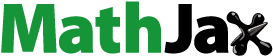
ABSTRACT
Various extensions of the environmentally-conscious quality function deployment (QFD) were explored to address sustainable product design. To provide a systematic computational platform, the integration of fuzzy set theory and multiple criteria decision making (MCDM) methods becomes a popular approach. However, most applications of the fuzzy QFD-MCDM approaches are concentrated on the product planning phase, which limits overall sustainability considerations in the remaining design phases. Thus, this work adopts the means-end chain (MEC) model of the QFD, along with the sustainability agenda of the stakeholders. Succinctly, the proposed framework transforms the MEC model into a series of interconnected hierarchical networks, and each network representing a phase is translated into a supermatrix of the analytic network process (ANP). The fuzzy analytic hierarchy process (AHP) and fuzzy decision-making trial and evaluation laboratory (DEMATEL) are used to populate the supermatrix. To demonstrate its efficacy, an actual case study of vegetable cooking oil is reported in this work. The findings illustrate the capability of the proposed framework in translating the sustainability agenda into insights in the design phases. This consistency of information flow allows stronger associations of the design parameters, ensuring communication of sustainability agenda across phases. The limitations and managerial implications are also discussed.
1. Introduction
In the mid-1960s, quality function deployment (QFD) was introduced by Akao (Citation1972, Citation1990) mainly as a tool for translating customer requirements into meaningful design characteristics. For decades, it has been successfully used as a means in improving the quality of planning, designing, and developing a product as well as strengthening the communication among and between groups, teams, and customers (Chan and Wu Citation2002a; Ko Citation2015; Sivasamy et al. Citation2016). Initially, the QFD is used to convert product characteristics into precise quality control points (Chan and Wu Citation2002b). It is widely considered a customer-oriented visual (Devanathan et al. Citation2010) and an analytical tool that is now fundamental in product design and development (Chan and Wu Citation2002b). It provides an elegant and systematic approach in the translation of customer requirements (CRS) or customer attributes into design requirements (DRS) – also termed by some authors as technical characteristics (Younesi and Roghanian Citation2015) or engineering characteristics (Lee et al. Citation2010; Liu Citation2011). The translation process is carried out using a series of interrelated matrices (Besterfield et al. Citation2004). Chen and Ko (Citation2009) described the QFD in four organised phases: (1) relating the voice of the customer to product design requirements; (2) translating them into parts characteristics; (3) manufacturing operations; and (4) production requirements. The first phase of the QFD is inspired by the house of quality (HoQ) (Younesi and Roghanian Citation2015), and it is considered the central construct of QFD. Majority of published articles on QFD-based product design focus only on the HoQ, representing the first phase of the QFD (e.g., Milunovic Koprivica and Filipovic Citation2018; Zheng, Xu, and Xie Citation2019; Mistarihi, Okour, and Mumani Citation2020).
Recently, in the pursuit of integrating environmental requirements with the traditional DRS, several extensions of the QFD have been introduced in the current literature. The earliest attempt was made by Hochman and O’Connell (Citation1993). Since then, such QFD extensions that highlight environmental considerations have been flourishing. These extensions could be categorised into three main streams. The first stream explicitly incorporates environmental requirements along with the conventional CRS in the evaluation of the DRS. Some popular variants include QFD-DfE (design for environment) (Rahimi and Weidner Citation2002), among others. Another set of works highlights the integration of life cycle assessment (LCA) into the QFD (e.g., Sakao et al. Citation2002; Vinodh and Jayakrishna Citation2014). Lastly, the use of the Theory of Inventive Problem Solving (TRIZ) with green QFD has also gained attention in the domain literature (e.g., Sakao Citation2007; Yeh, Huang, and Yu Citation2011). Following the notion that environmental and economic conditions are deemed insufficient and social considerations become an emerging concern, the direction for sustainable product design which integrates the triple-bottom-line (TBL) requirements (i.e., economic, environmental, and social dimensions) has become an emerging topic for the last decade (Ahmad et al. Citation2018). The conventional approach of dealing with the design parameters, which may offer counterintuitive results when dealing with sustainability, gives rise to more systematic approaches that offer formal computational platforms, allowing design teams to carry out the evaluation process with greater flexibility, mobility across applications, and tractability of computations.
On developing a systematic computational framework of the QFD, the use of multiple criteria decision making (MCDM) methods became a popular approach. Generally, incorporating MCDM methods advances three important aspects of the HoQ: (1) identifying priority CRS, (2) relating DRS with CRS, and (3) aggregating judgements and determining the overall priority DRS. However, it was almost immediately realised that such integration of MCDM methods into the QFD could be augmented with the use of fuzzy set theory (FST), particularly when the design team elicits judgements necessary in most MCDM tools. FST is a possibility theory that, when brought within the context of decision-making, deals with the ambiguity and uncertainty of human decisions (Zadeh Citation1965). Such use is properly motivated as design parameters in the QFD are mostly subjective with limited measurement systems, and human judgements leading to design decisions involving incomplete information. The adoption of fuzzy MCDM into the QFD is almost considered routine (e.g., Zaim et al. Citation2014; Yazdani et al. Citation2017; Wu, Liu, and Wang Citation2017). When fuzzy MCDM is integrated into the QFD, the domain literature performs a combination of two or more of the following actions. First, the FST along with an MCDM (e.g., analytic hierarchy process (AHP)) or a network structuring tool (e.g., decision-making evaluation trial and evaluation laboratory (DEMATEL)) is used to determine the priority weights of the CRS (e.g., Soota Citation2017; Wu, Liu, and Wang Citation2017; Yazdani et al. Citation2017; Mistarihi, Okour, and Mumani Citation2020). Second, a network structuring tool (e.g., fuzzy DEMATEL, analytic network (ANP)) is used to capture the interrelationships among the CRS (e.g., Lin et al. Citation2010; Younesi and Roghanian Citation2015; Wu, Liu, and Wang Citation2017; Yazdani et al. Citation2017; Yazdani, Wang, and Chan Citation2020). Third, similar to the second action, but this time the intertwined relationships of the DRS are addressed via a network structuring tool (e.g., Lin et al. Citation2010; Mistarihi, Okour, and Mumani Citation2020). Finally, an MCDM (or fuzzy MCDM) tool is used to determine the overall priority of the DRS (e.g., Soota Citation2017; Yazdani et al. Citation2017; Wu, Liu, and Wang Citation2017; Fargnoli and Haber Citation2019). It can be revealed that most published works deal with the first and fourth actions, which may limit some inherent information regarding the interrelationships among design parameters.
While domain scholars are persistent in their interest in such integration of QFD and environmental requirements (i.e., also known as partial sustainability), the development of more comprehensive QFD extensions, especially those that address holistic sustainability considerations, is seen to grow slower (Ahmad et al. Citation2018). When sustainability becomes the focus of product design, the TBL of Elkington (Citation1998) is considered the landmark, taking into account environmental stewardship, economic growth, and social well-being. Embracing the sustainability agenda in the manufacturing industry is viewed as a cornerstone in fast-tracking the development of technologies and programmes that would help curb environmental impacts as manufacturing firms face a high amount of waste, materials, and energy requirements, and addressing these challenges pose opportunities for improvement. Despite this importance, the collection of sustainable product design tools is limited (Ahmad et al. Citation2018). One limitation in the current literature of sustainable product design, particularly with the FST and QFD-MCDM framework, is that most applications are concentrated on the product planning phase, and the remaining phases of product development are omitted in the process. When product planning is only addressed, the translation of design priorities to other equally important phases is compromised. It may imply that the sustainability agenda of the customers (or stakeholders) might not be well addressed in the different phases of product development. This myopic view limits the holistic integration of sustainability in product design. Thus, sustainable product design requires an overarching framework that connects the design decisions in the product planning phase down to the production planning phase. In addition, the current FST and QFD-MCDM integration may not be sufficient when considered within the context of connecting design decisions along with the four phases of product development as it fails to account for the four crucial actions when relating the ‘whats’ and the ‘hows’ of the HoQ – the basic tool in translating ‘whats’ into ‘hows’. For instance, to the best of our knowledge, an integrated platform of combining the four actions (i.e., prioritisation of CRS, inner dependencies among CRS, inner dependencies among DRS, and prioritisation of DRS) is missing in the current literature. Such a gap induces information loss, limiting the effective flow of decision priorities across phases of product development.
To overcome these limitations, this work adopts the following framework. First, the CRS are expanded to stakeholder requirements (SRS). This choice is properly motivated. Since internal and external stakeholders control the TBL requirements, current literature emphasises the significance of stakeholders in carrying out the sustainability agenda. Ocampo and Promentilla (Citation2016) pointed out that stakeholders play an important role in the sustainability efforts of the firm as they have established perspectives, experiences, and resources vital to addressing sustainability issues. On this note, it is crucial for product design teams to consider not just the requirements of the ‘customers’ regardless of being environmentally-conscious (e.g., QFDE by Masui, Sakao, and Inaba Citation2001), but to all relevant stakeholders of the product. Thus, for the QFD to support sustainable product design, explicit consideration of stakeholders’ interests must be implemented. Current literature fails to explicitly address the requirements of different stakeholders, particularly social dimensions (Gmelin and Seuring Citation2014; Ahmad et al. Citation2018). Secondly, the means-end chain model promoted by Chen and Ko (Citation2010) and Chen and Ko (Citation2011) is adopted to address the transition of information across product development phases. The means-end chain model translates the current design phase parameters (i.e., ‘whats’) into the next design phase parameters (i.e., ‘hows’), a robust approach to maintaining information flow. It ensures that the requirements of stakeholders, including the sustainability agenda, are reflected continuously in the remaining phases of product development. Finally, to support the means-end chain model, we advance the current FST and QFD-MCDM integration by constructing a hierarchical network that reflects a holistic approach to determining the priority design phase parameters based on four defined actions. In particular, the fuzzy AHP determines the priority weights of previous design phase parameters (i.e., the ‘whats’). Next, the fuzzy DEMATEL is used to identify the important relationships among the previous design phase parameters and among current design phase parameters. Once known, the fuzzy AHP generates the priority weights of these interdependent relationships of parameters. Then, fuzzy AHP relates the current design parameters (i.e., the ‘hows’) to the ‘whats’ by determining the priority weights of the former. The final step requires constructing the corresponding ANP supermatrix that reflects the proposed hierarchical network in order to identify the priority ‘hows’. Integrating this series of hierarchical networks into the means-end chain model offers an overarching platform that ensures the continuous flow of the sustainability agenda across all phases of product development. This framework forms a major contribution to the development of tools for sustainable product design.
Thus, this work aims to demonstrate the methodological framework for sustainable product design that addresses the sustainability agenda of various stakeholders in a means-end chain model with the proposed fuzzy QFD-MCDM as its computational platform. Note that this framework was conceptually formulated by Ocampo et al. (Citation2016), with a recent application reported in Ocampo et al. (Citation2020). However, an elaborate discussion of the framework along with empirical insights is missing, and this work bridges these gaps. For instance, the procedural approach enumerated in the technical note of Ocampo et al. (Citation2016) is reportedly difficult for practitioners to implement due to its insufficient discussion on the proposed methodological process. To obtain crucial insights on how the framework advances sustainable product design, a new case application (i.e., vegetable oil industry) is reported. In summary, the contributions of this work are threefold: (1) it offers an elaborate discussion on the proposed interconnected hierarchical network structures which operationalise the means-end chain model of the QFD, (2) it provides important details on the required computational process in solving the hierarchical networks, and (3) it demonstrates an actual case study of designing a vegetable oil in order to gain empirical insights on the efficacy of the proposed framework for sustainable product design. This paper is organised as follows. Section 2 reviews the literature of the QFD, its extensions, and the cutting-edge methodological approaches attached to it. Section 3 discusses some relevant preliminaries of the fuzzy set theory, fuzzy AHP/ANP, fuzzy DEMATEL, and the proposed framework. The application of the framework in designing a vegetable cooking oil from product planning to production planning is presented in Section 4. Section 5 offers a discussion of insights obtained from the results of the case study. It ends with a conclusion and discussion of future work in Section 6.
2. Literature review
The use of the QFD in product design and development has been phenomenal for the last few decades. Its framework effectively translates customer requirements (CRS) into design characteristics, ensuring that the product represents and satisfies the desires of the customers (Akao Citation1972, Citation1990). The process at which the CRS are translated into specific product design requirements is represented by interconnected matrices (Besterfield et al. Citation2004). The QFD is described in four overarching phases, encompassing product, parts, process, and production planning (Chen and Ko Citation2009). Despite being described in four phases, most reported works are confined within the HoQ – the product planning phase (e.g., Mistarihi, Okour, and Mumani Citation2020). Its wide applications are evident in a number of published works that reviewed and monitored its progress, particularly during the two decades (e.g., Chan and Wu Citation2002a, Citation2002b; Carnevalli and Miguel Citation2008; Sharma, Rawani, and Barahate Citation2008; Mehrjerdi Citation2010; Xu, Xu, and Xie Citation2010; Sivasamy et al. Citation2016). Substantial applications of the QFD have been observed in healthcare (Gremyr and Raharjo Citation2013), education (Aytac and Deniz Citation2005; Agrawal and Sharma Citation2014), manufacturing (Oke Citation2013), and food industry (Costa, Dekker, and Jongen Citation2000).
Due to the persistent environmental impacts associated with the lifecycle of products (Tukker and Jansen Citation2006), integrating environmental requirements into the product planning and design has become an important point of departure (Bovea and Pérez-Belis Citation2012; Den Hollander, Bakker, and Hultink Citation2017; Ferro, Bonollo, and Cruz Citation2021). With QFD as a tool, several modifications have been introduced in the domain literature in an attempt to accommodate environmental concerns on top of the quality-related CRS. The first attempt at integrating some environmental conditions into the QFD was put forward by Hochman and O’Connell (Citation1993). One stream of advances in this area involves the inclusion of environmental requirements along with the CRS in the traditional QFD. These variants include the QFD-DfE, which relates customer satisfaction, cost reduction, and environmental impacts (Rahimi and Weidner Citation2002), QFDEnvironment by Masui, Sakao, and Inaba (Citation2001), environmental QFD which integrates environmental and technological requirements (Kato and Kimura Citation2003), the Eco-VOC in the QFD where the voice of environmentally conscious customers are addressed (Yim Citation2003), the voice of the environment approach using indicator 99 which is coined as E12QFD (Ernzer, Sakao, and Mattheiß Citation2004), QFD to design environmental conscious services (Sakao Citation2007), the Eco-QFD that deploys market demands into environmental requirements proposed by Ernzer and Birkhofer (Citation2003) and adopted by Kuo, Wu, and Shieh (Citation2009), the IGQFD of Cagno and Trucco (Citation2007) which explored Green-QFD II while excluding cost analysis. Another stream of models emphasises the role of LCA in integrating environmental concerns into the QFD. These include the green QFD-II, which is based on life cycle costing and LCA (Zhang Citation1999), the green QFD-III, which combines the life cycle impact assessment and the green HoQ (Mehta and Wang Citation2001), the QFD/LCA that integrates the LCA into QFDE (Sakao et al. Citation2002), and the integrated LCA and environmentally conscious QFD (ECQFD) and (Vinodh and Rathod Citation2010; Vinodh and Jayakrishna Citation2014). Another emerging stream of advances is the incorporation of the TRIZ into the green QFD. For instance, Sakao (Citation2007) proposed the QFDE/LCA/TRIZ that applies LCA and TRIZ to QFDE, Yeh, Huang, and Yu (Citation2011) integrated the QFD and TRIZ along with green considerations, and Donnici et al. (Citation2019) exemplified how QFD and TRIZ complement in the development of innovative urban transportation means. A summary of these advances is detailed in (). Note that this list is nowhere intended to be exhaustive.
Table 1. Extensions of the QFD that integrate environmental considerations
Literature reviews on the recent developments of the QFD along with its emerging applications and trends were reported by Chan and Wu (Citation2002a, Citation2002b), Puglieri, Ometto, and Miguel (Citation2011), Oke (Citation2013), Abdolshah and Moradi (Citation2013). While domain scholars are persistent in their interest in such integration of QFD and environmental requirements, the development of more comprehensive QFD extensions, especially those that address holistic sustainability considerations, popularly under the umbrella of sustainable product design, are gaining attention. Central to sustainable product design is the inclusion of social and environmental perspectives into the traditional CRS. Some methodological approaches of sustainable product design in the context of the QFD include the use of benchmarking (Hosseinpour, Peng, and Gu Citation2015), TRIZ (Caligiana et al. Citation2017; Frizziero et al. Citation2018), LCA (Chiu and Chu Citation2012; Romli et al. Citation2015; Tao and Yu Citation2018), and optimisation (Chowdhury et al. Citation2016; Cheaitou, Gardoni, and Hamdan Citation2019), among others.
Along with the onset of emerging attention for sustainable product design is integrating MCDM methods into the QFD. The role of the MCDM is to promote an analytical and computational platform of the traditional QFD in order to inform the design team on the priority design parameters. Demonstrated in various works, the use of the MCDM methods overcomes the limited computational approach of the conventional QFD, reflecting a more responsive consideration of the inherent relationships among CRS and DRS. The combination is deemed viable since HoQ embodies a matrix approach in the QFD (Aghdam, Mahdavi, and Vahidi Citation2015), and MCDM tools mostly deal with matrix operations. Aside from product design, some applications of QFD-MCDM integration include supplier selection (Dursun and Karsak Citation2013; Rajesh and Malliga Citation2013), workstation selection (Onar et al. Citation2016), supply chain (Chowdhury and Quaddus Citation2015), and health care service design (Chowdhury and Quaddus Citation2016). Emerging works including Lee et al. (Citation2010), Kim, Lee, and Youn (Citation2010), and Younesi and Roghanian (Citation2015) have integrated single MCDM tool or hybrid MCDM tools in QFD as an approach to providing a framework towards sustainable product design. Among several MCDM methods used in QFD-MCDM integration, the AHP, and its generalised form, the ANP are popularly used. The AHP and ANP provide a priority scale from pairwise comparisons of elements (e.g., design parameters) with respect to the higher element in the hierarchy or network. With the aid of the Saaty (Citation1980) fundamental ratio scale, AHP and ANP are applicable and suitable for the quantification of the priorities of CRS and DRS of the QFD. Rajesh and Malliga (Citation2013) integrated AHP with QFD for supplier selection, where AHP was used to compute the weights of CRS and the individual scores for each supplier. Chadawada et al. (Citation2015) have similarly used AHP in QFD for facility location selection. Pal, Ravi, and Bhargava (Citation2007) combined ANP and QFD to incorporate the inner-dependence issues of CRS and DRS in the HoQ for rapid tooling route selection for metal casting.
The use of FST within the QFD-MCDM framework becomes popular because it attempts to address the uncertainty and ambiguity in decision-making, especially when stakeholders in the traditional QFD express their judgements in the natural language (Li Citation2013; Abdolshah and Moradi Citation2013; Zaim et al. Citation2014). The major difference between the conventional QFD and the fuzzy QFD, as demonstrated in the current literature, is that the judgements that relate CRS and DRS of the HoQ of the former are expressed traditionally as symbols with corresponding quantitative scores (Cohen Citation1995), while the latter uses linguistic variables with corresponding fuzzy representations to deal with the imprecision of judgements (Zaim et al. Citation2014; Wu and Ho Citation2015). Vinodh and Chintha (Citation2011) explored the use of the fuzzy QFD to design modular switches that conform to sustainability. Liu (Citation2011) adopted fuzzy QFD for product design and prototype selection. Zaim et al. (Citation2014) integrated FST in the relationship matrix between CRS and DRS. Zhang, Yang, and Liu (Citation2014) used FST, HoQ, and TRIZ for ergonomic product design. Huang et al. (Citation2019) utilised the proportional hesitant fuzzy linguistic terms sets rather than precise numerical values to describe the relationships between CRS and engineering requirements to improve the effectiveness of the QFD. Along with the DEMATEL, Younesi and Roghanian (Citation2015) provided an extension of the QFDE and used fuzzy DEMATEL to incorporate causal relationships among 13 criteria and identified threshold value to select relevant criteria for sustainable product design. They then used the fuzzy ANP to determine the best design criteria. Yan and Ma (Citation2015) implemented FST to model the group behaviours of the QFD. With goal programming integrated into the QFD, FST was adopted by Chen, Ko, and Yeh (Citation2017) for new product planning. Vinodh et al. (Citation2017) explored the use of fuzzy QFD to obtain priority CRS, sustainability parameters, and sustainability initiatives. Yazdani et al. (Citation2017) addressed the relationships between CRS and engineering characteristics using the DEMATEL and argued that DEMATEL facilitates building up the underlying relationship diagram, dividing the considered CRs into cause and effect groups. On the other hand, Wu, Liu, and Wang (Citation2017) used the hesitant fuzzy sets and the DEMATEL to address the interrelationships among CRS and determine their priority weights, while the hesitant fuzzy Vlsekriterijumska Optimizacija I Kompromisno Resenje (VIKOR) method is used to identify the priority engineering characteristics.
To summarise these streams of fuzzy QFD-MCDM approaches, particularly leading to sustainable product design, a combination of two or more actions are carried out in identifying the priority DRS or engineering characteristics: (1) FST, usually in the form of fuzzy numbers, is used to elicit imprecise judgements on the importance of CRS within the framework of an MCDM method (e.g., AHP), (2) the intertwined relationships among the CRS are addressed with the use of analytic tools or MCDM tools (e.g., fuzzy DEMATEL or fuzzy ANP), (3) the intertwined relationships among the DRS are also addressed with the use of analytic tools or MCDM tools (e.g., fuzzy DEMATEL or ANP), and (4) MCDM methods (e.g., AHP, ANP, TOPSIS, VIKOR) are used to obtain the relative importance of the DRS in consideration of the CRS, resulting to the determination of the priority weights within the framework of FST in judgement elicitation of the decision-makers or design team. Nonetheless, these four actions have not been implemented in a single proposed framework, despite their significance in establishing a comprehensive structure that addresses the underlying relationships among design parameters.
The majority of works on sustainable product design, along with the computational platform of FST and QFD-MCDM, only considers the use of the HoQ in the first phase of the QFD (i.e., the product planning phase) while leaving the rest of the product development phases behind. Despite its potential, the use of four-phase QFD in the current literature is scarce, and its use within the domain of sustainable product design is just reported lately. For instance, Shahin and Nikneshan (Citation2008) explored the association of QFD and customer relationship management, and their approach is anchored on the comparison of the QFD phases in product development. However, without a rigorous computational platform, its methodological insight into product development is limited. On the other hand, Yeh, Huang, and Yu (Citation2011) demonstrated the integration of TRIZ in the four phases of the QFD in designing a notebook computer which reflects the innovation and green considerations of the customers. Although outside the literature of sustainable product design, Chen and Ko (Citation2010) offered an interesting platform by building a series of fuzzy linear programming models based on the means-end chain model for the four phases of the QFD process in determining the fulfilment levels of the design parameters of each phase. As shown in (), the means-end chain concept offers a promising framework that ensures the flow of interests of the CRS along with the phases of product development. The efficacy of this concept was again demonstrated by Chen and Ko (Citation2011) with extended fuzzy nonlinear programming formulations. Analogous to the means-end chain concept, Dai and Blackhurst (Citation2012) proposed a four-phase QFD applied to sustainable supplier assessment with the aid of the AHP in the prioritisation process. While the four phases they reflected are not within the domain of product development, their approach on the means-end chain, where the ‘hows’ in the previous phase become the ‘whats’ in the next phase, provides an interesting insight in ensuring the continuity of information across phases. Still, outside the sustainable product design literature, the means-end chain model of the four phases of the QFD was adopted by Singgih, Trenggonowati, and Karningsih (Citation2013) for developing a product that integrates the Kano concept, manufacturing time, and cost. In their framework, the satisfaction and dissatisfaction levels of the ‘whats’ in the current phase are reflected in the ‘hows’ of the next phase. Similarly, using a fuzzy QFD, Liu (Citation2009) extended the usual product planning phase to the design deployment phase using a means-end chain concept. Despite the potential of the means-end chain model in reflecting the sustainability-conscious CRS along with the remaining phases of the QFD, its application is not explored in sustainable product design literature.
Figure 1. Means-end chain model for the phases of the QFD (adapted from Chen and Ko Citation2010, Citation2011)
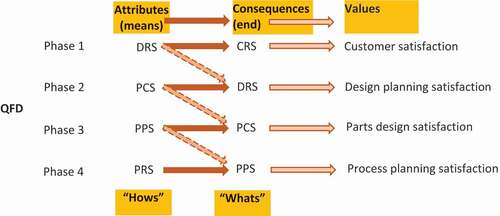
Ahmad et al. (Citation2018) provided a review on sustainable product design and found out that most applications could only be labelled as focusing on partial sustainability, particularly on environmental and economic considerations. They also argue that many partial sustainability tools are based on the QFD, and the application is limited to the early design phase. Ahmad et al. (Citation2018) noted that sustainable product design tools are still premature, and some of them are used in the later design stage. One interesting QFD-based concept for advancing sustainable product design may be explored along with the means-end chain model with MCDM and FST as the computational platforms. While most applications of the QFD rest only on the product planning phase, their sustainability-related insights may not be reflected in the succeeding equally important phases of product development. The means-end chain model overcomes this limitation by enabling a continuous flow of information from the CRS (i.e., sustainability-conscious requirements) down to product planning, design deployment, process planning, and production planning phases. This ensures that the sustainability agenda of the customers (i.e., may be extended to stakeholders) are reflected in all phases of product design. Meanwhile, the FST and MCDM approaches provide a systematic computational framework that captures the subjectivity and imprecision of human judgements (i.e., obtained from the design team) as well as the complexity of decision-making. This integration in the context of sustainable product design is offered recently by Ocampo et al. (Citation2016), with a later application in food products reported in Ocampo et al. (Citation2020). The innovation explores the use of the ANP, with FST, DEMATEL, and AHP as its components, in an attempt to holistically reflect the complex interrelationships among design parameters along with the means-end chain model. The QFD-MCDM integration offered by Ocampo et al. (Citation2016) promotes stronger associations of design parameters and, in effect, limits information loss. This capability supplements the means-end chain model by ensuring that the initial sustainability agenda of the stakeholders for the product are represented in all design decisions across the four phases of product development.
3. Preliminaries
For brevity, the discussion on the HoQ as the central tool in translating CRs into DRs is omitted here as it is widely presented in the domain literature. For instance, see Ocampo et al. (Citation2020) for a concise discussion of the HoQ. We proceed directly to the analytical tools that are relevant in aiding the HoQ to holistically capture the complexity and uncertainty when design stakeholders elicit judgements, as well as in navigating the transitions of preferences along with the phases of the QFD.
3.1. Fuzzy set theory
The FST was introduced by Zadeh (Citation1965) as a framework in computing information with associated vagueness and uncertainty. This monumental work has set forth decades of applications, among them on decision-making under multiple criteria (Carlsson and Fullér Citation1996; Abdullah Citation2013; Mardani, Jusoh, and Zavadskas Citation2015). In classical set theory, a set is a collection of objects , where
represents the universe of discourse. For any
and
, Definition 1 describes the characteristic function.
Definition 1: (Characteristic function) Let where
is a linear space. The characteristic function
of
is defined as
Here, is a crisp set. With vague boundaries of membership, the fuzzy set is defined in Definition 2.
Definition 2: (Fuzzy set). Let be a universe of discourse and
.
is a standard fuzzy set if there exists a membership function
, such that
. The set of 2-tuple
is a fuzzy set where
is a membership function of
.
Fuzzy numbers are fuzzy subsets of . A fuzzy number is a convex normalised fuzzy set in
with a piecewise continuous membership function. Kaufmann and Gupta (Citation1985) provided an introductory discussion of fuzzy numbers and their arithmetic operations. For computational ease, a left-right (L-R) fuzzy number is commonly adopted.
Definition 3: A fuzzy number is of L-R type if there exists a membership function of the form
where is the modal value of
and
are the left and right spreads of
, respectively.
A particular case of an L-R type fuzzy number is a triangular fuzzy number (TFN).
Definition 4. A TFN can be defined as
,
,
, and the membership function
is as follows
Definition 5. Let two TFNs and
. The arithmetic operations of these two TFNs are as follows:
3.2. Fuzzy analytic hierarchy process/analytic network process (AHP/ANP)
The ANP was proposed by Saaty (Citation2001) as the general theory of analysing complex decision problems that are designed as a network of decision components and elements. It is considered as the generalisation of the AHP (Saaty Citation1980), where the latter assumes that the decision problem is structured as a hierarchy. The local priorities or weights obtained from solving the pairwise comparison matrix (PCM) described in the AHP are placed in appropriate locations in the supermatrix. To augment the AHP in dealing with the ambiguity of human decisions present in eliciting judgements in the (PCM), the fuzzy set theory is integrated into the computational framework of the AHP. These approaches include fuzzy logarithmic least squares method (Van Laarhoven and Pedrycz Citation1983), a modified fuzzy logarithmic least squares method (Boender, De Graan, and Lootsma Citation1989), geometric mean method (Buckley Citation1985), an extent analysis method (Chang Citation1996), fuzzy least-squares priority method (Xu Citation2000), fuzzy preference programming method (Mikhailov Citation2003), and the Lambda-Max method (Csutora and Buckley Citation2001). The following steps were adopted from Mikhailov and Tsvetinov (Citation2004) to carry out the required computations of the fuzzy AHP in generating the non-fuzzy weights of the elements in the PCM.
Step 1. Set up the fuzzy PCM represented as:
where is a TFN representing the fuzzy decision when comparing element
against element
with respect to a parent element as elicited by a decision-maker
. Decision-makers elicit judgements based on the linguistic variables with corresponding TFNs, as shown in ().
Table 2. Linguistic scale for the PCM (Tseng et al. Citation2008)
Step 2. Aggregate the judgements of the decision-makers using the weighted geometric mean method shown in EquationEquation (9)
(9)
(9) . The resulting aggregate fuzzy PCM
is presented in EquationEquation (10)
(10)
(10) .
Step 3. Compute for the non-fuzzy priority weights of the elements in the aggregate fuzzy PCM using the nonlinear optimisation model proposed by Mikhailov and Tsvetinov (Citation2004) as shown in EquationEquation (11)(11)
(11) . The model in EquationEquation (11)
(11)
(11) can compute for the weights of sparse PCMs, i.e., incomplete PCMs resulting from the hesitance of decision-makers in eliciting judgements for a particular element or some elements in the matrix.
subject to:
;
;
;
,
;
,
where is the non-fuzzy weight of element
. The consistency of the initial set of fuzzy judgements made by decision-makers can be measured using EquationEquation (11)
(11)
(11) . If the optimal value
, all solution ratios completely satisfy the fuzzy judgements indicating consistency of the initial set of fuzzy judgements. Otherwise, if
, the solution ratios of the fuzzy judgements are strongly inconsistent.
Considering a decision problem described as a decision network, the local priority vectors obtained using EquationEquation (11)(11)
(11) are populated in the initial supermatrix. The initial supermatrix is then transformed into a column stochastic supermatrix, where each column sum is unity. Finally, the column stochastic supermatrix is raised to sufficiently large powers such that rows are identical. This matrix becomes a limiting supermatrix, and each column represents the global priority vector of the decision elements. As long as supermatrix representation is a primitive, irreducible matrix in a strongly connected digraph, the initial supermatrix converges to a limiting supermatrix (Saaty Citation2001).
3.3. Fuzzy DEMATEL
The DEMATEL method was developed by the Battelle Memorial Institute of Geneva for a Science and Human Affairs Program in the 1970s as a tool based on graph theory that considers a system as a graph, where elements and the causal relationships of these elements are represented as vertices and edges, respectively. It accomplishes two objectives: (1) determine the total causal relationships among elements based on direct and indirect relations, and (2) categorise these elements into the net cause and net effect groups. These enable a better understanding of the elements under consideration, which are often intertwined in convoluted problems (Gabus and Fontela Citation1972, Citation1973). Due to its broad applicability in addressing systems defined by elements with complex interrelationships, the DEMATEL has gained popularity in various domain applications. The review of its methodologies and applications in the last decade was put forward by Sheng-Li et al. (Citation2018). The computational algorithm of the DEMATEL is not presented here for brevity, as it is widely described in the literature, even in the domain of sustainable product design (e.g., Singh and Sarkar Citation2020). Similarly, due to the inherent vagueness and uncertainty of human judgements, particularly when decision-makers elicit the degree of causal relationships among the elements, an increasing number of DEMATEL applications adopt the integration of the FST to form the fuzzy DEMATEL approach. In this work, the fuzzy DEMATEL formulation of Lin and Wu (Citation2008) is adopted.
Step 1. Construct the fuzzy direct relation matrix () for each expert decision-maker
, where
, using the linguistic evaluation scale shown in ().
is represented in EquationEquation (12)
(12)
(12) .
Table 3. Linguistic evaluation scale for describing the direct relationships (Tseng Citation2011)
where represents the fuzzy judgement of a decision-maker
when asked about the degree of impact of element
on element
.
Step 2. Aggregate the fuzzy judgements of decision makers using EquationEquation (13)
(13)
(13) . The arithmetic operations on fuzzy numbers presented in EquationEquation (4)
(4)
(4) to EquationEquation (7)
(7)
(7) are applied here.
where as shown in EquationEquation (14)
(14)
(14)
Step 3. Obtain the fuzzy normalised direct relation matrix using EquationEquation (15)
(15)
(15)
where .
Lin and Wu (Citation2008) argued that the transformation of linear scale must be used as a way for normalisation to transform the adopted scales into linear scales. For instance, define as in EquationEquation (16)
(16)
(16) and
is obtained by
Define three crisp matrices by supposing . The elements in these matrices are extracted from
and are presented using EquationEquation (17)
(17)
(17) .
Step 4. Compute for the fuzzy total relation matrix using EquationEquation (18)
(18)
(18) .
where matrix contains TFNs
in which,
, and
;
;
Step 5. Defuzzify the entries in the fuzzy total relation matrix using the centroid method shown as:
Step 6. Experts are asked to set up a threshold value . This value indicates the minimum degree of acceptable impact of one element on another element. If
, then the impact of element
on
is significant. Otherwise if
, the impact is not significant.
Step 7. Set up the reachability matrix by locating the values in the non-fuzzy total-relation matrix, which satisfies
. If so, then
. Otherwise,
.
3.4. The proposed framework: representing the means-end chain model as interconnected hierarchical network structures
The general methodology outlined in this section was reported by Ocampo et al. (Citation2016), with an application demonstrated in Ocampo et al. (Citation2020). Here, we provide a more elaborate and straightforward discussion of the proposed framework, which would allow practitioners to apply it to other product design problems that aim for sustainability. We start by presenting the different sequential phases of product development, as shown in (). The four phases are structured sequentially in such a way that output parameters of one phase become input parameters of the next phase. To further elucidate this relationship, () illustrates how to design information flows from the first phase to the last phase of the QFD.
() describes the following information flow of design parameters. First, the stakeholder requirements (SRS) are exogenously defined, which reflect the sustainability agenda of various stakeholders. These requirements become input information in the product planning phase. This phase performs decision-making processes that would result in priority design requirements (DRS). Note that this process, and the corresponding approaches that deal with it, is highly reported in the product design literature, particularly with the HoQ. The priority DRS parameters are then inputs to the design deployment phase, which produces a set of priority parts characteristics (PCS). Decisions in this phase involve selecting materials, determining parts design parameters, selecting machines and equipment, and other associated tasks. These parameters become inputs to the process planning phase. The decision-makers involved in this phase would make decisions that would identify process parameters (PPS). Finally, the PPS are inputs to the production planning phase. This phase produces the production operations (POS) parameters, which form crucial information to operations management. Note that the product design team identifies these variables (i.e., SRS, DRS, PCS, PPS, and POS) for each product. The overall transition of information along the phases of the QFD allows the reflection of the initial requirements associated with the sustainability agenda of various stakeholders. With this, an analysis of sustainable product design initiatives merely at the product design phase would fall short of a comprehensive perspective on how design parameters are identified in the remaining phases of product development that would support sustainability. Briefly, such an analysis would result in significant information loss in the remaining activities of product development. The majority of the literature reported in the current literature has not bridged this gap, specifically in relation to sustainable product design. The proposed framework, previously proposed by Ocampo et al. (Citation2016) and Ocampo et al. (Citation2020), provides a platform that would significantly advance this gap.
The proposed methodological platform ensures that the initial requirements of various stakeholders, including the sustainability agenda, are promoted for all design parameters throughout the four phases of QFD described in (). It is inspired by the means-end chain model for the four phases of the QFD promoted by Chen and Ko (Citation2011). The main research question addressed in this framework can be explicitly stated as follows: Given the stakeholder requirements that conform to sustainability, what must be the design decisions of all phases of product development, including product planning, design deployment, process planning, and production planning? In general, a series of steps are carried out in each phase to determine the priority weights of the phase design parameters. The series of steps in each phase, as shown in (), forms a hierarchical network of decision elements consistent with the framework of the ANP (e.g., Promentilla et al. Citation2006). The decision network, shown in (), consists of three decision components: the goal (i.e., achieving sustainable product design), the previous design phase parameters, and the current design phase parameters. The network allows capturing the interdependence relationships of decision elements within decision components. There exist dependence relationships of previous design phase parameters with respect to the goal and the dependence relationships of current design phase parameters with respect to previous design phase parameters. In (), the goal component serves as the control element in achieving a strongly connected network (or digraph) (Promentilla et al. Citation2006).
In the context of the ANP, () can be represented as a supermatrix. To aid in the illustration of the computational process, () is provided as the general initial ANP supermatrix for each phase. Note that a supermatrix is a partitioned matrix containing submatrices with column priority vectors. All possible dependence relationships (i.e., the majority are reflected in the decision network of ) are represented as submatrices in (). First, the priority vectors of the parameters of the previous phase are stored in submatrix . Aside from the product design phase, this vector represents the global weights (i.e., obtained from the limiting process of the ANP supermatrix) of the design parameters of the previous phase. For the product design phase, submatrix
reflects the priority vector of the SRS obtained using the fuzzy AHP. Second, the interdependence relationships among previous design phase parameters as well as the interdependence relationships among current design phase parameters are generated via fuzzy DEMATEL. Third, the priority vectors of these interdependence relationships are obtained from the PCMs of the fuzzy AHP. The priority vectors representing the interdependence relationships among previous design phase parameters are reflected in submatrix
. On the other hand, submatrix
represents the priority vectors of the interdependence relationships among current design phase parameters. Fourth, using fuzzy AHP, the priority vectors of current design phase parameters with respect to previous design phase parameters are shown in submatrix
. Fifth, due to the absence of corresponding dependence relationships in (), submatrices
and
are zero matrices in the initial ANP supermatrix. Lastly, for structural purposes (i.e., the presence of the control goal element), submatrices
,
, and
are unit vectors in ().
Figure 6. The initial ANP supermatrix of each phase for solving the global priority weights of the design parameters
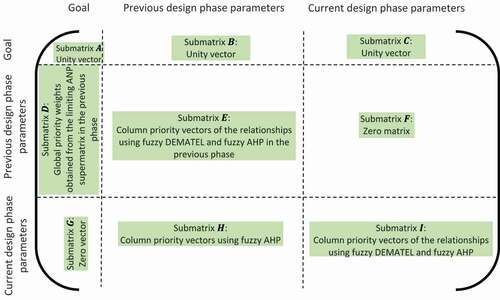
The general procedure of the proposed framework described in () is as follows.
Step 1: Derive the priority vector of the SRS. The design team elicits judgements in the corresponding PCM using the linguistic scale in (). The priority vector is obtained using the fuzzy AHP approach described in EquationEquation (8)(8)
(8) to EquationEquation (11)
(11)
(11) .
Step 2: Determine the interdependence relationships among SRS as well as among DRS using the fuzzy DEMATEL approach presented in EquationEquation (12)(12)
(12) to EquationEquation (20)
(20)
(20) . The linguistic scale in () is used for the design team to elicit judgements in the initial direct relation matrices.
Step 3: Obtain the priority vectors of the interdependence relationships among SRS and among DRS using the fuzzy AHP approach.
Step 4: Following the fuzzy AHP approach again, generate the local priority vectors of the DRS with respect to the SRS.
Step 5: With the priority vectors generated in Step 1 and Step 3, populate the initial supermatrix (i.e., reflecting ) corresponding to the decision network of the product design phase.
Step 6: Obtain the global priority vector of the DRS by generating the limiting supermatrix. Normalise the vector such that the sum of the priorities is unity. These normalised priorities reflect the design decisions of the first phase.
Step 7: Construct the corresponding initial supermatrix for the design deployment phase. The global priority vector of the DRS is populated in submatrix . The priority vectors of submatrix
in the product design phase supermatrix are populated in the submatrix
of the current supermatrix. Repeat Step 2 to Step 4 to populate submatrices
and
. Obtain the global priority vector of the PCS by generating the limiting supermatrix. The normalised priorities reflect the design decisions of the second phase.
Step 8: Construct the corresponding initial supermatrix for the process planning phase. The global priority vector of the PCS is populated in submatrix . The priority vectors of submatrix
in the design deployment phase supermatrix are populated in the submatrix
of the current supermatrix. Repeat Step 2 to Step 4 to populate submatrices
and
. Obtain the global priority vector of the PPS by generating the limiting supermatrix. The normalised priorities reflect the design decisions of the third phase.
Step 9: Construct the corresponding initial supermatrix for the production planning phase. The global priority vector of the PPS is populated in submatrix . The priority vectors of submatrix
in the process planning phase supermatrix are populated in the submatrix
of the current supermatrix. Repeat Step 2 to Step 4 to populate submatrices
and
. Obtain the global priority vector of the POS by generating the limiting supermatrix. The normalised priorities reflect the design decisions of the fourth phase.
This procedural approach enables the priorities to flow across the phases, which implies that the SRS towards sustainability are reflected in all product development phases.
4. The application of the proposed framework: a case of designing a vegetable cooking oil
The dataset of Ocampo et al. (Citation2016) obtained from the XYZ Company is presented in this work to demonstrate the application of the proposed framework. The Wong brothers founded the company in May of 1959 in Cebu (Philippines). Through the years, XYZ has grown to be one of the country’s biggest Filipino-owned pharmaceutical companies and even distributes products globally through its numerous international partners across Asia and the UAE. Today, XYZ is already a leading manufacturer and distributor of liniments, consumer health products, home care, food and food supplements, medical equipment and devices, veterinary products, and pesticides. Sun Valley vegetable cooking oil is one of the company’s food products and becomes the focus of the case study. Early in 2021, a vegetable oil shortage was reported. The Philippines has become the third-largest importer of Malaysian palm oil, after China and Japan, among the 18 countries in the Pacific Region. Thus, the vegetable oil of the country has been in the state of ballooning shortage. Along with other sustainability issues, designing the Sun Valley vegetable cooking oil is considered in this work. () provides the design parameters of the QFD.
Table 4. The design parameters (Ocampo et al. Citation2016)
Ten decision-makers were surveyed to elicit the required expert judgements of the proposed framework.
Step 1: Derive the priority vector of the SRS. () provides the priorities of the SRS.
Table 5. The priority vector of the stakeholder requirements
Step 2: Determine the interdependence relationships among SRS as well as among DRS. Using the fuzzy DEMATEL approach, () summarises the interdependent relationships (i.e., via adjacency matrices) among SRS and among DRS. For brevity, the details are not reflected here. Note that the value 1 represents the existence of the significant causal relationship of the row element on the column element. Otherwise, the value 0 indicates no causal relationship. These realisations are obtained by implementing Step 1 to Step 7 of Section 3.3. (i.e., on fuzzy DEMATEL)
Table 6. The adjacency matrices of SRS and DRS
Step 3: Obtain the priority vectors of the interdependence relationships among SRS and among DRS. Using the fuzzy AHP, the column priority vectors for those with corresponding causal relationships in () are shown in ().
Table 7. The priority vectors of the interdependent relationships among SRS and among DRS
Step 4: With the fuzzy AHP approach, generate the local priority vectors of the DRS with respect to the SRS. These vectors are shown in ().
Table 8. Local priority vectors of DRS with respect to the SRS
Step 5: Populate the initial supermatrix for the product design phase. It is shown in ().
Table 9. The initial supermatrix for the product design phase
Step 6: Obtain the global priority vector of the DRS. The normalised global priority vector is presented in ().
Table 10. The global priority vector of the design requirements
Step 7: Construct the corresponding initial supermatrix for the design deployment phase. The global priority vector of the DRS is populated in submatrix . The priority vectors of submatrix
in the product design phase supermatrix are populated in the submatrix
of the current supermatrix. Repeat Step 2 to Step 4 to populate submatrices
and
. The required steps are shown in (, respectively. Obtain the global priority vector of the PCS by generating the limiting supermatrix, as shown in (). The normalised priorities reflect the design decisions of the second phase. These priorities are shown in ().
Table 11. The priority vectors of the interdependent relationships among PCS
Table 12. Local priority vectors of PCS with respect to the DRS
Table 13. The initial supermatrix for the design deployment phase
Table 14. The global priority vector of the parts characteristics
Step 8: Construct the corresponding initial supermatrix for the process planning phase. The global priority vector of the PCS is populated in submatrix . The priority vectors of submatrix
in the design deployment phase supermatrix are populated in the submatrix
of the current supermatrix. Repeat Step 2 to Step 4 to populate submatrices
and
. These are shown in (), respectively. Obtain the global priority vector of the PPS by generating the limiting supermatrix, as shown in (). In (), the normalised priorities that reflect the design decisions of the third phase are presented.
Table 15. The priority vectors of the interdependent relationships among PPS
Table 16. Local priority vectors of PPS with respect to the PCS
Table 17. The initial supermatrix for the process planning phase
Table 18. The global priority vector of the process parameters
Step 9: Construct the corresponding initial supermatrix for the production planning phase. The global priority vector of the PPS is populated in submatrix . The priority vectors of submatrix
in the process planning phase supermatrix are populated in the submatrix
of the current supermatrix. Repeat Step 2 to Step 4 to populate submatrices
and
, shown in (), respectively. Obtain the global priority vector of the POS by generating the limiting supermatrix as described in (). The normalised priorities, shown in (), reflect the design decisions of the fourth phase.
Table 19. The priority vectors of the interdependent relationships among POS
Table 20. Local priority vectors of POS with respect to the PPS
Table 21. The initial supermatrix for the production operations phase
Table 22. The global priority vector of the production operations
5. Discussion and managerial insights
The product planning phase, the first phase of the QFD, translates stakeholder requirements into a set of design requirements. The expanded stakeholder requirements explored in this work advance the traditional notion of customer requirements in the domain literature, as the latter may be limited in view of the sustainability agenda. With the interests of various stakeholders integrated into the phases of product development, a more holistic perspective that is direct towards sustainability is guaranteed. It is presumed that these stakeholder requirements contain the sustainability agenda of various stakeholders. In the case study, results show that FDA compliance (SR6) has the highest priority for the case vegetable cooking oil, followed by requirements related to safe for consumption (SR3), and then by three SRS with the same weights, namely the product quality (SR1), proper wastewater and solid disposal (SR4), and competitive pricing (SR10). The Food and Drug Administration (FDA) is a government agency responsible for protecting and promoting public health through the regulation and supervision of food safety, among others. Along with safe for consumption (SR3), FDA compliance (SR6) is a crucial requirement in the development of the case product, which is also linked to Good 2 (zero hunger), Goal 3 (good health and well-being), Goal 12 (responsible consumption and production), and Goal 17 (partnerships for the goals) of the UN Sustainable Development Goals (United Nations Citation2021). The other three priority SRS are linked to the traditional economic goal (e.g., SR1 and SR10) and environmental initiatives (e.g., SR4). This reflection of the priority SRS, particularly in the social dimensions and public health, may not be realised with the use of most QFD extensions in the domain literature, as they are confined to economic and environmental requirements. With the case product, the least priority SRS is the differentiator (SR9) requirement, which is associated with a marketing goal. The rank order of the SRS is as follows: SR6SR3
SR1
SR4
SR10
SR11
SR2
SR8
SR5
SR7
SR9.
Translated from the stakeholder requirements, the most priority design requirement for the case product is the GMP and HACCP accreditation (DR8), followed by the requirement related to accepting only safe raw materials (DR2) and treating wastewater and segregating solid waste (DR6). Accreditation for GMP is part of the quality system; it ensures that the case vegetable oil product is consistently produced according to established quality standards. It attempts to eliminate contamination, mix-ups, and errors. On the other hand, HACCP is a systematic preventive approach to food safety from hazards in the production process. With this, GMP and HACCP accreditation would help the organisation build up its quality brand for ensuring competitive advantage. For instance, if customers want to choose between two suppliers with only one having a GMP and HACCP accreditation, customers are more likely to choose the former since having this accreditation means that the supplier has an established process that conforms to product safety and quality. The second priority in the DRS relates again to product safety of raw materials (i.e., DR2) and environmental considerations (i.e., DR6). These priority DRS reflect well the priority requirements of the stakeholders. On the other hand, the requirement of adding Vitamin A to the product’s formula (DR7) rests at the bottom of the priority list of DRS, as its corresponding SR (i.e., fortify product with Vitamin A (SR5)) is of the tail end of the list of priority SRS. The complete priority list of DRS is as follows: DR8DR2
DR6
DR1
DR3
DR5
DR4
DR11
DR10
DR12
DR9
DR7.
In the second phase of the QFD (i.e., the process planning phase), the design requirements are translated into parts characteristics. In the case of vegetable oil product, the most priority in the list of PCS is the requirement related to following standard specifications, procedures, and processes (PC1), which are associated with the specifications of oil such as colour, odour, and taste. This characteristic is highly linked to the most priority DR (i.e., DR8) in the previous phase. Subscribing to GMP and HACCP accreditation would necessitate the case firm to follow certain established product specifications, particularly in relation to the required raw materials. Fulfiling with standard specifications would increase customers’ reliability on the product. This may set forth guidelines in important decision areas of the firm, particularly in supplier selection. For instance, the case firm would want to have a supplier capable of providing them with consistent product specifications, such as the weight, dimensions, colour, among others, in order to minimise if not eliminate product inconsistencies. PC1 is followed by characteristics associated with do not accept defective raw materials (PC2) and study and compare your product and competitors’ (PC4). They are likewise reflective of the priority DRS of the previous phase. Due to the less priority attached to establishing pricing strategy at par with competitors (DR11) in the design deployment phase, the characteristic relating to being aware of competitors’ prices (PC6) ranks last in the priority list of PCS. In summary, the rank order of PCS is as follows: PC1PC2
PC4
PC5
PC3
PC6.
The process planning phase translates the PCS into process parameters. With the proposed framework, the parameter in relation to criticising all aspects of the product and making improvements (PP3) yields the highest priority in this phase. It is followed by careful inspection of raw materials (PP1) and providing work instructions (PP6). These parameters are consistent with the priority characteristics associated with following standard specifications and examining raw materials in the design deployment phase. When process managers in the production floor implement crucial measures for incoming raw materials, down to upholding standard product specifications, processes, and systems, they must initiate a continuous review and holistic improvements in their processes. Thus, these priority PPS are closely linked to PCS and subsequently to DRS and SRS, illustrating the constant translation of priority requirements of stakeholders along with the product development phases. This observation is consistent with the last PP in the list, i.e., concerns observing and understanding competitor’s pricing strategy (PP5), as its corresponding PC in the previous phase ranks last on the priority list. The priority list of the process planning phase yields the following: PP3PP1
PP6
PP4
PP2
PP5.
In the final phase, the PPS are translated into priority production operations. In the case of vegetable oil product, the most priority PO is for production managers to consult experts regularly (PO2) to seek expert advice on the best courses of action on how to make improvements to their operations. Next in the priority list is relating to regular updating of work instructions (PO4). These priority parameters of the production planning phase reflect the priority parameters of the previous design phases of product development. In particular, expert consultation regarding production operations (PO2) supports continuous improvement efforts (PP3), raw material evaluation (PP1), and establishing standard work instructions (PP6) in the process planning phase. These PPS consequently support standards (i.e., product specifications, procedures, and processes) (PC1) and quality raw materials (PC2) in the design deployment phase. These are then linked to achieving product safety accreditation standards (DR8), raw materials (DR2), and environmental considerations (DR6) in the product planning phase, which can be ultimately traced back to stakeholder requirements, such as compliance to FDA standards (SR6) and safe consumption (SR3) of the product. The product planning phase yields the following priority order of the parameters: PO2PO4
PO1
PO3.
Although the findings of this work are case-specific and idiosyncratic, they are encouraging and are important starting points of discussions for managers and practitioners seeking to implement sustainable product design. They must be used to complement previous findings in the field, particularly those exploring food product design and production. Nevertheless, the proposed framework proves a methodological advantage over other QFD extensions, most notably from realising that it supports the consistency of translating the requirements of the stakeholders, including those related to environmental and social components of the product and its corresponding processes and operations, into meaningful insights for design teams in QFD phases. This consistency of priority flows from the stakeholder requirements down to the phases of product development allows stronger interlinkages of priorities of the design parameters, ensuring that any sustainability agenda of the stakeholders are translated across all important phases of product, process, and operations design. Thus, unlike most literature which only focuses on the first phase for engaging in sustainable product design, the proposed methodological framework highlights the consistent transition of sustainability requirements along with all design phases in a manner that is analytic, under an environment that captures complexity and uncertainty in decision making.
Although the proposed methodology offers important contributions for holistically designing sustainable products, it holds certain limitations. First, the priority parameters of each design phase are highly dependent on expert judgements. Thus, the composition of the design team who would be tasked to elicit judgements in the framework of the fuzzy AHP and fuzzy DEMATEL is crucial in attaining meaningful design insights. Second, achieving a consensus of judgements is a challenge in the AHP and DEMATEL methods. More elaborate and responsive judgement aggregation methodologies (e.g., Delphi, aggregation operators) aside from EquationEquation (9)(9)
(9) and EquationEquation (13)
(13)
(13) may be needed to facilitate a more representative group judgement. Third, as in any QFD-based design framework, the choice of the design parameters of the different phases is an important step in effectively informing the design team on the specific requirements for the product, processes, or systems. Thus, design managers must ensure that the members of the team must have technical knowledge about the product and its corresponding processes. Otherwise, the practical value of the design insights may be weaker. Finally, the computational process of the proposed methodology is quite complex, and for obvious practical reasons, analysts may consider the development of software and applications that would carry out the required computations.
6. Conclusion and future work
Despite the importance of sustainable product design in the sustainability discourse, which would address resource consumption, energy use, and waste generation, various tools to support its agenda are limited in the domain literature. The use of the QFD has been central to product design, and various extensions, particularly on integrating environmental considerations into the conventional customer requirements, have become a popular topic for the last two decades. With the onset of the holistic sustainability agenda, emerging directions in the literature promote the advances of going beyond the economic and environmental requirements to include social dimensions. Simultaneously, to encourage a more systematic computational platform that would address the relationships of design parameters, prioritisation requirements, and ambiguity in the decision-making process of the QFD, integrating fuzzy set theory and MCDM methods into the QFD (i.e., particularly the HoQ) has recently gained increasing attention. However, most applications are limited to the product planning phase of the QFD, leaving other phases of product development outside the analysis. This remains an important gap as the sustainability agenda reflected in the customer requirements may not be translated into the design parameters of the succeeding phases of product development. Thus, addressing this gap by developing a platform that ensures the continuous flow (e.g., means-end chain model) of the initial sustainability agenda offers a promising step towards sustainable product design. When taken within this context, current FST and MCDM integrations into the QFD may yield some crucial information loss that may not complement well with the potential information flow of the means-end chain model.
Thus, this work overcomes these limitations by proposing interconnected hierarchical networks to represent the means-end chain model. In addition, instead of addressing conventional customer requirements, the sustainability agenda are obtained from the stakeholders. Constructed for each phase, the hierarchical network allows the implementation of four crucial information: (1) prioritisation of the previous design phase parameters (i.e., the ‘whats’) using fuzzy AHP, (2) identification of relevant interrelationships among ‘whats’ and among ‘hows’ with fuzzy DEMATEL, and generation of priority weights of the interdependent relationships among ‘whats’ and among ‘hows’ with fuzzy AHP, (3) prioritisation of ‘hows’ to each ‘what’ with fuzzy AHP, and (4) the ANP supermatrix aggregates these priority vectors generated in (1) through (3) in order to identify the overall priority ‘hows’. This methodological innovation highlights the contribution of this work. The proposed framework was applied in an actual case study of designing a vegetable cooking oil. The case-specific results suggest that compliance to FDA standards and safe consumption of the product are priority stakeholder requirements. These priority agenda generate design requirements linked to product safety accreditation standards, raw materials, and environmental considerations in the product planning phase. These parameters support compliance of standards (i.e., product specifications, procedures, and processes) and acceptance of quality raw materials in the design deployment phase. To support these parts design parameters, processes must promote continuous improvement efforts, raw material evaluation, and standard work instructions in the process planning phase. Finally, production managers regularly consulting experts to seek expert advice for constinuous improvements in their production operations, and regular updating of work instructions becomes the priority parameters in the production planning phase.
These findings are not universal, but they can be starting points of discussions for managers and practitioners seeking a sustainable product design for vegetable cooking oils. Most importantly, they demonstrate that the proposed framework supports the consistency of translating the sustainability agenda into design insights in the product development phases. This consistency of information flow allows associations of priorities of the design parameters, ensuring that any sustainability agenda of the stakeholders are communicated across all design phases. Thus, the proposed framework inspired by the means-end chain model is potentially beneficial for sustainable product design, especially in an environment that embodies complexity and uncertainty in decision making. Furthermore, its platform offers flexibility in other domain applications under different products and expert groups, with greater computational tractability. Also, the process-oriented nature of the proposed methodology and the discussion resulting from its application add much to the learning such an approach creates. For future work, the proposed method could be adopted in the sustainable design of other products. Other MCDM methods (e.g., entropy weight method, best-worst method) could be explored on behalf of fuzzy AHP in the hierarchical network structure. The use of fuzzy decision maps (Tzeng et al. Citation2010) could unify interrelationship identification and prioritisation processes required to populate the ANP supermatrix of each phase. Finally, other methods that capture uncertainty in decision-making (e.g., intuitionistic fuzzy sets, neutrosophic sets, type-2 fuzzy sets, grey theory) could be an interesting agenda for future work.
Disclosure Statement
No potential conflict of interest was reported by the author(s).
References
- Abdolshah, M., and M. Moradi. 2013. “Fuzzy Quality Function Deployment: An Analytical Literature Review.” Journal of Industrial Engineering 682532. doi:https://doi.org/10.1155/2013/682532.
- Abdullah, L. 2013. “Fuzzy Multi Criteria Decision Making and Its Applications: A Brief Review of Category.” Procedia-Social and Behavioral Sciences 97: 131–136. doi:https://doi.org/10.1016/j.sbspro.2013.10.213.
- Aghdam, M. M., I. Mahdavi, and J. Vahidi. 2015, March. “House of Quality Improvement by New Design Requirements Generation.” In 2015 International Conference on Industrial Engineering and Operations Management (IEOM), IEEE, Dubai, UAE, 1–9.
- Agrawal, T., and J. Sharma. 2014. “Quality Function Deployment in Higher Education: A Literature Review.” International Journal of Service Science, Management, Engineering, and Technology 5 (1): 1–13. doi:https://doi.org/10.4018/ijssmet.2014010101.
- Ahmad, S., K. Y. Wong, M. L. Tseng, and W. P. Wong. 2018. “Sustainable Product Design and Development: A Review of Tools, Applications and Research Prospects.” Resources, Conservation and Recycling 132: 49–61. doi:https://doi.org/10.1016/j.resconrec.2018.01.020.
- Akao, Y. 1972. “New Product Development and Quality Assurance–quality Deployment System.” Standardization and Quality Control 25 (4): 7–14.
- Akao, Y. 1990. Quality Function Deployment: Integrating Customer Requirements into Product Design. trans. G. H. Mazur. Cambridge, M.A:Productivity Press.
- Aytac, A., and V. Deniz. 2005. “Quality Function Deployment in Education: A Curriculum Review.” Quality & Quantity 39 (4): 507–514. doi:https://doi.org/10.1007/s11135-004-6814-8.
- Besterfield, D., C. Besterfield-Michna, G. Besterfield, and M. Besterfield-Sacre. 2004. Total Quality Management. India: Pearson Education.
- Boender, C. G. E., J. G. De Graan, and F. A. Lootsma. 1989. “Multi-criteria Decision Analysis with Fuzzy Pairwise Comparisons.” Fuzzy Sets and Systems 29 (2): 133–143. doi:https://doi.org/10.1016/0165-0114(89)90187-5.
- Bovea, M. D., and V. Pérez-Belis. 2012. “A Taxonomy of Ecodesign Tools for Integrating Environmental Requirements into the Product Design Process.” Journal of Cleaner Production 20 (1): 61–71. doi:https://doi.org/10.1016/j.jclepro.2011.07.012.
- Buckley, J. J. 1985. “Fuzzy Hierarchical Analysis.” Fuzzy Sets and Systems 17 (3): 233–247. doi:https://doi.org/10.1016/0165-0114(85)90090-9.
- Cagno, E., and P. Trucco. 2007. “Integrated Green and Quality Function Deployment.” International Journal of Product Lifecycle Management 2 (1): 64–83. doi:https://doi.org/10.1504/IJPLM.2007.012875.
- Caligiana, G., A. Liverani, D. Francia, L. Frizziero, and G. Donnici. 2017. “Integrating QFD and TRIZ for Innovative Design.” Journal of Advanced Mechanical Design, Systems, and Manufacturing 11 (2): JAMDSM0015. doi:https://doi.org/10.1299/jamdsm.2017jamdsm0015.
- Carlsson, C., and R. Fullér. 1996. “Fuzzy Multiple Criteria Decision Making: Recent Developments.” Fuzzy Sets and Systems 78 (2): 139–153. doi:https://doi.org/10.1016/0165-0114(95)00165-4.
- Carnevalli, J. A., and P. C. Miguel. 2008. “Review, Analysis and Classification of the Literature on QFD—Types of Research, Difficulties and Benefits.” International Journal of Production Economics 114 (2): 737–754. doi:https://doi.org/10.1016/j.ijpe.2008.03.006.
- Chadawada, R., A. Sarfaraz, K. Jenab, and H. Pourmohammadi. 2015. “Integration of AHP-QFD for Selecting Facility Location.” Benchmarking: An International Journal 22 (3): 411–425. doi:https://doi.org/10.1108/BIJ-06-2013-0064.
- Chan, L. K., and M. L. Wu. 2002a. “Quality Function Deployment: A Comprehensive Review of Its Concepts and Methods.” Quality Engineering 15 (1): 23–35. doi:https://doi.org/10.1081/QEN-120006708.
- Chan, L. K., and M. L. Wu. 2002b. “Quality Function Deployment: A Literature Review.” European Journal of Operational Research 143 (3): 463–497.
- Chang, D. Y. 1996. “Applications of the Extent Analysis Method on Fuzzy AHP.” European Journal of Operational Research 95 (3): 649–655. doi:https://doi.org/10.1016/0377-2217(95)00300-2.
- Cheaitou, A., M. Gardoni, and S. Hamdan. 2019. “A Decision-making Framework for Environmentally Sustainable Product Design.” Concurrent Engineering 27 (4): 295–304. doi:https://doi.org/10.1177/1063293X19870841.
- Chen, L. H., and W. C. Ko. 2009. “Fuzzy Linear Programming Models for New Product Design Using QFD with FMEA.” Applied Mathematical Modelling 33 (2): 633–647. doi:https://doi.org/10.1016/j.apm.2007.11.029.
- Chen, L. H., and W. C. Ko. 2010. “Fuzzy Linear Programming Models for NPD Using a Four-phase QFD Activity Process Based on the Means-end Chain Concept.” European Journal of Operational Research 201 (2): 619–632. doi:https://doi.org/10.1016/j.ejor.2009.03.010.
- Chen, L. H., and W. C. Ko. 2011. “Fuzzy Nonlinear Models for New Product Development Using Four-phase Quality Function Deployment Processes.” IEEE Transactions on Systems, Man, and Cybernetics-Part A: Systems and Humans 41 (5): 927–945. doi:https://doi.org/10.1109/TSMCA.2010.2104140.
- Chen, L. H., W. C. Ko, and F. T. Yeh. 2017. “Approach Based on Fuzzy Goal Programing and Quality Function Deployment for New Product Planning.” European Journal of Operational Research 259 (2): 654–663. doi:https://doi.org/10.1016/j.ejor.2016.10.028.
- Chiu, M. C., and C. H. Chu. 2012. “Review of Sustainable Product Design from Life Cycle Perspectives.” International Journal of Precision Engineering and Manufacturing 13 (7): 1259–1272. doi:https://doi.org/10.1007/s12541-012-0169-1.
- Chowdhury, M. M. H., and M. A. Quaddus. 2015. “A Multiple Objective Optimization Based QFD Approach for Efficient Resilient Strategies to Mitigate Supply Chain Vulnerabilities: The Case of Garment Industry of Bangladesh.” Omega 57: 5–21. doi:https://doi.org/10.1016/j.omega.2015.05.016.
- Chowdhury, M. M. H., and M. A. Quaddus. 2016. “A Multi-phased QFD Based Optimization Approach to Sustainable Service Design.” International Journal of Production Economics 171: 165–178. doi:https://doi.org/10.1016/j.ijpe.2015.09.023.
- Cohen, L. 1995. Quality Function Deployment: How to Make QFD Work for You. Boston, MA, USA: Addison-Wesley Publishing Company.
- Costa, A. I. A., M. Dekker, and 4. 7. 4. W. Jongen. 2000. “Quality Function Deployment in the Food Industry: A Review.” Trends in Food Science & Technology 11 (9–10): 306–314. doi:https://doi.org/10.1016/S0924-2244(01)00002-4.
- Csutora, R., and J. J. Buckley. 2001. “Fuzzy Hierarchical Analysis: The Lambda-Max Method.” Fuzzy Sets and Systems 120 (2): 181–195. doi:https://doi.org/10.1016/S0165-0114(99)00155-4.
- Dai, J., and J. Blackhurst. 2012. “A Four-phase AHP–QFD Approach for Supplier Assessment: A Sustainability Perspective.” International Journal of Production Research 50 (19): 5474–5490. doi:https://doi.org/10.1080/00207543.2011.639396.
- Den Hollander, M. C., C. A. Bakker, and E. J. Hultink. 2017. “Product Design in a Circular Economy: Development of a Typology of Key Concepts and Terms.” Journal of Industrial Ecology 21 (3): 517–525. doi:https://doi.org/10.1111/jiec.12610.
- Devanathan, S., D. Ramanujan, W. Z. Bernstein, F. Zhao, and K. Ramani. 2010. “Integration of Sustainability into Early Design through the Function Impact Matrix.” Journal of Mechanical Design 132 (8): 081004. doi:https://doi.org/10.1115/1.4001890.
- Donnici, G., L. Frizziero, D. Francia, A. Liverani, and G. Caligiana. 2019. “Innovation Design Driven by QFD and TRIZ to Develop New Urban Transportation Means.” Australian Journal of Mechanical Engineering 1–17. doi:https://doi.org/10.1080/14484846.2019.1615259.
- Dursun, M., and E. E. Karsak. 2013. “A QFD-based Fuzzy MCDM Approach for Supplier Selection.” Applied Mathematical Modelling 37 (8): 5864–5875. doi:https://doi.org/10.1016/j.apm.2012.11.014.
- Elkington, J. 1998. “Partnerships from Cannibals with Forks: The Triple Bottom Line of 21st‐century Business.” Environmental Quality Management 8 (1): 37–51. doi:https://doi.org/10.1002/tqem.3310080106.
- Ernzer, M., and H. Birkhofer. 2003, May. “How to Carry Out Life Cycle design?-Methodical Support for Product Developers.” In Proceedings of the 1st International Workshop on Sustainable Consumption, Tokyo, Japan, 19–20.
- Ernzer, M., T. Sakao, and C. Mattheiß. 2004. “Effective and Efficient Application of Eco-QFD.” In DS 32: Proceedings of DESIGN 2004, the 8th International Design Conference, Dubrovnik, Croatia.
- Fargnoli, M., and N. Haber. 2019. “A Practical ANP-QFD Methodology for Dealing with Requirements’ Inner Dependency in PSS Development.” Computers & Industrial Engineering 127: 536–548. doi:https://doi.org/10.1016/j.cie.2018.10.042.
- Ferro, P., F. Bonollo, and S. A. Cruz. 2021. “Product Design from an Environmental and Critical Raw Materials Perspective.” International Journal of Sustainable Engineering 14 (1): 1–11. doi:https://doi.org/10.1080/19397038.2020.1719445.
- Frizziero, L., D. Francia, G. Donnici, A. Liverani, and G. Caligiana. 2018. “Sustainable Design of Open Molds with QFD and TRIZ Combination.” Journal of Industrial and Production Engineering 35 (1): 21–31. doi:https://doi.org/10.1080/21681015.2017.1385543.
- Gabus, A., and E. Fontela. 1972. “World Problems, an Invitation to Further Thought within the Framework of DEMATEL.” Battelle Geneva Research Center, Geneva, Switzerland, 1–8.
- Gabus, A., and E. Fontela. 1973. “Perceptions of the World Problematique: Communication Procedure, Communicating with Those Bearing Collective Responsibility.” Battelle Geneva Research Centre, Geneva, Switzerland.
- Gmelin, H., and S. Seuring. 2014. “Determinants of a Sustainable New Product Development.” Journal of Cleaner Production 69: 1–9. doi:https://doi.org/10.1016/j.jclepro.2014.01.053.
- Gremyr, I., and H. Raharjo. 2013. “Quality Function Deployment in Healthcare: A Literature Review and Case Study.” International Journal of Health Care Quality Assurance 26 (2): 135–146. doi:https://doi.org/10.1108/09526861311297343.
- Hochman, S. D., and P. A. O’Connell. 1993. “Quality Function Deployment: Using the Customer to Outperform the Competition on Environmental Design.” Proceedings of the 1993 IEEE International Symposium on Electronics and the Environment, Arlington, VA, USA, 165–172.
- Hosseinpour, A., Q. Peng, and P. Gu. 2015. “A Benchmark-based Method for Sustainable Product Design.” Benchmarking: An International Journal 22 (4): 643–664. doi:https://doi.org/10.1108/BIJ-09-2014-0092.
- Huang, J., X. Y. You, H. C. Liu, and S. L. Si. 2019. “New Approach for Quality Function Deployment Based on Proportional Hesitant Fuzzy Linguistic Term Sets and Prospect Theory.” International Journal of Production Research 57 (5): 1283–1299. doi:https://doi.org/10.1080/00207543.2018.1470343.
- Kato, S., and F. Kimura. 2003. “Systematization of Product Life Cycle Technology Utilizing the QFD Method.” In Proceedings of Ecodesign 2003: Third International Symposium On Environmentally Conscious Design And Inverse Manufacturing, Tokyo, Japan.
- Kaufmann, A., and M. M. Gupta. 1985. Introduction to Fuzzy Arithmetic: Theory and Applications. New York: Van Nostrand Reinhold Company.
- Kim, T., H. K. Lee, and E. M. Youn. 2010, July. “Product Family Design Based on Analytic Network Process.” In The 40th International Conference on Computers & Indutrial Engineering, IEEE, Awaji City, Japan, 1–6.
- Ko, W. C. 2015. “Construction of House of Quality for New Product Planning: A 2-tuple Fuzzy Linguistic Approach.” Computers in Industry 73: 117–127. doi:https://doi.org/10.1016/j.compind.2015.07.008.
- Kuo, T. C., H. H. Wu, and J. I. Shieh. 2009. “Integration of Environmental Considerations in Quality Function Deployment by Using Fuzzy Logic.” Expert Systems with Applications 36 (3): 7148–7156. doi:https://doi.org/10.1016/j.eswa.2008.08.029.
- Lee, A. H., H. Y. Kang, C. Y. Yang, and C. Y. Lin. 2010. “An Evaluation Framework for Product Planning Using FANP, QFD and Multi-choice Goal Programming.” International Journal of Production Research 48 (13): 3977–3997. doi:https://doi.org/10.1080/00207540902950845.
- Li, Q. 2013. “A Novel Likert Scale Based on Fuzzy Sets Theory.” Expert Systems with Applications 40 (5): 1609–1618. doi:https://doi.org/10.1016/j.eswa.2012.09.015.
- Lin, C. J., and W. W. Wu. 2008. “A Causal Analytical Method for Group Decision-making under Fuzzy Environment.” Expert Systems with Applications 34 (1): 205–213. doi:https://doi.org/10.1016/j.eswa.2006.08.012.
- Lin, Y., H. P. Cheng, M. L. Tseng, and J. C. Tsai. 2010. “Using QFD and ANP to Analyze the Environmental Production Requirements in Linguistic Preferences.” Expert Systems with Applications 37 (3): 2186–2196. doi:https://doi.org/10.1016/j.eswa.2009.07.065.
- Liu, H. T. 2009. “The Extension of Fuzzy QFD: From Product Planning to Part Deployment.” Expert Systems with Applications 36 (8): 11131–11144. doi:https://doi.org/10.1016/j.eswa.2009.02.070.
- Liu, H. T. 2011. “Product Design and Selection Using Fuzzy QFD and Fuzzy MCDM Approaches.” Applied Mathematical Modelling 35 (1): 482–496. doi:https://doi.org/10.1016/j.apm.2010.07.014.
- Mardani, A., A. Jusoh, and E. K. Zavadskas. 2015. “Fuzzy Multiple Criteria Decision-making Techniques and applications–Two Decades Review from 1994 to 2014.” Expert Systems with Applications 42 (8): 4126–4148.
- Masui, K., T. Sakao, and A. Inaba. 2001, December. “Quality Function Deployment for Environment: QFDE (1st Report)-a Methodology in Early Stage of DfE.” In Proceedings Second International Symposium on Environmentally Conscious Design and Inverse Manufacturing, IEEE, Tokyo, Japan, 852–857.
- Mehrjerdi, Y. Z. 2010. “Quality Function Deployment and Its Extensions.” International Journal of Quality & Reliability Management 27 (6): 616–640. doi:https://doi.org/10.1108/02656711011054524.
- Mehta, C., and B. Wang. 2001. “Green Quality Function Deployment III: A Methodology for Developing Environmentally Conscious Products.” Journal of Design and Manufacturing Automation 1 (1–2): 1–16. doi:https://doi.org/10.1080/15320370108500198.
- Mikhailov, L. 2003. “Deriving Priorities from Fuzzy Pairwise Comparison Judgements.” Fuzzy Sets and Systems 134 (3): 365–385. doi:https://doi.org/10.1016/S0165-0114(02)00383-4.
- Mikhailov, L., and P. Tsvetinov. 2004. “Evaluation of Services Using a Fuzzy Analytic Hierarchy Process.” Applied Soft Computing 5 (1): 23–33. doi:https://doi.org/10.1016/j.asoc.2004.04.001.
- Milunovic Koprivica, S., and J. Filipovic. 2018. “Application of Traditional and Fuzzy Quality Function Deployment in the Product Development Process.” Engineering Management Journal 30 (2): 98–107. doi:https://doi.org/10.1080/10429247.2018.1438027.
- Mistarihi, M. Z., R. A. Okour, and A. A. Mumani. 2020. “An Integration of a QFD Model with Fuzzy-ANP Approach for Determining the Importance Weights for Engineering Characteristics of the Proposed Wheelchair Design.” Applied Soft Computing 90: 106136. doi:https://doi.org/10.1016/j.asoc.2020.106136.
- Ocampo, L. A., A. M. B. Jumao-as, J. J. T. Labrador, and A. M. O. Rama. 2016. “On a Generic Framework for Sustainable Product Design: An Application to a Food Product.” Journal of Agriculture and Technology Management 19 (1): 9–21.
- Ocampo, L. A., J. J. T. Labrador, A. M. B. Jumao-as, and A. M. O. Rama. 2020. “Integrated Multiphase Sustainable Product Design with a Hybrid Quality Function Deployment–multi-attribute Decision-making (QFD-MADM) Framework.” Sustainable Production and Consumption 24: 62–78. doi:https://doi.org/10.1016/j.spc.2020.06.013.
- Ocampo, L. A., and M. A. B. Promentilla. 2016. “Development of a Sustainable Manufacturing Strategy Using Analytic Network Process.” International Journal of Business and Systems Research 10 (2–4): 262–290. doi:https://doi.org/10.1504/IJBSR.2016.075744.
- Oke, S. A. 2013. “Manufacturing Quality Function Deployment: Literature Review and Future Trends.” Engineering Journal 17 (3): 79–103. doi:https://doi.org/10.4186/ej.2013.17.3.79.
- Onar, S. Ç., G. Büyüközkan, B. Öztayşi, and C. Kahraman. 2016. “A New Hesitant Fuzzy QFD Approach: An Application to Computer Workstation Selection.” Applied Soft Computing 46: 1–16. doi:https://doi.org/10.1016/j.asoc.2016.04.023.
- Pal, D. K., B. Ravi, and L. S. Bhargava. 2007. “Rapid Tooling Route Selection for Metal Casting Using QFD–ANP Methodology.” International Journal of Computer Integrated Manufacturing 20 (4): 338–354. doi:https://doi.org/10.1080/09511920600883229.
- Promentilla, M. A. B., T. Furuichi, K. Ishii, and N. Tanikawa. 2006. “Evaluation of Remedial Countermeasures Using the Analytic Network Process.” Waste Management 26 (12): 1410–1421. doi:https://doi.org/10.1016/j.wasman.2005.11.020.
- Puglieri, F. N., A. Ometto, and P. A. C. Miguel. 2011. “Eco-design Methods for Developing New Products Based on QFD: A Literature Analysis.” Product: Management and Development 9 (1): 23–29. doi:https://doi.org/10.4322/pmd.2011.003.
- Rahimi, M., and M. Weidner. 2002. “Integrating Design for Environment (Dfe) Impact Matrix into Quality Function Deployment (QFD) Process.” The Journal of Sustainable Product Design 2 (1): 29–41. doi:https://doi.org/10.1023/B:JSPD.0000016418.79201.e7.
- Rajesh, G., and P. Malliga. 2013. “Supplier Selection Based on AHP QFD Methodology.” Procedia Engineering 64: 1283–1292. doi:https://doi.org/10.1016/j.proeng.2013.09.209.
- Romli, A., P. Prickett, R. Setchi, and S. Soe. 2015. “Integrated Eco-design Decision-making for Sustainable Product Development.” International Journal of Production Research 53 (2): 549–571. doi:https://doi.org/10.1080/00207543.2014.958593.
- Saaty, T. L. 1980. The Analytic Hierarchy Process. New York, USA: McGraw-Hill.
- Saaty, T. L. 2001. Decision Making with Dependence and Feedback: The Analytic Network Process. 2nd ed. Pittsburg: RWS Publications.
- Sakao, T. 2007. “A QFD-centred Design Methodology for Environmentally Conscious Product Design.” International Journal of Production Research 45 (18–19): 4143–4162. doi:https://doi.org/10.1080/00207540701450179.
- Sakao, T., K. Masui, M. Kobayashi, and A. Inaba. 2002, November. “QFDE (Quality Function Deployment for Environment) and LCA: An Effective Combination of Tools for DfE.” In The Fifth International Conference on EcoBalance, Tsukuba, Japan, 473–476.
- Shahin, A., and P. Nikneshan. 2008. “Integration of CRM and QFD.” The TQM Journal 20 (1): 68–86. doi:https://doi.org/10.1108/09544780810842910.
- Sharma, J. R., A. M. Rawani, and M. Barahate. 2008. “Quality Function Deployment: A Comprehensive Literature Review.” International Journal of Data Analysis Techniques and Strategies 1 (1): 78–103. doi:https://doi.org/10.1504/IJDATS.2008.020024.
- Sheng-Li, S., Y. Xiao-Yue, L. Hu-Chen, and P. Zhang. 2018. “DEMATEL Technique: A Systematic Review of the State-of-the-art Literature on Methodologies and Applications.” Mathematical Problems in Engineering 3696457. doi:https://doi.org/10.1155/2018/3696457.
- Singgih, M. L., D. L. Trenggonowati, and P. D. Karningsih. 2013, August. “Four Phases Quality Function Deployment (QFD) by considering KANO Concept, Time and Manufacturing Cost.” In: Proceedings of The Second International Conference on Engineering and Technology Development (ICETD), Lampung, Indonesia.
- Singh, P. K., and P. Sarkar. 2020. “A Framework Based on Fuzzy Delphi and DEMATEL for Sustainable Product Development: A Case of Indian Automotive Industry.” Journal of Cleaner Production 246: 118991. doi:https://doi.org/10.1016/j.jclepro.2019.118991.
- Sivasamy, K., C. Arumugam, S. R. Devadasan, R. Murugesh, and V. M. M. Thilak. 2016. “Advanced Models of Quality Function Deployment: A Literature Review.” Quality & Quantity 50 (3): 1399–1414. doi:https://doi.org/10.1007/s11135-015-0212-2.
- Soota, T. 2017. “Integrated Approach for Sustainable Product Development Using QFD and ANP.” International Journal of Industrial and Systems Engineering 25 (1): 59–75. doi:https://doi.org/10.1504/IJISE.2017.080688.
- Tao, J., and S. Yu. 2018. “Product Life Cycle Design for Sustainable Value Creation: Methods of Sustainable Product Development in the Context of High Value Engineering.” Procedia CIRP 69: 25–30. doi:https://doi.org/10.1016/j.procir.2017.11.099.
- Tseng, M. L. 2011. “Using a Hybrid MCDM Model to Evaluate Firm Environmental Knowledge Management in Uncertainty.” Applied Soft Computing 11 (1): 1340–1352. doi:https://doi.org/10.1016/j.asoc.2010.04.006.
- Tseng, M. L., Y. H. Lin, A. S. F. Chiu, and J. C. H. Liao. 2008. “Using FANP Approach on Selection of Competitive Priorities Based on Cleaner Production Implementation: A Case Study in PCB Manufacturer, Taiwan.” Clean Technologies and Environmental Policy 10 (1): 17–29. doi:https://doi.org/10.1007/s10098-007-0109-4.
- Tukker, A., and B. Jansen. 2006. “Environmental Impacts of Products: A Detailed Review of Studies.” Journal of Industrial Ecology 10 (3): 159–182. doi:https://doi.org/10.1162/jiec.2006.10.3.159.
- Tzeng, G. H., W. H. Chen, R. Yu, and M. L. Shih. 2010. “Fuzzy Decision Maps: A Generalization of the DEMATEL Methods.” Soft Computing 14 (11): 1141–1150. doi:https://doi.org/10.1007/s00500-009-0507-0.
- United Nations. 2021. “World Food Safety Day 7 June” Accessed 19 February 2021. https://www.un.org/en/observances/food-safety-day
- Van Laarhoven, P. J., and W. Pedrycz. 1983. “A Fuzzy Extension of Saaty’s Priority Theory.” Fuzzy Sets and Systems 11 (1–3): 229–241. doi:https://doi.org/10.1016/S0165-0114(83)80082-7.
- Vinodh, S., and G. Rathod. 2010. “Integration of ECQFD and LCA for Sustainable Product Design.” Journal of Cleaner Production 18 (8): 833–842. doi:https://doi.org/10.1016/j.jclepro.2009.12.024.
- Vinodh, S., and K. Jayakrishna. 2014. “Development of Integrated ECQFD, LCA and Sustainable Analysis Model.” Journal of Engineering, Design and Technology 12 (1): 102–127. doi:https://doi.org/10.1108/JEDT-07-2011-0052.
- Vinodh, S., K. J. Manjunatheshwara, S. K. Sundaram, and V. Kirthivasan. 2017. “Application of Fuzzy Quality Function Deployment for Sustainable Design of Consumer Electronics Products: A Case Study.” Clean Technologies and Environmental Policy 19 (4): 1021–1030. doi:https://doi.org/10.1007/s10098-016-1296-7.
- Vinodh, S., and S. K. Chintha. 2011. “Application of Fuzzy QFD for Enabling Sustainability.” International Journal of Sustainable Engineering 4 (4): 313–322. doi:https://doi.org/10.1080/19397038.2010.536853.
- Wu, S. M., H. C. Liu, and L. E. Wang. 2017. “Hesitant Fuzzy Integrated MCDM Approach for Quality Function Deployment: A Case Study in Electric Vehicle.” International Journal of Production Research 55 (15): 4436–4449. doi:https://doi.org/10.1080/00207543.2016.1259670.
- Wu, Y. H., and C. C. Ho. 2015. “Integration of Green Quality Function Deployment and Fuzzy Theory: A Case Study on Green Mobile Phone Design.” Journal of Cleaner Production 108: 271–280. doi:https://doi.org/10.1016/j.jclepro.2015.09.013.
- Xu, J., X. Xu, and S. Q. Xie. 2010. “A Comprehensive Review on Recent Developments in Quality Function Deployment.” International Journal of Productivity and Quality Management 6 (4): 457–494. doi:https://doi.org/10.1504/IJPQM.2010.035893.
- Xu, R. 2000. “Fuzzy Least-squares Priority Method in the Analytic Hierarchy Process.” Fuzzy Sets and Systems 112 (3): 395–404. doi:https://doi.org/10.1016/S0165-0114(97)00376-X.
- Yan, H. B., and T. Ma. 2015. “A Group Decision-making Approach to Uncertain Quality Function Deployment Based on Fuzzy Preference Relation and Fuzzy Majority.” European Journal of Operational Research 241 (3): 815–829. doi:https://doi.org/10.1016/j.ejor.2014.09.017.
- Yazdani, M., P. Chatterjee, E. K. Zavadskas, and S. H. Zolfani. 2017. “Integrated QFD-MCDM Framework for Green Supplier Selection.” Journal of Cleaner Production 142: 3728–3740. doi:https://doi.org/10.1016/j.jclepro.2016.10.095.
- Yazdani, M., Z. X. Wang, and F. T. Chan. 2020. “A Decision Support Model Based on the Combined Structure of DEMATEL, QFD and Fuzzy Values.” Soft Computing 24 (16): 12449–12468. doi:https://doi.org/10.1007/s00500-020-04685-2.
- Yeh, C. H., J. C. Huang, and C. K. Yu. 2011. “Integration of Four-phase QFD and TRIZ in Product R&D: A Notebook Case Study.” Research in Engineering Design 22 (3): 125–141. doi:https://doi.org/10.1007/s00163-010-0099-9.
- Yim, H. 2003, December. “Eco-voice of Consumer (VOC) on QFD.” In 2003 EcoDesign 3rd International Symposium on Environmentally Conscious Design and Inverse Manufacturing, IEEE, Tokyo, Japan, 618–625.
- Younesi, M., and E. Roghanian. 2015. “A Framework for Sustainable Product Design: A Hybrid Fuzzy Approach Based on Quality Function Deployment for Environment.” Journal of Cleaner Production 108: 385–394. doi:https://doi.org/10.1016/j.jclepro.2015.09.028.
- Zadeh, L. A. 1965. “Fuzzy Sets.” Information and Control 8 (3): 338–353. doi:https://doi.org/10.1016/S0019-9958(65)90241-X.
- Zaim, S., M. Sevkli, H. Camgöz-Akdağ, O. F. Demirel, A. Y. Yayla, and D. Delen. 2014. “Use of ANP Weighted Crisp and Fuzzy QFD for Product Development.” Expert Systems with Applications 41 (9): 4464–4474. doi:https://doi.org/10.1016/j.eswa.2014.01.008.
- Zhang, F., M. Yang, and W. Liu. 2014. “Using Integrated Quality Function Deployment and Theory of Innovation Problem Solving Approach for Ergonomic Product Design.” Computers & Industrial Engineering 76: 60–74. doi:https://doi.org/10.1016/j.cie.2014.07.019.
- Zhang, Y. 1999. “Green QFD-II: A Life Cycle Approach for Environmentally Conscious Manufacturing by Integrating LCA and LCC into QFD Matrices.” International Journal of Production Research 37 (5): 1075–1091. doi:https://doi.org/10.1080/002075499191418.
- Zheng, P., X. Xu, and S. Q. Xie. 2019. “A Weighted Interval Rough Number Based Method to Determine Relative Importance Ratings of Customer Requirements in QFD Product Planning.” Journal of Intelligent Manufacturing 30 (1): 3–16. doi:https://doi.org/10.1007/s10845-016-1224-z.