Abstract
Variation at 17 microsatellite loci was analyzed for about 50,000 coho salmon Oncorhynchus kisutch sampled from 274 locations ranging from Russia to California (but largely from British Columbia), and the variation was applied to estimate stock composition in mixed-stock fishery samples. High resolution of mixed-stock samples was possible; accurate estimates of stock composition were available for coho salmon originating from 39 regions (Russia, 1 region; Yukon River, 1; southeast Alaska, 1; British Columbia, 28; Washington, 5; Columbia River, 1; Oregon, 1; California, 1). The power of a locus in providing accurate estimates of stock composition of simulated single-population mixtures was related to the number of alleles observed at the locus. Approximately 800 alleles were observed across the 17 microsatellites. Analysis of known-origin samples indicated that accurate regional estimates of stock composition were obtained; estimates from 37 of 39 regions had accuracy greater than 90%. Estimated stock compositions of five mixed-fishery samples collected in British Columbia and the San Juan Islands (Washington) reflected the presence and timing of migration of the local populations. Microsatellites provided accurate estimates of stock composition from many locations in the British Columbia distribution of coho salmon.
Received July 5, 2011; accepted October 22, 2011
Information on stock identification of salmon in mixed-stock fisheries is vital for enabling fishery managers to decide on the timing and area of local salmon fisheries. Various techniques have been used to provide estimates of stock composition, and the general objective is to provide the greatest resolution among the stocks or populations present while doing so at the cheapest practical cost per fish. If stock composition information is critical for guiding managers in opening fisheries at specific locations and at specific times during spawning migrations, then the technique applied must also enable rapid estimation of stock composition. Stock composition information is important in determining locations of ocean residence for specific stocks of immature salmon, the migration routes used by immature salmon to reach seasonal rearing areas, and the routes used by maturing salmon to return to natal rivers.
A traditional method of stock identification for coho salmon Oncorhynchus kisutch employed coded-wire tags (CWTs; Jefferts et al. 1963) to determine the origins of individuals. The number of CWTs recovered was expanded depending upon the marking rate at the hatchery and the sampling rate in the fishery. Estimates of stock composition were obtained from these expansions. However, in some jurisdictions the recent practice of clipping the adipose fins of all juveniles from hatcheries, regardless of whether those fish received CWTs, has substantially increased the complexity of CWT recovery and interpretation of the results. The marking of all hatchery fish is used to focus harvest on abundant hatchery stocks through mark-selective fisheries (i.e., release of unmarked wild fish). Thus, the assumption of equal exploitation for marked and unmarked fish is violated, thereby limiting the ability of CWTs to provide reliable estimates of stock composition where both hatchery and wild populations are exploited.
Development of DNA markers has led to new avenues of research for using genetic variation in estimating stock composition in mixed-stock coho salmon fisheries. Microsatellites have been investigated, and population structure was reported to be regionally based (Small et al. Citation1998a, Citation1998b; Beacham et al. Citation2001; Smith et al. Citation2001; Olsen et al. Citation2003; Ford et al. Citation2004; Bucklin et al. Citation2007; Johnson and Banks Citation2008). A regionally based population structure is essential for stock identification applications, as individuals in the mixture from populations that are not in the baseline used for stock composition estimation will generally be assigned to other sampled populations from the same region. Microsatellites, along with variation at the major histocompatibility complex (MHC), were extensively applied in the late 1990s to estimate stock composition in the Canadian fisheries in which coho salmon were caught (Beacham et al. Citation2001). Microsatellites also provided the basis for estimating the stock composition of juvenile coho salmon sampled off the coasts of Washington and Oregon (Van Doornik et al. Citation2007).
For other species of Pacific salmon, the accuracy and precision of estimated stock composition have been reported to be related to the number of alleles observed at a microsatellite locus; loci with more alleles are generally more powerful than those with fewer alleles (Beacham et al. Citation2005, Citation2006, Citation2008b). Similarly, the accuracy and precision of estimated stock composition have been reported to increase as the number of microsatellites used in the estimation increases (Beacham et al. Citation2005, Citation2006, Citation2008b). The two main factors that directly influence accuracy and precision of stock composition estimates are (1) the completeness of the baseline and (2) the number and power of the genetic markers used in the estimation of stock composition. Much of the work in Pacific salmon genetic stock identification has focused on increasing the resolution (higher accuracy and higher precision) of the stock composition estimates while at the same time maintaining or decreasing the cost of analysis per individual. The accuracy and precision of estimated stock compositions reported by Beacham et al. (Citation2001) were derived from applying eight microsatellite loci and two MHC loci with a baseline of 138 coho salmon populations originating largely from British Columbia. The resolution of estimated stock compositions reported by Beacham et al. (Citation2001) would likely be improved by increasing the number and regional representation of populations included in the baseline and by increasing the number of genetic markers used in the analysis.
In the present study, we evaluated the utility of using variation at 17 microsatellites for coho salmon stock identification applications in British Columbia. This evaluation was conducted by examining the accuracy and precision of estimated stock compositions for individual microsatellites and combinations of microsatellites through analysis of simulated mixtures and estimation from actual samples taken from fisheries in the coastal waters of British Columbia. The mixtures were resolved by using a 274-population baseline that incorporated populations from Russia to California. We demonstrated that sufficient population allele frequency variation exists at 17 microsatellites to enable accurate estimation of coho salmon stock composition to 39 reporting regions for mixed-stock samples by using this 274-population baseline. Furthermore, the accuracy and precision of estimated stock compositions from the baseline of eight microsatellites and two MHC loci (outlined by Beacham et al. Citation2001) were compared with the accuracy and precision obtained by using the current 17-microsatellite baseline.
METHODS
Collection of DNA samples and laboratory analysis.—Tissue samples were generally collected from mature coho salmon, although in some instances juveniles were sampled. Samples were preserved in a 95% ethanol solution and were sent to the Molecular Genetics Laboratory at the Pacific Biological Station, Department of Fisheries and Oceans Canada (DFO). The DNA was extracted from the tissue samples by using a variety of methods, including a Chelex resin protocol (as outlined by Small et al. 1998), a QIAGEN 96-well DNeasy procedure (QIAGEN, Mississauga, Ontario), or a Promega Wizard SV96 Genomic DNA Purification System. Once the extracted DNA was available, variation was surveyed at 17 microsatellite loci: Ots3 (Banks et al. Citation1999), Ots101, Ots103 (Nelson and Beacham Citation1999), Ots213 (Greig et al. Citation2003), OtsG253b (Williamson et al. Citation2002), Omy325 (O’Connell et al. Citation1997), Omy1011 (Spies et al. Citation2005), One13m (Scribner et al. Citation1996), One111 (Olsen et al. Citation2000), Ogo2 (Olsen et al. Citation1998), Oki1, Oki10 (Smith et al. Citation1998), Oki100 (Beacham et al. Citation2008a), Oki101 (Beacham et al. Citation2011b), Ssa407 (Cairney et al. Citation2000), Ocl8 (Condrey and Bentzen Citation1998), and P53 (Baker et al. Citation2002).
Polymerase chain reaction (PCR) DNA amplifications were conducted by using a DNA Engine Tetrad-2 thermal cycler (BioRad, Hercules, California) in 6-μL volumes consisting of 0.15 units of Taq polymerase, 1 μL of extracted DNA, 1× PCR buffer (QIAGEN), 60 μM of each nucleotide, 0.40 μM of each primer, and deionized H2O. Specific PCR conditions for each locus are outlined by Beacham et al. (Citation2011b). The PCR fragments were initially size-fractionated in denaturing polyacrylamide gels by using an ABI Model 377 automated DNA sequencer (Applied Biosystems, Inc. [ABI], Foster City, California), and genotypes were scored with ABI Genotyper version 2.5 by using an internal lane sizing standard. Later in the study, microsatellites were size-fractionated in an ABI Model 3730 capillary DNA sequencer, and genotypes were scored with ABI GeneMapper version 3.0 by using an internal lane sizing standard. Allele identifications between the two sequencers were standardized by analyzing approximately 600 individuals on both platforms and then converting the sizing in the gel-based data set to match that obtained from the capillary-based set.
Baseline populations.—The baseline survey involved the analysis of about 50,000 coho salmon representing 274 populations from Russia, Alaska, Canada, Washington, Oregon, and California (). The sampling sites or populations surveyed in each geographic region were outlined by Beacham et al. (Citation2011b); most of the samples were collected after 1990. Populations with fewer than 30 individuals sampled were removed from the baseline; two populations were added when samples became available (Clayoquot River on the west coast of Vancouver Island; Marble River on the northwestern coast of Vancouver Island); and the baseline populations were summarized by geographic regions and river drainages (; ). Information on regional population structure has been outlined previously by Beacham et al. (Citation2011b). Estimates of Weir and Cockerham's (Citation1984) genetic differentiation index F ST for each locus over all populations were calculated with FSTAT version 2.9.3.2 (Goudet Citation1995). Allele frequencies for all populations surveyed in this study are available at the DFO Molecular Genetics Laboratory website (www.pac.dfo-mpo.gc.ca/science/facilities-installations/pbs-sbp/mgl-lgm/data-donnees/index-eng.htm).
FIGURE 1 Map of the Pacific Rim, indicating the general geographic regions where coho salmon from 39 reporting regions were surveyed (see ; QCI = Queen Charlotte Islands; Vancouver I = Vancouver Island; R = River). Specific populations in the regions are outlined by Beacham et al. (2011b: their Appendix 1).
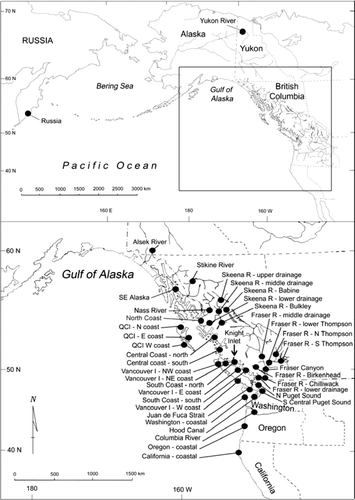
TABLE 1 Summary of the number of sampling sites or populations of coho salmon within each geographic region listed in . A complete listing of the populations is outlined by Beacham et al. (2011b: their Appendix 1); populations with fewer than 30 sampled individuals were removed from the baseline used for stock identification (N = number of populations included within the given region). The range of population sample sizes within each region is shown in parentheses.
Estimation of stock composition in single-population samples.—Analysis of single-population samples was used to evaluate the power of individual microsatellites for estimation of stock composition. Genotypic frequencies were determined for each locus in each population, and Statistical Package for the Analysis of Mixtures (SPAM) version 3.7 (Debevec et al. Citation2000) was used to estimate stock composition of simulated single-population samples. The use of SPAM was restricted to analyzing the power of individual loci. The Rannala and Mountain (1997) correction to baseline allele frequencies was used in the analysis; this was done so that the simulated mixture from a specific population would not contain fish with alleles that were not observed in the baseline samples from that population. All loci were considered to conform to Hardy–Weinberg equilibrium (HWE), and expected genotypic frequencies were determined from the observed allele frequencies. Reported stock compositions for simulated single-population samples are the bootstrap mean estimate of each mixture of 150 fish (100% from a single population); the mean and variance estimates were derived from 1,000 bootstrap simulations. Each baseline population and simulated single-population sample were sampled with replacement in order to simulate random variation involved in the collection of the baseline and fishery samples.
Power of individual microsatellites.—The accuracy and precision of estimated stock compositions for simulated single-population samples were evaluated for each microsatellite locus individually for 39 single-population samples (Russia to California distribution). One population was chosen, largely based on available sample size, from each of the 39 geographic regions outlined in . Each locus was analyzed separately for each of the 39 populations used in the single-population simulations. Accuracy and precision (i.e., SD) were then averaged over the 39 single populations for each locus. A logarithmic function (in Microsoft Excel) was used to compare the mean values of accuracy and precision (as observed from the 39 simulated samples) with the number of alleles observed at each locus. For Oki100, only the first 99 alleles could be incorporated into the analysis. The 39 test populations representing the 39 regions outlined in were as follows: Distvenichna River in region 1, Porcupine River in region 2, Berners River in region 3, Klukshu River in region 4, Scud River in region 5, Zolzap Creek in region 6, Sangan River in region 7, Pallant River in region 8, Mercer Creek in region 9, Oona River in region 10, Sustut River in region 11, upper Babine River in region 12, Toboggan Creek in region 13, Kitwanga River in region 14, Kasiks River in region 15, Hartley Bay in region 16, Martin River in region 17, Kakweiken River in region 18, Tenderfoot Creek in region 19, Quatse River in region 20, Nanaimo River in region 21, Stephens Creek in region 22, Nitinat River in region 23, Birkenhead River in region 24, Chilliwack River in region 25, Chehalis River in region 26, Nahatlatch River in region 27, McKinley Creek in region 28, Coldwater River in region 29, Lemieux Creek in region 30, Eagle River in region 31, Skykomish River in region 32, Minter Creek in region 33, Dewatto River in region 34, Dungeness River in region 35, Queets River in region 36, Lewis River in region 37, Siuslaw River in region 38, and Noyo River in region 39.
The relationship between total allele number and accuracy or precision was investigated by starting with the locus that had the highest number of observed alleles (Oki100) and then determining the average accuracy and precision over the 39-population set. A second locus with the next-highest number of observed alleles (OtsG253b) was then added to estimate stock composition over the 39-population set. Additional loci were added sequentially (in reverse of the order listed in ) to the suite of microsatellites until 16 loci were incorporated into the suite used for stock composition estimation. Limitations of SPAM software precluded One111 from being added to the suite. A function was fitted to the observed results to compare accuracy and precision with the total number of observed alleles in the microsatellite suite used for stock composition estimation.
TABLE 2 Number of alleles per locus, genetic differentiation index F ST, mean accuracy (%), and mean SD (%) for estimated percentage compositions of simulated single-population mixtures (correct = 100%) for 39 test populations of coho salmon (see Methods) distributed from Russia to California. The number of alleles and F ST were calculated from the population survey outlined by Beacham et al. (Citation2011b). For Oki100, only the first 99 alleles could be incorporated into the analysis.
Estimation of stock composition in mixed-stock samples.—The next stage of the analysis was to analyze a multipopulation mixture sample from a single geographic region or reporting group. Because fewer analyses were required at this stage, analysis of mixture samples was conducted with a Bayesian procedure (cBayes; Neaves et al. 2005). The cBayes software was used for all subsequent applications outlined in the study. The cBayes analyses required substantially more computer analytical time than did the SPAM software for analysis of an individual sample. Previous applications of both SPAM and cBayes to the same mixed-stock sample suggested that accuracy was improved by use of cBayes (Beacham et al. Citation2005). In the analysis, ten 20,000-iteration Monte Carlo Markov chains of estimated stock compositions were produced; the initial starting value for each chain was set at 0.90 for a particular population, and this population differed for each chain. Estimated stock compositions were considered to have converged when the shrink factor was less than 1.2 for the 10 chains (Pella and Masuda Citation2001), and thus the starting values were considered to be irrelevant. Stock composition estimates converged before 20,000 iterations, and no further improvements in estimates were observed beyond 20,000 iterations. Therefore, the use of 20,000 iterations was set as the standard in the analysis. The last 1,000 iterations from each of the 10 chains were then combined, and for each fish the probability of originating from each population in the baseline was determined. These individual probabilities were summed over all fish in the sample and then divided by the number of fish sampled to provide the point estimate of stock composition. Standard deviations of estimated stock compositions were determined based on the final 1,000 iterations from each of the 10 chains incorporated in the analysis.
Analysis of known-origin, single-region mixtures.—In the analysis of a sample from a single reporting region, a mixture sample was created by removing the same number of fish from each population in the region to create a 100-fish sample; the baseline allele frequencies for each population in the region were then recalculated by excluding the fish that were selected for the mixture sample. This analysis was conducted for each of the 39 reporting regions evaluated in our survey. For some regions, it was not possible to remove 100 fish from the baselines and still have acceptable remaining baseline population sample sizes, so mixture sample sizes of 50 or fewer fish were analyzed in those cases. In this portion of the analysis, all fish in each mixture were derived from populations that belonged to the 274-population baseline. In an additional independent analysis, samples were available from populations that were excluded from the 274-population baseline owing to small sample size. All such samples were pooled within a region to provide an additional test of the power of the baseline for stock composition analysis. In this case, the mixtures contained no fish from the baseline populations, and this test was dependent upon regional population structure for correct estimation of stock composition.
Analysis of known-origin, multiregion mixtures.—The final stage in the analysis prior to application in actual fishery samples was to estimate stock compositions of known-origin, multiregion samples that were completely independent of the baseline used in the estimation. Known-origin samples were developed by pooling the fish sampled from small-sample-size populations into multiregional collections. None of the coho salmon in these samples originated from the baseline populations; therefore, these samples were anticipated to provide a more-stringent test than actual mixed-stock fishery samples, in which at least some of the fish would presumably originate from populations in the baseline. Five known-origin, multiregion mixture samples that had a geographic basis were evaluated: (1) a northern sample composed of 187 individuals from the Alsek River, Stikine River, and Queen Charlotte Islands; (2) 76 fish originating entirely from the Skeena River; (3) 381 fish derived from central and southern British Columbia populations; (4) 518 individuals obtained entirely from Fraser River populations; and (5) a southern sample consisting of 288 fish from Vancouver Island, Washington, the Columbia River, and California.
Analysis of mixed-stock samples.—Analysis of mixtures in which all fish originated from populations in the baseline and mixtures in which all fish originated from populations outside of the baseline provided some bounds of the accuracy of estimated stock compositions that may be expected when applied to actual fishery samples of unknown origin. Actual mixed-stock fishery samples probably contain some individuals from populations that are represented in the baseline used for stock composition estimation and some individuals from populations that are not represented in the baseline. Analysis of divergent samples can aid in evaluating whether the estimated stock compositions are within expectations for the location and timing of sample collection.
FIGURE 2 Relationship between the number of alleles observed at a microsatellite locus and (A) the average percentage accuracy to population or (B) the standard deviation obtained for single-population mixtures of 39 coho salmon test populations (see Methods) by using only a single locus and the 274-population Pacific Rim baseline. The software program SPAM was used to estimate stock compositions. Numbers next to data points correspond to the numbered loci listed in .
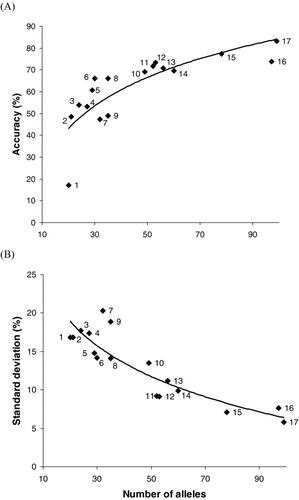
We tested the microsatellites used in stock composition estimation by analyzing five mixed-stock samples of coho salmon (n = 83–392 fish) with divergent geographic origins. The geographic distribution of these samples, spanning sites from the north coast of the Queen Charlotte Islands (British Columbia) to the San Juan Islands (northern Washington), suggested that divergent estimates of stock composition should be obtained when analyzed with the 274-population baseline. Samples were derived from recreational, commercial, and test fisheries and from juvenile surveys.
RESULTS
Power of Individual Microsatellites
At the 17 microsatellite loci in the coho salmon populations surveyed, the number of alleles ranged from 20 to 105 per locus (). The number of alleles observed at a locus was related to the estimated accuracy of stock composition for the single-population mixtures of the 39 test populations (). This relationship was described by the following fitted equation (r 2 = 0.67):
FIGURE 3 Relationship between the mean accuracy of estimated stock composition to population and the genetic differentiation index F ST for 17 microsatellite loci used to estimate the percent composition of single-population mixtures (correct = 100%) for 39 coho salmon test populations (see Methods). Numbers next to data points correspond to the numbered loci listed in .
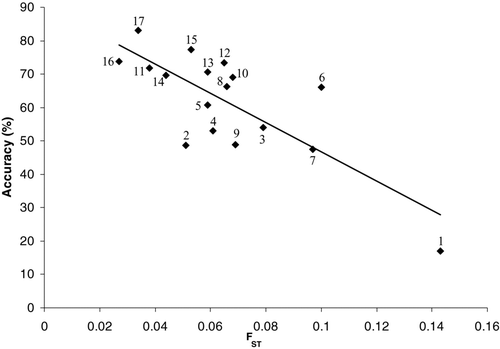
Number of Alleles in Relation to Accuracy and Precision of Estimated Stock Compositions
The total number of alleles used to estimate stock composition was related to the accuracy and precision of estimated stock compositions. Starting with the locus that had the largest number of alleles (Oki100) and sequentially adding loci with progressively fewer alleles produced stock composition estimates of increasing accuracy (). The relationship was described by the fitted equation (r 2 = 0.94)
FIGURE 4 Relationship between the total number of alleles used in stock composition estimation and (A) the average percentage accuracy to population or (B) the standard deviation obtained for single-population mixtures of 39 coho salmon test populations (see Methods) by using the 274-population Pacific Rim baseline. The software program SPAM was used to estimate stock compositions.
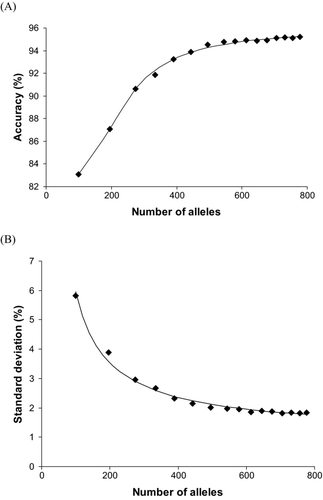
Precision of estimated stock compositions was related to the number of alleles employed in estimation. Continual addition of loci (i.e., increasing the number of alleles) resulted in stock composition estimates of continually increasing precision (). The relationship was described by the following fitted equation (r 2 = 0.99):
Analysis of Known-Origin, Single-Region Mixtures
The application of the current 17-microsatellite baseline resulted in estimated stock compositions of generally higher accuracy and higher precision in comparison with the previous baseline of eight microsatellites and two MHC loci (Beacham et al. Citation2001). Regions with the largest increase in estimated accuracy included the east coast of Vancouver Island (accuracy increased by 9%), north Puget Sound (9%), Hood Canal (22%), Washington coast (20%), and the Columbia River (15%; ). Accurate regional estimates of stock composition were obtained when known-origin mixtures of coho salmon originating solely from baseline populations within a single region were evaluated with the 17-microsatellite baseline. For example, estimated stock compositions for the single-region mixtures were above 90% for all but 2 of the 39 regional reporting groups evaluated (). The two exceptions were north Puget Sound and south–central Puget Sound, where there was not a strong separation between current populations in the baseline. A mixture of 100% north Puget Sound origin was estimated as consisting of 89.8% north Puget Sound and 7.3% south–central Puget Sound, whereas a mixture of 100% south–central Puget Sound was estimated as consisting of 80.0% south–central Puget Sound and 19.3% north Puget Sound. Other than these two cases, there was a very strong regional separation for stock composition analysis among the 39 reporting regions as long as fish in the mixtures originated solely from populations in the baseline.
The number of individuals available for mixed-stock analysis from populations that did not belong to the baseline was limited in most regions (). Accuracy of regional stock composition estimates was variable and depended on the general geographic area included in the analysis. For example, estimates of stock composition were generally accurate for samples originating from coastal areas of the Queen Charlotte Islands: accuracy was greater than 90% for all three regions evaluated (). In the Skeena River drainage, only 61% of a 24-fish sample was correctly estimated to originate from the Babine River (two populations in the baseline), and 39% of the sample was estimated to originate from other Skeena River regions. For the southern portion of the central British Columbia coast (hereafter, “Central Coast”), 88% of the sample was correctly estimated to region and 11% was estimated to originate from the adjacent region (northern Central Coast). Similar patterns were observed for samples from Vancouver Island, where accuracy of estimated stock composition of regional samples was 72% and allocations were also made to other Vancouver Island regions. The accuracy of estimated stock compositions for Vancouver Island regions was typically less than that obtained for the same regions when only fish from baseline populations were included in the mixtures (). In the Fraser River drainage, individuals were available from six of eight regions defined in the drainage, and estimated accuracy of stock compositions from these regions was above 90% for five of the six regions evaluated. The sample estimated with the most error was the south–central Puget Sound sample, which was composed entirely of individuals from the Deschutes River; 88% of this sample was allocated to a northern Puget Sound source.
TABLE 3 Estimated stock composition (%; SD in parentheses) for single-region mixtures of 100 coho salmon derived from a 274-population baseline. The eight-locus set of markers includes the eight microsatellites reported by Beacham et al. (Citation2001); the 10-locus set includes the previous eight microsatellites as well as two major histocompatibility complex loci (Beacham et al. Citation2001); and the 17-locus set includes the 17 microsatellites surveyed in the present study. Regional known-origin samples were developed by randomly removing individual fish from baseline populations in proportion to the number of regional populations, re-estimating allele frequencies for all populations in the region, and then using this modified baseline to estimate stock composition in the sample of known origin. Subsequently, individuals (N) from populations that were not included in the 274-population baseline (i.e., due to small sample size) were pooled by region, and stock composition (%) was estimated.
Analysis of Known-Origin, Multiregion Mixtures
All five known-origin, multiregion mixtures were composed entirely of fish sampled from populations that were not included in the 274-population baseline. The first sample evaluated was composed of coho salmon from the Alsek River, Stikine River, and the three coasts of the Queen Charlotte Islands. Estimated regional stock compositions were within 3% of actual values for four of the five regions present in the mixture; 2.5% of the sample was estimated to have been derived from other regions in the baseline (). The second sample evaluated was composed entirely of fish that were derived from the Skeena River drainage. Some misallocation occurred among regions within the drainage, as some fish from the upper Skeena and Babine River regions were allocated to the middle Skeena and Bulkley River regions. Only 1.1% of the sample was allocated to baseline populations outside of the Skeena River drainage ().
TABLE 4 True (expected) and estimated (observed) stock compositions (%; SD in parentheses) of known-origin, mixed-stock samples (N = sample size) of coho salmon. The multiregion mixture samples were constructed by combining population samples (previously outlined by Beacham et al. Citation2011b) that were not included in the baseline (i.e., due to population sample size being < 30 individuals) and additional small-sample-size populations that were surveyed but previously unreported.
The third sample was composed of fish that were derived from mainland British Columbia populations situated south of the Skeena River and north of the Fraser River. Estimated regional stock compositions were within 4% of actual values for all four regions represented in the mixture, whereas 2.5% of the sample was estimated to have been derived from populations in other regions (). The fourth sample evaluated was composed entirely of fish derived from the Fraser River drainage. Samples were available from populations in six of the eight regions defined for the drainage. Estimated stock compositions were within 5% of actual values for all six regions and were within 2% of the actual values for four of the six regions (). Allocations to regions other than the Fraser River regions totaled 2.2% of the sample.
The fifth sample evaluated was composed of fish from Vancouver Island, Washington, the Columbia River, and California. Estimated stock compositions were within 4% of actual values for five of the eight regions that were represented in the mixture (). The largest errors in estimated stock composition were observed for the Washington coast and Hood Canal regions, as the contributions of both regions were underestimated by 5–7% (). Misallocations to populations in regions that were not represented in the mixture totaled 9.4%; the northeastern coast of Vancouver Island was the region that received the largest erroneous allocation (4.8%).
Analysis of Mixed-Stock Samples
Five real mixed-stock samples of unknown origin were evaluated for the study. Sample 1 consisted of fish that were collected in late July and August 2004 from a recreational fishery near Langara Island in the northern coastal region of the Queen Charlotte Islands. Large regional contributors to this sample were identified as the northern Central Coast (28%), the north coast of the Queen Charlotte Islands (16%), and the southern Central Coast (13%); the Nass River (8%), Stikine River (6%), and Skeena River (lower drainage; 4%) regions also contributed to the sample (). The coho salmon in the sample were identified as being largely northern or central British Columbia in origin, with some contributions from the northeastern coast of Vancouver Island and the northern part of the southern British Columbia coast (hereafter, “South Coast”).
Sample 2 was derived from juvenile coho salmon sampled in the Strait of Georgia during late June 2009; therefore, these fish had reared in the marine environment for only a few months. Coho salmon of Fraser River origin were the most abundant in the sample (44%), followed by fish from the east coast of Vancouver Island (31%), northern Puget Sound (17%), and the southern South Coast (7%). All of these regions are geographically adjacent to the Strait of Georgia. Sample 3 was obtained as bycatch from a directed pink salmon fishery located south of the Fraser River mouth in mid-September 2009. Coho salmon of Fraser River origin dominated the sample and were estimated to comprise 67% of the sample. Regions including the Fraser River canyon and areas upstream (middle Fraser and Thompson rivers) were estimated to contribute 33% of the Fraser River component (). Other significant regional contributions to the sample were estimated as originating from the southern South Coast (16%) and the east coast of Vancouver Island (12%).
Sample 4 was obtained in mid-October 2005 from a fishery conducted in an area adjacent to the San Juan Islands, northern Washington. Coho salmon of Fraser River origin were estimated to contribute 60% of the sample, and only 0.5% of the Fraser River component was estimated to have originated upstream of the lower Fraser River region (). Other regional contributions were estimated as 21% from the east coast of Vancouver Island, 9% from the southern South Coast, and 5% from northern Puget Sound (). Sample 5 consisted of fish that were collected by beach seining in the main-stem Fraser River upstream from the Harrison River confluence during mid-September 2009. All coho salmon in this sample were estimated to have been derived from regions upstream from the Harrison River; the North Thompson River contributed the largest regional component (46%) to the sample ().
TABLE 5 Estimated stock compositions (%; SD in parentheses) of five mixed-stock samples of coho salmon. Samples were obtained from the following fisheries (1) Langara Island recreational fishery along the north coast of the Queen Charlotte Islands (54°14′N, 133°02′W; 21 July–28 August 2004; sample size N = 392); (2) Strait of Georgia (49°30′N, 123°40′W; 30 June–2 July 2009; N = 310); (3) bycatch in the pink salmon Oncorhynchus gorbuscha fishery located south of the Fraser River mouth (49°03′N, 123°10′W; 14–17 September 2008; N = 83); (4) San Juan Islands (48°20′N, 122°50′W; 17–23 October 2005; N = 180); and (5) main-stem Fraser River upstream from the Harrison River mouth (49°13′N, 121°50′W; 22–23 September 2009; N = 101). Estimated stock compositions were derived from applying a 274-population baseline for each sample and summing population estimates by region. Blank cells indicate that the regional estimate was 0.0%.
DISCUSSION
Population Structure
In Pacific salmon, genetic population structure is typically regionally based or river drainage based. Population structure of coho salmon conforms to this pattern; a regionally based population structure has been observed throughout the range of the species’ spawning distribution in North America (Olsen et al. Citation2003; Bucklin et al. Citation2007; Johnson and Banks Citation2008; Beacham et al. Citation2011b). A regionally based population structure is generally required in the application of genetic markers for stock composition estimation, as an important assumption in the application is that the portion of the mixed-stock sample derived from populations outside of the baseline is allocated to sampled populations from the same region. This assumption reduces the cost and complexity of developing a baseline for stock composition analysis. Given the general results from testing the baseline with mixtures composed entirely of unsampled populations, coho salmon population structure thus meets the important condition that unsampled populations contributing to mixed fishery samples will likely be allocated to sampled populations within the same region. In applications where errors in regional estimation are considered to be too large for satisfactory use, then it will be necessary to sample more baseline populations in the region in order to enhance the reliability of regional estimates of stock composition.
Loci used in stock composition estimation are assumed to conform to HWE for the baseline populations (Debevec et al. Citation2000). In our application, two microsatellites (Oki100 and Ots103) deviated from HWE expectations for some of the populations (Beacham et al. Citation2011b). If loci that deviate from HWE are included in the baseline for stock composition analysis, will this result in estimated stock compositions that are less accurate or less precise than stock compositions obtained by excluding such loci? After investigating the effects of including loci that deviated from HWE across a range of species, we have concluded that accuracy and precision of stock compositions are in fact improved by including these loci in the set used for stock composition analysis (Beacham et al. Citation2001, Citation2005, Citation2006). In other studies, loci that did not conform to HWE were included in stock identification applications or assignment of individuals, and the investigators reported that stock composition or individual assignment estimates were accurate (Van Doornik et al. Citation2007; Carlsson Citation2008; Griffiths et al. Citation2010). Thus, we conclude that the conformity of genotypic frequencies to HWE for all loci included in stock composition analysis is not essential.
Number of Alleles
The number of alleles observed at a microsatellite locus is a key predictor in the effectiveness of a locus for determining stock composition. Initially, simulation studies suggested that loci with more alleles were more powerful than those with modest numbers of alleles (Kalinowski Citation2002, Citation2004). Later empirical applications provided more evidence on which to base conclusions concerning locus stock identification power. Studies conducted in our laboratory on stock identification of sockeye salmon O. nerka (Beacham et al. Citation2005), Chinook salmon O. tshawytscha (Beacham et al. Citation2006, Citation2008b), and chum salmon O. keta (Beacham et al. Citation2009) have consistently indicated that the number of alleles observed at a microsatellite locus is positively correlated with the power of that locus to provide accurate and precise estimates of stock composition. In general, loci with larger numbers of alleles were more effective in providing more-accurate and more-precise stock composition estimates than were loci with smaller numbers of alleles, provided that sample sizes of the baseline populations were adequate to estimate allele frequencies with only modest sampling error.
Does employing more microsatellite alleles always produce more-accurate and more-precise estimates of stock composition? Previous empirical studies on sockeye salmon (Beacham et al. Citation2005), Chinook salmon (Beacham et al. Citation2006), and chum salmon (Beacham et al. Citation2009) indicated that adding more loci consistently resulted in estimates of higher accuracy and precision, and the effect on precision was more pronounced than the effect on accuracy. Similarly, through simulation analysis, Anderson (Citation2010) reported that accuracy of individual assignment to specific populations increased with every additional locus used (up to 50 loci) in the analysis. The empirical studies indicated a rapid improvement in accuracy of estimated stock compositions until 100–200 alleles were employed; the use of additional alleles resulted in diminishing returns for accuracy per allele employed, whereas variance of the estimates continued to decline. With 17 loci available for use in estimating coho salmon stock composition, there is some ability to modify the set of loci used in stock identification applications to meet the levels of accuracy and precision required in the application. In general, if fewer loci can be used in estimating stock composition because the resolution or precision of the estimated stock composition exceeds that required in the application and if cost sensitivity of the analysis exists with respect to cost per fish, then options are available to reduce costs by reducing the number of loci surveyed.
Accuracy and Precision of Estimated Stock Compositions
Evaluation of the reliability of stock composition estimates typically starts with the analysis of simulated samples. Anderson et al. (Citation2008) and Anderson (Citation2010) suggested that overly optimistic estimates of performance by the genetic markers and the baseline employed may result from the analysis of simulated or nonindependent samples. This may be true in some cases, but analysis of such samples provides an initial starting phase for evaluation. If analysis of simulated mixtures derived entirely from existing baseline populations cannot be resolved with sufficient accuracy for application, then no further analysis need be conducted. In this case, additional discriminatory genetic markers must be added to the suite used for stock identification applications. If analysis of simulated mixtures produces satisfactory resolution in estimates of stock composition, then the next phase of the evaluation requires the analysis of known-origin samples that are independent of the baseline used for stock composition estimation. This formed the starting point of our study, as previous simulation analyses had indicated sufficient resolution of stock composition estimates. These known-origin samples, which were derived entirely from existing baseline populations but were not included in estimation of population allele frequencies, generally provided reasonably accurate estimates of stock composition for the reporting regions. Analysis of mixture samples derived entirely from populations that are not included in the baseline provides a very challenging test of the ability of the genetic markers and the baseline to provide reliable regional estimates of stock composition. In essence, results from these analyses should provide the base of estimated accuracy of regional components. If estimated accuracy from an individual region is considered unsatisfactory, one possible solution to enhance accuracy is to survey additional populations from the region in order to provide a more-comprehensive basis on which to estimate stock compositions. Increasing the number of fish surveyed in each population within the region is another potential solution (Beacham et al. Citation2011a). Even if reliable stock composition estimates are obtained from known-origin samples derived entirely from populations included in the baseline, there is still a potential for inaccurate estimates of stock composition in real fisheries applications if a significant portion of the mixed-stock sample is derived from populations or regions that are inadequately represented in the baseline. The application of the baseline to estimation of stock composition for actual mixed-stock samples is a means to evaluate whether the presence of unsampled populations in the mixture will cause bias in estimated stock compositions.
The 10 markers outlined by Beacham et al. (Citation2001) for coho salmon stock identification included eight microsatellites and two MHC loci. The two MHC loci were analyzed individually through denaturing gradient gel electrophoresis, which required gels to be poured manually and samples to be loaded manually. Analysis of the MHC variation was more demanding technically than analysis of microsatellite variation, and results from the MHC analysis were available more slowly than those from the microsatellite analysis. Because of requirements for increasing resolution of estimated coho salmon stock composition in British Columbia applications, in the current study we redesigned the set of markers used for stock composition estimation to be entirely microsatellite based, with the proviso that the suite of microsatellites employed could be analyzed with no more than four injections on an automated DNA sequencer. To do so, we incorporated 10 new microsatellites in the application and we omitted one previously analyzed locus (Ots2) that displayed limited value in stock identification applications.
The baseline evaluated in the current study consisted of 274 populations with a geographical range from Russia to California, but the study centered on developing a baseline with an emphasis on British Columbia populations. Because coho salmon from regions outside of British Columbia can occur in fisheries within British Columbia, it is necessary to include representative populations from regions that may be represented in mixed-stock fishery samples. However, to apply this baseline to the estimation of stock compositions in fisheries outside of British Columbia, more-detailed sampling of baseline populations in the local area would be required.
In our study, we analyzed five mixed-stock samples of coho salmon with a 274-population baseline. Sample 1 (fish from the recreational fishery adjacent to Langara Island) was estimated to be dominated by coho salmon originating from rivers in northern or central coastal British Columbia. Given the location of the recreational fishery, we would expect that coho salmon from the north coast of the Queen Charlotte Islands would be observed in the catch. Contributions by Stikine, Nass, and Skeena River populations in northern British Columbia would also be expected to occur in the fishery. Similarly, contributions by populations from the Central Coast, northern Vancouver Island, and the northern South Coast were expected, as the fishery occurred prior to the beginning of the return spawning migration in the fall. Sample 2 (juveniles obtained from the Strait of Georgia after a few months of marine rearing) would logically be expected to contain fish originating from regions adjacent to the Strait of Georgia. Estimated stock composition of sample 2—largely originating from the Fraser River, the east coast of Vancouver Island, the southern South Coast, and northern Puget Sound—was consistent with the physical location of the sample and the life history stage sampled.
Sample 3 (coho salmon bycatch in a mid-September, pink salmon-directed fishery south of the Fraser River mouth) would be expected to contain salmon of largely Fraser River origin, with emphasis on those populations that have an earlier fall spawning migration. These were exactly the results obtained from the estimated stock composition. Coho salmon of Fraser River origin dominated the sample, and populations from regions upstream of the Fraser River canyon (middle Fraser, Lower Thompson, North Thompson, and South Thompson rivers) were estimated to contribute 33% of the sample. These results were in contrast to those obtained for sample 4 (fish from a mid-October, coho salmon-directed fishery near the San Juan Islands). The San Juan Islands fishery targets migrating coho salmon, and given its physical location we would expect coho salmon of Fraser River origin to constitute a sizable portion of the sample. Fish of Fraser River origin were identified as significant contributors to the sample (60%), but there was virtually no contribution (<1%) from populations upstream of the Fraser River canyon. The 1-month difference in timing for samples 3 and 4 resulted in a marked difference in the composition of the Fraser River-origin coho salmon in the catch, in accordance with the observed differences in migration timing between upper and lower river populations.
Given the physical location of sample 5 (coho salmon sampled in the Fraser River while migrating to their natal rivers for spawning), it should have consisted entirely of fish from populations upstream of the Harrison River's confluence with the Fraser River. These were exactly the results estimated from the mixed-stock analysis, as 100% of the fish were estimated to have originated from populations upstream from the Harrison River mouth. In summary, microsatellites provided reliable estimates of coho salmon stock composition in local fishery samples, even when there was a wide distribution of populations potentially available for allocation.
ACKNOWLEDGMENTS
A very substantial effort was undertaken to obtain the coho salmon samples used in this study. We thank J. C. Garza and D. J. Teel (National Marine Fisheries Service [NMFS]) for providing samples from California, Oregon, and the Columbia River; J. B. Shaklee (Washington Department of Fish and Wildlife) for providing samples from Washington State populations, including the Columbia River; various staff of the DFO and First Nations for baseline sample collection; and northern DFO staff for conducting or supervising the Skeena River and Central Coast collections. We also acknowledge the various agencies and organizations whose staff collected samples in northern British Columbia, including the Gitxsan Watershed Authority and the Skeena Fisheries Commission in the Skeena River drainage and the Nisga’a First Nation in the Nass River watershed. The Haida Fisheries Program assisted in collecting the Queen Charlotte Islands samples. We are grateful to R. L. Wilmot (NMFS Auke Bay Laboratory) and S. Miller (U.S. Fish and Wildlife Service, Anchorage Laboratory) for providing the southeast Alaska samples; P. Milligan (DFO, Whitehorse) for supervising collections in the Yukon River drainage within Canada; N. Varnavskaya (Kamchatka Research Institute of Fisheries and Oceanography) for providing samples from Russian populations; and G. Kirby (Northwest Indian Fisheries Commission) for providing the San Juan Islands fishery sample. L. Fitzpatrick drafted the map. Funding for this study was provided by DFO.
Subject editor: Donald Noakes, Thompson Rivers University, British Columbia, Canada
Notes
aOriginally incorporated by Beacham et al. (Citation2001).
aMixture sample size was set at 50 or fewer fish.
aTwelve microsatellites were used for estimation of stock composition (i.e., P53, Ocl8, Omy1011, One13, and Ots213 were excluded).
REFERENCES
- Anderson , E. C. 2010 . Assessing the power of informative subsets of loci for population assignment: standard methods are upwardly biased . Molecular Ecology Resources , 10 : 701 – 710 .
- Anderson , E. C. , Waples , R. S. and Kalinowski , S. T. 2008 . An improved method for predicting the accuracy of genetic stock identification . Canadian Journal of Fisheries and Aquatic Sciences , 65 : 1475 – 1486 .
- Baker , J. , Bentzen , P. and Moran , P. 2002 . Molecular markers distinguish coastal cutthroat trout from coastal rainbow trout/steelhead and their hybrids . Transactions of the American Fisheries Society , 131 : 404 – 417 .
- Banks , M. A. , Blouin , M. S. , Baldwin , B. A. , Rashbrook , V. K. , Fitzgerald , H. A. , Blankenship , S. M. and Hedgecock , D. 1999 . Isolation and inheritance of novel microsatellites in Chinook salmon (Oncorhynchus tshawytscha) . Journal of Heredity , 90 : 281 – 288 .
- Beacham , T. D. , Candy , J. R. , Jonsen , K. L. , Supernault , J. , Wetklo , M. , Deng , L. , Miller , K. M. and Withler , R. E. 2006 . Estimation of stock composition and individual identification of Chinook salmon across the Pacific Rim using microsatellite variation . Transactions of the American Fisheries Society , 135 : 861 – 888 .
- Beacham , T. D. , Candy , J. R. , McIntosh , B. , MacConnachie , C. , Tabata , A. , Kaukinen , K. , Deng , L. , Miller , K. M. , Withler , R. E. and Varnavskaya , N. V. 2005 . Estimation of stock composition and individual identification of sockeye salmon on a Pacific Rim basis using microsatellite and major histocompatibility complex variation . Transactions of the American Fisheries Society , 134 : 1124 – 1146 .
- Beacham , T. D. , Candy , J. R. , Supernault , K. J. , Ming , T. , Deagle , B. , Schultz , A. , Tuck , D. , Kaukinen , K. , Irvine , J. R. , Miller , K. M. and Withler , R. E. 2001 . Evaluation and application of microsatellite and major histocompatibility complex variation for stock identification of coho salmon in British Columbia . Transactions of the American Fisheries Society , 130 : 1116 – 1155 .
- Beacham , T. D. , Candy , J. R. , Wallace , C. , Urawa , S. , Sato , S. , Varnavskaya , N. V. , Le , K. D. and Wetklo , M. 2009 . Microsatellite stock identification of chum salmon on a Pacific Rim basis . North American Journal of Fisheries Management , 29 : 1757 – 1776 .
- Beacham , T. D. , McIntosh , B. and Wallace , C. 2011a . A comparison of polymorphism of genetic markers and population sample sizes required for mixed-stock analysis of sockeye salmon (Oncorhynchus nerka) in British Columbia . Canadian Journal of Fisheries and Aquatic Sciences , 68 : 550 – 562 .
- Beacham , T. D. , Varnavskaya , N. V. , Le , K. D. and Wetklo , M. 2008a . Determination of population structure and stock identification of chum salmon (Oncorhynchus keta) in Russia, determined with microsatellites . Fishery Bulletin , 106 : 245 – 256 .
- Beacham , T. D. , Wetklo , M. , Deng , L. and MacConnachie , C. 2011b . Coho salmon population structure in North America determined from microsatellites . Transactions of the American Fisheries Society , 140 : 253 – 270 .
- Beacham , T. D. , Wetklo , M. , Wallace , C. , Olsen , J. B. , Flannery , B. G. , Wenburg , J. K. , Templin , W. D. , Antonovich , A. and Seeb , L. W. 2008b . The application of microsatellites for stock identification of Yukon River Chinook salmon . North American Journal of Fisheries Management , 28 : 283 – 295 .
- Bucklin , K. A. , Banks , M. A. and Hedgecock , D. 2007 . Assessing genetic diversity of protected coho salmon (Oncorhynchus kisutch) populations in California . Canadian Journal of Fisheries and Aquatic Sciences , 64 : 30 – 42 .
- Cairney , M. , Taggart , J. B. and Hoyheim , B. 2000 . Characterization of microsatellite and minisatellite loci in Atlantic salmon (Salmo salar L.) and cross-species amplification in other salmonids . Molecular Ecology , 9 : 2175 – 2178 .
- Carlsson , J. 2008 . Effects of microsatellite null alleles on assignment testing . Journal of Heredity , 99 : 616 – 623 .
- Condrey , M. J. and Bentzen , P. 1998 . Characterization of coastal cutthroat trout (Oncorhynchus clarki clarki) microsatellites and their conservation in other salmonids . Molecular Ecology , 7 : 787 – 789 .
- Debevec , E. M. , Gates , R. B. , Masuda , M. , Pella , J. , Reynolds , J. M. and Seeb , L. W. 2000 . SPAM (version 3.2): statistics program for analyzing mixtures . Journal of Heredity , 91 : 509 – 510 .
- Ford , M. J. , Teel , D. , Van Doornik , D. M. , Kuligowski , D. and Lawson , P. W. 2004 . Genetic population structure of central Oregon Coast coho salmon (Oncorhynchus kisutch) . Conservation Genetics , 5 : 797 – 812 .
- Goudet , J. 1995 . FSTAT A program for IBM PC compatibles to calculate Weir and Cockerham's (1984) estimators of F-statistics (version 1.2) . Journal of Heredity , 86 : 485 – 486 .
- Greig , C. , Jacobson , J. P. and Banks , M. A. 2003 . New tetranucleotide microsatellites for fine-scale discrimination among endangered Chinook salmon (Oncorhynchus tshawytscha) . Molecular Ecology Notes , 3 : 376 – 379 .
- Griffiths , A. M. , Machado-Schiaffino , G. , Dillane , E. , Coughlan , J. , Horreo , J. L. , Bowkett , A. E. , Minting , P. , Toms , S. , Roche , W. , Gargan , P. , McGinnity , P. , Cross , T. , Bright , D. , Garcia-Vazquez , E. and Stevens , J. R. 2010 . Genetic stock identification of Atlantic salmon (Salmon salar) in the southern part of the European range . BMC Genetics [online serial] , 11 : 31
- Jefferts , K. B. , Bergman , P. K. and Fiscus , H. F. 1963 . A coded-wire identification system for macro-organisms . Nature (London) , 198 : 460 – 462 .
- Johnson , M. A. and Banks , M. A. 2008 . Genetic structure, migration, and patterns of allelic richness among coho salmon (Oncorhynchus kisutch) populations of the Oregon coast . Canadian Journal of Fisheries and Aquatic Sciences , 65 : 1274 – 1285 .
- Kalinowski , S. T. 2002 . How many alleles per locus should be used to estimate genetic distances? . Heredity , 88 : 62 – 65 .
- Kalinowski , S. T. 2004 . Genetic polymorphism and mixed-stock fisheries analysis . Canadian Journal of Fisheries and Aquatic Sciences , 61 : 1075 – 1082 .
- Neaves , P. I. , Wallace , C. G. , Candy , J. R. and Beacham , T. D. 2005 . CBayes: computer program for mixed stock analysis of allelic data, version v4.02 . Available: http://www.pac.dfo-mpo.gc.ca/sci/mgl/Cbayes_e.htm. (December 2011)
- Nelson , R. J. and Beacham , T. D. 1999 . Isolation and cross species amplification of microsatellite loci useful for study of Pacific salmon . Animal Genetics , 30 : 228 – 229 .
- O’Connell , M. , Danzmann , R. G. , Cornuet , J. M. , Wright , J. M. and Ferguson , M. M. 1997 . Differentiation of rainbow trout populations in Lake Ontario and the evaluation of the stepwise mutation and infinite allele mutation models using microsatellite variability . Canadian Journal of Fisheries and Aquatic Sciences , 54 : 1391 – 1399 .
- Olsen , J. B. , Bentzen , P. and Seeb , J. E. 1998 . Characterization of seven microsatellite loci derived from pink salmon . Molecular Ecology , 7 : 1087 – 1089 .
- Olsen , J. B. , Miller , S. J. , Spearman , W. J. and Wenburg , J. K. 2003 . Patterns of intra-and inter-population genetic diversity in Alaskan coho salmon: implications for conservation . Conservation Genetics , 4 : 557 – 569 .
- Olsen , J. B. , Wilson , S. L. , Kretschmer , E. J. , Jones , K. C. and Seeb , J. E. 2000 . Characterization of 14 tetranucleotide microsatellite loci derived from sockeye salmon . Molecular Ecology , 9 : 2185 – 2187 .
- Pella , J. and Masuda , M. 2001 . Bayesian methods for analysis of stock mixtures from genetic characters. U.S . National Marine Fisheries Service Fishery Bulletin , 99 : 151 – 167 .
- Rannala , B. and Mountain , J. L. Detecting immigration by using multilocus genotypes . Proceedings of the National Academy of Sciences of the USA . Vol. 94 , pp. 9197 – 9201 .
- Scribner , K. T. , Gust , J. R. and Fields , R. L. 1996 . Isolation and characterization of novel salmon microsatellite loci: cross-species amplification and population genetic applications . Canadian Journal of Fisheries and Aquatic Sciences , 53 : 833 – 841 .
- Small , M. P. , Beacham , T. D. , Withler , R. E. and Nelson , R. J. 1998a . Discriminating coho salmon (Oncorhynchus kisutch) populations within the Fraser River, British Columbia using microsatellite DNA markers . Molecular Ecology , 7 : 141 – 155 .
- Small , M. P. , Withler , R. E. and Beacham , T. D. 1998b . Population structure and stock identification of British Columbia coho salmon (Oncorhynchus kisutch) based on microsatellite DNA variation. U.S . National Marine Fisheries Service Fishery Bulletin , 96 : 843 – 858 .
- Smith , C. T. , Koop , B. F. and Nelson , R. J. 1998 . Isolation and characterization of coho salmon (Oncorhynchus kisutch) microsatellites and their use in other salmonids . Molecular Ecology , 7 : 1613 – 1621 .
- Smith , C. T. , Nelson , R. J. , Wood , C. C. and Koop , B. F. 2001 . Glacial biogeography of North American coho salmon (Oncorhynchus kisutch) . Molecular Ecology , 10 : 2775 – 2785 .
- Spies , I. B. , Brasier , D. J. , O’Reilly , P. T. L. , Seamons , T. R. and Bentzen , P. 2005 . Development and characterization of novel tetra-, tri-, and dinucleotide microsatellite markers in rainbow trout (Oncorhynchus mykiss) . Molecular Ecology Notes , 5 : 278 – 281 .
- Van Doornik , D. M. , Teel , D. J. , Kuligowski , D. R. , Morgan , C. A. and Casillas , E. 2007 . Genetic analyses provide insight into the early ocean stock distribution and survival of juvenile coho salmon off the coasts of Washington and Oregon . North American Journal of Fisheries Management , 27 : 220 – 237 .
- Weir , B. S. and Cockerham , C. C. 1984 . Estimating F-statistics for the analysis of population structure . Evolution , 38 : 1358 – 1370 .
- Williamson , K. S. , Cordes , J. F. and May , B. P. 2002 . Characterization of microsatellite loci in Chinook salmon (Oncorhynchus tshawytscha) and cross-species amplification in other salmonids . Molecular Ecology Notes , 2 : 17 – 19 .