a Space Mission during the Loss of the Main Control System Using Virtualization.” In Spaceops Conference 2014. AIAA American Institute of Aeronautics and Astronautics, Pasadena.
- Graniszewski, W., and A. Arciszewski. 2016. “Performance Analysis of Selected Hypervisors (Virtual Machine Monitors – VMMs.” International Journal of Electronics and Telecommunications 62 (3): 231–236.
- Gründwald, R., M. Ragwitz, F. Sensfuss, and J. Winkler 2012. “Regenerative Energieträger zur Sicherung der Grundlast in der Stromversorgung.” Technical Report 147, Office for the estimation of technologies at the German Bundestag.
- Hamilton, J. 2011. “Cloud Computing Is Driving Infrastructure Innovation.” Presented at Stanford Computer Forum, Stanford, CA.
- Hintemann, R. 2014. “Consolidation, Colocation, Virtualization, and Cloud Computing: The Impact of the Changing Structure of Data Centers on Total Electricity Demand.” In ICT Innovations for Sustainability, edited by L. M. Hilty and B. Aebischer, 125–136. Springer.
- Hintemann, R., and J. Clausen 2014. “Rechenzentren in Deutschland: Eine Studie zur Darstellung der wirtschaftlichen Bedeutung und der Wettbewerbssituation.” Technical report, Borderstep Institute.
- Hintemann, R., and K. Fichter 2010. “Materialbestand der Rechenzentren in Deutschland.” Technical report, Borderstep Institute.
- HP. 2016. “HP Power Supplies.”
- Intel. 2009. “The Problem of Power Consumption in Servers.”
- Kontorinis, V. Sampson, J, Zhang, LE, Aksanli, B, Homayoun, H, Rosing, TS, Tullsen, DM. et al. 2012. “Battery Provisioning and Associated Costs for Data Center Power Capping.” Technical Report CS2012-0985, Department of Computer Science and Engineering, UC San Diego.
- Koomey, J. 2006. “A Simple Model for Determining True Total Cost of Ownership for Data Centers.” Technical report, Stanford University.
- Koomey, J. 2007. “A Simple Model for Determining True Total Cost of Ownership for Data Centers.” Technical report, Uptime Institute.
- Koomey, J. G., S. Berard, M. Sanchez, and H. Wong. 2009. “Assessing Trends in the Electrical Efficiency of Computation over Time.” IEEE Annals of the History of Computing.
- Koomey, J. G., S. Berard, M. Sanchez, and H. Wong. 2011. “Implications of Historical Trends in the Electrical Efficiency of Computing.” Annals of the History of Computing 33 (3): 46–54.
- Landauer, R. 1982. “Uncertainty Principle and Minimal Energy Dissipation in the Computer.” International Journal of Theoretical Physics 21 (3/4): 283–297.
- Lenovo. 2016. “Providing Energy Efficient Solutions.”
- McCallum, J. C. 2002. “Price-Performance of Computer Technology.” In The Computer Engineering Handbook, edited by G. Oklobdzija, 4: 1–18. Boca Raton, FL: CRC Press.
- McCallum, J. C. 2015. “John C. McCallum.” http://www.jcmit.net
- Mell, W.-D. 2003. “Jenseits von TCO: TCL-Kennzahlen (Total Cost of Lifecycle) als Planungs- und Argumentationshilfen.”
- Miau, J. J., and R. Holdaway, editors. 2000. Reducing the Cost of Spacecraft Ground Systems and Operations. Dordrecht; London: Springer.
- Moore, G. E. 1965. “Cramming More Components onto Integrated Circuits.” Electronics 38 (8): 114–117.
- Newegg Inc. 2012. “Newegg – Online Retailer of Computer Hardware.” http://www.newegg.com
- Pauli, D. 2008. “Think ITIL Will Reduce Cost? You’re Wrong.” Computer-world Australia.
- PC Magazine. 2016. “PC Magazine.” http://www.pcmag.com
- Persse, J. 2012. The ITIL Process Manual. Zaltbommel: Van Haren Publishing.
- Pultz, J. E. 2011. “10 Key Actions to Reduce IT Infrastructure and Operations Cost Structure.”
- Rupp, K. 2014. “CPU, GPU and MIC Hardware Characteristics over Time.”
- Sandau, R. 2006. International Study on Cost-Effective Earth Observation Missions. Boca Raton: CRC Press.
- Schäppi, B., Przywara, B, Bellosa, F, Bogner, T, Weeren, S, Harrison, R, Anglade, A. 2009. “Energy Efficient Servers in Europe.”
- Schembri, T., and O. Boisseau 2015. “On-line Museum since 1985.”
- Schmidt, P., and A. Roos 2009. “New Enterprise Hard Drives 6 GB/s SAS and 200 MB/s.”
- Spear, T. 1998. “NASA FBC Task Final Report.”
- Stengel, S. 2018. http://oldcomputers.net/
- Turner, W. P., and J. H. Seader 2006. “Dollars per kW Plus Dollars per Square Foot are a Better Datacenter Cost Model than Dollars per Square Foot Alone.” Technical report, University of Florida.
- United States Environmental Protection Agency. 2018. “Greenhouse Gas Inventory Data Explorer, Selection of Energy.” https://www.epa.gov/ghgemissions/overview-greenhouse-gases
- Uptime Institute. 2018. “Data Center Site Infrastructure Tier Standard: Topology.”
- Villarrubia, C. 2013. “Portugal Telecom Opens Model Data Center in Portugal.”
- Ward, D. 2010. “Faster, Better, Cheaper Revisited. Program Management Lessons from NASA.” Defense AT&L, March-April:48–52.
- Wertz, J. R., and W. Larson. 1996. Reducing Space Mission Cost. Dordrecht: Springer.
- Wertz, J. R., and W. Larson, editors. 1999. Space Mission Analysis and Design. Dordrecht; Boston: Kluwer.
- Williams, P. 2016. “Data Centers – What are the Costs of Ownership?”
- Wright, L. A., S. Kemp, and I. M. Williams. 2011. “Carbon Footprinting: Towards a Universally Accepted Definition.” Carbon Management 2 (1): 61–72.