ABSTRACT
Animal-derived antibody sources, particularly, transgenic mice that are engineered with human immunoglobulin loci, along with advanced antibody generation technology platforms have facilitated the discoveries of human antibody therapeutics. For example, isolation of antigen-specific B cells, microfluidics, and next-generation sequencing have emerged as powerful tools for identifying and developing monoclonal antibodies (mAbs). These technologies enable not only antibody drug discovery but also lead to the understanding of B cell biology, immune mechanisms and immunogenetics of antibodies. In this perspective article, we discuss the scientific merits of animal immunization combined with advanced methods for antibody generation as compared to animal-free alternatives through in-vitro-generated antibody libraries. The knowledge gained from animal-derived antibodies concerning the recombinational diversity, somatic hypermutation patterns, and physiochemical properties is found more valuable and prerequisite for developing in vitro libraries, as well as artificial intelligence/machine learning methods to discover safe and effective mAbs.
Recently, Gray et al.Citation1 raised scientific and ethical concerns toward animal immunization for antibody generation, and claimed that non-animal derived universal or naive libraries can generate antibodies with greater versatility and reproducibility than immunization-based methods. Scientific concerns were mainly raised on the use of unsequenced animal-derived polyclonals and hybridomas, which are now commonly replaced with monoclonal antibodies (mAbs) and well-characterized hybridomas, respectively, for affinity reagents and therapeutic applications. Further in their correspondence,Citation1 Gray et al. stated that non-animal-derived universal antibody libraries contain an enormous repertoire of structurally diverse antibody genes that is equal or greater than that of a naive immune system, from which binders against essentially any target can be generated. In our view, however, mAbs generated from animal-derived methods are highly diverse, antigen-specific, developable and unmatched to those that are derived from the in vitro methods. This is because in vivo-generated mAbs evolve through highly orchestrated B cell immune mechanisms, such as clonal selection specific to antigens with diverse lineages and somatic hypermutation in germinal center B cells, particularly, for complex antigens.Citation2 In addition, other secondary mechanisms of diversificationCitation3 and rare chromosomal integrations into variable regionsCitation4 also contribute to antibody diversification that cannot be recapitulated by in vitro methods. Specifically, hybridoma technology has a unique advantage in retaining their native heavy and light chain paired assembly, and consequently high solubility.Citation5 Further, technological advances have blurred species boundaries as the hybridoma approach was made widely applicable across phylogenetically distinct species.Citation6 This may have an important application in the isolation of mAbs against human targets that could be otherwise limited by self-tolerance to mammalian-conserved epitopes.Citation7
In-vitro display-derived libraries cannot yet be regarded as universal, but only as complementary to animal-derived methods. For example, Saggy et al.Citation8 performed a comparative analysis that evaluated hits from the in vitro phage display vs. next-generation sequencing (NGS) methods using antibodies produced by B cells from immunized mice. Remarkably, they found that phage display hits were often low-abundance sequences in the NGS, whereas NGS-derived high-abundance sequences did not express well in the phage, and thus were not recovered. In another study, it was shown that phage display and hybridoma methods yield antibodies with distinct mechanisms and epitopes.Citation9 Therefore, these studies demonstrated that, while both the in vivo and in vitro strategies could result in antigen-specific mAbs, they were quite complementary in terms of sequences, targeted epitopes, and functions.
Furthermore, among several in vitro phage display-derived human antibodies approved by the US Food and Drug Administration (FDA),Citation10,Citation11 adalimumab (Humira®) was the first, and it became the best-selling antibody drug on the market. However, importantly, Humira® was discovered by a process known as “guided selection” using a murine mAb as the original template.Citation12 Most of the mAbs currently approved by the FDA are from hybridoma technology derived either from wild type or more recently using human immunoglobulin (Ig) transgenic mice, and the list also includes the first immunization-derived, humanized nanobody caplacizumab.Citation10 At one instance, it was reported that phage display-derived therapeutic antibodies are enriched with aliphatic contents along antibody loops and exhibit higher aggregation and poly-specificity compared to non-phage display-derived antibodies.Citation13 The successful development of any antibody therapeutic, whether animal-derived or non-animal-derived, ultimately depends on key properties such as manufacturability and clinical tolerability of the molecules. The larger number of approved animal-derived antibodies are proven to have these properties as compared to in vitro-derived antibodies.Citation14
Gray et al.Citation1 also viewed animal immunization as the tip of an antibody iceberg and in vitro recombinant antibody generation methods as larger submerged fractions. In doing so, they largely undervalued scientific merits and recent technological innovations that have greatly revolutionized immunization-based methods and enabled the exploration of the antibody repertoire space (). Mainly, human immunoglobulin transgenic mice and technological developments, including microfluidic chip-based hybridomas,Citation15 antigen-specific single B cell isolation,Citation16–18 single-cell droplet microfluidic screening for antigen-specific antibodies,Citation19,Citation20 natively paired immune libraries,Citation21 and NGS-based immune repertoire mining,Citation22 have allowed a more efficient sampling and capturing of the animal-derived antigen-specific antibody repertoire landscape, which has deepened our understanding of antibody biology. Particularly, the large-scale natively paired VH-VL antibody discovery technologiesCitation23–25 have the capacity to impact antibody biological developments. These technologies have enabled merging the benefits of animal immunization with the power of display library screening and human antibody repertoire mining. More recently, we established the use of small volumes of blood from immunized mice to isolate antigen-specific antibodies for potential therapeutic use (unpublished data). Such a cutting-edge antibody discovery approach can be useful in generating antibodies to multiple antigens using the same cohort of mice, thereby potentially restricting the number of animals needed. All these indicate that there is a universe of possibilities yet to be explored in animal-derived antibody repertoire using new technologies.
Figure 1. Advanced technologies expose the vastness of animal-derived antibody repertoire. Recent developments using humanized mouse, advanced hybridomas, isolation of antigen specific bulk and single B cells, immunized display libraries, droplet microfluidics technique and immune repertoire data mining through NGS have paved the way for capturing the expanding universe of animal-derived antibodies that are schematically shown as isolated, clustered and networks of dots within the growing spheres
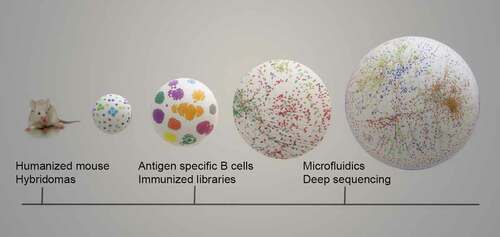
Recently, in silico rational design of antibodies in a modularCitation26 and epitope-specific mannerCitation27 and computational method for immune repertoire miningCitation28 have emerged as third-generation antibody discovery methods. These newly developed in silico methods have utilized the sequence and structural information from antibodies derived from immunization and in vitro methods. More recently, immune organoids from human tonsils and other lymphoid tissues have been developed with a potential for the discovery of antigen-specific antibodies, mimicking key germinal center features including somatic hypermutation and affinity maturation.Citation29 We expect that artificial intelligence (AI)- and machine learning (ML)-based methodsCitation30–32 could essentially exploit the best of both worlds of in vivo- and in vitro-generated methods, large-scale naïve and antigen-specific antibody sequence and structure data,Citation33–35 knowledge of immune repertoire and literacy,Citation36–38 help design feature-controlled antibody libraries and developable antibodies,Citation39,Citation40 which, in turn, would ultimately solve scientific and ethical problems in antibody generation.
In conclusion, any advanced biomedical scientific research in general can raise ethical concerns when involving the in vivo use of animals or patients as data subjects. As of now, animal-free antibody universal libraries exist only as utopian alternatives to immunization methods and are neither well-defined nor matured enough for the replacement of animal immunization. In contrast, there has been a substantial and concerted effort in academic and biopharmaceutical research communities to improve immunization strategies. In this regard, DNA-based immunization has evolved as a powerful technology platform for mAb generation using animals.Citation41 In addition, use of animals has benefited the development and validation of mRNA immunization,Citation42 which has contributed to the successful development of COVID-19 vaccines. Several anti-SARS-CoV-2 neutralizing human mAbs that are now in clinical studies were identified from immunization strategies, such as VelocImmune® (Regeneron), and through use of convalescent blood samples from patients.Citation43 Thus, immunization-based methods along with the evolution and advent of new technologies will lead to the rapid identification and generation of mAbs in the areas of therapeutics and other applications. Because the knowledge of complete immunogenetic diversity and other characteristics required for developing large, universal in vitro libraries are not yet substantiated and still in its infancy, immunization-dependent antibody generation will continue to be a powerful method that can complement and co-exist with in vitro and in silico methods. We envisage that in the future, advanced experimental and AI/ML-enabled technologies may merge the unique capabilities of in vivo, in vitro and in silico methods for antibody discovery.
Abbreviations
AI, artificial intelligence; FDA, Food and Drug Administration; Ig, immunoglobulin; mAb, monoclonal antibody; ML, machine learning; NGS, next-generation sequencing
Author contributions
All authors discussed and contributed to the manuscript.
Competing interests
All authors are Sanofi employees, and may hold shares and/or stock options in the company. The company had no role in this manuscript.
Acknowledgments
We thank Sarah Tao for critical reading of the manuscript, Dietmar Hoffmann and Ally Hatton for the internal review. We also thank Rebecca Sendak, Thomas Bouquin and Thierry Decelle for their feedback and suggestions on the manuscript.
References
- Gray A, Bradbury ARM, Knappik A, Pluckthun A, Borrebaeck CAK, Dubel S. Animal-free alternatives and the antibody iceberg. Nat Biotechnol. 2020;38(11):1234–4. doi:10.1038/s41587-020-0687-9.
- Finney J, Yeh CH, Kelsoe G, Kuraoka M. Germinal center responses to complex antigens. Immunol Rev. 2018;284(1):42–50. doi:10.1111/imr.12661.
- Briney BS, Crowe JE Jr. Secondary mechanisms of diversification in the human antibody repertoire. Front Immunol. 2013;4:42. doi:10.3389/fimmu.2013.00042.
- Tan J, Pieper K, Piccoli L, Abdi A, Perez MF, Geiger R, Tully CM, Jarrossay D, Ndungu FM, Wambua J, et al. A LAIR1 insertion generates broadly reactive antibodies against malaria variant antigens. Nature. 2016;529:105–09. doi:10.1038/nature16450.
- Spencer S, Bethea D, Raju TS, Giles-Komar J, Feng Y. Solubility evaluation of murine hybridoma antibodies. MAbs. 2012;4(3):319–25. doi:10.4161/mabs.19869.
- Parray HA, Shukla S, Samal S, Shrivastava T, Ahmed S, Sharma C, Kumar R. Hybridoma technology a versatile method for isolation of monoclonal antibodies, its applicability across species, limitations, advancement and future perspectives. Int Immunopharmacol. 2020;85:106639. doi:10.1016/j.intimp.2020.106639.
- Gjetting T, Gad M, Frohlich C, Lindsted T, Melander MC, Bhatia VK, Grandal MM, Dietrich N, Uhlenbrock F, Galler GR, et al. Sym021, a promising anti-PD1 clinical candidate antibody derived from a new chicken antibody discovery platform. MAbs. 2019;11(4):666–80. doi:10.1080/19420862.2019.1596514.
- Saggy I, Wine Y, Shefet-Carasso L, Nahary L, Georgiou G, Benhar I. Antibody isolation from immunized animals: comparison of phage display and antibody discovery via V gene repertoire mining. Protein Eng Des Sel. 2012;25(10):539–49. doi:10.1093/protein/gzs060.
- Rossant CJ, Carroll D, Huang L, Elvin J, Neal F, Walker E, Benschop JJ, Kim EE, Barry ST, Vaughan TJ. Phage display and hybridoma generation of antibodies to human CXCR2 yields antibodies with distinct mechanisms and epitopes. MAbs. 2014;6(6):1425–38. doi:10.4161/mabs.34376.
- Lu RM, Hwang YC, Liu IJ, Lee CC, Tsai HZ, Li HJ, Wu HC. Development of therapeutic antibodies for the treatment of diseases. J Biomed Sci. 2020;27:1.
- Frenzel A, Schirrmann T, Hust M. Phage display-derived human antibodies in clinical development and therapy. MAbs. 2016;8(7):1177–94. doi:10.1080/19420862.2016.1212149.
- Osbourn J, Groves M, Vaughan T. From rodent reagents to human therapeutics using antibody guided selection. Methods. 2005;36(1):61–68. doi:10.1016/j.ymeth.2005.01.006.
- Kaleli NE, Karadag M, Kalyoncu S. Phage display derived therapeutic antibodies have enriched aliphatic content: insights for developability issues. Proteins. 2019;87(7):607–18. doi:10.1002/prot.25685.
- Jain T, Sun T, Durand S, Hall A, Houston NR, Nett JH, Sharkey B, Bobrowicz B, Caffry I, Yu Y, et al. Biophysical properties of the clinical-stage antibody landscape. Proc Natl Acad Sci U S A. 2017;114(5):944–49. doi:10.1073/pnas.1616408114.
- Zhang W, Li R, Jia F, Hu Z, Li Q, Wei Z. A microfluidic chip for screening high-producing hybridomas at single cell level. Lab Chip. 2020;20(21):4043–51. doi:10.1039/D0LC00847H.
- Tiller T. Single B cell antibody technologies. N Biotechnol. 2011;28(5):453–57. doi:10.1016/j.nbt.2011.03.014.
- Scheid JF, Mouquet H, Feldhahn N, Seaman MS, Velinzon K, Pietzsch J, Ott RG, Anthony RM, Zebroski H, Hurley A, et al. Broad diversity of neutralizing antibodies isolated from memory B cells in HIV-infected individuals. Nature. 2009;458:636–40. doi:10.1038/nature07930.
- Wrammert J, Smith K, Miller J, Langley WA, Kokko K, Larsen C, Zheng NY, Mays I, Garman L, Helms C, et al. Rapid cloning of high-affinity human monoclonal antibodies against influenza virus. Nature. 2008;453(7195):667–71. doi:10.1038/nature06890.
- Keating SM, Mizrahi RA, Adams MS, Asensio MA, Benzie E, Carter KP, Chiang Y, Edgar RC, Gautam BK, Gras A, et al. Generation of recombinant hyperimmune globulins from diverse B-cell repertoires. Nat Biotechnol. 2021. doi:10.1038/s41587-021-00894-8.
- Shembekar N, Hu H, Eustace D, Merten CA. Single-cell droplet microfluidic screening for antibodies specifically binding to target cells. Cell Rep. 2018;22(8):2206–15. doi:10.1016/j.celrep.2018.01.071.
- Adler AS, Bedinger D, Adams MS, Asensio MA, Edgar RC, Leong R, Leong J, Mizrahi RA, Spindler MJ, Bandi SR, et al. A natively paired antibody library yields drug leads with higher sensitivity and specificity than a randomly paired antibody library. MAbs. 2018;10(3):431–43. doi:10.1080/19420862.2018.1426422.
- Reddy ST, Ge X, Miklos AE, Hughes RA, Kang SH, Hoi KH, Chrysostomou C, Hunicke-Smith SP, Iverson BL, Tucker PW, et al. Monoclonal antibodies isolated without screening by analyzing the variable-gene repertoire of plasma cells. Nat Biotechnol. 2010;28(9):965–69. doi:10.1038/nbt.1673.
- Rajan S, Kierny MR, Mercer A, Wu J, Tovchigrechko A, Wu H, Dall'Acqua WF, Xiao X, Chowdhury PS. Recombinant human B cell repertoires enable screening for rare, specific, and natively paired antibodies. Commun Biol. 2018;1(1):5. doi:10.1038/s42003-017-0006-2.
- Wang B, DeKosky BJ, Timm MR, Lee J, Normandin E, Misasi J, Kong R, McDaniel JR, Delidakis G, Leigh KE, et al. Functional interrogation and mining of natively paired human VH:VL antibody repertoires. Nat Biotechnol. 2018;36(2):152–55. doi:10.1038/nbt.4052.
- Goldstein LD, Chen YJ, Wu J, Chaudhuri S, Hsiao YC, Schneider K, Hoi KH, Lin Z, Guerrero S, Jaiswal BS, et al. Massively parallel single-cell B-cell receptor sequencing enables rapid discovery of diverse antigen-reactive antibodies. Commun Biol. 2019;2(1):304. doi:10.1038/s42003-019-0551-y.
- Sormanni P, Aprile FA, Vendruscolo M. Third generation antibody discovery methods: in silico rational design. Chem Soc Rev. 2018;47(24):9137–57. doi:10.1039/C8CS00523K.
- Nimrod G, Fischman S, Austin M, Herman A, Keyes F, Leiderman O, Hargreaves D, Strajbl M, Breed J, Klompus S, et al. Computational design of epitope-specific functional antibodies. Cell Rep. 2018;25(8):2121–31 e5. doi:10.1016/j.celrep.2018.10.081.
- Richardson E, Galson JD, Kellam P, Kelly DF, Smith SE, Palser A, Watson S, Deane CM. A computational method for immune repertoire mining that identifies novel binders from different clonotypes, demonstrated by identifying anti-pertussis toxoid antibodies. MAbs. 2021;13(1):1869406. doi:10.1080/19420862.2020.1869406.
- Wagar LE, Salahudeen A, Constantz CM, Wendel BS, Lyons MM, Mallajosyula V,Jatt LP, Adamska JZ, Blum LK, Gupta N, et al. Modeling human adaptive immune responses with tonsil organoids. Nat Med. 2021;27(1):125–35. doi:10.1038/s41591-020-01145-0.
- Liu G, Zeng H, Mueller J, Carter B, Wang Z, Schilz J, Horny G, Birnbaum ME, Ewert S, Gifford DK. Antibody complementarity determining region design using high-capacity machine learning. Bioinformatics. 2020;36(7):2126–33. doi:10.1093/bioinformatics/btz895.
- Graves J, Byerly J, Priego E, Makkapati N, Parish SV, Medellin B, Berrondo M. A review of deep learning methods for antibodies. Antibodies (Basel). 2020;9(2):9. doi:10.3390/antib9020012.
- Greiff V, Yaari G, Cowell L. Mining adaptive immune receptor repertoires for biological and clinical information using machine learning. Current Opinion in Systems Biology. 2020;24:109–19. doi:10.1016/j.coisb.2020.10.010.
- DeKosky BJ, Lungu OI, Park D, Johnson EL, Charab W, Chrysostomou C, Kuroda D, Ellington AD, Ippolito GC, Gray JJ, et al. Large-scale sequence and structural comparisons of human naive and antigen-experienced antibody repertoires. Proc Natl Acad Sci U S A. 2016;113(19):E2636–45. doi:10.1073/pnas.1525510113.
- Akbar R, Robert PA, Pavlovic M, Jeliazkov JR, Snapkov I, Slabodkin A, Weber CR, Scheffer L, Miho E, Haff IH, et al. A compact vocabulary of paratope-epitope interactions enables predictability of antibody-antigen binding. Cell Rep. 2021;34(11):108856. doi:10.1016/j.celrep.2021.108856.
- Papageorgiou L, Maroulis D, Chrousos GP, Eliopoulos E, Vlachakis D. Antibody clustering using a machine learning pipeline that fuses genetic, structural, and physicochemical properties. Adv Exp Med Biol. 2020;1194:41–58.
- Csepregi L, Ehling RA, Wagner B, Reddy ST. Immune literacy: reading, writing, and editing adaptive immunity. iScience. 2020;23(9):101519. doi:10.1016/j.isci.2020.101519.
- Marks C, Deane CM. How repertoire data are changing antibody science. J Biol Chem. 2020;295(29):9823–37. doi:10.1074/jbc.REV120.010181.
- Rees AR. Understanding the human antibody repertoire. MAbs. 2020;12(1):1729683. doi:10.1080/19420862.2020.1729683.
- Amimeur T, Shaver JM, Ketchem RR, Taylor JA, Clark RH, Smith J, Van Citters D, Siska CC, Smidt P, Sprague M, et al. Designing feature-controlled humanoid antibody discovery libraries using generative adversarial networks. bioRxiv. 2020;2020:12.024844.
- Shin J-E, Riesselman AJ, Kollasch AW, McMahon C, Simon E, Sander C, Manglik A, Kruse AC, Marks DS. Protein design and variant prediction using autoregressive generative models. bioRxiv. 2021;757252.
- Liu S, Wang S, Lu S. DNA immunization as a technology platform for monoclonal antibody induction. Emerg Microbes Infect. 2016;5(1):e33. doi:10.1038/emi.2016.27.
- Pardi N, Hogan MJ, Porter FW, Weissman D. mRNA vaccines - a new era in vaccinology. Nat Rev Drug Discov. 2018;17:261–79.
- Tuccori M, Ferraro S, Convertino I, Cappello E, Valdiserra G, Blandizzi C, Maggi F, Focosi D. Anti-SARS-CoV-2 neutralizing monoclonal antibodies: clinical pipeline. MAbs. 2020;12(1):1854149. doi:10.1080/19420862.2020.1854149.