ABSTRACT
We previously reported that thousands of transcripts in the mouse and zebrafish significantly increased in abundance in a time series spanning from life to several days after death. Transcript abundances were determined by: calibrating each microarray probe using a dilution series of pooled RNAs, fitting the probe-responses to adsorption models, and back-calculating abundances using the probe signal intensity of a sample and the best fitting model. The accuracy of the abundance measurements was not assessed in our previous study because individual transcript concentrations in the calibration pool were not known. Accurate transcript abundances are highly desired for modeling the dynamics of biological systems and investigating how systems respond to perturbations. In this study, we show that accurate transcript abundances can be determined by calibrating the probes using a calibration pool of transcripts with known concentrations. Instructions for determining accurate transcript abundances using the Gene Meter approach are provided.
High-throughput gene expression measurements are affected by noise originating from the applied technologies (i.e., DNA microarray.Citation1 and sequencingCitation2 To mitigate these problems, researchers use data manipulation and normalization protocols.Citation3 While solving some problems, these protocols create new ones; e.g., different normalizations yield different interpretations.Citation4 As an alternative, the ‘Gene Meter’ (GM) approach calibrates the dose response of a technology with a dilution series of gene or transcript targets.Citation5,Citation6 This article demonstrates the approach applied to DNA microarrays. The same logic is applicable to DNA sequencing, which have been reported earlier.Citation7 The response of each probe is fitted to Freundlich, Langmuir or linear adsorption models, probe-specific parameters are calculated, and the ‘noisy’ or ‘insensitive’ probes are identified and removed from further analysis. The reason adsorption models were used versus general fitting functions (such as a polynomial) is because they have been previously implemented in DNA microarray research and they have a low number of degrees of freedom, which enable assessment of the fit of the experimental data to the model.
Probes that sufficiently fit the best model are retained and later used to calculate the abundance of a specific gene or gene transcript in a biological sample. Normalization of the calibrated output is not required because the derived models take into consideration the nonlinearity of the microarray signal.
In our recent study,Citation8 we reported the relative abundances of transcripts because the calibration models were based on a dilution series of RNAs with unknown transcript abundances. While the transcript abundances from the same gene can be compared in different samples,Citation9 direct comparisons of different genes is not warranted because the abundances used for the calibration are not known. Comparing transcript abundances of multiple genes to one another is highly desired because it could lead to a more thorough understanding of gene regulation in complex biological systems; e.g., gene expression of cancer cells in response to therapeutic treatments. Although not demonstrated yet, accurate determination of transcript abundances of all genes could be possible by calibrating the models using a dilution pool of transcripts that have known abundances.
In this study, our objective was to investigate the accuracy and limitations of DNA microarrays that have been calibrated using known target concentrations. In addition, the Results and Discussion section of this article may serve as a manual for those who plan to use the Gene Meter approach in their research.
The accuracy and precision of the GM was assessed by calibrating and measuring concentrations of 10 in vitro transcribed labeled 16S rRNA targets. The 10 cloned gene targets were: Mucispirillum schaedleri, Mycoplasma hyorhinis, an unclassified Lachnospiraceae, Helicobacter hepaticus stain 1, H. hepaticus stain 2, Odoribacter sp., Ureaplasma sp, Mucispirillum sp, Alistipes sp, and Lactococcus plantarum. The concentrations of the labeled 16S rRNAs for the coarse- (C1 to C8) and fine- (F1 to F8) grain dilution series are shown in Tables S1 and S2, respectively.
The DNA microarrays consisted of 100 negative-control probes and 5,813 probes targeting one of the 10 16S rRNA gene sequences (Table S3). Each probe was replicated at least 10 times (Table S4).
Concentration-signal intensity (SI) responses
Typical responses of perfectly matching probes are shown in . To fit the adsorption models, the first and last points of the isotherms were removed because the responses of some probes were below the limits of detection or approached saturation, respectively.
Figure 1. Average signal intensity ± standard error by dilution for 3 perfectly matched probes. Each datum point is the average of 10+ replicates. Open circles were used to calculate the adsorption curves (Langmuir, Freundlich, Linear); closed circles were not used. The known concentrations of 16S rRNA transcripts are shown beside each datum point in picomoles (coarse) or fentomoles (fine).
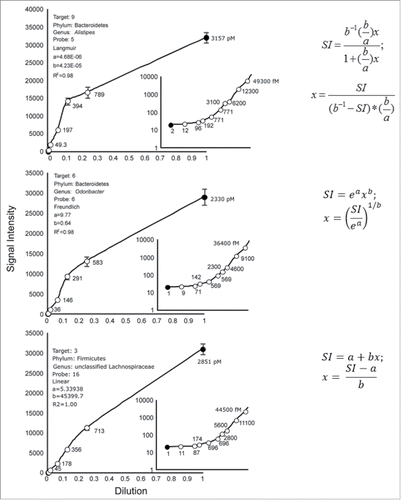
Approx. 63% of all perfect matched probes yielded linear isotherms, 22% yielded Freundlich isotherms, and 15% yielded Langmuir isotherms, which is aligned with another study.Citation10 For example, the Langmuir, Freundlich and linear models best explained the SIs of the dilution data for Probes 5, 6 and 16, respectively.
Back-calculating transcript abundances
Calculations of the transcript abundances for the Probes 9, 6, and 16 are shown in . The known target concentrations at the first dilution are shown at the top of each panel and are based on Table S1.
Figure 2. Calculations of transcript target concentrations from Langmuir, Freundlich and linear calibration models. Transcript concentrations of targets 9, 6, and 16 at a dilution of 1.0 are shown. The top panel shows the determination of transcript abundance based on an arbitrary SI of 15000 RFU, the middle panel shows the transcript abundances based on an arbitrary SI of 6000 RFU, and the lower panel shows the abundances based on an arbitrary SI of 5000 RFU.
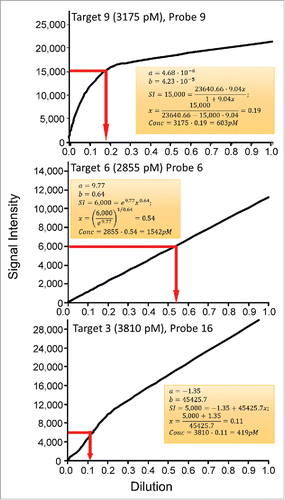
Accuracies of measured transcript abundances
The accuracies of transcript abundances were determined by calculating concentrations of targets in the samples C5 and F4 (Tables S1 and S2). The calculations were compared with the actual average transcript abundances (average ± stdev, n = 10 targets; 0.7 ± 0.1 pM for C5 and 47.1 ± 6.5 pM for F4).
Many of the predicted target abundances for C5 targets were less than zero (n = 559, closed bars) but the highest frequency was 5 pM (). The actual target concentration was 0.7 ± 0.1 pM. The difference between actual and predicted abundances was presumably because some of the adsorption isotherms were flat at the limits of detection (see bottom inset of ).
Figure 3. Accuracy of Gene Meter transcript abundances for perfectly-match probes that are unique to one target (n = 1758 targets). Closed bars, average known target concentration ± stdev was 0.7 ± 0.1 pM (n = 10 targets), while the predicted target concentration could not be determined because many targets had values of less than 0 pM (n = 559); Open bars, average known target concentration ± stdev is 47.1 ± 6.5 pM (n = 10 targets), while the determined average target concentration was 45.2 ± 10.1 pM (median = 43.9).
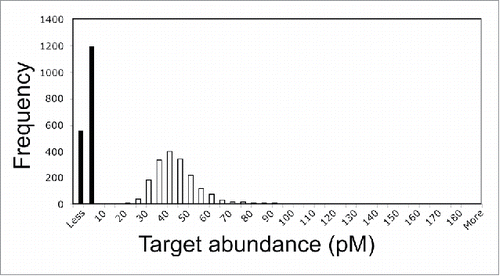
The predicted target abundance (closed bars) for F4 targets was 45.2 ± 10.1 pM (median = 43.9 pM) (). A 2-tailed T-test indicated no statistically significant difference between the actual and predicted abundances.
Accurate and precise measurement of gene expression dynamics is needed to understand how complex biological systems function. Our results provide a step-forward toward this goal. The key to determining accurate transcript abundances is calibrating the transcripts with known abundances beforehand. The new challenge now becomes cloning genes and expressing transcripts to make the dilution pool. While this challenge is laborious and time consuming, once a DNA microarray (or DNA sequencing pipeline) has been calibrated, there is no needed to recalibrate it in the future.
Methods
rRNA and microarray design
257 clones of bacterial 16S rRNA genes were obtained from a mouse gut extract. The 16S rRNA genes of 10 clones were sequenced (GenBank accession numbers KY694752 to KY694760) and their taxonomic affiliations determined using the Seqmatch in the RDP database (http://rdp.cme.msu.edu/seqmatch) (Table S3). A custom-designed 25-nt Agilent microarray was constructed using the gene sequences (Table S4). The microarray design included 100 additional oligonucleotide probes to serve as negative controls since they do not match any gene sequences.
RNA synthesis and labeling
In vitro RNA synthesis of the clones was generated using RiboMAX (Promega). The concentrations of the synthesized rRNAs were determined using Nanopore. The 10 rRNAs were mixed in equimolar quantities in a stock solution, and the pool was labeled using ULYSIS. The labeled RNA was purified and the yield determined to be 1807.8 ng, with a base/dye ratio was 81.
Preparation of dilutions, fragmentation, and hybridization conditions
The pooled labeled RNAs was diluted with a solution of yeast tRNA. To expand the dynamic range, we conducted 2 dilution series: one was designated as coarse-grain (C1 to C8) and the other fine-grain (F1 to F8).
For the coarse-grain dilution series (Table S1), the stock dilution solution consisted of 5 µl of yeast tRNA and 1500 µL of dH20. The first dilution solution consisted of 10 µL of the labeled RNA mixed with 70 µL of the stock dilution. The second dilution solution consisted of 10 µL of the first dilution solution and 70 µL of the stock solution. The third to eighth dilution solutions were made in a similar fashion; the previous solution was used to make the next solution.
For the fine-grain dilution series (Table S2), the first dilution solution consisted of 5 µl of the pooled labeled RNA and 35 µL of the stock dilution solution (above). The second dilution solution consisted of 10 µL of the first dilution solution and 30 µL of the stock solution. The third to eighth dilution solutions were made in a similar fashion; the previous solution was used to make the next solution.
The coarse- and fine-grain dilution solutions were stored at −80°C.
Fragmentation of the labeled RNA was conducted using the original Agilent “One-Color Microarray-Based Gene Expression Analysis,” Version 6.5. 19 µl of each diluted solution was mixed in separate tubes with 5uL of 10X Blocking Agent and 1uL of 25X Fragmentation Buffer, incubated at 60°C for exactly 30 min to fragment RNA, immediately cooled on ice for one minute, added 25 uL of 2x GEx Hybridization Buffer to stop the fragmentation reaction. The fragmented labeled RNA (40 µL) were hybridized to 8-plex microarray at 48°C for 17 to 18 h.
Fitting of the adsorption isotherms
The fitting of the models involved transforming the data to a straight line and calculating 2 parameters, a and b. Software used for these calculations is located at: http://web.evolbio.mpg.de/∼alexander.pozhitkov/microarray123/.
Abbreviations
GM | = | Gene Meters |
SI | = | signal intensities |
RFU | = | relative fluorescence units |
Acknowledgments
The work was supported by funds from the Max Planck Society.
References
- Tu Y, Stolovitzky G, Klein U. Quantitative noise analysis for gene expression microarray experiments. Proc Natl Acad Sci USA 2002; 99:14031; PMID:12388780; https://doi.org/10.1073/pnas.222164199
- Amend AS, Seifert KA, Bruns TD. Quantifying microbial communities with 454 pyrosequencing: Does read abundance count? Mol Ecol 2010; 19:5555; PMID:21050295; https://doi.org/10.1111/j.1365-294X.2010.04898.x
- Barash Y, Dehan E, Krupsky M, Franklin W, Geraci M, Friedman N, Kaminski N. Comparative analysis of algorithms for signal quantitation from oligonucleotide microarrays. Bioinformatics 2004; 20:839-46; PMID:14751998; https://doi.org/10.1093/bioinformatics/btg487
- Harr B, Schlo ̈tterer C. Comparison of algorithms for the analysis of Affymetrix microarray data as evaluated by co-expression of genes in known operons. Nucl Acids Res 2006; 34:e8; PMID:16432259; https://doi.org/10.1093/nar/gnj010
- Pozhitkov AE, Noble PA, Bryk J, Tautz D. A revised design for microarray experiments to account for experimental noise and uncertainty of probe response. PLoS One 2014; 9:e91295; PMID:24618910; https://doi.org/10.1371/journal.pone.0091295
- Harrison A, Binder H, Buhot A, Burden CJ, Carlon E, Gibas, C, Gamble LJ, Halperin A, Hooyberghs J, Kreil, DP, et al. Physico-chemical foundations underpinning microarray and next-generation sequencing experiments. Nucl Acids Res 2013; 41:2779-96; PMID:23307556; https://doi.org/10.1093/nar/gks1358
- Hunter MC, Pozhitkov AE, Noble PA. Microbial signatures of oral dysbiosis, periodontitis and edentulism revealed by gene meter methodology. J Microbiol. Methods 2016; 131:85-101; PMID:27717873; https://doi.org/10.1016/j.mimet.2016.09.019
- Pozhitkov AE, Neme R, Domazet-Loo T., Leroux BG, Soni S, Tautz D, Noble PA. Tracing the dynamics of gene transcripts after organismal death. Open Biol 2017; 7:160267; PMID:28123054; https://doi.org/10.1098/rsob.160267
- Hunter MC, Pozhitkov AE, Noble PA. Accurate predictions of post-mortem interval using linear regression analyses of gene meter expression data. Forensic Sci Internat 2017; 275:90-101. https://doi.org/10.1016/j.forsciint.2017.02.027
- Pozhitkov AE, Boubeb I, Brouwer MH, Noble PA. Beyond Affymetrix arrays: Expanding the set of known hybridization isotherms and observing pre-wash signal intensities. Nucl Acids Res 2010; 38:e28; PMID:19969547; https://doi.org/10.1093/nar/gkp1122