ABSTRACT
Today, in addition to economic issues, companies face social and environmental challenges in designing their products to stay in a competitive world. One of the essential steps in product sustainability is material selection. Determining the optimal combination of materials to make multi-component products is complicated because there are several material alternatives for each component. In this paper, an integer programming approach is proposed to select materials for products that are composed of many components. In this model, several issues are integrated and solved simultaneously: material selection, supplier selection, manufacturing process selection, assembly process selection, and end-of-life option determination. The objectives of this model are to minimise the producer’s cost and the environmental impact through the entire product life cycle. In order to validate the proposed model, a case study has been conducted in the automotive industry. Finally, by sensitivity analyses, weight reduction on cost and environmental impact has been investigated. The results indicate that lightweight materials are not always the best option in terms of environmental consideration.
1. Introduction
In recent years, there has been an increasing interest for developed and developing nations to achieve sustainable development. ‘Sustainable development is development that meets the needs of the present without compromising the ability of future generations to meet their own needs’ (World Commission on Environment and Development, 1987). Sustainability has three aspects, namely economic, social, and environmental. Designing sustainable products is one of the approaches that companies use to move towards sustainability (Zarandi et al., 2011). Today, in competitive markets, companies in general and automakers in particular have found that they can improve their public image by introducing sustainability issues in their products (Ameli et al., 2016a). One of the most important steps in producing sustainable products is materials selection. Material selection is essentially a multi-objective/multi-criteria decision-making process which should simultaneously take into account the technical, economic, environmental, and social impacts (Hosseinijou, Mansour, and Shirazi Citation2014). During the engineering design process, material selection has a profound impact on the energy consumption and emissions of greenhouse gases (GHG) during the entire product life cycle. The incorrect selection of material(s) will result in increased environmental pollution and energy consumption, and ultimately the market failure of the product. In addition, it will have an adverse effect on the company reputation and interests (Bi et al, Citation2017).
Due to the importance of sustainable production in the automotive industry, this industry has been explored in this paper. A global growth in vehicle production has significantly increased the global fuel demand, material requirements and GHG emissions. Consequently, sustainability has become a critical issue in the automotive industry to significantly reduce the environmental impact of automobiles around the world (Mayyas, 2012). Several stringent laws and regulations have been set up in relation to environmental issues in the automotive industry such as European Union CO2 regulation and End-Of-Life (EOL) vehicle directive. In response to these laws and regulations, automotive manufacturer has begun actions to meet sustainability requirements such as considering the EOL phase during the design process or reducing vehicle weight in order to improve fuel efficiency (Schöggl et al., 2017). However, automakers still face many problems in terms of sustainability issues in their product design.
Sustainable automobile design should be based on a comprehensive approach in order to create a balance between environmental, economic and social dimensions. There are many approaches to assess the environmental impact of the product. Some of the most important example are as follows: ISO 14040 series, ISO/TS 14067: 2013 and Eco-indicator 99 (Ameli et al., 2016b). To calculate the environmental impact, performing a comprehensive life cycle assessment (LCA) is very complex, time-consuming and costly and requires a wide range of data (Mirdar Harijani et al., 2017a; Ameli et al., 2016b). The carbon footprint (CF) is used as a measure for assessing the environmental impact of a product by many researchers (Le and Lee, 2013; Su et al., 2012; Song and Lee, 2010) which assesses a product’s global warming potentials due to its GHG emissions. Therefore, in this research, the CF is used as an indicator for calculating the environmental impact. However, other alternative indicators can also be used.
Considering only the use phase for material selection at the design stage is very unrealistic. According to Geyer (2007), reduction in GHG emission in one phase only (such as the use phase) may lead to an increase in GHG emission in other phases. This emphasises the importance of a holistic approach such as LCA.
Although a large portion of the GHG emissions (about 70–90%, as shown in ) over the entire life cycle of a vehicle is related to the use phase, it does not mean that other phases of the vehicle life cycle are free from any emissions (Geyer, 2007). Changes in vehicle design usually reduce GHG emissions in many stages of the vehicle life cycle, and any change in other phases can affect the use phase and reduce the amount of emissions at this stage (Geyer, 2007).
Material selection in vehicle design potentially affects GHG emissions at all stages of the life cycle and vice versa, which means that the choice of different alternatives in each phase affects the type of selected materials (see ) (Geyer, 2007).
Figure 2. The impact of materials selection on GHG emissions throughout the product life cycle (Geyer, 2007).
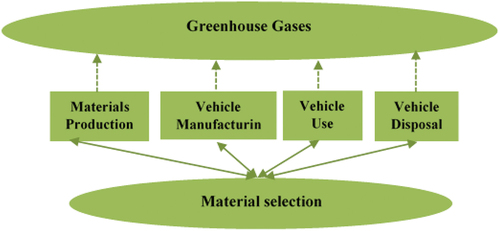
Recently, researchers have shown increased interests on the concept of multi-material lightweight vehicles, by which the best materials for a special auto-body component are selected in order to fulfil performances and minimise the overall weight (Goede et al. Citation2009). In addition, environmental and economic issues should be considered simultaneously in this selection. Determining the optimal combination of materials to make auto parts is very important. As each product consists of many components and there are different material alternatives for each component, therefore there are many potential solutions for selection of optimal material(s). This places important emphasis on the design stage of a product life cycle (Bi et al, Citation2017). Different alternatives in different stages need to be addressed simultaneously for multi-material selection, which makes this problem extremely complex. When a sustainable material is being selected for a product, it is essential to select supplier, manufacturing and assembly process and EOL options at the same time. Consider a product that contains ‘n’ components, each component has ‘m’ material alternatives, for each material there are ‘s’ supplier, ‘t’ manufacturing process, ‘a’ assembly process, and finally for each of these material alternatives ‘o’ EOL options are available, so mn×sn×tn×an×on solutions are feasible. Therefore, the cost estimation and environmental impact assessment are very time-consuming and difficult, and it is impossible to handle these issues by considering these volumes of design alternatives to achieve optimal configuration.
In this paper, a multi-objective optimisation model is developed in order to select the optimal combination of materials for automobile industry in the shortest possible time. The objectives are the minimisation of the environmental impact (the GHG emissions) and the producer’s cost through the products’ entire life cycle. This model can be used for simultaneous selection of different issues, namely material alternative, supplier, manufacturing and assembling process and EOL option determination of each part within a product.
The rest of this paper is organised as follow: in section 2, the literature review and motivation for this research are discussed. In section 3, the developed multi-objective model is introduced. A case study is presented in section 4, and the results are explained in section 5. The sensitivity analysis is reported in section 6 and the conclusion is presented in the last section.
2. Literature review
In order to improve the product sustainability aspects, a number of methods, tools, and strategies for sustainable material selection have been developed by various researchers. By reviewing the literature, it could be concluded that researches could be classified in three categories, namely (see ): 1. Life-cycle analysis methods, 2. Multi-criteria decision making (MCDM) approaches and 3. Multi-objective decision making (MODM).
Life-cycle analysis methods Mayyas et al. (Citation2012) developed a comprehensive LCA framework to select sustainable materials for automobile Body-In-White (BIW) (see for an introduction to BIW). In their paper, the LCA has been used as a basic approach to assess the performance of BIW throughout its lifecycle. Tharumarajah and Koltun (Citation2010) examined the environmental impacts of using magnesium in the manufacturing of automobile instrument panel. By using the LCA tool, the GHG impacts of instrument panel made of steel, plastics, bio-plastic and magnesium were estimated and compared over the entire life cycle. Muñoz et al. (Citation2006) used the LCA approach to compare the environmental impacts and EOL options of automobile door panels that are currently made of polymers with a sample designed for recycling based on polyolefin. Witik et al. (Citation2011) used LCA and Life Cycle Costing (LCC) to compare lightweight composites with steel and magnesium to manufacture automobile components. The results showed that lighter materials are not always the best option from an environmental perspective. Stoffels et al. (Citation2018) consider sustainable product design as a multi-dimensional project. They stated that the product, manufacturing process, and material should be considered simultaneously. They developed a software based on life cycle assessment, databases, and another qualitative measurement for selecting the different combinations for solving the issue.
The LCA needs data for analysis, including the amount of energy consumption and GHG emissions throughout the entire life cycle from material extraction to the end of life. Therefore, the implementation of LCA due to the complexity and diversity in the time frame and the high number of inputs and outputs to the system, is a very costly, time-consuming and difficult task (Ameli et al., 2016b).
Multi-criteria decision-making methods A variety of methods were developed to apply sustainability considerations with a lifecycle approach for choosing sustainable materials of automobile BIW. These models that were adopted in a number of papers are as follows: fuzzy TOPSIS, Analytic Hierarchy Process (AHP), and clustering methods (Mayyas, 2012; Mayyas, Omar, and Hayajneh Citation2016, 2011). Govindan, Madan Shankar, and Kannan (Citation2015) proposed a MCDM approach for selecting sustainable materials in the construction industry. They simultaneously considered all three environmental, economic and social dimensions. Their proposed model includes the integrated mixing method Dematel, Analytical Network Process (ANP) and TOPSIS. Jayakrishna et al. (Citation2016) considered two issues simultaneously: material selection and the choice of EOL options by using the grey-relational-analysis method. The method chooses the optimal option in terms of economic, technical, and environmental aspects. Girubha and Vinodh (Citation2012) used the fuzzy VIKOR model, along with LCA, for selecting sustainable materials in vehicle instrument panel. Hosseinijou, Mansour, and Shirazi (Citation2014) provided a method for evaluating the social impacts of material selection, and used AHP for social life cycle impact assessment. They investigated the social performance of cement and steel as building materials. The main goal in their study was to identify the strengths and weaknesses of these two materials in terms of social sustainability and provide suggestions for improving them. They proposed a methodology based on Social Life Cycle Assessment (SLCA) to assess important social aspects.
Mathematical programming methods Few articles have used mathematical programming techniques for selecting sustainable materials. Zhou et al. presented a multi-objective decision method with a combination of genetic algorithms and artificial neural networks to select materials for design of sustainable products (Zhou, Yin, and Hu Citation2009). Sakundarini et al. (2013) introduced a genetic algorithm to solve the developed multi-objective model for the selection of recyclable materials. In order to show the applicability of the developed multi-objective model, it was used on an automobile’s side mirror. Mohan et al. (2016) presented a new methodology using the multi-objective optimisation and Design of Experiment (DOE) technique to achieve an optimal material selection for the BIW components. Tao et al. (Citation2016) presented a comprehensive optimisation model for selecting green materials with energy considerations. A dual model was proposed for products with several components. A combination optimisation approach called chaos quantum group leader algorithm (CQGLA) was proposed to select the optimal mix of materials with the least energy consumption while meeting the expected quality and cost. Bi et al. (Citation2017) proposed a comprehensive multi-objective optimisation model for automatically selecting multi materials based on cloud computing. They used the local search-differential group leader algorithm (LS-DGLA), to solve the proposed model. Zhou, Xiahou, and Liu (Citation2021) developed a multi-objective optimisation model for designing sustainable products under uncertainty, and for obtaining the optimal design solution, they use a new Topsis method.
2.1. Research gap analysis
A summary of the reviewed literature on the selection of sustainable materials is presented in . Then the research gap is identified, and the novelty of this research is presented. Based on the literature review, the following results were obtained
Most articles focus on one phase of product life cycle (mostly use or production) in order to select and design sustainable materials. However, there are environmental and economic impacts throughout the entire life cycle of a product. For choosing the appropriate material, the effects of other phases such as: selection of the right supplier, the production process or the EOL options, which directly affect the type of selected material(s), have not been considered so far.
Due to the importance of product EOL, material selection and EOL option should be considered simultaneously. Considering the EOL decisions in the material selection phase will make recycling easier and less costly (Ameli et al., 2016a). Literature search indicates that few articles have been devoted to this issue.
Most articles have focused on material selection for a particular component, and the choice of material for a complex product consisting of a large number of parts (such as an automobile) has not been considered.
Table 1. Summary of the literature review.
3. Model formulation
3.1. The main concepts of the model
Each product has a life cycle consisting of four distinct phases, with each phase having different subsections (Keoleian and Sullivan, 2012):
Simultaneous consideration of all stages of the vehicle’s lifecycle for the selection of sustainable materials is essential. In each stage, several factors strongly influence the type of material selection. The factors considered in each of the above-mentioned phases are as follows: suppliers, manufacturing, assembly processes and EOL options, respectively.
demonstrates the relationship between alternatives of materials, suppliers, manufacturing/assembly processes and EOL options for one part within a product for the developed model. It is assumed that the new product consists of n parts, which is indicated by index i. In , the selection of alternatives for part 1 is shown as an example. Then, the appropriate material should be selected for each part. The number of material alternatives for each part is mi, which is indicated by index j. At the early stage of the design process, the selection of appropriate materials should be based on simultaneous consideration for the choice of suppliers, manufacturing/assembly processes and product EOL options. This ensures that the most suitable material(s) is selected from the economic and environmental perspective. The optimal alternative selection for each part will be extremely time-consuming and difficult.
Figure 4. Relationship between different alternatives to design new product development in the proposed model.
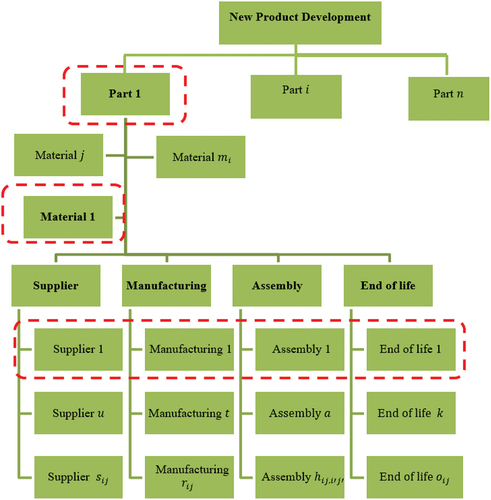
In this study, a mathematical model was developed that helps designers to select the optimal combination of alternatives in the shortest possible time. The schematic structure of the proposed model is shown in .
3.2 The Mathematical modelling
Based on the description given in the previous section, the variables and parameters of the proposed model are presented in , followed by the objective functions and associated constraints.
Table 2. Symbols used in the mathematical model.
3.2.1. The objective functions
The general form of the economic and environmental objective functions are in accordance with Equationequations (1)(1) and (Equation2
(2) ).
Each product has a life cycle of various stages. In order to achieve the total cost and environmental impact for the entire product life cycle, the cost and environmental impact of each stage must be calculated separately and then added together. Details of how to calculate the cost and environmental impact at each phase are given below. Initially, the equation to calculate the costs is given, and then the method to obtain the environmental impact is explained.
Cost equations
C1: The cost of first stage (materials supply)
The first stage is related to supplying materials from different suppliers. The decision variable at this stage is the selection of a suitable supplier. For Original Equipment Manufacturer (OEMs), supplier’s location is very important for selecting materials. Therefore, the cost at this stage is obtained by Equationequation (3)(3) .
C2: The cost of second stage (manufacturing/assembly process)
EquationEquation (4)(4) represents the costs of second phase. The first section is the manufacturing cost, and the last section is the assembly cost.
Since the cost of assembling part i into part is equal to the cost of assembling part
into part i (
), so the value of
is from i + 1 to n.
C3: The cost of third stage (use, maintenance and repairs)
Since the components are not used alone and are assembled together and then the entire vehicle is used, the costs associated with the use phase should be examined at the product level. In order to assess the costs and environmental impact of this phase, first the vehicle fuel consumption should be determined. Fuel consumption has a direct correlation with lifetime span and vehicle weight and is calculated according to Equationequation (5)(5) .
in which, ε is fuel economy. Its value for the vehicle examined in this paper is 0.15 litre/ (100 kg × 100 km) (Koffler and Rohde-Brandenburger, 2010), which indicates that a product with a weight of 100 kg over a distance of 100 km consumes 0.15 litre of fuel. Therefore, the vehicle use cost is equal to .
For calculating the maintenance and repair costs, it is assumed that the defective component is replaced with a new one. Therefore, the cost of maintenance and repairs includes the total costs of material supply, manufacturing process, replacement and EOL. Finally, Equationequation (6)(6) shows the costs of this stage.
in which is the frequency of maintenance and repair of part i made of material j during the entire of the vehicle life cycle.
C4: The cost of fourth stage (End of life)
The final phase is the management of product EOL which is the most important phase for preventing environmental pollution (Ameli et al., 2016b). In order to reach the EOL, the entire product should be disassembled at the outset. The EOL options investigated in this study are reuse, remanufacture, recycle and disposal. Product disassembly and EOL costs are calculated by Equationequation (7)(7) .
As Equationequation (1)(1) shows, by summation of the C1, C2, C3 and C4, the economic objective function is obtained that should be minimised.
Environmental impact equations
The environmental objective function is calculated based on the approach that was adopted to obtain the economic objective function. EquationEquation (8)(8) calculates the environmental impact of producing and transporting the raw materials. EquationEquation (9)
(9) determines the environmental impact of manufacturing and assembly of parts. The environmental impact of use, maintenance and repair phase is computed using Equationequation (10)
(10) . EquationEquation (11)
(11) calculates the environmental impact of EOL management.
As shown in Equationequation (2)(2) , by summation of the E1, E2, E3 and E4, the environmental objective function is obtained that should be minimised.
3.2.2. The constraints
The relationships among the variables and parameters are defined via developing several equality and inequality constraints.
Constraint (12) is related to component performance, where the level of component performance should not be less than the specified value. The performance of each component is usually expressed qualitatively and its evaluation is based on the opinion of the experts. Constraint (13) is related to the total product performance. The performance of each part is multiplied by its importance, and the total product function is obtained.
EquationEquation (14)(14) controls that the total recycling rate should not be less than the minimum acceptable recycling rate. In order to calculate the recycling rate of the product, first the total weight of the recycled materials should be calculated by Equationequation (24)
(24) . Then, the total recycling rate is determined by Equationequation (25)
(25) .
is the minimum acceptable recycling rate.
Constraints 15 to 19 ensure that each selected component is made up of only one component and has one supplier, one production/assembly process and only one EOL option. Since constraint 18 is non-linear, Equationequations 26(26) to 30 convert it to a linear one.
Constraint (20) states that if a particular material is selected for a part, the same material must be selected for a number of other components. The constraint (21) states that if a particular material is selected for a single part, that material should not be selected for a number of other components. Constraint (22) controls the maximum total weight of the product, and constraint (23) assures that the corresponding variables are binary.
4. The case study
After considering different products in terms of the variety and importance of material selection, the automobile BIW in general and components such as chassis, closure, and bumper, in particular, were selected for the case study. The reason for this selection is that a large percentage of the vehicle weight is allocated to the automobile’s body. Approximately of the car’s total weight (see ) is related to BIW and other closures (Lutsey Citation2010). By improving the material of these parts in terms of sustainability, we can ensure that a large part of our designed car complies with sustainability conditions. Because of this, most manufacturers have been focusing on these components.
Figure 6. Vehicle mass breakdown by system and components (A. Mayyas et al. Citation2012).
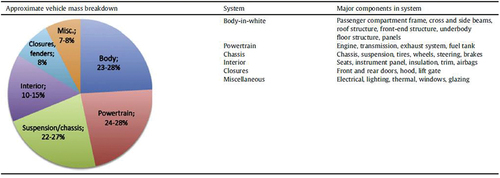
In this section, an example is presented that has been extracted from Iran Khodro Automobile Company, and in particular, its parts manufacturer, Sapco Company, was considered. Iran-Khodro is the largest Iranian automaker. To compete in the market and export, Iran-Khodro must design its products with economic and environmental dimensions. It is common in the automobile industry to reduce the cost and time of developing a new product using existing platforms.
In this research, four general categories of automotive parts have been investigated: The body of the car (includes 50 components), closures (including 16 main panels), chassis (including 4 components) and bumper (1 component), in total 70 components have been investigated.
As discussed in the previous section, different variables should be considered simultaneously for selecting the right and sustainable materials. These variables are material, supplier, manufacturing and assembly process, and end of life solution. In , different alternatives considered in this case study are shown.
Material alternatives for these components in this study are classified into six general categories: steels, advanced high strength steels (AHSS), stainless steel, aluminium, magnesium, and composites such as carbon fibre-reinforced plastics (CFRP) and glass fibre-reinforced plastics (GFRP). Choosing the right material for an engineer can be very difficult, especially if the material is used for the first time in the automobile body. For this reason, a methodology was developed in this paper which supports the engineer in the early and most important design phase for material selection.
For sustainable material selection, in addition to making trade-offs between economic and environmental issues, technical requirements for each component should be identified and ranked. In the proposed mathematical model, the technical criteria are considered as constraints, which were explained in the previous section. In , some of the most important criteria used in this paper are shown. The weight of the technical criteria and the score of each sub-function are extracted from (Goede et al. Citation2009; A. Mayyas et al. Citation2012; A. T.Citation2012; Mayyas, Omar, and Hayajneh Citation2016) references.
After determining the different criteria and alternatives for each component, the required information is compiled from the Iranian automobile industry. This information includes the cost of purchasing and shipping from different suppliers, the cost of manufacturing and assembly using different types of processes, the cost of end-of-life operations, the distance of suppliers from component makers, and the weight of each component.
The environmental impact of all of the above alternatives must also be calculated. In order to calculate the CF values, Gabi softwareFootnote1 was used. To illustrate the data collection procedure, provides examples for obtaining the required information. This figure presents the information compiled for different design alternatives for the new automobile door. The information in this figure was obtained using interviews with Sapco experts and Gabi software. illustrates the life cycle inventory (LCI) modelling of the automobile door made of steel in GaBi. A similar analysis for each design alternative of the new car should be conducted.
5. Results
The developed model is a multi-objective linear model. By increasing the number of components, which increases the alternatives of the materials, the complexity of the model increases exponentially. Assume a product that contains only five components and for each component there are two material alternatives, each of the material alternatives has at least two suppliers, for each material, at least two different methods of production and assembly are applicable, and finally each of these material alternatives has four EOL options. Therefore, there will be or
alternatives to select. Adding only one component will increase the number of alternatives to
. Therefore, it is obvious that the selection of best configuration is extremely challenging and even impossible, while the developed model provides the automakers with a tool to select the optimal alternatives in the shortest possible time.
There is no optimal answer to multi-objective problems, but a set of effective (or optimal) pareto solutions for these issues is achievable. A multi-objective decision problem (MODM) is introduced as follow (Steuer and Piercy Citation2005):
represents the number of goals.
is an effective point, If and only if there are no points like
, that
. In fact, at least for one
(Steuer and Piercy Citation2005).
One of the accurate methods for solving multi-objective optimisation problems is the individual optimisation method. In this method, the answer to each single-objective problem is considered an effective answer for the multi-objective problem. In other words, the model is solved each time by considering one of the objectives and the ideal answers obtained from each objective form effective answers. If all the ideal answers are equal, that answer will be the best answer to the multi-objective problem (Diwekar Citation2020). Finally, after solving each of the models separately, the Epsilon constraint method was used in order to obtain the optimal Pareto solutions. In this method, assuming that all objectives are maximum type, the objective function with higher priority is considered as the main objective function of the single-objective problem and for other objective functions, a lower limit is considered. This lower limit should naturally be smaller than the ideal answer to those objectives. If the objectives in the problem are of the minimal type, a high limit should be considered for them(Ehrgott and Ruzika Citation2008).
First, the environmental impact and the cost of an existing vehicle manufactured by an Iranian automotive company were investigated. The cost of the base sample is $ and its carbon footprint, is
. If only the cost function is considered for optimisation in this model, then the values of $
and
kg are obtained for the cost and environmental functions, respectively. For the model, only with the environmental objective function, the values of the cost and environmental functions are $
and
kg, respectively.
In order to obtain Pareto optimal solutions, the environmental objective function was considered as a constraint in the cost function, and the model was run for times.
Pareto’s solutions were determined using the GAMS v 24.1.3 (see reference (Kucukkoc et al., Citation2015) for an introduction to GAMS software) and solved using CPLEX 12.1. The results are shown in and .
Table 3. Results of the model implementation.
This chart (see ) can be used at design phase of a new car in order to balance the cost and CF. By reducing the environmental impact, the cost increases. In this diagram, two distinct sections are recognisable ( and
). In section
, costs are very sensitive to environmental impact, and a slight reduction in the environmental impact drastically increases costs, so these areas are not very attractive to automakers, as a negligible reduction in environmental impact incurs significant cost. But the
-segment is important for automakers as reduction in CF has no significant impact on costs and designers can select materials within this range without concern relating to increases in environmental impact.
The percentage of alternative distributions including material, manufacturing process, assembly process and EOL option that is eventually used in section, the area that is attractive to automakers in pareto optimal solution, is shown in . The final selected suppliers are not displayed due to confidentiality.
Figure 12. The Percentage of material distribution at optimal points (Material- manufacturing process- Assembly process- End of life).
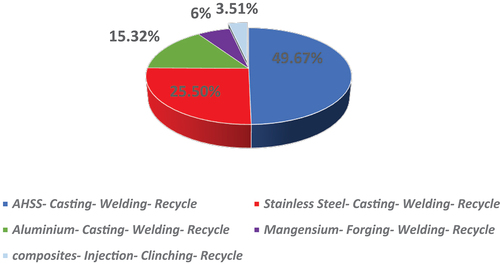
As shown in , AHSS has a higher percentage than other materials. AHSS is growing, only partially adopted, and more is coming. AHSS is a cost-effective solution and is well adapted to the existing production processes. Due to the high cost of switching production processes, the current production process casting and welding has been selected as the optimal ones. Since a case study has been conducted in the Iranian automotive industry, the recycling end-of-life option has been the best. The other two options (Reuse and Remanufacture) will lead to high costs due to the country’s lack of proper infrastructure. Furthermore, the disposal EOL option will also result in very high environmental impacts. Therefore, AHSS has economic and environmental advantages against competing materials. We also emphasise that this result was for a case study in a specific country, and the results may vary depending on different circumstances. However, the proposed model can be used for different cases, and the results are acceptable.
According to , if each stage of the product life cycle is considered individually, the percentage of selected materials in each phase will be different. For example, if only the use phase is considered, the lightest material will be selected, while this light-weight material is not suitable for end-of-life options; for other steps, this is similar. Therefore, all steps should be considered simultaneously for the purpose of selecting sustainable material.
6. Sensitivity analysis
In order to analyse the effect of weight on economic and environmental objectives, the upper bound of weight has been changed from to
, and the optimal values of costs and environmental impacts are compared in and .
Table 4. Sensitivity analysis on vehicle weight.
As shown in , the environmental impact has decreased by reducing the weight, but the cost has increased. Based on this analysis, decision-makers can change the weight of an automobile’s body in relation to the total cost and environmental impact of the product. One of the important factors for the automotive industry is to use light materials to reduce the environmental impact of their products. But in this project only a weight reduction is considered, as this amount of reduction can reduce the environmental impact of the product. Excessive weight reduction can have the opposite effect and increase environmental impacts due to the non-recyclability of lightweight materials. Indeed, the use of lightweight materials in the automobile BIW reduces safety and contrary to technical limitations in the model, so the excessive weight reduction is not allowed. Accordingly, the use of different materials combined in the automobile body to reduce
of the weight reduces the environmental impact and fulfil the expected performance.
7. Conclusion
In this paper, a mathematical model was developed that optimised the selection of materials from number of alternatives for products that are composed of large number of components. Many issues such as selection of materials, suppliers, manufacturing and assembly processes as well as EOL options were considered simultaneously. The objective of the proposed model was to minimise costs and environmental impact, namely carbon footprint during product’s entire life cycle.
A case study in the automotive industry was conducted to validate the developed integer programming model. A total of 70 components consisting of 50, 16 and 4 for body panels, closures and chassis, respectively, plus a bumper were investigated.
Pareto’s solution using GAMS software was determined and is presented in and the graph of . In the sections of the graph represented by ‘α’, any reduction in CF by selecting different materials resulted in significant increase in costs. The section of the graph is represented by ‘β’, ranging from 471,146 to 49,246 kg CO2e, any reduction in environmental impact does not greatly influence the materials’ costs, and hence this section could be of interest to designers.
represents the percentage of material distribution with EOL options in mind and indicates that AHSS is the most suitable material for conducted case study. The results of show that for various phases of product life cycle, investigated materials perform differently but for the entire life cycle, AHSS performs more favourably.
A sensitivity analysis was also conducted to investigate the effect of body panel weight reduction on costs and CF. The body panel weight of the conducted case study was about 500 kg, and reduction in weight of about 30% was investigated. As expected, the reduction in weight resulted in an increase in costs while reducing the resultant environmental impact
Research innovations
The concept of sustainable development has attracted the attention of researchers for decades. Moving towards sustainable production and consumption is one of the concepts of sustainable development that requires a quantitative tool for measuring and decision making. In order to achieve these goals, it is essential to review the methods of selecting materials. The innovations of this research in material selection with a sustainable development approach are:
Integrated EOL considerations into material selection decisions
Consider supplier, manufacturing, assembly process and EOL options variables during the process of optimal sustainable material selection.
Consideration of legal requirements in sustainable material selection
Consideration of technical requirements in sustainable material selection.
A recommended future direction is to consider the social sustainability indicators in the process of selecting sustainable materials and providing a method for their evaluation. Another interesting future research area is in modelling; considering uncertainty in the proposed mathematical model, stochastic programming can be used at the appropriate stages in the process. Considering the desirability for objective functions versus optimising the model, it can create another kind of modelling and problem-solving methods in this research field. For example, goal programming method can be used.
Supplemental Material
Download ()Disclosure statement
No potential conflict of interest was reported by the author(s).
Supplementary material
Supplemental data for this article can be accessed online at https://doi.org/10.1080/19397038.2022.2080888
Notes
References
- Bi, L., Y. Zuo, F. Tao, et al. 2017. “Energy-Aware Material Selection for Product with Multicomponent under Cloud Environment.” Journal of Computing and Information Science in Engineering 17 (3): 31007. doi:10.1115/1.4035675.
- Diwekar, U. M. 2020. Introduction to Applied Optimization. Cham: Springer International Publishing (Springer Optimization and Its Applications). doi:10.1007/978-3-030-55404-0.
- Ehrgott, M., and S. Ruzika. 2008. “Improved ε-constraint Method for Multiobjective Programming.” Journal of Optimization Theory and Applications 138 (3): 375–396. doi:10.1007/s10957-008-9394-2.
- Girubha, R. J., and S. Vinodh. 2012. “Application of Fuzzy VIKOR and Environmental Impact Analysis for Material Selection of an Automotive Component.” Journal Of Materials&Design. Elsevier Ltd 37: 478–486. doi:10.1016/j.matdes.2012.01.022.
- Goede, M., M. Stehlin, L. Rafflenbeul, et al. 2009. “Super Light Car-lightweight Construction Thanks to a multi-material Design and Function Integration.” European Transport Research Review 1 (1): 5–10. doi:10.1007/s12544-008-0001-2.
- Govindan, K., K. Madan Shankar, and D. Kannan. 2015. “Sustainable Material Selection for Construction Industry - A Hybrid Multi Criteria Decision Making Approach.” Renewable and Sustainable Energy Reviews 55: 1274–1288. doi:10.1016/j.rser.2015.07.100.
- Hosseinijou, S. A., S. Mansour, and M. A. Shirazi. 2014. “Social Life Cycle Assessment for Material Selection: A Case Study of Building Materials.” International Journal of Life Cycle Assessment 19 (3): 620–645. doi:10.1007/s11367-013-0658-1.
- Jayakrishna, K., S. Vinodh, V. Sakthi Sanghvi, et al. 2016. “Application of GRA for Sustainable Material Selection and Evaluation Using LCA.” Journal of the Institution of Engineers (India): Series C. Springer India 97 (3): 309. doi:10.1007/s40032-016-0283-4.
- Kucukkoc, I. 2015. “A Mathematical Model and Artificial Bee Colony Algorithm for the Lexicographic Bottleneck mixed-model Assembly Line Balancing Problem.” In Journal of Intelligent Manufacturing, 1–13. Springer.
- Lutsey, N. 2010. “Review of Technical Literature and Trends Related to Automobile mass-reduction Technology.” Institute of Transportation Studies 1 (3): 1–40. Available at: https://escholarship.org/uc/item/9t04t94w
- Mayyas, A. 2012a. Expert System Based Approach for Body-in-White Structural Panels Using Numerical Ranking and Sustainability Indices. Clemson University.
- Mayyas, A., M. A. Omar, and M. T. Hayajneh. 2016. “Eco-material Selection Using Fuzzy TOPSIS Method.” International Journal of Sustainable Engineering 9 (5): 292–304. doi:10.1080/19397038.2016.1153168.
- Mayyas, A., A. Qattawi, M. Omar, et al. 2012. “Design for Sustainability in Automotive Industry: A Comprehensive Review.” Renewable and Sustainable Energy Reviews 16 (4): 1845–1862. DOI:10.1016/j.rser.2012.01.012.
- Mayyas, A., Q. Shen, A. Mayyas, et al. 2011. “Using Quality Function Deployment and Analytical Hierarchy Process for Material Selection of Body-In-White.” Materials & Design. Elsevier Ltd 32 (5): 2771–2782. doi:10.1016/j.matdes.2011.01.001.
- Mayyas, A. T. 2012b. “Life Cycle assessment-based Selection for a Sustainable Lightweight body-in-white Design.” Energy Elsevier 39 (1): 412–425. doi:10.1016/j.energy.2011.12.033.
- Muñoz, I., J. Rieradevall, X. Domènech, et al. 2006. “Using LCA to Assess Eco-design in the Automotive Sector Case Study of a Polyolefinic Door Panel.” The International Journal of Life Cycle Assessment. Ecomed 11 (5): 323–334. doi:10.1065/lca2005.05.207.
- Steuer, R. E., and C. A. Piercy. 2005. “A Regression Study of the Number of Efficient Extreme Points in Multiple Objective Linear Programming.” European Journal of Operational Research 162 (2): 484–496. doi:10.1016/j.ejor.2003.09.014.
- Stoffels, P., J. Kaspar, D. Bähre, et al. 2018. “Integrated Product and Production Engineering Approach - A Tool-Based Method for A Holistic Sustainable Design, Process and Material Selection.” Procedia Manufacturing. Elsevier B.V 21:790–797. doi:10.1016/j.promfg.2018.02.185.
- Tao, F., L. N. Bi, Y. Zuo, et al. 2016. “A Hybrid Group Leader Algorithm for Green Material Selection with Energy Consideration in Product Design.” CIRP Annals - Manufacturing Technology 65 (1): 9–12. doi:10.1016/j.cirp.2016.04.086.
- Tharumarajah, A., and P. Koltun. 2010. “Improving Environmental Performance of Magnesium Instrument Panels.” Resources, Conservation and Recycling. Elsevier B.V 54 (12): 1189–1195. doi:10.1016/j.resconrec.2010.03.014.
- Witik, R. A., J. Payet, V. Michaud, et al. 2011. “Assessing the Life Cycle Costs and Environmental Performance of Lightweight Materials in Automobile Applications.” Composites Part A: Applied Science and Manufacturing. Elsevier Ltd 42 (11): 1694–1709. doi:10.1016/j.compositesa.2011.07.024.
- Zhou, -C.-C., G.-F. Yin, and X.-B. Hu. 2009. “Multi-objective Optimization of Material Selection for Sustainable Products: Artificial Neural Networks and Genetic Algorithm Approach.” Materials & Design. Elsevier Ltd 30 (4): 1209–1215. doi:10.1016/j.matdes.2008.06.006.
- Zhou, J., T. Xiahou, and Y. Liu. 2021. “Multi-objective optimization-based TOPSIS Method for Sustainable Product Design under Epistemic Uncertainty.” Applied Soft Computing. Elsevier B.V 98: 106850. doi:10.1016/j.asoc.2020.106850.