ABSTRACT
Theoretical approaches based on dynamical systems theory can provide useful frameworks to guide experiments and analysis techniques when investigating cortical network activity. The notion of phase transitions between qualitatively different kinds of network dynamics has been such a framework inspiring novel approaches to neurophysiological data analysis over the recent years. One particular intriguing hypothesis has been that cortical networks reside in the vicinity of a phase transition. Although the final verdict on this hypothesis is still out, trying to understand cortex dynamics from this viewpoint has recently led to interesting insights on cortical network function with relevance for clinical practice.
Brain activity should neither be completely random nor completely ordered. If the brain were to react with random activity every time it received an incoming stimulus, the interaction with the outside world would likely be incoherent and unpredictable. Similarly, if brain activity were completely ordered or synchronous all the time, there would be no variability in the ways to react and interact with the environment. It is easy to see that both extremes are clearly not appropriate to describe cortical brain function and should be dismissed.
What is more interesting is how exactly networks of neurons such as in the cortex transition from disorder to order. Previous computationalCitation1 and in vitroCitation2 network studies have pointed to effective connectivity, i.e. the degree to which neurons are interconnected and “excite” each other, as a critical player controlling the level of order and disorder in cortical networks. When connectivity is low, network activity is disordered; as neurons become more and more interconnected, a point is reached where order abruptly emerges (). Such a rapid change is well described by what is called a phase transition in physics. For network activity to be neither completely disordered nor completely ordered, neuronal networks have to be operating near such a phase transition - at the transition between an ordered and a disordered phase which is controlled by effective connectivity.
Figure 1. Increasing evidence from computationalCitation1, cell cultureCitation2 and humanCitation3 studies suggests a close link between cortical network synchrony and effective connectivity. As connectivity, or more generally excitability, is increased, network synchrony increases rapidly (schematically depicted by purple line). Long-term monitoring indicates that human cortical networks reside in the vicinity of this synchronization transition (black histogram) whereas AED, on average, shift networks toward lower synchrony (blue histogram). The link between synchrony and excitability allows an approximate mapping of these synchronization changes to excitability (black dotted lines). AEDs shift cortical excitability to lower levels. Conversely, wake moves cortical networks to higher excitability (and synchrony) which is recovered by sleep.
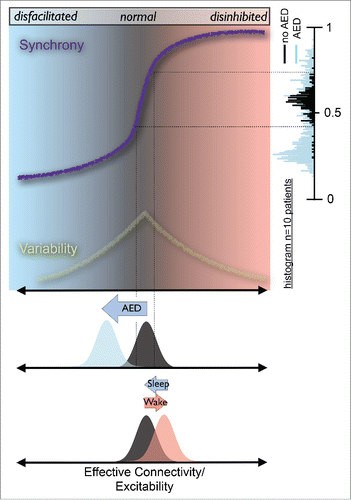
In a recent study,Citation3 we provided evidence that this is actually the case in living human brains. Specifically, we analyzed the amount of order by quantifying the synchrony in cortical networks of epilepsy patients while the number and dosage of antiepileptic drugs (AEDs) were varied. Most AEDs reduce the ways neurons can excite each other by decreasing the excitability of individual neurons, enhancing inhibitory synaptic transmission or inhibiting excitatory synaptic transmission,Citation4 and therefore essentially reduce the effective connectivity in similar ways as reported before.Citation1,2 We found that when no AEDs were administered, networks would, on average, settle somewhere in the middle between order and disorder (, black histogram). When effective connectivity was reduced by AEDs, networks shifted more toward the disordered, asynchronous state (, blue histogram).
These histograms also provide evidence that cortical network activity alternates to some degree between different levels of synchrony (or order). Even when no AEDs were administered, networks fluctuated between states with higher and states with lower synchrony, but on average remained in the vicinity of the transition from low to high order. There has been considerable debate related to the question whether cortical networks are poised at or near a critical phase transition.Citation5-7 In this context, the variability to be observed in the histograms in our work can be interpreted as some indication that physiological cortical networks do not operate at one particular point, such as a critical point in phase space, all the time. Instead they fluctuate while maintaining a certain mean value. In the language of dynamical systems, network dynamics constantly fluctuates and thereby visits sub- and supercritical states while, on average, remains in the vicinity of the transition disorder to order. Without antiepileptic drugs, networks are consequently on average closer to the phase transition, while antiepileptic drugs, on average, drive networks toward the subcritical, disordered regime.
The observed ability of AED to gradually shift network dynamics to different mean synchronization values in human cortical networks can potentially provide further insights as to the particular type of transition governing the progression from disorder to order. An interesting candidate is a transcritical bifurcation. A transcritical bifurcation is characterized by 2 fixed points which interchange stability upon variation of a control parameter.Citation8 In our report,Citation3 the 2 fixed points would correspond to the asynchronous and the synchronous network states, and the control parameter is the effective connectivity or, more generally, excitability. The transition between them can be expected to be comparatively gradual in a transcritical bifurcation which could explain the relatively gradual changes in synchrony upon administration or reduction of AEDs.
Pathological changes in excitability of cortical tissue commonly underlie the initiation and spread of seizure activity in epilepsy patients; monitoring of excitability and controlling its degree using AEDs is of prime importance for clinical care and treatment. To date, however, reliable measures of cortical excitability have been difficult to obtain. Quantitative markers of excitability can potentially guide more objective and individualized treatment approaches and give reliable feedback on the effect of antiepileptic drug treatment in epilepsy patients. The measure of network synchronyCitation3 provides a step toward such an excitability biomarker. By monitoring spontaneous brain activity, the amount of order in the brain can be quantified, providing information about the underlying excitability of brain networks (). Discovery of this mapping between network synchrony and excitability in human brain networks was largely guided by interpreting previous work on computers and cell cultures in the context of dynamical systems theory. It therefore provides a good example of how a theoretical framework such as phase transitions can help uncover findings with interesting implications for the neurological treatment of epilepsy patients as well as for the understanding of the role of sleep.Citation6
Disclosure of potential conflicts of interest
No potential conflicts of interest were disclosed.
Funding
This study was supported by the Intramural Research Program of the National Institute of Mental Health (ZIAMH002797).
References
- Meisel C, Gross T. Adaptive self-organization in a realistic neural network model. Phy Rev E 2009; 80:061917; http://dx.doi.org/10.1103/PhysRevE.80.061917
- Yang H, Shew WL, Roy R, Plenz D. Maximal variability of phase synchrony in cortical networks with neuronal avalanches. J Neurosci 2012; 32:1061-72; PMID:22262904; http://dx.doi.org/10.1523/JNEUROSCI.2771-11.2012
- Meisel C, Schulze-Bonhage A, Freestone D, Cook M, Achermann A, Plenz D. Intrinsic excitability measures track antiepileptic drug action and uncover increasing/decreasing excitability during the wake/sleep cycle. Proc Natl Acad Sci USA 2015; 112:14694-14699; http://dx.doi.org/10.1073/pnas.1513716112
- Bialer M, White HS. Key factors in the discovery and development of new antiepileptic drugs. Nat Rev Drug Discov 2010; 9:68-82; PMID:20043029; http://dx.doi.org/10.1038/nrd2997
- Beggs JM, Plenz D. Neuronal avalanches in neocortical circuits. J Neurosci 2003; 23:11167-77; PMID:14657176
- Meisel C, Olbrich E, Shriki O, Achermann P. Fading signatures of critical brain dynamics during sustained wakefulness in humans. J Neurosci 2013; 33:17363-72; PMID:24174669; http://dx.doi.org/10.1523/JNEUROSCI.1516-13.2013
- Chialvo D. Emergent complex neural dynamics. Nat Phys 2010; 6:744-50; http://dx.doi.org/10.1038/nphys1803
- Strogatz S. Nonlinear dynamics and chaos: with applications to physics, biology, chemistry, and engineering. Boulder: Westview Press, 2001.