ABSTRACT
There is intense and widespread interest in developing monoclonal antibodies as therapeutic agents to treat diverse human disorders. During early-stage antibody discovery, hundreds to thousands of lead candidates are identified, and those that lack optimal physical and chemical properties must be deselected as early as possible to avoid problems later in drug development. It is particularly challenging to characterize such properties for large numbers of candidates with the low antibody quantities, concentrations, and purities that are available at the discovery stage, and to predict concentrated antibody properties (e.g., solubility, viscosity) required for efficient formulation, delivery, and efficacy. Here we review key recent advances in developing and implementing high-throughput methods for identifying antibodies with desirable in vitro and in vivo properties, including favorable antibody stability, specificity, solubility, pharmacokinetics, and immunogenicity profiles, that together encompass overall drug developability. In particular, we highlight impressive recent progress in developing computational methods for improving rational antibody design and prediction of drug-like behaviors that hold great promise for reducing the amount of required experimentation. We also discuss outstanding challenges that will need to be addressed in the future to fully realize the great potential of using such analysis for minimizing development times and improving the success rate of antibody candidates in the clinic.
Introduction
While antibodies have long been considered to be promising therapeutics, this promise has only been realized in the clinic in the past few decades.Citation1–5 During this time, monoclonal antibodies (mAbs) have garnered widespread attention as therapeutics due to their many favorable properties that confer high bioactivity via their high affinities, specificities and, in some cases, potent effector functions. In the clinic, mAbs have shown efficacy for neutralizing pathogens, inactivating endogenous molecules, and modulating the immune system. mAbs have also succeeded as therapeutics because of their favorable safety profiles, including low immunogenicity for human or humanized antibodies as well as low toxicities.Citation6,Citation7 Another key reason for the success of antibody therapeutics is their favorable developability properties, including typically high solubilities, low aggregation propensities, low viscosities, and slow clearance rates.Citation8,Citation9
Nevertheless, most antibody lead candidates identified using either in vivo or in vitro discovery methods are not immediately suitable for therapeutic use and require additional protein engineering to increase affinity or specificity, reduce immunogenicity, and/or reduce physical and chemical liabilities.Citation10,Citation11 This optimization process is difficult and expensive, and it is complicated by common trade-offs between important properties due to their highly interrelated nature.Citation12,Citation13 One particularly notable challenge that further complicates this process is the inability to measure many key antibody developability properties until later stages of the development process when sufficient amounts of high-purity antibody are available for evaluation. This delayed diagnosis of issues related to antibody developability greatly reduces the success and throughput of generating drug-like antibodies with increased likelihood of success in the clinic.
Therefore, there is substantial interest in developing methods to predict or measure antibody developability properties much earlier in the development process, and particularly during the discovery stage (). This review focuses on advances in this area, prioritizing advances in computational prediction and rational design of antibodies and antibody mutants with drug-like properties, as well as the high-throughput experimental identification of antibodies with such properties. We focus on the latest advances and outstanding challenges related to predicting and screening antibody properties that are of particular importance to the generation of drug-like antibodies, including antibody folding stability, self-association, off-target binding, solubility, aggregation, pharmacokinetics (PK), and immunogenicity.
Figure 1. The development process for antibody therapeutics is being improved by early assessment of antibody developability using emerging high-throughput experimental and computational methods. The development pipeline for antibody therapeutics is limited by the difficulty in assessing key biophysical properties at the antibody discovery and protein engineering stages to identify drug-like antibodies with increased likelihood of success in the clinic. These experimental limitations stem from extremely limited quantities of high-quality (purified) protein available for analysis, especially at the stage of antibody discovery. This has motivated recent advances in high-throughput experimental methods that are compatible with extremely dilute antibody solutions, as well as computational methods for evaluating antibody properties and designing antibody variants with improved properties. These advances stand to substantially improve the development of efficacious therapeutic antibodies. Each developability property is highlighted at one or more stages at which it is typically evaluated
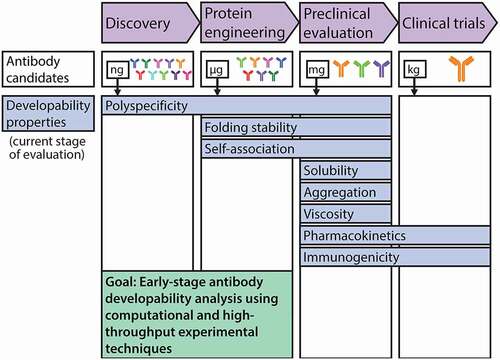
Folding stability
High conformational (folding) stability is vital for antibody therapeutics to maintain their conformational integrity during diverse manufacturing, formulation, and physiological conditions.Citation14–16 Antibody unfolding can result in the loss of protein activity and formation of protein aggregates that can be immunogenic or even toxic.Citation17,Citation18 In general, antibodies are highly stable, and there are well-established experimental methods for high-throughput screening and selection of stable drug candidates during early stage evaluation of large panels or libraries of natural and synthetic antibodies.Citation19,Citation20 However, these initial lead candidates often require further optimization of other properties such as affinity or immunogenicity, which can result in a significant loss of folding stability due to the intrinsic trade-offs between such biophysical properties.Citation12,Citation13 Conventional trial-and-error approaches for re-engineering unstable antibodies often have low success rates and require multiple rounds of the time- and resource-intensive production, purification, and experimental evaluation of soluble protein variants. Therefore, the development of novel methodologies for the rational design of stable antibodies is vital, especially for affinity maturation and humanization applications given the potential of these methods to promote antibody destabilization.
Conventional strategies for affinity maturation have focused primarily on the mutation of surface residues, often those within or adjacent to the antigen-binding site.Citation21–23 However, a recent study demonstrated the utility of mutagenizing sites in the VH/VL interface for improving antibody affinity and stability.Citation24 The study identified a cluster of eight residues at the VH/VL interface of an anti-lysozyme single-chain variable fragment (scFv) that were tolerant to mutations via a deep mutational scan. The VH/VL interfacial area is known to mediate antibody assembly and can have a large impact on the flexibility of the variable region, conformation of the binding loops, and packing efficiency of the two domains. Therefore, it was hypothesized that optimization of the interface could significantly enhance multiple antibody properties. The scFv was re-engineered with mutations introduced at all eight sites, and the resulting variant was found to have greatly improved affinity and folding stability, indicating promise for this method of antibody optimization.
To remove the need for experimental deep mutational scans, a computational approach was used to identify favorable mutations at the VH/VL interface.Citation24 Changes in the native-state energy (ΔΔG) for each of the 19 possible amino acid substitutions for all residues located at the VH/VL interface were calculated. This information was combined with an evolutionary conservation score (position-specific scoring matrix (PSSM)) that represents the site-specific frequencies of each amino acid in natural antibodies to inform selection of multiple mutations that are predicted to cooperatively enhance antibody properties. This approach, named AbLIFT, was then fully automated and made available via a web server for accessible and widespread use. AbLIFT was validated by successfully predicting affinity- and stability-enhancing mutations for two additional antibodies. Calculations for all combinations of the predicted mutations were modeled in Rosetta and ranked based on their energies. Several of the best predicted antibody variants were produced as soluble scFvs and experimentally evaluated, with many demonstrating improved behavior for both affinity and stability. These improvements also persisted after reformatting the scFvs into IgGs. These successes demonstrate the power of AbLIFT toward guiding the rational design of antibody variants with improved biophysical properties. Nevertheless, this approach can also be computationally demanding, requiring thousands of CPU hours, which may limit its widespread application. Regardless, this approach could be used to improve the rational affinity maturation of antibodies while maintaining high folding stability.
Similar to affinity maturation, the process of humanization, a requisite for reducing the immunogenicity of antibodies discovered via animal immunization, often results in reduced folding stability.Citation25,Citation26 Humanization presents a key challenge by requiring major perturbations to the antibody structure while seeking to maintain other functional and biophysical properties such as affinity and stability. This is a difficult task that is further complicated by low-throughput methods for repetitive antibody cloning, expression, purification, and evaluation. To address this challenge, a computational approach was developed that requires only antibody homology models for predicting humanizing mutations that maintain folding stability. This approach, Computationally Driven Antibody Humanization (CoDAH), is an algorithm that uses structural energy information from homology models to identify humanizing mutations that minimally affect folding stability. CoDAH uses a human string content (HSC) score, based on the number of times a nine amino acid scanning window matches perfectly with the analogous region of the most similar human germline antibody, for an evaluation of humanness. Pareto optimization of the humanness score and homology model-derived structural energies facilitates identification of humanizing mutations that are predicted to maintain folding stability. When applied to a murine (anti-tumor antigen) antibody, CoDAH was successful at predicting sets of humanizing mutations for antibodies that retained both binding affinity and stability. In contrast, a variant of the antibody humanized with a more conventional approach of grafting complementarity-determining regions (CDRs) failed to express, likely due to poor stability. Further validation came from evaluation of CoDAH-guided humanization of cetuximab ().Citation27 In this study, CoDAH-informed designs were successful in humanizing the parental murine antibody while maintaining high stability. Although several mutation sites overlapped between the two methods (), CoDAH designs consistently outperformed CDR grafting, achieving humanization while maintaining rotomeric and thermal stability (). In addition, CoDAH designs better maintained affinity while CDR grafted designs often exhibited dramatic losses in affinity binding (), demonstrating the usefulness of this computational method for informing the rational design of humanized antibody candidates.
Figure 2. Computational design methods for minimizing stability trade-offs during antibody humanization. (a) A novel computational design method (CoDAH) for humanizing antibodies was used to predict humanizing mutations (green) for a murine antibody that otherwise would not be mutated using traditional CDR grafting humanizing designs (purple), as well as additional mutations common to both methods (blue). (b) Both traditional CDR grafting and CoDAH humanization results in antibodies with improved humanness, but the CoDAH designs also maintain, and even improve, rotomeric stability. (c) CoDAH designs also generally exhibit similar or improved thermal stability. (d) Both humanization methods result in antibody variants with similar affinity as the parental antibody, although several CDR grafting designs display reduced affinity. The figure is adapted with permission from a previous publication.Citation27
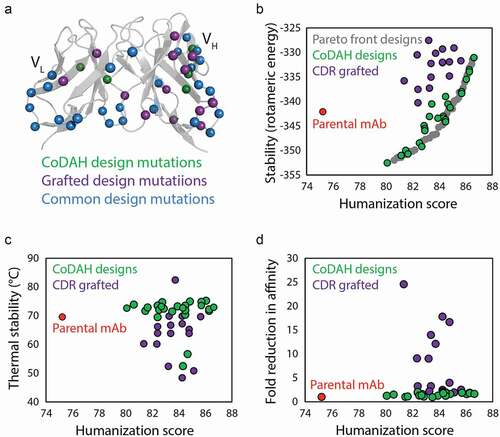
In addition to rational protein design, direct prediction of folding stability also holds great promise, especially through the use of machine learning algorithms. Of particular importance are artificial neural networks (ANNs), which are adept at recognizing patterns within highly non-linear data. ANNs are a collection of connected artificial neurons that receive inputs and produce outputs based on weights associated with each neuron. During the training process, penalties accrued from inaccurate predictions are backpropagated to update the weights and improve the accuracies of the predictions. This allows neural networks to efficiently converge on accurate models, making them extremely valuable. However, neural networks are also prone to overfitting, which can limit their ability to predict accurate values for new data. This limitation has plagued the biopharmaceutical field due to the limited quantity and diversity of available data.
The problem of overfitting was addressed in recent work by simplifying protein features and the structure of an ANN for the prediction of antibody biophysical properties indicative of folding stability at various formulation conditions.Citation28 To reduce collinearity of features and overfitting of the algorithm, only the count of each amino acid in the antibody sequence and the solution conditions of the viscosity measurements were used as features. The algorithm was then constructed with just five nodes in a single layer. The simplicity of the algorithm facilitates knowledge transfer from the fitting process, as the final weights of the nodes could be used to identify the amino acids and formulation conditions that contribute most to the prediction of the biophysical properties. Fifteen ANNs were trained on 144 data points (six IgGs at eight pH conditions with three salt concentrations) with cross-validation using a ‘leave-two-proteins-out’ method. Prediction of melting temperatures was highly accurate, with only one model having test accuracy less than 85%, while predictions of aggregation onset temperatures and diffusion interaction parameters (kD) were more variable with lower average test accuracies (~80%), which may be partially due to data imbalance. Knowledge transfer methods revealed that formulation conditions were important features for the prediction of all three biophysical properties, indicating the importance of choosing antibody formulations with care. However, this result could be heavily influenced by the greater diversity in formulation conditions (24) than antibody variants (six) in this study. As larger and more comprehensive datasets become available, these simplified neural net approaches should be revisited as they may generate even more accurate predictions of antibody folding stability.
Antibody self- and nonspecific interactions
Antibody colloidal interactions are also important indicators of developability and drug-like behavior. It was recently found that measurements of two colloidal properties, self-association and nonspecific interactions, could statistically discriminate between approved antibody therapeutics and those antibodies that either failed or were still in clinical development.Citation29 These important colloidal interactions are commonly associated with two different aspects of drug developability, namely in vitro antibody formulation behavior (solubility, viscosity, and opalescence)Citation30,Citation31 and in vivo antibody bioavailability (PK).Citation32,Citation33
Therapeutic antibodies are formulated at variable concentrations (~1-200 mg/mL) dictated by the route of administration; high antibody concentrations (>50-100 mg/mL) are a common requirement for subcutaneous or intraocular applications.Citation31,Citation34,Citation35 Self-association under these conditions can result in the formation of reversible entangled networks, facilitated by the bivalent nature of antibodies, that can result in highly viscoelastic solutions that are challenging to manufacture and administer.Citation36,Citation37 While desirable viscosity profiles are vital to the success of antibody therapeutics, they are extremely challenging to assess during early stage antibody development due to very limited quantities and concentrations of purified antibody that are incompatible with the requirements for viscosity measurements. To address this limitation, studies have sought to evaluate antibody self-association at lower antibody concentrations (<10 mg/mL) to identify either antibody variants or formulation conditions that minimize attractive antibody self-interactions and the corresponding viscous behaviors when antibodies are formulated at high concentration (>100 mg/mL).Citation38–43
One notable recent study demonstrated the value of measuring colloidal self-association at lower antibody concentrations to identify antibody variants with favorable solution properties at high formulation concentrations (~150 mg/mL).Citation43 At such high concentrations, the investigators found that a diverse set of 59 antibodies displayed variable solution properties, as some mAbs solutions became viscous (>30 cP) while others became opalescent (>12 NTU). Interestingly, the behaviors seemed to be exclusive, with no mAbs exhibiting both behaviors. Moreover, the investigators found that the diffusion interaction parameter (kD), which measures colloidal self-interactions and hydrodynamic interactions, was the best single predictor of high-concentration solution behaviors (viscosity and opalescence). Positive values or slightly negative values of kD generally reflect repulsive antibody self-interactions, while more negative values generally reflect attractive antibody self-interactions.Citation42–45 Impressively, a kD cutoff of >+20 mL/g could identify poorly behaved antibodies (viscosity >30 cP or opalescence >12 NTU at pH 6 and 10 mM histidine) with 95% accuracy. This result suggests that strong, long-range electrostatic repulsive interactions govern favorable solution properties at high antibody concentrations typical of formulation conditions (pH 6 and 10 mM histidine). Comparison of kD with several experimental and calculated descriptors revealed that antibody isoelectric point (pI) and net charge displayed the highest positive correlations, further demonstrating the key role of repulsive electrostatic interactions in high-concentration antibody solution behavior. While this study substantially advances our understanding of mediators of colloidal solution behaviors, a limitation is that it is not possible to perform measurements of the diffusion interaction parameter during the early-stage discovery of antibody candidates due to the requirements for relatively high-protein concentrations (1–10 mg/mL). Therefore, this study highlights the need for methods for measuring the diffusion interaction parameter or surrogates thereof at much lower antibody concentrations (<0.1 mg/mL).
One promising approach to address this need for these low-concentration measurements is affinity-capture self-interaction nanoparticle spectroscopy (AC-SINS),Citation46–48 which uses gold nanoparticles that display shifts in their plasmon wavelength due to interactions between the particles. Anti-human capture antibodies are first adsorbed onto the particles, which are then stabilized by thiolated polyethylene glycol. These conjugates are then used to capture human mAbs. The immunogold conjugates interact according to the degree of self-association exhibited by the immobilized mAbs, as judged by shifts in the plasmon wavelengths. These measurements have been shown to correlate with other experimental measurements, such as diffusion interaction parameters, solubilities, and viscosities.Citation47,Citation49,Citation50 Nevertheless, it is challenging to perform AC-SINS measurements at typical formulation conditions (low ionic strength solutions at pH 5–6) due to the low stability of the conjugates at such conditions. Therefore, future work should focus on further refining the AC-SINS method and evaluating its ability to robustly identify antibody variants with desirable viscosity properties in concentrated antibody formulations.
These and other limitations of early-stage experimental analysis of antibodies highlight the need for computational methods for predicting these properties. Recent advances in the rational design of proteins with decreased levels of self-association have involved identifying self-association motifs and hotspots via computational methodologies.Citation49,Citation51–53 Local electrostatic and hydrophobic interactions, amplified by molecular crowding in highly concentrated solutions, have often been implicated in antibodies that display high self-association and viscosity.Citation54–57 In-depth investigations of individual antibodies have provided some insight into possible mechanisms of self-association, and can help to inform their computational identification and motivate rational protein design. For example, the presence and distribution of charged residues in the antigen-binding site have been implicated in the unacceptably high viscosity of a well-studied antibody (omalizumab).Citation56,Citation58,Citation59 It has been shown that disruption of patches of negatively and positively charged residues decreases charge asymmetry and reduces viscosity.Citation51,Citation56,Citation60 This is theorized to result from reduced attraction between oppositely charged patches, which is especially important at low ionic strength conditions and formulation pHs near the antibody pI.Citation56,Citation61
However, the complexities of how attractive antibody self-interactions promote high antibody viscosity go well beyond electrostatic interactions. This was elegantly demonstrated for a highly associative antibody exhibiting abnormally high viscosity at antibody concentrations as low as 10 mg/mL.Citation62 In particular, the investigators evaluated the role of hydrophobic residues in antibody variable regions on self-association. They designed several charged and non-charged point mutations based on self-association hotspots identified by hydrogen-deuterium exchange (HDX)Citation63 and computational analysis.Citation62 Experimental evaluation showed that decreased number of hydrophobic residues in the variable domains reduced hydrophobicity and self-association both directly due to solvent-exposed mutations and indirectly due to buried mutations that presumably altered protein conformation. Additional experimental and computational modeling analysis revealed that two distinct hydrophobic patches – one in VH and the other in VL – together mediated self-association. Disruption of either hydrophobic patch with a single mutation resulted in an approximate four-fold reduction of viscosity, revealing the significant impact of subtle changes in antibody CDR composition on antibody viscosity. A notable limitation of this study is that the viscosity-reducing mutations also significantly reduced affinity by one to two orders of magnitude, which is consistent with other reports of trade-offs between antibody affinity and multiple biophysical properties.Citation12,Citation13,Citation64–68
A recent bioinformatics study of >100 clinical-stage mAbs highlighted the potential importance of hydrophobic residues in antibody CDRs in mediating self-association and nonspecific binding.Citation69 The use of phage display as a discovery technology has been linked to the production of antibodies with suboptimal biophysical properties relative to those produced by the immune system due to reduced quality control during in vitro selection relative to in vivo generation.Citation29,Citation70–72 In particular, it was posited that antibodies discovered via phage display generally contain elevated levels of hydrophobic residues in the CDRs. Interestingly, the authors found that particular CDRs in the heavy chain (HCDR2 and HCDR3) and light chain (LCDR3) contained increased levels of aliphatic residues for antibodies generated using phage display. These results may explain, at least in part, why the phage display-derived antibodies have higher average levels of self-association relative to the immunization-derived antibodies.Citation29 More generally, these and other results suggest that the presence of hydrophobic and charged patches in antigen-binding sites are important to consider during the design of antibody mutants with reduced self-association, but their relative importance is highly specific to the individual molecule. In addition, self-association motifs are often key mediators of antigen binding, indicating that simply eliminating charged and/or hydrophobic residues is insufficient for the robust design of optimized antibody variants.Citation73
While rational design and computational methods can be used to inform selections of mutations that may reduce self-association and viscosity of particular antibodies with abnormal properties, it is much more difficult to predict the risk of new antibodies for displaying abnormally high levels of self-association and/or viscosity based only on their sequences and corresponding predicted three-dimensional structures. This is particularly important during early-stage discovery when the large numbers of variants limit the feasibility of extensive experimentation. Therefore, in silico predictors of antibodies with increased risk for abnormally high viscosity have been developed that only require antibody sequences or, in some cases, predicted structures.Citation74,Citation75 One such method, referred to as the Sharma method, is based on the hypothesis that electrostatic and hydrophobic interactions dominate antibody viscoelastic behavior.Citation74 In particular, the authors evaluated three sequence-based properties of antibody variable regions, namely the Fv net charge (pH 5.5), the product of the net charges of VH and VL (charge symmetry at pH 5.5), and a measure of the hydrophobicity of antibody CDRs and other regions within the Fv (hydrophobicity index). They found that viscosity is correlated with the Fv net charge (r of −0.8) and charge symmetry (r of −0.8) for a panel of 14 mAbs. This suggests that charge repulsion reduces viscosity and charge asymmetry (e.g., oppositely charged variable regions) increases viscosity, which is consistent with other findings.Citation56 The correlation of viscosity with hydrophobicity was weaker (r of 0.6), which suggests that electrostatic interactions are generally more important than hydrophobic interactions in mediating abnormally high viscosity, although exceptions have been observed.Citation51,Citation62 Principal component regression of these three parameters resulted in a linear equation for calculating antibody viscosities at 180 mg/mL (pH 5.5 and 200 mM arginine-HCl) that are strongly correlated with experimental viscosity measurements for the set of 14 antibodies (r of 0.9).
The findings of the Sharma studyCitation74 have since been expanded upon in a second computational approach to viscosity prediction based on molecular features such as antibody negative charge and charge asymmetry.Citation76 In this method, homology models are used to calculate a spatial charge map (SCM) of the antibody variable region surface. An SCM score is calculated for each atom in the variable regions by summing partial charges for all atoms of amino acid side chains that are solvent exposed and predicted to be located within 10 Å. An SCM score is calculated for the variable regions via the absolute value of the sum of all negative atom SCM scores, fundamentally representing the negatively charged and exposed surface area in the variable regions. High variable region SCM scores are considered high risk for viscosity issues, although an exact SCM cutoff was not reported. This method was used to correctly identify five high viscosity antibodies from a 19 antibody dataset. However, three independent SCM cutoffs were chosen to correctly classify subgroups of antibodies from different companies. While demonstrating validation of features such as negative charge and charge asymmetry as important culprits of suboptimal antibody viscoelastic behavior, future work should address the need for more general cutoffs to accurately classify viscous antibodies using this method.
A related method for predicting antibody viscosity, referred to as the Tomar method, was developed to predict concentration-dependent antibody viscosity values instead of viscosities at a single antibody concentration.Citation75 This method is based on the observation that the exponential concentration dependence of solution viscosity can be linearized as [ln (normalized viscosity) = lnA+B*(mAb concentration)], and that the coefficient A is relatively constant while the coefficient B is variable for different antibodies. Coefficient A represents the theoretical relative viscosity at infinite dilution and was modeled by the average fit value for 16 experimentally measured viscosity curves. Coefficient B represents the exponential dependence of viscosity on antibody concentration. Interestingly, the investigators developed an equation for the coefficient B that was a linear combination of eight structure-based parameters, including charge (VH, VL and hinge net charge at pH 5.8) and hydrophobicity (IgG hydrophobic surface area). The structure-based parameters were obtained through full-length homology models of the antibodies, eliminating the need for crystal structures. The final model demonstrated good performance for predicting the measured viscosity curves of 16 antibodies (p-value < 0.05).
A more recent study highlighted key challenges in developing methods that are generally applicable for predicting antibody viscosity.Citation77 In hopes of remedying unacceptably high viscosity (>20 cP for mAb concentrations > 80 mg/mL) for an antibody specific for platelet-derived growth factor (PDGF), the investigators performed two iterative rounds of structure-guided mutagenesis and property evaluation (). In the first round, a highly accurate homology model was used to identify potentially beneficial mutations that would not reduce affinity, focusing on reducing the number of negatively charged residues or patches in the Fv region without introducing rare residues (based on natural human diversity) at conserved framework positions (). SharmaCitation74 and TomarCitation75 viscosity scores were also calculated for selected combinations of mutations and only those with improved scores were produced and evaluated. Of the 18 mutants that were tested experimentally, two had a common mutation in light chain CDR2 and showed modestly improved viscoelastic behavior. However, the most improved variant with this beneficial mutation was found to have low chemical stability, which was improved by altering the lambda germline to produce the Round 2 parental antibody (). The new parental variant was taken into a second round of optimization, but due to this low rate of success in the first round, design constraints were relaxed and mutations were biased toward those disrupting negatively charged patches in VH and VL (near the beneficial mutation in light chain CDR2 from round 1) without reducing the predicted affinity. Of the 22 variants produced and evaluated following the second round of design, two variants displayed the desired viscosity properties (<20 cP at 150 mg/mL mAb) and could be formulated at >150 mg/mL. These variants also displayed similar affinities (KD of ~100-300 pM) as wild type (KD of 95 pM). It is also particularly interesting that several similar types of charged mutations, which resulted in similar increases in antibody positive charge or reduction in negative charge, failed to alter the viscosity (). This underscores the surprisingly specific nature of protein–protein interactions that mediate antibody viscosity and raises concerns about the predictive power of methods that only consider general antibody properties (e.g., VL charge). Indeed, the investigators showed that multiple previous methods, including the Sharma and Tomar methods, had only modest ability to predict the relatively large differences in viscosity for closely related antibody variants in this study. This highlights both substantial challenges and opportunities for improving antibody viscosity predictions, which are critical for improving early-stage antibody developability analysis.
Figure 3. Evaluation of mutations that reduce viscosity at high antibody concentrations. (a) The impact of Fv mutations that modify antibody charge on viscosity was systematically evaluated to test the role of charge-related properties in mediating viscous antibody behavior. Systematic alteration of Fv charge in an anti-PDGF antibody rarely resulted in reduced viscosity, even when predicted to reduce viscosity by multiple conventional scoring methods. (b) Antibody variants with modest reductions in viscosity achieved in Round 1 (green) were further optimized in Round 2 through mutagenesis of negatively charged patches in the variable regions, which produced two antibody mutants that could be concentrated above 150 mg/mL while maintaining low viscosity (<20 cP). (c) Fv charge partially but incompletely describes the viscosity behavior, demonstrating the complicated relationship between antibody viscosity and charge properties. The figure is adapted with permission from a previous publication.Citation77
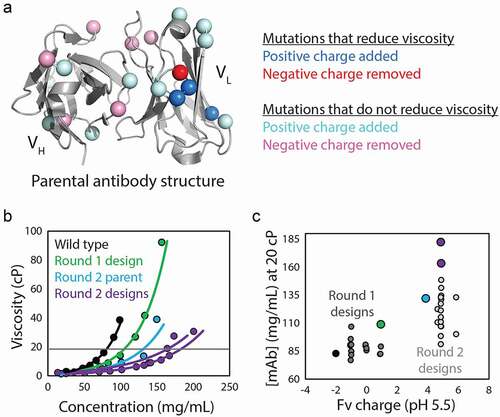
In addition to antibody self-association and the resulting viscoelastic behaviors, nonspecific interactions can significantly affect the developability and in vivo efficacy of antibody therapeutics. High levels of nonspecific interactions have been shown to correlate with abnormally high clearance rates, and such interactions significantly impact drug distribution within the body and can reduce bioavailability as well as therapeutic efficacy.Citation78–80 Additionally, antibody off-target binding decreases the opportunity for binding to the therapeutic target, which can reduce efficacy and potentially induce adverse effects. As observed for antibody self-association, investigations of antibody polyreactivity have revealed that these undesirable off-target interactions also are mediated by hydrophobic and electrostatic interactions.Citation81–84
To identify developability issues that could give rise to detrimental antibody properties, including self- and nonspecific interactions, the Therapeutic Antibody Profiler (TAP) was developed to easily analyze antibody sequence data and evaluate levels of risk relative to other clinical-stage therapeutics.Citation85 TAP uses five features that describe properties of antibodies linked to nonspecific interactions (electrostaticsCitation82–84), self-interactions (charge asymmetryCitation56,Citation81), aggregation (hydrophobicityCitation86,Citation87), and antigen binding (CDR loop lengthCitation88). The five TAP features identified were: (1) total CDR loop length; (2) surface hydrophobicity near the CDRs; (3) patches of positive charge near the CDRs; (4) patches of negative charges near the CDRs; and (5) variable region charge asymmetry. The thresholds were set by evaluating current clinical-stage antibodies with the assumption that candidates in Phase 2 and 3 clinical trials generally possess favorable properties. Antibodies predicted to be at high risk for developability issues are those with property values outside the ranges observed for the clinical-stage antibodies, while antibodies with moderate risk are those with properties that are within, but near the extremes of, the ranges observed for the clinical-stage antibodies. This approach is simple and fast, which makes it a logical first step in assessing potential developability risks for antibody therapeutics.
More recently, methods were reported that predict the relative risk for abnormal levels of nonspecific and self-interactions based on antibody variable region sequences and predicted site-specific solvent accessibilities.Citation53 The investigators found that over enrichment of specific types of positively charged (e.g., Arg) and non-charged (e.g., Gln, Tyr and Pro) residues and underrepresentation of specific types of negatively charged (e.g., Asp) and non-charged (e.g., Asn) residues, especially in the CDRs, was linked to increased risk for self-association and nonspecific binding. By combining 12 chemical rules that limit the minimum and maximum number of solvent-exposed residues in antibody CDRs and variable domains, the investigators were able to flag most clinical-stage antibodies (78%) with the highest levels of nonspecific and self-interactions while flagging few antibodies (8%) with the lowest levels of nonspecific and self-interactions. Interestingly, this methodology was also used to guide the re-engineering of a clinical-stage antibody (emibetuzumab) with high levels of nonspecific and self-interactions. The investigators identified key CDR sites predicted to mediate nonspecific and self interactions, diversified them with combinations of mutations predicted to increase antibody specificity, sorted the resulting libraries for low levels of nonspecific binding using yeast surface display, and performed deep sequencing of large panels of selected antibodies. Encouragingly, the selected antibody variants with significant reductions in nonspecific binding were well described by the chemical rules, as the experimentally selected variants with high specificity showed reduced numbers of violations of the chemical rules. Moreover, all of the best sets of mutations that reduced antibody nonspecific binding resulted in the introduction of at least one negatively charged CDR residue, suggesting that moderate levels of negatively charged residues in the CDRs can be beneficial for specificity. However, abnormally high levels of negative charge in antibody CDRs, which are rarely observed in clinical-stage antibodies,Citation53 can result in elevated levels of self-association, leading to high viscosity and nonspecific interactions.Citation56,Citation58,Citation59
Other powerful approaches to predict antibody nonspecific interactions have also been reported. A recent model was developed for the prediction of cross-interaction chromatography (CIC) retention times from antibody primary sequence.Citation89 CIC is an experimental method, first reported for characterization of globular proteins and then applied to antibodies,Citation90–92 that measures the interaction of proteins of interest (e.g., mAbs) with a second protein (e.g., a second mAb) or protein mixture (e.g., polyclonal antibody mixture) immobilized on chromatography column matrices. Antibodies with high levels of nonspecific interactions have been shown to associate with immobilized human polyclonal antibodies and display long retention times relative to those with low levels of nonspecific interactions.Citation90 Nonspecific interaction measurements obtained using this approach have been shown to correlate with aggregation propensity, solubility, and in vivo clearance rates.Citation90,Citation93 A quantitative structure–activity relationship (QSAR) model was developed using multiple types of sequence-based descriptors for the prediction of cross-interaction measurements using data from 46 humanized IgG1s. Antibody sequences were aligned and descriptors were generated using multiple computational programs (eMBOSS, Pepstat and ProtDCal) in addition to three amino acid scales (Z-scale, T-scale, and MS-WHIM). The best resulting model was found to use descriptors calculated from the running sum of a 13-amino acid scanning window and displayed high correlation when evaluated on a hold-out test set of antibodies (R2 of 0.99 and RMSE of 0.13). These findings support previous work demonstrating the importance of localized hydrophobic and charged patches resulting from the clustering of multiple residues that mediate undesirable antibody interactions.Citation67,Citation74,Citation94,Citation95 While the use of homology modeling has previously been shown to enable the discovery of such nonspecific interaction patches,Citation76 these findings demonstrate that they can also be effectively represented using only primary amino acid sequences. Therefore, this computational method can be used to analyze extremely large sets of antibodies for which structural modeling would be too computationally intensive, especially if such models are trained on larger and more diverse antibody datasets in the future.
Antibody aggregation and solubility
Antibody solubility and aggregation propensity are also key concerns during therapeutic development, especially during drug manufacturing and storage.Citation96 Formation of high molecular weight species is particularly concerning due to the potential ability of aggregates to elicit immunogenic responses in patients that can lead to accelerated clearance and pose serious safety concerns.Citation97,Citation98 While there are well-established analytical methods like size-exclusion chromatography (SEC) and dynamic light scattering for characterizing existing aggregates, there is still a great need for high-throughput screening methods for evaluating the aggregation potential of large numbers of antibodies available at the low concentrations, quantities and purities available during early-stage discovery and development.
A number of innovative experimental methods have been reported for identifying aggregation-prone antibodies. For example, a high-throughput protein conformational array based on Luminex multiplexing technology has been reported for investigating antibody aggregation. Citation99 In this assay, an array of 34 polyclonal antibodies that bind unique and typically buried epitopes in the antibody framework are immobilized on beads doped with unique combinations of dyes. The array is used to bind mAbs of interest under various conditions. Binding events are analyzed through detection of the dyes on beads, representing both reversible and irreversible unfolding of specific antibody regions that can associate to form aggregates. The assay was successfully applied to the identification of aggregation-prone antibody regions, thus enabling targeted antibody engineering. Additionally, this assay also was used to detect antibody aggregation for the myriad of possible solution conditions (buffer type, pH, temperature, and ionic strength) that antibodies are subjected to during manufacturing, storage, and administration. The high-throughput nature of this assay was demonstrated to be amenable to screening multiple formulation conditions and could also be easily adapted for parallel screening of the unfolding and aggregation behaviors of panels of early-stage lead candidates in standard manufacturing and formulation conditions. In addition, only small quantities (~0.01 mg) of antibody at relatively dilute concentrations (~0.1 mg/mL) are required for this assay.
For evaluation of native antibody aggregation, the tripartite TEM β-lactamase enzyme assay was adapted to link aggregation to E. coli cell growth.Citation100 For this assay, scFvs were inserted between two domains of the β-lactamase enzyme. Expression of the resulting tripartite fusion protein resulted in antibiotic resistance if the scFv remained soluble and did not disrupt folding of the enzyme. It was found that antibiotic resistance, measured via cell growth, was inversely correlated with scFv aggregation propensity, as measured by SEC and AC-SINS. This assay is also compatible with antibody library screening, making it a high-throughput deselection tool for aggregation-prone antibodies. However, the assay appears to primarily deselect antibodies prone to native aggregation, which does not address aggregation induced by chemical or thermal stresses common in antibody production and manufacturing processes. Nevertheless, these and other important findingsCitation99,Citation101,Citation102 have made it easier to experimentally evaluate antibody aggregation at early stages of antibody drug discovery for larger numbers of candidates (>100-1000 mAbs).
In addition, the rational design of antibodies with increased resistance to aggregation has been extensively addressed in recent years using various computational methods, which reduces the need for extensive experimentation.Citation89,Citation103–106 New algorithms for predicting aggregation-prone regions have been developed and existing methods have been significantly improved. For example, a recent combination of older tools for predicting aggregation-prone regions (TANGO and FoldX) resulted in the development of a new algorithm, Solubis, which has improved prediction accuracy due to the addition of structural information for distinguishing between surface exposed and buried aggregation-prone regions.Citation104,Citation105,Citation107,Citation108 Solubis uses the ability of TANGO to predict aggregation-prone regions that are at risk of β-strand amyloid aggregation in peptides and proteinsCitation103 based on biophysical parameters such as hydrophobicity, charge, and β-strand propensity. TANGO predictions of aggregation-prone regions are then combined with calculations of local free energies of folding stability (ΔGcontrib) assuming that aggregation-prone regions are only at risk for promoting aggregation if they are located in unstable regions.
Solubis was validated for the prediction of aggregation hotspots in an aggregation-prone antibody ().Citation107 Two critical aggregation hotspots were identified in the CDRs, one in light chain CDR2 (L2) and one in heavy chain CDR3 (H3) (). Changes in aggregation propensity and stability were calculated for mutations at each residue located in the wild-type aggregation-prone regions. Mutations predicted to disrupt aggregation as well as enhance local stability without disturbing the protein globally were selected for production and evaluation (). Several mutations for both CDRs were found to be beneficial, resulting in further reduction of aggregation when combined. The variants engineered with mutations that disrupt aggregation hotspots identified by Solubis generally displayed increased aggregation temperatures that approached the corresponding melting temperatures (), as well as other improved biophysical properties such as increased monomer content and expression titers. However, the variants with the greatest reduction in aggregation also demonstrated a total loss of antigen binding, exemplifying the difficulties of co-optimizing multiple antibody properties. Considering both antibody aggregation propensity and affinity, the best variant showed modest improvement in aggregation temperature, maintenance of antigen binding, and dramatically increased expression titer. Overall, this study demonstrates the power of combining structural features, including those obtained from homology models, and TANGO predictions of aggregation-prone regions for improving the design and selection of aggregation-resistant antibodies.
Figure 4. A computational method (Solubis) for predicting and remediating antibody aggregation hotspots. (a) Solubis identified two aggregation hotspots in an anti-VEGF antibody (PDB: 2FJF), namely one in light chain CDR2 (L2; blue) and one in heavy chain CDR3 (H3; green). (b) The two regions (circled in red) were predicted to be aggregation prone (high TANGO Score) and unstable (less negative free energy of folding). (c) Mutations in both CDRs L2 and H3 that were predicted to reduce aggregation (reduced TANGO Score) and improve folding stability (larger negative change in the free energy of folding) were selected to be evaluated. (d) Experimental evaluation of the antibody mutants revealed increased resistance to aggregation, as judged by increased aggregation temperatures that approached the antibody melting temperatures, particularly for variants with mutations in both CDRs. The figure is adapted with permission from a previous publication.Citation107
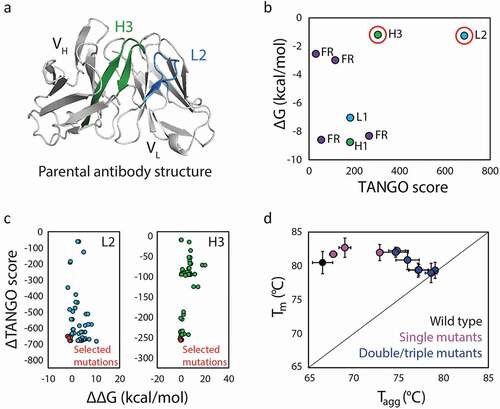
In addition to Solubis, another powerful method has been developed for calculating the relative aggregation propensity of proteins in terms of a spatial aggregation propensity (SAP) score.Citation106 This approach predicts aggregation hotspots by summing hydrophobicities of amino acids (weighted by solvent accessibility) within a defined radius of residues to calculate SAP scores for specific protein regions. High SAP scores indicate high risk for aggregation, which can be used to identify optimal regions for targeted mutagenesis. Recently, SAP was used to rationally design a biobetter of bevacizumab, an anti-vascular endothelial growth factor antibody used in the treatment of cancer and macular degeneration.Citation109 This mAb is aggregation prone, which limits the concentration of its therapeutic formulation and requires particular care during administration to avoid aggregation events. For these reasons, it is attractive to develop biobetter versions of this antibody. The high aggregation propensity of bevacizumab was addressed through SAP analysis, leading to the identification of ten high-risk residues in the antigen-binding fragment. Six of those residues were mutated in a rational protein design scheme, avoiding proline as well as residues in the antigen-binding site. Most of these selected residues were mutated to lysine to disrupt a hydrophobic patch predicted to mediate antibody aggregation. Encouragingly, all of the variants displayed reduced aggregation during accelerated stability studies and increased folding stability without reducing binding affinity. Bevacizumab variants were also generated by introducing glycosylation sites to disrupt four aggregation hotspots identified by SAP. The glycovariants displayed lower levels of aggregation, although they did not have improved folding stability as observed for the lysine variants. Overall, this study further validates the ability of SAP analysis to successfully guide the efficient rational design of aggregation-resistant mAbs.
Despite the power of rational design methods, most current approaches require antibody structural information in the form of crystal structures or homology models. This limits the throughput of such computational methods and their application to screening aggregation propensities of large numbers of early-stage mAb candidates. One approach developed to address this problem combines high-throughput experimental assays and machine learning methods to predict aggregation propensites using only antibody primary sequences.Citation86 This approach uses an aggregation screening assay in an ELISA-based format. In this method, the capture and detection antibodies are the same, preventing detection antibodies from binding to captured proteins unless multiple, higher-order species of the target protein are bound simultaneously. Importantly, this assay is well correlated with SEC results. The high-throughput nature of this assay enabled the generation of an aggregation dataset with >450 antibodies representing high sequence and structural diversity.
Next, machine learning models were developed to predict the measured antibody aggregation behavior based only on antibody sequence information.Citation86 These models used QSAR features extracted from antibody primary sequences, which consisted of several amino acid characteristics summed over a seven amino acid sliding window. These features were used to predict binary classification of aggregation behavior determined by a set threshold for the ELISA aggregation assay. It was found that specific machine learning methods (e.g., AdaBoost algorithm) performed best and achieved 75–78% accuracy (area under the curve of 0.76). Furthermore, the model outperformed a structure-based method (Developability Index) that combines SAP scores and antibody net charge for a set of 45 antibodies (84% accuracy for the machine learning models versus 53% accuracy for the Developability Index method).Citation87 These experimental and computational results show promise for the generation of large data sets and the prediction of aggregation propensites, especially at early stages of antibody drug development.
In addition to low aggregation propensity, high antibody solubility is also vital to meet formulation and manufacturing requirements. For many routes of administration (e.g., subcutaneous and intraocular), therapeutic antibodies must be soluble enough to be formulated at high concentrations (>100 mg/mL) in order to deliver sufficiently large doses with limited injection volume and frequency to ensure patient compliance and comfort. In addition, therapeutics must remain soluble at high antibody concentrations during the various processing steps encountered during the manufacturing process that can promote protein precipitation. To further complicate matters, hydrophobic and charged residues in the CDRs that are often implicated in reduced solubility are typically important for affinity and cannot be easily altered without loss of antigen binding. Therefore, it is important to develop computational tools that can efficiently guide the rational design of highly soluble antibodies as well as predict the solubility behavior of antibodies.
One such approach (CamSol) was developed to computationally screen potential antibody (and other protein) variants and select mutations predicted to enhance solubility while maintaining binding affinity and folding stability.Citation110 The algorithm calculates an intrinsic solubility profile from the protein sequence using a linear combination of physicochemical descriptors for each residue while considering the nature of neighboring residues. This solubility profile is then further corrected using structural information to account for solvent accessibility. Additional incorporation of structural information allows the deprioritization of residues predicted to be insoluble that are critical for proper folding, thus minimizing potential effects on folding stability. After identification of promising residues, the intrinsic solubility profiles are used to rapidly evaluate and rank all possible substitutions and insertions at the identified sites. When applied to a single-domain (VH) antibody, all seven of the CamSol-designed variants displayed increased solubility in a manner well correlated with the calculated solubility scores (R2 = 0.98). Notably, this was also accomplished without significantly affecting antigen-binding activity. CamSol has additionally been demonstrated to accurately predict the solubility of an IgG and eight of its mutants isolated during affinity maturation using phage display.Citation111 After removal of an outlier antibody, the calculated solubility scores correlated well with experimental solubility measurements (Pearson correlation coefficients of 0.93–0.97). Despite the limited number and diversity of antibodies used to validate CamSol, the results to date show promise for computational evaluation of antibody solubility. Moreover, the minimal computational expense of CamSol enables rapid and efficient analysis of large antibody panels for selecting leads or designing improved antibody variants.
In addition to CamSol, several machine learning models that predict protein solubility have been developed, most of which were trained on a dataset compiled from the Protein expression purification and crystallization Data Base (PepcDB) and Protein Data Bank (PDB).Citation112–115 This dataset consists of >82,000 proteins classified as ‘soluble’ or ‘insoluble’, although the classifications are determined by the databases using undefined criteria, resulting in uncertainty regarding the extent of solubilities for the proteins in the database. This is particularly problematic when attempting to predict solubility at extremely high concentrations. Despite this shortcoming, the dataset is quite extensive and provides an excellent opportunity to apply machine learning techniques for classifying protein solubilities and the elucidation of factors that mediate protein solubility. One of the developed algorithms, PaRSnIP (Protein Solubility Predictor), is a gradient boosting machine learning algorithm trained on a subset of this dataset (~70,000 after removing sequences with high sequence similarity) for solubility classification and feature extraction.Citation114 The algorithm is a nonlinear ensemble of regression trees trained on over 8,000 protein features with the final model achieving a test accuracy of 0.87 and a Matthews correlation coefficient of 0.74. Feature extraction additionally revealed that the features related to the fraction of highly solvent exposure residues (>65%) and the frequency of specific tripeptide combinations (particularly IHH) were the best predictors of poor protein solubility. A similar solubility predictor, DeepSol, was recently developed by training a convolutional neural network on the same dataset and achieved comparable classification accuracy and Matthew’s correlation coefficient relative to PaRSnIP on an independent test set.Citation113 Both models performed better than the previous state-of-the-art prediction algorithm, PROSO II, a support vector machine trained on the same dataset.Citation112
The same dataset was used in the development of another machine learning-based tool for predicting protein solubility, named SKADE.Citation115 SKADE is a neural network designed with neural attention architecture that enables highly accurate classification of solubility behavior and identification of features important for classification with more transparency than traditional neural networks. It also facilitates the identification of promising mutagenesis sites for the improvement of solubility behavior, making it particularly attractive for antibody applications. SKADE is composed of two neural network branches that both consist of two layers of bidirectional Gated Recurrent Networks (GRUs). The final layer of the networks use LeakyReLU to learn residue-specific solubility contributions or SoftMax to learn region-specific solubility contributions as the activation functions for the prediction and attention branches, respectively. The primary protein sequence is fed into the algorithm, translated into a 20-dimensional embedding that represents the 20 amino acids, and passed to both branches for analysis by the GRU layers. The outputs from both branches are tensors that are combined to generate a final scalar prediction. The algorithm was then trained on the protein dataset and tested on an independent test set with 2,000 sequences. SKADE performed comparably or slightly worse than DeepSolCitation113 and PaRSnIPCitation114 in predicting protein solubility and worse than CamSolCitation110 in predicting the impact of mutations on solubility. However, SKADE is only trained on sequence data, and therefore can be applied more broadly than the other algorithms. Additionally, SKADE was able to predict β-sheet aggregation in a similar manner as tools developed specifically to predict β-sheet aggregation, demonstrating enhanced generalizability. Additional analysis of the neural attention architecture revealed that protein termini were by far the most important regions for solubility classification, and therefore are good targets for protein engineering to improve solubility. While methodologies may be used for enhanced rational design of antibody therapeutics, the algorithms have yet to be validated on antibodies.
Another important aspect of the solubility of antibody therapeutics was addressed by the development of the algorithm SODA.Citation116 SODA is an algorithm designed to predict the change in solubility upon mutation. The algorithm uses a linear combination of amyloid structure aggregation energy (PASTA), ESpritz disorder propensity, negative Kyte–Doolittle hydrophobicity profile, α-helix structure propensity, and β-sheet structure propensity. Coefficients for the linear regression model were trained on a dataset of 443 amino acid substitutions in 71 proteins.Citation117 The model predicted solubility decreases with higher accuracy than solubility increases, which was hypothesized to be a result of the strong contribution of aggregation propensity descriptors to the predictions. The algorithm also performed comparably to CamSolCitation110 and PROSO II.Citation112 A web server was developed to automate predictions of solubility changes for various protein mutations. Implementation of the web server was demonstrated, but not experimentally validated, on a human germline antibody. While these methods show great promise, there is still much work needed to extend them to diverse types of antibodies and to apply them to address problems specific to the field of antibody development.
Antibody pharmacokinetics
PK properties strongly influence the therapeutic efficacy of a drug by determining its residence time in the body and ability to reach desired targets. While mAbs are well known for having relatively long half-lives, there are several reported examples of abnormally fast antibody clearance,Citation80,Citation82,Citation118 which may necessitate more frequent dosing. This is inconvenient and undesirable for patients and can reduce compliance. Fast clearance rates may even prevent efficient drug extravasation and distribution, thereby reducing bioavailability and target binding. Antibody clearance rates are known to be influenced by antibody charge, hydrophobicity, glycosylation, and receptor binding, all of which affect nonspecific and target-mediated clearance.Citation119–121 PK profiles are important to evaluate during drug development, but are also prohibitively slow and costly to directly measure during early-stage development due to the large required amount of drug product. Animal models are also complicated, and in some cases, incompatible with the human mAbs of interest. Additionally, even the most advanced in vivo models may not translate well to humans due to shortcomings in allometric scaling and discrepancies between animal and human physiology. Efficient methods of in vitro experimentation and computational modeling are needed for evaluation of PK properties early in drug development but suffer from many of the same issues as in vivo analysis, such as low throughput and poor translation to humans. Recent advances in experimental methods have addressed these challenges with some success,Citation120–122 enabling the generation of larger datasets for the eventual development of predictive computational tools.
Antibody Fc interaction with the neonatal receptor (FcRn) has been studied in depth due to its involvement in antibody recycling and transcytosis pathways and influence on antibody clearance.Citation123 Antibodies that are internalized into cells nonspecifically can bind to FcRn in a pH-dependent manner in endosomes and be released back into solution to avoid degradation. Failure to efficiently bind or release FcRn can accelerate clearance.Citation124,Citation125 Transcytosis assays have been developed that measure the pH-dependent transport of antibodies across a thin layer of Madin–Darby canine kidney (MDCK) cells engineered to express human FcRn. These assays have been used to measure differences in FcRn binding between antibodies with engineered and non-engineered Fc regions.Citation126 While the transcytosis measurements of antibody Fc variants correlate well with their PK profiles, measurements of antibodies with a wide range of antibody Fc regions have not shown similar agreement. An improved assay has been developed using stably transfected MDCK cells and the evaluation of transcytosis in more physiologically relevant conditions ().Citation121 The assay is conducted in media containing bovine serum albumin, which is also recycled by FcRn, but has a poorly understood influence on the binding of antibodies.Citation127 Additionally, conditions were altered such that antibody uptake primarily occurs by pinocytosisCitation121 rather than pH-dependent FcRn binding that dominated uptake in the original assay.Citation126 These changes more accurately recreate the in vivo processes that influence antibody PK. A panel of 53 mAbs with diverse Fc regions was evaluated with this improved assay, and measured rates of transcytosis were well correlated in a positive manner with human clearance data (i.e., faster clearance correlated with greater transcytosis; R2 > 0.8) ().Citation121 Interestingly, transcytosis measurements poorly correlated with FcRn affinity and nonspecific-binding measurements evaluated using the baculovirus particle-binding assay, suggesting that the in vitro transcytosis assay captures the combined effects of multiple events that accurately reflect in vivo recycling and transcytosis pathways. Notably, mAbs with high pIs (>8.5) displayed a higher risk for fast antibody clearance than lower pI mAbs (). This assay represents a substantial advance for in vitro screening, and can be used to evaluate a much wider variety of candidate antibodies than would be practical to evaluate in vivo.
Figure 5. Cell culture-based evaluation of FcRn-mediated antibody transcytosis correlates well with antibody clearance in vivo. (a) An in vitro transcytosis assay involves growing a monolayer of Madin–Darby canine kidney (MDCK) cells that overexpress human FcRn and measuring the amount of IgG in the outer chamber after extended incubation with excess IgG loaded in the inner chamber. (b) Measured rates of in vitro antibody transcytosis correlate with antibody clearance measured in humans (Pearson’s r = 0.90 and Spearman’s ρ = 0.77). (c) For a subset of antibodies that have been further experimentally characterized, isoelectric point (pI) displays some correlation with in vivo antibody clearance rates, as antibodies with high pIs have increased risk for fast antibody clearance (Pearson’s r = 0.57 and Spearman’s ρ = 0.57). The figure is adapted with permission from a previous publication.Citation121
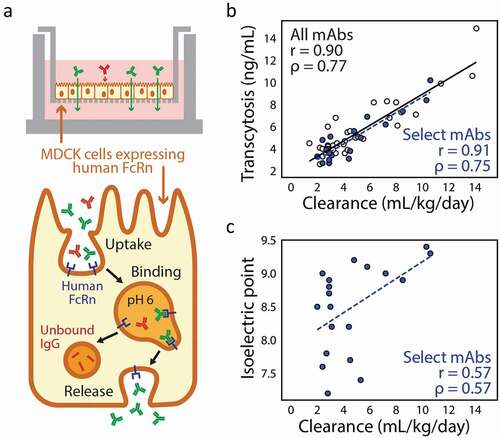
PK profiles can also be mechanistically modeled with physiologically based pharmacokinetic (PBPK) models using input parameters describing tissue distribution.Citation128 While such parameters are most accurately obtained from in vivo animal models, these experiments are again extremely difficult and costly, thus limiting the utility of such models. However, a recent study has revealed relationships between in vivo measurements with those obtained from in vitro experimental assays that are much higher throughput, greatly expanding the utility of PBPK models.Citation79 In particular, AC-SINS has been shown to be correlated with rates of antibody clearance in humans, possibly through detection of nonspecific binding that contributes to antibody clearance. Recently, researchers have generated PBPK models using only experimental measurements of AC-SINS, FcRn binding affinity, and physiological parameters to predict the in vivo clearance of 31 mAbs measured in mouse models.Citation129 A 16 compartment PBPK model was constructed with one plasma compartment and 15 tissue compartments, each consisting of six sub-compartments representing organs that can be modeled with single-cell mechanistic models of FcRn trafficking. Calibrated predictions showed significant agreement with PK profiles measured in mouse models with high accuracy for predicting problematic PK parameters such as half-life (90%), volume of distribution (90%) and clearance rates (71%). This PBPK model and the transcytosis assayCitation121 represent substantial advances in accurately predicting antibody PK profiles that require much less time and protein than in vivo models. However, the need for purified protein and limited throughput (particularly for the cell-based transcytosis assay) still do not allow for the early screening of large antibody panels to identify PK-related risks in early discovery. Therefore, further development of high-throughput experimental screens is necessary, in addition to improved understanding of the underlying mechanisms of poor PK behavior. Such advances would inform the design of in vitro systems that efficiently and accurately represent complex human physiology and the rational design of antibodies with improved PK profiles.
Recent studies have also investigated individual problematic antibodies as well as larger sets of more diverse mAbs and revealed various physicochemical properties that are associated with poor PK profiles.Citation74,Citation118,Citation130 Evaluation of an extensive dataset of 61 antibodies and their measured clearance rates in cynomolgus monkeys confirmed previous reports that antibody IgG properties such as pI and hydrophobic index were weakly correlated with clearance values.Citation74 However, the sum of CDRs L1, L3, and H3 hydrophobic index scores and the charge of the variable regions at pH 5.5 were found to discriminate between antibodies with high and low clearance with 75–86% accuracy. Well-behaved antibodies generally had hydrophobic index scores <4 and Fv charges between 0 and +6, highlighting the importance of electrostatic and hydrophobic interactions on nonspecific clearance. This classification model was further used to guide the rational design of two test antibodies, one with desirable clearance rates and a second one with rapid clearance.Citation131 Introduction of mutations that altered the hydrophobic index scores of CDRs L1, L3, and H3 as well as the Fv net charge significantly altered the measured cynomolgus clearance rates of several variants. Mutants with Fv net charge increased beyond the recommended range exhibited more rapid clearance for both antibodies, while mutants with Fv charge decreased toward the center of the recommended range exhibited greatly reduced clearance for a mAb that displayed fast clearance. These results confirm that Fv and CDR charge and hydrophobicity are important determinants of antibody developability, but the study is limited by the relatively small panel of analyzed antibodies. Improvements in experimental assays to more efficiently measure clearance rates and other PK properties would enable the generation of much larger and more extensive datasets for the development of generalizable computational models.
In addition to antibody clearance, bioavailability is extremely important for the efficacy of antibody drugs delivered through subcutaneous injection, which is the most desired route of administration due to patient convenience. Subcutaneous bioavailability describes the fraction of injected antibody that reaches systemic circulation for distribution to the target tissues and is difficult to predict due to the current deficits in mechanistic understanding of the various transport processes. Based on previous studies that found connections between antibody properties and bioavailability,Citation132–134 six antibodies were recently investigated in depth.Citation130 Multiple antibody properties were found to affect subcutaneous bioavailability, including polyspecificity, aggregation propensity, and thermal stability. Additionally, antibody variants with reduced local positive charge, higher specificity, higher thermal stability, and reduced aggregation potential had more favorable measured bioavailability. Protein engineering efforts to improve several of the undesirable biophysical properties of a suboptimal mAb, including self-association, precipitation, and poor expression, have provided additional evidence that poor PK can be related to various biophysical properties.Citation118 Systematic mutation of three highly interactive hydrophobic residues implicated in self-association resulted in a variant with significantly reduced aggregation propensity, increased half-life in vivo, and reduced nonspecific binding various tissues without loss of antigen binding. Overall, the improvement of multiple biophysical and PK properties suggests that the molecular determinants of poor PK behavior can also impact antibody aggregation and nonspecific binding. While various developability criteria have been presented as disparate properties, they are highly interconnected, especially for in vivo properties such as PK. Encouragingly, these findings suggest that the simultaneous consideration of multiple biophysical properties such as aggregation and nonspecific binding can guide the evaluation and improvement of complex, in vivo PK properties.
Antibody immunogenicity
The immunogenicity of antibodies, defined as their propensity to elicit immune responses, is also an important property of therapeutic proteins that affects their developability.Citation135 Such immune responses generally occur due to the recognition of antibodies as foreign substances in the body, resulting in their rapid removal and development of immune system memory. Antibodies discovered through animal immunization often are recognized by the human immune system as foreign due to differences in their sequences and structures between species. Early efforts in decreasing immunogenicity generally involved humanization of these foreign, often murine-derived, antibodies through grafting of the desired CDR loops onto human antibody frameworks.Citation136 However, this process often leads to reduction in function and folding stability. Recent advances have focused on improving the rational design of variants with increased humanness and high affinity.Citation26 For example, scoring methods for quantifying the level of humanness that evaluate correlations between residues at different positions during the humanization process have been improved.Citation137 Advances have also been made in animal immunization techniques through the development of transgenic mice with humanized immune systems that produce fully human antibodies.Citation136 However, a recent study found that mAbs from such mice are prone to rare mutations in framework regions due to somatic hypermutation that compromise their biophysical properties and immunogenicity profiles.Citation138 Antibody display methods, such as phage and yeast display, are also important for generating and screening of fully human synthetic libraries, thus eliminating the need for antibody humanization.Citation139,Citation140 Most recently, there is great interest in using human-derived B-cells in conjunction with next-generation sequencing technologies for rapid and efficient generation of fully human mAbs.Citation141–143 However, these discovery platforms lack efficient negative selection processes to deselect for self-reactivity relative to naturally derived antibodies, and therefore are at higher risk for immunogenicity problems. The ubiquity of this issue demonstrates a great need for experimental and computational approaches for diagnosing and reducing the immunogenicity of antibody candidates.
Immunogenicity is a difficult property to experimentally measure because adverse reactions are caused by a cascade of immunological events that vary greatly even between different individuals.Citation135 These events are controlled by complex and poorly understood feedback mechanisms that are difficult to represent through experimentation. One of the main immunogenic risks for antibody therapeutics, particularly for treating chronic illnesses that require repeated and long-term antibody administration, is the development of anti-drug antibodies (ADAs). ADAs are formed after professional antigen-presenting cells, such as dendritic cells, interact with and endocytose antibody drugs. The cells then cleave the antibody drugs into short peptides, some of which may be displayed by major histocompatibility complex class II (MHCII) molecules on the cell surface.Citation144–146 T cells must then recognize these presented peptides as foreign and become activated, initiating various immune responses that include the generation of ADAs, which circulate in the body as part of the adaptive immune memory and neutralize subsequent doses of the therapeutic (). This neutralization accelerates drug clearance and decreases drug efficacy due to the inability of the therapeutic to bind its target.Citation147 Prediction of immunogenic responses to antibody therapeutics is vital, as adverse reactions can render antibody therapeutics inactive or harmful, depending on many coalescing factors in a complicated and unpredictable manner.
Figure 6. Repeated administration of therapeutic antibodies can lead to the development of anti-drug antibodies and immunogenicity. Antibody therapeutics are first internalized by APCs and processed into peptides, some of which are presented by the major histocompatibility complex II (MHCII) as foreign antigens to naïve T cells (top). Activated T cells then proliferate, release chemokines, and activate naïve B-cells (middle). Activated B-cells mature into plasma cells and produce ADAs in large quantities, leading to immunogenicity (bottom). While there are currently no reported assays for directly predicting clinical immunogenicity, various steps in the ADA development process can be modeled and predicted
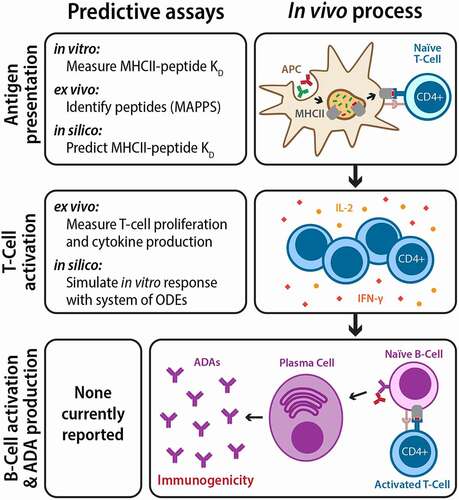
While an accurate picture of the immunogenicity profile of antibody candidates cannot be constructed before it has been administered and monitored long term in a large and diverse population, several experimental techniques have been developed for preclinical risk assessment.Citation148–150 These techniques generally involve the assessment of known key steps in the initial immune response, such as MHCII binding and T-cell activation, through ex vivo cellular assays. MHCII binding is evaluated through MHC-associated peptide proteomics (MAPPs) in which mAbs are incubated with human-derived dendritic cells and the displayed peptides are identified with mass spectrometry to determine antibody regions with high immunogenic risk.Citation148,Citation149 This assay has been demonstrated to successfully identify immunogenic sequences of problematic antibodies such as infliximabCitation149 and describe the likelihood of ADA development.Citation148 T-cell activation can be assessed through measurement of cell proliferation (via flow cytometry or [3H]-thymidine incorporation) and production of cytokines such as interleukin-2 (IL-2) or interferon (IFN)-γ (via enzyme-linked immunospot or ELISpot assays) following co-incubation of donor-derived T cells with antigen-presenting cells (APCs; naïve or pre-incubated with therapeutic mAb). Both T-cell proliferation and cytokine secretion have been shown to relate to rates of clinical immunogenicity.Citation150 This importance of assessing T-cell activation in addition to MHCII peptide presentation was highlighted in a recent study of two mAbs (secukinumab and ixekizumab) with the same target antigen, but with different rates of clinical immunogenicity.Citation151 While the MAPPs assay identified similar numbers of potentially antigenic peptides for the two mAbs, subsequent T-cell assays (IFN-γ ELISpot) revealed that only those of ixekizumab acted as T-cell epitopes, leading to increased frequency of mAb-specific T cells. The major limitation of these experimental assays is the necessary usage of donor-derived cells, which requires careful and extensive processing and represents a small fraction of patient diversity. Similar to preclinical animal trials, these cellular assays provide vital experimental risk assessment but are impractical for screening large numbers of candidate mAbs in early discovery.
To broaden screening capabilities, computational tools have been developed that identify regions in protein sequences that are at high risk for recognition by MHCII molecules with relatively high accuracy and which can be used in screening or rational design of mAbs.Citation152–154 These tools are built with binding data specific to the major alleles for MHCII molecules, thus covering the majority of the population while also enabling customization based on the target population. Recently, the computational tool EpiSweep was developed for the rational design of functional protein variants and libraries with decreased immunogenicity.Citation154 EpiSweep uses existing tools for identifying immunogenic epitopes to mutate and predict immunogenicity following mutation. This program also evaluates the effects of these epitope-deleting mutations on function and stability using sequence- or structure-based scoring methods. This enables the identification of a series of variants or library designs on the Pareto frontier that represent the trade-offs minimal possible between immunogenicity and function. β-lactamase variants with several mutations designed with EpiSweep demonstrated markedly decreased immunogenic potential (based on degree of MHCII binding to wild-type versus mutated peptides) with similar or slightly decreased activity and thermostability as the wild type.Citation155,Citation156 Additionally, a combinatorial β-lactamase library with several mutations at the ‘elbow’ of the Pareto frontier designed by EpiSweep displayed decreased MHCII peptide binding and high overall activity and stability. The highest performing individual variants were isolated from a designed library with up to 30 mutations. One of these variants contained 14 mutations, displayed the same melting temperature and better enzymatic activity than wild type, and only showed significant T-cell activation (measured via IL-2 ELISpot) for one of the 18 donors, as compared to eight of 18 donors for the wild type.Citation157
An additional computational tool, TCPro, has been developed to assess the immunogenicity profiles of therapeutic proteins in silico.Citation153 Predicting the clinical immune response (especially ADA development) is complicated because it involves protein processing, antigen presentation by MHCII molecules, T-cell activation, and ultimately B-cell activation. Nevertheless, TCPro uses recent advances in computational methods to incorporate analyses of different genotypes present among the population, which enables coverage of a greater and more relevant amount of genetic diversity than is possible experimentally. TCPro simulates T-cell proliferation and IL-2 secretion assays using non-linear ordinary differential equations.Citation153 Predictions are generated using three user inputs: 1) amino acid sequences of the therapeutic proteins; 2) ratios of CD4+ cells that will bind to the drugs (FP); and 3) MHCII genotypes of the individual or populations of interest. MHCII binding affinities (predicted by NetMCHIIpan), randomly assigned counts of various cell types (within reported ranges), and drug concentrations are then input to the system of differential equations that model the time-dependent dynamics of the T-cell populations. Individuals predicted to have significantly greater T-cell proliferation and IL-2 secretion in the presence of protein drugs are considered immunogenic. User-defined populations of specified size are modeled by simulating individuals with the same genotype distribution as the population of interest, with the percentage of responders given as the final output. TCPro was able to predict the percentage of donors responding in both ex vivo assays for experimentally characterized peptide fragments from a blood clotting factor and three control proteins with mean absolute percent error of 4.2%. TCPro predictions for 15 therapeutic antibodies and other proteins were generally found to correlate with rates of clinically observed immunogenicity, often better than ex vivo experimental assays.
While these computational tools were developed for application toward diverse types of therapeutic proteins, they can easily be applied to mAbs. However, the frequency of uptake and processing by APCs may differ between mAbs and other therapeutic proteins, thereby affecting the likelihood of developing ADAs. Both experimental and computational methods rely on simulating simplified versions of several key steps in ADA development for the prediction of clinical immunogenicity, which do not fully represent the complex cascade of events performed by diverse immune cells involved in a true immune response. Advancements in this field will need to consider the function of B cells, which interact with activated T cells to produce ADAs but also act as APCs, though less efficiently than professional APCs such as dendritic cells. Additionally, ADAs are most problematic upon repeated drug administration, but none of the existing methods discussed here considers the effects of immunological memory. Nevertheless, preclinical experimental assessment is vital toward ensuring patient safety, and computational tools such as EpiSweepCitation154 and TCProCitation153 represent substantial advances in screening candidates and guiding rational protein engineering.
Conclusions
Despite the impressive advances in using mAbs as potent therapeutics, there are still many challenges that continue to frustrate their efficient development. There remains great need for high-throughput experimental techniques that facilitate the evaluation of biophysical properties at extremely low concentrations and which require low quantities of protein available at the antibody discovery stage. This is of particular importance for in vivo properties, such as PK and immunogenicity, that suffer from a critical lack of key experimental data and may be evaluated as late as after the initiation of clinical trials. In addition, the systematic generation of high-quality data from these high-throughput techniques will be invaluable to further the understanding of biophysical properties for enhanced rational design and computational de-risking of candidate molecules. This data will be critical for improving computational methods that show great promise for enhancing antibody developability yet have been limited to date by the lack of sufficiently large datasets. It will be important to broaden the scope of these computational methods to include full-length IgG modeling, as well as protein dynamics to mimic real formulation conditions. Increasing the application of machine learning methods for the analysis of emerging experimental datasets may yield great advances and warrants attention for future work to address the remaining challenges hindering the development of antibody therapeutics. Importantly, these methods will rely on improvements in feature extraction to accurately describe characteristics of antibodies that are deterministic of their developability properties, which is possibly the greatest remaining challenge. If the rapid progress in the field continues at the current pace, we expect that advances in the coming years related to evaluating and predicting antibody developability properties will substantially reduce late-stage failures and provide more effective and safer therapeutics for patients.
Abbreviations
PK: Pharmacokinetic; CDR: Complementarity-determining region; pI: Isoelectric point; Fv: variable fragment; scFv: single-chain variable fragment; SEC: Size-exclusion chromatography; SAP: Spatial Aggregation Propensity; PepcDB: Protein expression purification and crystallization Data Base; PDB: Protein Data Bank; ADA: anti-drug antibody; APC: antigen-presenting cell.
Conflicts of interest
The authors declare no conflict of interest.
Author contributions
E.K.M., L.W., P.G., and P.M.T wrote the manuscript. E.K.W. and L.W. prepared the figures.
Acknowledgments
We thank members of the Tessier lab for their feedback on drafts of this manuscript.
Additional information
Funding
References
- Buss NA, Henderson SJ, McFarlane M, Shenton JM, De Haan L. Monoclonal antibody therapeutics: history and future. Curr Opin Pharmacol. 2012;12:615–20. doi:10.1016/j.coph.2012.08.001.
- Borrebaeck CA, Carlsson R. Human therapeutic antibodies. Curr Opin Pharmacol. 2001;1:404–08. doi:10.1016/S1471-4892(01)00070-4.
- Brekke OH, Sandlie I. Therapeutic antibodies for human diseases at the dawn of the twenty-first century. Nat Rev Drug Discov. 2003;2:52–62. doi:10.1038/nrd984.
- Chiu ML, Gilliland GL. Engineering antibody therapeutics. Curr Opin Struct Biol. 2016;38:163–73. doi:10.1016/j.sbi.2016.07.012.
- Posner J, Barrington P, Brier T, Datta-Mannan A. Monoclonal antibodies: past, present and future. Handb Exp Pharmacol. 2019;260:81–141.
- Waldmann H. Human monoclonal antibodies: the benefits of humanization. Methods Mol Biol. 2019;1904:1–10.
- Almagro JC, Fransson J. Humanization of antibodies. Front Biosci. 2008;13:1619–33.
- Xu Y, Wang D, Mason B, Rossomando T, Li N, Liu D, Cheung JK, Xu W, Raghava S, Katiyar A, et al. Structure, heterogeneity and developability assessment of therapeutic antibodies. MAbs. 2019;11(2):239–64. doi:10.1080/19420862.2018.1553476.
- Jarasch A, Koll H, Regula JT, Bader M, Papadimitriou A, Kettenberger H. Developability assessment during the selection of novel therapeutic antibodies. J Pharm Sci. 2015;104:1885–98. doi:10.1002/jps.24430.
- Ministro J, Manuel AM, Goncalves J. Therapeutic antibody engineering and selection strategies. Adv Biochem Eng Biotechnol. 2020;171:55–86. doi:10.1007/10_2019_116.
- Kennedy PJ, Oliveira C, Granja PL, Sarmento B. Monoclonal antibodies: technologies for early discovery and engineering. Crit Rev Biotechnol. 2018;38:394–408. doi:10.1080/07388551.2017.1357002.
- Rabia LA, Desai AA, Jhajj HS, Tessier PM. Understanding and overcoming trade-offs between antibody affinity, specificity, stability and solubility. Biochem Eng J. 2018;137:365–74. doi:10.1016/j.bej.2018.06.003.
- Stimple SD, Smith MD, Tessier PM. Directed evolution methods for overcoming trade-offs between protein activity and stability. AIChE J. 2020;66:e16814. doi:10.1002/aic.16814.
- Le Basle Y, Chennell P, Tokhadze N, Astier A, Sautou V. Physicochemical stability of monoclonal antibodies: a review. J Pharm Sci. 2020;109:169–90.
- Lowe D, Dudgeon K, Rouet R, Schofield P, Jermutus L, Christ D. Aggregation, stability, and formulation of human antibody therapeutics. Adv Protein Chem Struct Biol. 2011;84:41–61.
- Perchiacca JM, Tessier PM. Engineering aggregation-resistant antibodies. Annu Rev Chem Biomol Eng. 2012;3:263–86. doi:10.1146/annurev-chembioeng-062011-081052.
- Rosenberg AS. Effects of protein aggregates: an immunologic perspective. Aaps J. 2006;8:E501–7. doi:10.1208/aapsj080359.
- Frokjaer S, Otzen DE. Protein drug stability: a formulation challenge. Nat Rev Drug Discov. 2005;4:298–306. doi:10.1038/nrd1695.
- Miller BR, Demarest SJ, Lugovskoy A, Huang F, Wu X, Snyder WB, Croner LJ, Wang N, Amatucci A, Michaelson JS, et al. Stability engineering of scFvs for the development of bispecific and multivalent antibodies. Protein Eng Des Sel. 2010;23(7):549–57. doi:10.1093/protein/gzq028.
- Goldberg DS, Lewus RA, Esfandiary R, Farkas DC, Mody N, Day KJ, Mallik P, Tracka MB, Sealey SK, Samra HS, et al. Utility of high throughput screening techniques to predict stability of monoclonal antibody formulations during early stage development. J Pharm Sci. 2017;106(8):1971–77. doi:10.1016/j.xphs.2017.04.039.
- Lippow SM, Wittrup KD, Tidor B. Computational design of antibody-affinity improvement beyond in vivo maturation. Nat Biotechnol. 2007;25:1171–76. doi:10.1038/nbt1336.
- Bostrom J, Lee CV, Haber L, Fuh G. Improving antibody binding affinity and specificity for therapeutic development. Methods Mol Biol. 2009;525:353–76. xiii.
- Kuroda D, Tsumoto K. Antibody affinity maturation by computational design. Methods Mol Biol. 2018;1827:15–34.
- Warszawski S, Borenstein Katz A, Lipsh R, Khmelnitsky L, Ben Nissan G, Javitt G, Dym O, Unger T, Knop O, Albeck S, et al. Optimizing antibody affinity and stability by the automated design of the variable light-heavy chain interfaces. PLoS Comput Biol. 2019;15(8):e1007207. doi:10.1371/journal.pcbi.1007207.
- Clark M. Antibody humanization: a case of the ‘Emperor’s new clothes’? Immunol Today. 2000;21:397–402. doi:10.1016/S0167-5699(00)01680-7.
- Choi Y, Hua C, Sentman CL, Ackerman ME, Bailey-Kellogg C. Antibody humanization by structure-based computational protein design. MAbs. 2015;7:1045–57. doi:10.1080/19420862.2015.1076600.
- Choi Y, Ndong C, Griswold KE, Bailey-Kellogg C. Computationally driven antibody engineering enables simultaneous humanization and thermostabilization. Protein Eng Des Sel. 2016;29:419–26. doi:10.1093/protein/gzw024.
- Gentiluomo L, Roessner D, Augustijn D, Svilenov H, Kulakova A, Mahapatra S, Winter G, Streicher W, Rinnan Å, Peters GHJ, et al. Application of interpretable artificial neural networks to early monoclonal antibodies development. Eur J Pharm Biopharm. 2019;141:81–89. doi:10.1016/j.ejpb.2019.05.017.
- Jain T, Sun T, Durand S, Hall A, Houston NR, Nett JH, Sharkey B, Bobrowicz B, Caffry I, Yu Y, et al. Biophysical properties of the clinical-stage antibody landscape. Proc Nat Acad Sci. 2017;114(5):944–49. doi:10.1073/pnas.1616408114.
- Falconer RJ. Advances in liquid formulations of parenteral therapeutic proteins. Biotechnol Adv. 2019;37:107412. doi:10.1016/j.biotechadv.2019.06.011.
- Shire SJ. Formulation and manufacturability of biologics. Curr Opin Biotechnol. 2009;20:708–14. doi:10.1016/j.copbio.2009.10.006.
- Lobo ED, Hansen RJ, Balthasar JP. Antibody pharmacokinetics and pharmacodynamics. J Pharm Sci. 2004;93:2645–68. doi:10.1002/jps.20178.
- Dostalek M, Gardner I, Gurbaxani BM, Rose RH, Chetty M. Pharmacokinetics, pharmacodynamics and physiologically-based pharmacokinetic modelling of monoclonal antibodies. Clin Pharmacokinet. 2013;52:83–124.
- Wang W, Singh S, Zeng DL, King K, Nema S. Antibody structure, instability, and formulation. J Pharm Sci. 2007;96:1–26. doi:10.1002/jps.20727.
- Viola M, Sequeira J, Seiça R, Veiga F, Serra J, Santos AC, et al. Subcutaneous delivery of monoclonal antibodies: how do we get there? J Control Release. 2018;286:301–14.
- Schmit JD, He F, Mishra S, Ketchem RR, Woods CE, Kerwin BA. Entanglement model of antibody viscosity. J Phys Chem B. 2014;118:5044–49. doi:10.1021/jp500434b.
- Tomar DS, Kumar S, Singh SK, Goswami S, Li L. Molecular basis of high viscosity in concentrated antibody solutions: strategies for high concentration drug product development. MAbs. 2016;8:216–28. doi:10.1080/19420862.2015.1128606.
- Liu J, Nguyen MD, Andya JD, Shire SJ. Reversible self-association increases the viscosity of a concentrated monoclonal antibody in aqueous solution. J Pharm Sci. 2005;94:1928–40. doi:10.1002/jps.20347.
- Kanai S, Liu J, Patapoff TW, Shire SJ. Reversible self-association of a concentrated monoclonal antibody solution mediated by Fab-Fab interaction that impacts solution viscosity. J Pharm Sci. 2008;97:4219–27. doi:10.1002/jps.21322.
- Arora J, Hu Y, Esfandiary R, Sathish HA, Bishop SM, Joshi SB, et al. Charge-mediated Fab-Fc interactions in an IgG1 antibody induce reversible self-association, cluster formation, and elevated viscosity. MAbs. 2016;8:1561–74. doi:10.1080/19420862.2016.1222342.
- Yadav S, Shire SJ, Kalonia DS. Viscosity behavior of high-concentration monoclonal antibody solutions: correlation with interaction parameter and electroviscous effects. J Pharm Sci. 2012;101:998–1011. doi:10.1002/jps.22831.
- Connolly Brian D, Petry C, Yadav S, Demeule B, Ciaccio N, Moore Jamie MR, Shire S, Gokarn Y. Weak interactions govern the viscosity of concentrated antibody solutions: high-throughput analysis using the diffusion interaction parameter. Biophys J. 2012;103:69–78. doi:10.1016/j.bpj.2012.04.047.
- Kingsbury JS, Saini A, Auclair SM, Fu L, Lantz MM, Halloran KT, Calero-Rubio C, Schwenger W, Airiau CY, Zhang J, et al. A single molecular descriptor to predict solution behavior of therapeutic antibodies. Sci Adv. 2020;6(32):eabb0372. doi:10.1126/sciadv.abb0372.
- Lehermayr C, Mahler HC, Mäder K, Fischer S. Assessment of net charge and protein-protein interactions of different monoclonal antibodies. J Pharm Sci. 2011;100:2551–62. doi:10.1002/jps.22506.
- Saluja A, Fesinmeyer RM, Hogan S, Brems DN, Gokarn YR. Diffusion and sedimentation interaction parameters for measuring the second virial coefficient and their utility as predictors of protein aggregation. Biophys J. 2010;99:2657–65. doi:10.1016/j.bpj.2010.08.020.
- Sule SV, Dickinson CD, Lu J, Chow CK, Tessier PM. Rapid analysis of antibody self-association in complex mixtures using immunogold conjugates. Mol Pharm. 2013;10:1322–31. doi:10.1021/mp300524x.
- Wu J, Schultz JS, Weldon CL, Sule SV, Chai Q, Geng SB, Dickinson CD, Tessier PM. Discovery of highly soluble antibodies prior to purification using affinity-capture self-interaction nanoparticle spectroscopy. Protein Eng, Design Sel. 2015;28:403–14. doi:10.1093/protein/gzv045.
- Liu Y, Caffry I, Wu J, Geng SB, Jain T, Sun T, Reid F, Cao Y, Estep P, Yu Y, et al. High-throughput screening for developability during early-stage antibody discovery using self-interaction nanoparticle spectroscopy. MAbs. 2014;6(2):483–92. doi:10.4161/mabs.27431.
- Shan L, Mody N, Sormani P, Rosenthal KL, Damschroder MM, Esfandiary R. Developability assessment of engineered monoclonal antibody variants with a complex self-association behavior using complementary analytical and in silico tools. Mol Pharm. 2018;15:5697–710. doi:10.1021/acs.molpharmaceut.8b00867.
- Geng SB, Wittekind M, Vigil A, Tessier PM. Measurements of monoclonal antibody self-association are correlated with complex biophysical properties. Mol Pharm. 2016;13:1636–45. doi:10.1021/acs.molpharmaceut.6b00071.
- Nichols P, Li L, Kumar S, Buck PM, Singh SK, Goswami S, Balthazor B, Conley TR, Sek D, Allen MJ, et al. Rational design of viscosity reducing mutants of a monoclonal antibody: hydrophobic versus electrostatic inter-molecular interactions. MAbs. 2015;7:212–30. doi:10.4161/19420862.2014.985504.
- Wolf Pérez AM, Sormanni P, Andersen JS, Sakhnini LI, Rodriguez-Leon I, Bjelke JR, Gajhede AJ, De Maria L, Otzen DE, Vendruscolo M, et al. In vitro and in silico assessment of the developability of a designed monoclonal antibody library. MAbs. 2019;11:388–400. doi:10.1080/19420862.2018.1556082.
- Zhang Y, Wu L, Gupta P, Desai AA, Smith MD, Rabia LA, Ludwig SD, Tessier PM. Physicochemical rules for identifying monoclonal antibodies with drug-like specificity. Mol Pharm. 2020;17(7):2555–69. doi:10.1021/acs.molpharmaceut.0c00257.
- Yadav S, Shire SJ, Kalonia DS. Factors affecting the viscosity in high concentration solutions of different monoclonal antibodies. J Pharm Sci. 2010;99:4812–29.
- Esfandiary R, Parupudi A, Casas-Finet J, Gadre D, Sathish H. Mechanism of reversible self-association of a monoclonal antibody: role of electrostatic and hydrophobic interactions. J Pharm Sci. 2015;104:577–86. doi:10.1002/jps.24237.
- Yadav S, Laue TM, Kalonia DS, Singh SN, Shire SJ. The influence of charge distribution on self-association and viscosity behavior of monoclonal antibody solutions. Mol Pharm. 2012;9:791–802. doi:10.1021/mp200566k.
- Li J, Cheng Y, Chen X, Zheng S. Impact of electroviscous effect on viscosity in developing highly concentrated protein formulations: lessons from non-protein charged colloids. Int J Pharm: X. 2019;1:100002.
- Yadav S, Liu J, Shire SJ, Kalonia DS. Specific interactions in high concentration antibody solutions resulting in high viscosity. J Pharm Sci. 2010;99:1152–68. doi:10.1002/jps.21898.
- Yadav S, Sreedhara A, Kanai S, Liu J, Lien S, Lowman H, Kalonia DS, Shire SJ. Establishing a link between amino acid sequences and self-associating and viscoelastic behavior of two closely related monoclonal antibodies. Pharm Res. 2011;28:1750–64. doi:10.1007/s11095-011-0410-0.
- Yadav S, Shire SJ, Kalonia DS. Viscosity analysis of high concentration bovine serum albumin aqueous solutions. Pharm Res. 2011;28:1973–83. doi:10.1007/s11095-011-0424-7.
- Sule SV, Cheung JK, Antochshuk V, Bhalla AS, Narasimhan C, Blaisdell S, et al. Solution pH that minimizes self-association of three monoclonal antibodies is strongly dependent on ionic strength. Mol Pharm. 2012;9:744–51. doi:10.1021/mp200448j.
- Geoghegan JC, Fleming R, Damschroder M, Bishop SM, Sathish HA, Esfandiary R. Mitigation of reversible self-association and viscosity in a human IgG1 monoclonal antibody by rational, structure-guided Fv engineering. MAbs. 2016;8:941–50. doi:10.1080/19420862.2016.1171444.
- Arora J, Hickey JM, Majumdar R, Esfandiary R, Bishop SM, Samra HS, Middaugh CR, Weis DD, Volkin DB. Hydrogen exchange mass spectrometry reveals protein interfaces and distant dynamic coupling effects during the reversible self-association of an IgG1 monoclonal antibody. MAbs. 2015;7:525–39. doi:10.1080/19420862.2015.1029217.
- Julian MC, Li L, Garde S, Wilen R, Tessier PM. Efficient affinity maturation of antibody variable domains requires co-selection of compensatory mutations to maintain thermodynamic stability. Sci Rep. 2017;7:45259. doi:10.1038/srep45259.
- Li D, Wang L, Maziuk BF, Yao X, Wolozin B, Cho YK. Directed evolution of a picomolar-affinity, high-specificity antibody targeting phosphorylated tau. J Biol Chem. 2018;293:12081–94. doi:10.1074/jbc.RA118.003557.
- Tiller KE, Li L, Kumar S, Julian MC, Garde S, Tessier PM. Arginine mutations in antibody complementarity-determining regions display context-dependent affinity/specificity trade-offs. J Biol Chem. 2017;292:16638–52. doi:10.1074/jbc.M117.783837.
- Wu S-J, Luo J, O’Neil KT, Kang J, Lacy ER, Canziani G, Baker A, Huang M, Tang QM, Raju TS, et al. Structure-based engineering of a monoclonal antibody for improved solubility. Protein Engineering Design and Selection. 2010;23(8):643–51. doi:10.1093/protein/gzq037.
- Houlihan G, Gatti-Lafranconi P, Lowe D, Hollfelder F. Directed evolution of anti-HER2 DARPins by SNAP display reveals stability/function trade-offs in the selection process. Protein Eng, Design Sel. 2015;28:269–79. doi:10.1093/protein/gzv029.
- Kaleli NE, Karadag M, Kalyoncu S. Phage display derived therapeutic antibodies have enriched aliphatic content: insights for developability issues. Proteins: Struct, Funct, Bioinf. 2019;87:607–18. doi:10.1002/prot.25685.
- Spencer S, Bethea D, Raju TS, Giles-Komar J, Feng Y. Solubility evaluation of murine hybridoma antibodies. MAbs. 2012;4:319–25. doi:10.4161/mabs.19869.
- Bradbury AR, Sidhu S, Dübel S, McCafferty J. Beyond natural antibodies: the power of in vitro display technologies. Nat Biotechnol. 2011;29:245–54. doi:10.1038/nbt.1791.
- Nilvebrant J, Tessier PM, Sidhu SS. Engineered Autonomous Human Variable Domains. Curr Pharm Des. 2016;22:6527–37. doi:10.2174/1381612822666160921143011.
- Baran D, Pszolla MG, Lapidoth GD, Norn C, Dym O, Unger T, et al. Principles for computational design of binding antibodies. Proc Nat Acad Sci. 2017;201707171.
- Sharma VK, Patapoff TW, Kabakoff B, Pai S, Hilario E, Zhang B, Li C, Borisov O, Kelley RF, Chorny I, et al. In silico selection of therapeutic antibodies for development: viscosity, clearance, and chemical stability. Proc Nat Acad Sci. 2014;111(52):18601–06. doi:10.1073/pnas.1421779112.
- Tomar DS, Li L, Broulidakis MP, Luksha NG, Burns CT, Singh SK, Kumar S. In-silico prediction of concentration-dependent viscosity curves for monoclonal antibody solutions. mAbs. 2017;9:476–89. doi:10.1080/19420862.2017.1285479.
- Agrawal NJ, Helk B, Kumar S, Mody N, Sathish HA, Samra HS, Buck PM, Li L, Trout BL. Computational tool for the early screening of monoclonal antibodies for their viscosities. mAbs. 2016;8(1):43–48. doi:10.1080/19420862.2015.1099773.
- Apgar JR, Tam ASP, Sorm R, Moesta S, King AC, Yang H, Kelleher K, Murphy D, D’Antona AM, Yan G, et al. Modeling and mitigation of high-concentration antibody viscosity through structure-based computer-aided protein design. PLOS ONE. 2020;15(5):e0232713. doi:10.1371/journal.pone.0232713.
- Xu Y, Roach W, Sun T, Jain T, Prinz B, Yu T-Y, et al. Addressing polyspecificity of antibodies selected from an in vitro yeast presentation system: a FACS-based, high-throughput selection and analytical tool. Protein Eng, Design Sel. 2013;26:663–70.
- Avery LB, Wade J, Wang M, Tam A, King A, Piche-Nicholas N, et al. Establishing in vitro in vivo correlations to screen monoclonal antibodies for physicochemical properties related to favorable human pharmacokinetics. MAbs. 2018;10:244–55. doi:10.1080/19420862.2017.1417718.
- Hötzel I, Theil FP, Bernstein LJ, Prabhu S, Deng R, Quintana L, et al. A strategy for risk mitigation of antibodies with fast clearance. MAbs. 2012;4:753–60. doi:10.4161/mabs.22189.
- Datta-Mannan A, Thangaraju A, Leung D, Tang Y, Witcher DR, Lu J, et al. Balancing charge in the complementarity-determining regions of humanized mAbs without affecting pI reduces non-specific binding and improves the pharmacokinetics. MAbs. 2015;7:483–93. doi:10.1080/19420862.2015.1016696.
- Li B, Tesar D, Boswell CA, Cahaya HS, Wong A, Zhang J, Meng YG, Eigenbrot C, Pantua H, Diao J, et al. Framework selection can influence pharmacokinetics of a humanized therapeutic antibody through differences in molecule charge. MAbs. 2014;6(5):1255–64. doi:10.4161/mabs.29809.
- Igawa T, Tsunoda H, Tachibana T, Maeda A, Mimoto F, Moriyama C, Nanami M, Sekimori Y, Nabuchi Y, Aso Y, et al. Reduced elimination of IgG antibodies by engineering the variable region. Protein Eng Des Sel. 2010;23(5):385–92. doi:10.1093/protein/gzq009.
- Rabia LA, Zhang Y, Ludwig SD, Julian MC, Tessier PM. Net charge of antibody complementarity-determining regions is a key predictor of specificity. Protein Eng Des Sel. 2018;31:409–18. doi:10.1093/protein/gzz002.
- Raybould MIJ, Marks C, Krawczyk K, Taddese B, Nowak J, Lewis AP, Bujotzek A, Shi J, Deane CM. Five computational developability guidelines for therapeutic antibody profiling. Proc Nat Acad Sci. 2019;116(10):4025–30. doi:10.1073/pnas.1810576116.
- Obrezanova O, Arnell A, de la Cuesta RG, Berthelot ME, Gallagher TR, Zurdo J, Stallwood Y. Aggregation risk prediction for antibodies and its application to biotherapeutic development. MAbs. 2015;7(2):352–63. doi:10.1080/19420862.2015.1007828.
- Lauer TM, Agrawal NJ, Chennamsetty N, Egodage K, Helk B, Trout BL. Developability index: a rapid in silico tool for the screening of antibody aggregation propensity. J Pharm Sci. 2012;101:102–15. doi:10.1002/jps.22758.
- Tsuchiya Y, Mizuguchi K. The diversity of H3 loops determines the antigen-binding tendencies of antibody CDR loops. Protein Sci. 2016;25:815–25. doi:10.1002/pro.2874.
- Kizhedath A, Karlberg M. Cross-interaction chromatography-based QSAR model for early-stage screening to facilitate enhanced developability of monoclonal antibody therapeutics. Biotechnol J. 2019;14:e1800696. doi:10.1002/biot.201800696.
- Jacobs SA, Wu SJ, Feng Y, Bethea D, O’Neil KT. Cross-interaction chromatography: a rapid method to identify highly soluble monoclonal antibody candidates. Pharm Res. 2010;27:65–71. doi:10.1007/s11095-009-0007-z.
- Tessier PM, Sandler SI, Lenhoff AM. Direct measurement of protein osmotic second virial cross coefficients by cross-interaction chromatography. Protein Sci. 2004;13:1379–90. doi:10.1110/ps.03419204.
- Teske CA, Blanch HW, Prausnitz JM. Chromatographic measurement of interactions between unlike proteins. Fluid Phase Equilib. 2004;219:139–48. doi:10.1016/j.fluid.2004.01.025.
- Kelly RL, Sun T, Jain T, Caffry I, Yu Y, Cao Y, et al. High throughput cross-interaction measures for human IgG1 antibodies correlate with clearance rates in mice. MAbs. 2015;7:770–77. doi:10.1080/19420862.2015.1043503.
- Perchiacca JM, Bhattacharya M, Tessier PM. Mutational analysis of domain antibodies reveals aggregation hotspots within and near the complementarity determining regions. Proteins. 2011;79:2637–47. doi:10.1002/prot.23085.
- Perchiacca JM, Ladiwala AR, Bhattacharya M, Tessier PM. Aggregation-resistant domain antibodies engineered with charged mutations near the edges of the complementarity-determining regions. Protein Eng Des Sel. 2012;25:591–601. doi:10.1093/protein/gzs042.
- Vázquez-Rey M, Lang DA. Aggregates in monoclonal antibody manufacturing processes. Biotechnol Bioeng. 2011;108:1494–508. doi:10.1002/bit.23155.
- Ratanji KD, Derrick JP, Dearman RJ, Kimber I. Immunogenicity of therapeutic proteins: influence of aggregation. J Immunotoxicol. 2014;11:99–109. doi:10.3109/1547691X.2013.821564.
- Moussa EM, Panchal JP, Moorthy BS, Blum JS, Joubert MK, Narhi LO, et al. Immunogenicity of therapeutic protein aggregates. J Pharm Sci. 2016;105:417–30. doi:10.1016/j.xphs.2015.11.002.
- Song Y, Yu D, Mayani M, Mussa N, Li ZJ. Monoclonal antibody higher order structure analysis by high throughput protein conformational array. MAbs. 2018;10:397–405. doi:10.1080/19420862.2017.1421880.
- Ebo JS, Saunders JC, Devine PWA, Gordon AM, Warwick AS, Schiffrin B, Chin SE, England E, Button JD, Lloyd C, et al. An in vivo platform to select and evolve aggregation-resistant proteins. Nat Commun. 2020;11:1816. doi:10.1038/s41467-020-15667-1.
- Zidar M, Šušterič A, Ravnik M, Kuzman D. High throughput prediction approach for monoclonal antibody aggregation at high concentration. Pharm Res. 2017;34:1831–39. doi:10.1007/s11095-017-2191-6.
- Li X, Geng SB, Chiu ML, Saro D, Tessier PM. High-throughput assay for measuring monoclonal antibody self-association and aggregation in serum. Bioconjug Chem. 2015;26:520–28. doi:10.1021/acs.bioconjchem.5b00010.
- Fernandez-Escamilla A-M, Rousseau F, Schymkowitz J, Serrano L. Prediction of sequence-dependent and mutational effects on the aggregation of peptides and proteins. Nat Biotechnol. 2004;22:1302–06. doi:10.1038/nbt1012.
- Van Durme J, De Baets G, Van Der Kant R, Ramakers M, Ganesan A, Wilkinson H, Gallardo R, Rousseau F, Schymkowitz J. Solubis: a webserver to reduce protein aggregation through mutation. Protein Eng Des Sel. 2016;29:285–89. doi:10.1093/protein/gzw019.
- De Baets G, Van Durme J, van der Kant R, Schymkowitz J, Rousseau F. Solubis: optimize your protein. Bioinformatics. 2015;31:2580–82. doi:10.1093/bioinformatics/btv162.
- Chennamsetty N, Voynov V, Kayser V, Helk B, Trout BL. Design of therapeutic proteins with enhanced stability. Proc Nat Acad Sci. 2009;106:11937–42. doi:10.1073/pnas.0904191106.
- van der Kant R, Karow-Zwick AR, Van Durme J, Blech M, Gallardo R, Seeliger D, Aßfalg K, Baatsen P, Compernolle G, Gils A, et al. Prediction and reduction of the aggregation of monoclonal antibodies. J Mol Biol. 2017;429(8):1244–61. doi:10.1016/j.jmb.2017.03.014.
- Guerois R, Serrano L. The SH3-fold family: experimental evidence and prediction of variations in the folding pathways. J Mol Biol. 2000;304:967–82. doi:10.1006/jmbi.2000.4234.
- Courtois F, Agrawal NJ, Lauer TM, Trout BL. Rational design of therapeutic mAbs against aggregation through protein engineering and incorporation of glycosylation motifs applied to bevacizumab. MAbs. 2016;8:99–112. doi:10.1080/19420862.2015.1112477.
- Sormanni P, Aprile FA, Vendruscolo M. The CamSol method of rational design of protein mutants with enhanced solubility. J Mol Biol. 2015;427:478–90. doi:10.1016/j.jmb.2014.09.026.
- Sormanni P, Amery L, Ekizoglou S, Vendruscolo M, Popovic B. Rapid and accurate in silico solubility screening of a monoclonal antibody library. Sci Rep. 2017;7:8200. doi:10.1038/s41598-017-07800-w.
- Smialowski P, Doose G, Torkler P, Kaufmann S, Frishman D. PROSO II – a new method for protein solubility prediction. FEBS J. 2012;279:2192–200. doi:10.1111/j.1742-4658.2012.08603.x.
- Khurana S, Rawi R, Kunji K, Chuang G-Y, Bensmail H, Mall R. DeepSol: a deep learning framework for sequence-based protein solubility prediction. Bioinformatics. 2018;34:2605–13. doi:10.1093/bioinformatics/bty166.
- Rawi R, Mall R, Kunji K, Shen C-H, Kwong PD, Chuang G-Y. PaRSnIP: sequence-based protein solubility prediction using gradient boosting machine. Bioinformatics. 2017;34:1092–98. doi:10.1093/bioinformatics/btx662.
- Raimondi D, Orlando G, Fariselli P, Moreau Y. Insight into the protein solubility driving forces with neural attention. PLoS Comput Biol. 2020;16:e1007722. doi:10.1371/journal.pcbi.1007722.
- Paladin L, Piovesan D, Tosatto SCE. SODA: prediction of protein solubility from disorder and aggregation propensity. Nucleic Acids Res. 2017;45:W236–w40. doi:10.1093/nar/gkx412.
- Yang Y, Niroula A, Shen B, Vihinen M. PON-Sol: prediction of effects of amino acid substitutions on protein solubility. Bioinformatics. 2016;32:2032–34. doi:10.1093/bioinformatics/btw066.
- Dobson CL, Devine PWA, Phillips JJ, Higazi DR, Lloyd C, Popovic B, Arnold J, Buchanan A, Lewis A, Goodman J, et al. Engineering the surface properties of a human monoclonal antibody prevents self-association and rapid clearance in vivo. Sci Rep. 2016;6:38644. doi:10.1038/srep38644.
- Ovacik M, Lin K. Tutorial on monoclonal antibody pharmacokinetics and its considerations in early development. Clin Transl Sci. 2018;11:540–52. doi:10.1111/cts.12567.
- Kraft TE, Richter WF, Emrich T, Knaupp A, Schuster M, Wolfert A, et al. Heparin chromatography as an in vitro predictor for antibody clearance rate through pinocytosis. MAbs. 2020;12:1683432. doi:10.1080/19420862.2019.1683432.
- Chung S, Nguyen V, Lin YL, Lafrance-Vanasse J, Scales SJ, Lin K, Deng R, Williams K, Sperinde G, Li JJ, et al. An in vitro FcRn- dependent transcytosis assay as a screening tool for predictive assessment of nonspecific clearance of antibody therapeutics in humans. MAbs. 2019;11:942–55. doi:10.1080/19420862.2019.1605270.
- Noguchi Y, Ozeki K, Akita H. Pharmacokinetic prediction of an antibody in mice based on an in vitro cell-based approach using target receptor-expressing cells. Sci Rep. 2020;10:16268. doi:10.1038/s41598-020-73255-1.
- Roopenian DC, Akilesh S. FcRn: the neonatal Fc receptor comes of age. Nat Rev Immunol. 2007;7:715–25. doi:10.1038/nri2155.
- Pyzik M, Sand KMK, Hubbard JJ, Andersen JT, Sandlie I, Blumberg RS. The neonatal Fc Receptor (FcRn): a misnomer? Front Immunol. 2019;10:10. doi:10.3389/fimmu.2019.01540.
- Schoch A, Kettenberger H, Mundigl O, Winter G, Engert J, Heinrich J, et al. Charge-mediated influence of the antibody variable domain on FcRn-dependent pharmacokinetics. Proc Natl Acad Sci U S A. 2015;112:5997–6002. doi:10.1073/pnas.1408766112.
- Chung S, Lin YL, Nguyen V, Kamen L, Zheng K, Vora B, et al. Development of a label-free FcRn-mediated transcytosis assay for in vitro characterization of FcRn interactions with therapeutic antibodies and Fc-fusion proteins. J Immunol Methods. 2018;462:101–05. doi:10.1016/j.jim.2018.07.004.
- Pyzik M, Rath T, Lencer WI, Baker K, Blumberg RS. FcRn: the architect behind the immune and nonimmune functions of IgG and albumin. J Immunol. 2015;194:4595–603. doi:10.4049/jimmunol.1403014.
- Glassman PM, Balthasar JP. Physiologically-based modeling of monoclonal antibody pharmacokinetics in drug discovery and development. Drug Metab Pharmacokinet. 2019;34:3–13. doi:10.1016/j.dmpk.2018.11.002.
- Jones HM, Zhang Z, Jasper P, Luo H, Avery LB, King LE, et al. A physiologically-based pharmacokinetic model for the prediction of monoclonal antibody pharmacokinetics from in vitro data. CPT: Pharm Syst Pharmacol. 2019;8:738–47.
- Datta-Mannan A, Estwick S, Zhou C, Choi H, Douglass NE, Witcher DR, Lu J, Beidler C, Millican R. Influence of physiochemical properties on the subcutaneous absorption and bioavailability of monoclonal antibodies. MAbs. 2020;12(1):1770028. doi:10.1080/19420862.2020.1770028.
- Bumbaca Yadav D, Sharma VK, Boswell CA, Hotzel I, Tesar D, Shang Y, Ying Y, Fischer SK, Grogan JL, Chiang EY, et al. Evaluating the use of antibody variable region (Fv) charge as a risk assessment tool for predicting typical cynomolgus monkey pharmacokinetics. J Biol Chem. 2015;290:29732–41. doi:10.1074/jbc.M115.692434.
- Khawli LA, Goswami S, Hutchinson R, Kwong ZW, Yang J, Wang X, Yao Z, Sreedhara A, Cano T, Tesar DB, et al. Charge variants in IgG1: isolation, characterization, in vitro binding properties and pharmacokinetics in rats. MAbs. 2010;2(6):613–24. doi:10.4161/mabs.2.6.13333.
- Zheng Y, Tesar DB, Benincosa L, Birnböck H, Boswell CA, Bumbaca D, Cowan KJ, Danilenko DM, Daugherty AL, Fielder PJ, et al. Minipig as a potential translatable model for monoclonal antibody pharmacokinetics after intravenous and subcutaneous administration. MAbs. 2012;4(2):243–55. doi:10.4161/mabs.4.2.19387.
- Datta-Mannan A, Lu J, Witcher DR, Leung D, Tang Y, Wroblewski VJ. The interplay of non-specific binding, target-mediated clearance and FcRn interactions on the pharmacokinetics of humanized antibodies. MAbs. 2015;7:1084–93. doi:10.1080/19420862.2015.1075109.
- Pendley C, Schantz A, Wagner C. Immunogenicity of therapeutic monoclonal antibodies. Curr Opin Mol Ther. 2003;5:172–79.
- Lonberg N. Human antibodies from transgenic animals. Nat Biotechnol. 2005;23:1117–25. doi:10.1038/nbt1135.
- Clavero-Álvarez A, Di Mambro T, Perez-Gaviro S, Magnani M, Bruscolini P. Humanization of antibodies using a statistical inference approach. Sci Rep. 2018;8:14820. doi:10.1038/s41598-018-32986-y.
- Venkataramani S, Ernst R, Derebe MG, Wright R, Kopenhaver J, Jacobs SA, Singh S, Ganesan R. In pursuit of stability enhancement of a prostate cancer targeting antibody derived from a transgenic animal platform. Sci Rep. 2020;10:9722. doi:10.1038/s41598-020-66636-z.
- Smith GP. Filamentous fusion phage: novel expression vectors that display cloned antigens on the virion surface. Science. 1985;228:1315–17. doi:10.1126/science.4001944.
- Nilvebrant J, Sidhu SS. Construction of synthetic antibody phage-display libraries. Methods Mol Biol. 2018;1701:45–60.
- Setliff I, Shiakolas AR, Pilewski KA, Murji AA, Mapengo RE, Janowska K, Richardson S, Oosthuysen C, Raju N, Ronsard L, et al. High-throughput mapping of B cell receptor sequences to antigen specificity. Cell. 2019;179(7):1636–46.e15. doi:10.1016/j.cell.2019.11.003.
- Goldstein LD, Chen Y-J-J, Wu J, Chaudhuri S, Hsiao Y-C, Schneider K, Hoi KH, Lin Z, Guerrero S, Jaiswal BS, et al. Massively parallel single-cell B-cell receptor sequencing enables rapid discovery of diverse antigen-reactive antibodies. Commun Biol. 2019;2:304. doi:10.1038/s42003-019-0551-y.
- Ouisse L-H, Gautreau-Rolland L, Devilder M-C, Osborn M, Moyon M, Visentin J, et al. Antigen-specific single B cell sorting and expression-cloning from immunoglobulin humanized rats: a rapid and versatile method for the generation of high affinity and discriminative human monoclonal antibodies. BMC Biotechnol. 2017;17:3.
- Sethu S, Govindappa K, Alhaidari M, Pirmohamed M, Park K, Sathish J. Immunogenicity to biologics: mechanisms, prediction and reduction. Arch Immunol Ther Exp (Warsz). 2012;60:331–44.
- Boehncke WH, Brembilla NC. Immunogenicity of biologic therapies: causes and consequences. Expert Rev Clin Immunol. 2018;14:513–23. doi:10.1080/1744666X.2018.1468753.
- De Groot AS, Scott DW. Immunogenicity of protein therapeutics. Trends Immunol. 2007;28:482–90. doi:10.1016/j.it.2007.07.011.
- Haraoui B, Cameron L, Ouellet M, White B. Anti-infliximab antibodies in patients with rheumatoid arthritis who require higher doses of infliximab to achieve or maintain a clinical response. J Rheumatol. 2006;33:31–36.
- Karle A, Spindeldreher S, Kolbinger F. Secukinumab, a novel anti-IL-17A antibody, shows low immunogenicity potential in human in vitro assays comparable to other marketed biotherapeutics with low clinical immunogenicity. MAbs. 2016;8:536–50. doi:10.1080/19420862.2015.1136761.
- Sekiguchi N, Kubo C, Takahashi A, Muraoka K, Takeiri A, Ito S, Yano M, Mimoto F, Maeda A, Iwayanagi Y, et al. MHC-associated peptide proteomics enabling highly sensitive detection of immunogenic sequences for the development of therapeutic antibodies with low immunogenicity. MAbs. 2018;10(8):1168–81. doi:10.1080/19420862.2018.1518888.
- Joubert MK, Deshpande M, Yang J, Reynolds H, Bryson C, Fogg M, Baker MP, Herskovitz J, Goletz TJ, Zhou L, et al. Use of in vitro assays to assess immunogenicity risk of antibody-based biotherapeutics. PLoS One. 2016;11(8):e0159328. doi:10.1371/journal.pone.0159328.
- Spindeldreher S, Karle A, Correia E, Tenon M, Gottlieb S, Huber T, Maillere B, Kolbinger F. T cell epitope mapping of secukinumab and ixekizumab in healthy donors. MAbs. 2020;12(1):1707418. doi:10.1080/19420862.2019.1707418.
- Singh H, Raghava GP. ProPred: prediction of HLA-DR binding sites. Bioinformatics. 2001;17:1236–37. doi:10.1093/bioinformatics/17.12.1236.
- Yogurtcu ON, Sauna ZE, McGill JR, Tegenge MA, Yang H. TCPro: an in silico risk assessment tool for biotherapeutic protein immunogenicity. AAPS J. 2019;21:96. doi:10.1208/s12248-019-0368-0.
- Choi Y, Verma D, Griswold KE, Bailey-Kellogg C. EpiSweep: computationally driven reengineering of therapeutic proteins to reduce immunogenicity while maintaining function. Methods Mol Biol. 2017;1529:375–98.
- Salvat RS, Choi Y, Bishop A, Bailey-Kellogg C, Griswold KE. Protein deimmunization via structure-based design enables efficient epitope deletion at high mutational loads. Biotechnol Bioeng. 2015;112:1306–18. doi:10.1002/bit.25554.
- Salvat RS, Parker AS, Guilliams A, Choi Y, Bailey-Kellogg C, Griswold KE. Computationally driven deletion of broadly distributed T cell epitopes in a biotherapeutic candidate. Cell Mol Life Sci. 2014;71:4869–80. doi:10.1007/s00018-014-1652-x.
- Salvat RS, Verma D, Parker AS, Kirsch JR, Brooks SA, Bailey-Kellogg C, et al. Computationally optimized deimmunization libraries yield highly mutated enzymes with low immunogenicity and enhanced activity. Proc Natl Acad Sci USA. 2017;114:E5085–e93. doi:10.1073/pnas.1621233114.