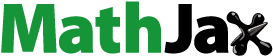
ABSTRACT
This study focuses on the long-term impacts of COVID-19 on telecommuting behavior. We seek to study the future of telecommuting, in the post-pandemic era, by capturing the evolution of observed behavior during the COVID-19 pandemic. To do so, we implemented a comprehensive multi-wave nationwide panel survey (the Future Survey) in the U.S. throughout 2020 and 2021. A panel Generalized Structural Equation Model (GSEM) was used to investigate the effects of two perceptual factors on telecommuting behavior: (1) perceived risk of COVID-19; and (2) perceived telecommuting productivity. The findings of this study reveal significant and positive impacts of productivity and COVID-risk perception on telecommuting behavior. Moreover, the findings indicate a potential shift in preferences toward telecommuting in the post-pandemic era for millennials, employees with long commute times, high-income, and highly educated employees. Overall, a potential increase in telecommuting frequency is expected in the post-pandemic era, with differences across socio-economic groups.
Introduction
The COVID-19 pandemic has affected how people socialize, work, shop, and travel worldwide, leading to severe health, economic, and social crises. By mid-July 2021, the World Health Organization (WHO) reported over 4 million deaths and more than 190 million infected cases (WHO Citation2021). Social distancing measures such as business lockdowns, travel restrictions, and COVID-19 case isolation are examples of protective measures that governments have established to slow down the spread of COVID-19. Meanwhile, many companies offered to telecommute to ensure business continuity during the pandemic, which accelerated its already growing trend.
Telecommuting is often defined as working remotely using Information and Communication Technologies (ICT) to reduce or eliminate daily commutes to and from work (Nilles Citation1988). Telecommuting can be divided into two classes: office-based and home-based. In office-based telecommuting, employees commute to a center closer to their home rather than commute to the main office. In contrast, in home-based telecommuting, employees do not commute and work remotely from home, which is the focus of this study. In the rest of this article, when we talk about telecommuting, we refer to the working from home (WFH) class of telecommuting.
Telecommuting helps alleviate environmental concerns by decreasing emissions and energy consumption (Koenig, Henderson, and Mokhtarian Citation1996; Mokhtarian, Handy, and Salomon Citation1995; Mokhtarian and Varma Citation1998; Zhu and Mason Citation2014). Moreover, it provides non-transportation-related benefits for employees (e.g. flexible work hours at home). Besides, there are possible cost savings for employees, such as daily commute expenses. Telecommuting may also be advantageous for employees with disabilities or those taking care of a dependent (Mahmassani et al. Citation1993). From the employers’ perspective, businesses can experience a reduction in operational costs, such as office spaces, facilities, and parking (Collins and Moschler Citation2009; Manoochehri and Pinkerton Citation2003). Despite these benefits, one of the most significant disadvantages of telecommuting is the feeling of isolation due to the lack of social interaction andcollaboration (Baruch Citation2001; Collins and Moschler Citation2009; Manoochehri and Pinkerton Citation2003). Furthermore, some employees may find the home environment distracting and inconvenient, resulting in lower telecommuting productivity. From employers’ perspective, potential high initial investments in telecommuting services could discourage businesses from adopting it (Mahmassani et al. Citation1993). In addition, flexibility of telecommuting should be properly managed otherwise it could lead to longer working hours and conflict life work boundaries (Ahrendt et al. Citation2020; DeFilippis et al. Citation2020; ILO Citation2021; The Economist Citation2020).
Although pandemic-induced telecommuting occurred out of necessity, many businesses are satisfied with this experience. They intend to continue telecommuting even when COVID-19 is no longer a concern (Business Insider Citation2020; CNN Citation2020; Courtney Citation2021; Salon et al. Citation2021a, Citation2021b). According to the Brookings Institution, up to half of the American employees now telecommute, which is more than twice what before the pandemic (Brooking Institution Citation2020). Given how receptive companies and employees are, there may be a long-term shift in favor of telecommuting (Brooking Institution Citation2020; Washington Post Citation2020). However, the future to a great extent remains unknown and must be researched.
This article sheds light on how telecommuting behavior evolved during the pandemic and how it will continue in the post-pandemic era, while it incorporates the effects of perceived productivity at home and the risk of exposure to COVID-19 on this evolution. In other words, we aim to confirm two hypotheses: First, the level of productivity that employees perceive influences their telecommuting behavior. Second, the level of the perceived risk of exposure to COVID-19 is correlated with telecommuting frequency. To achieve our goal, a generalized structural equation (GSEM) framework with a panel structure is developed in which we understand the underlying factors that define telecommuting productivity and risk perception and how such factors affect employees’ preferences to continue telecommuting at two different times during the pandemic as well as in the post-pandemic future. The findings of this study are built based on a comprehensive two-wave panel survey that we conducted in the U.S. from April 2020 to May 2021, exploring how COVID-19 has reshaped people’s activity-travel behavior.
The rest of this paper is organized as follows: in the following section, we briefly review previous studies on telecommuting before the pandemic, our proposed hypotheses, and telecommuting during the pandemic and after, then, we address how the current study contributes to the literature. In Section 3, we discuss a descriptive analysis of the data source. Next, we present the formulation of the GSEM framework in section 4. Section 5 then discusses the structure of the estimated model as well as detailed estimation results. We explore research findings in terms of policy implications in section 6. The article concludes with a summary of the key findings and suggestions for future research.
Literature review
Telecommuting before the pandemic
Telecommuting has always caught the interest of researchers toward exploring its potential benefits and assessing its inherent limitations. The term ‘telecommute’ was first discussed by Nilles in 1975 (Nilles, Gray, and Nilles Citation1975). Later in 1988, Nilles (Nilles Citation1988) discussed all types of telecommuting in detail and offered it as a work option to reduce commuting trips. Then, in 1991, Mokhtarian (Mokhtarian Citation1991) defined and discussed in depth the various aspects of telecommuting. Telecommuting rapidly got accepted all over the world in the early years of introducing it. The federal government and several states in the U.S. and many European countries started implementing telecommuting at that time, evidenced by Mokhtarian (Mokhtarian Citation1991),
There is a vast body of research on analyzing and characterizing telecommuting behavior before the pandemic (Asgari, Jin, and Mohseni Citation2014; Bagley and Mokhtarian Citation1997; Bernardino and Ben-Akiva Citation1996; Adriana Bernardino et al., Citation1993; Drucker and Khattak Citation2000; Hjorthol Citation2013; Matson et al. Citation2021a; Shabanpour et al. Citation2018; Yen and Mahmassani Citation1997). The pre-pandemic literature found socio-demographics, job characteristics, attitudes, and commute trip features to be important in telecommuting choice and frequency. In the following, we discuss these factors in detail.
The impact of socio-demographic characteristics on telecommuting behavior has been examined in several studies (Bagley and Mokhtarian Citation1997; Bernardino and Ben-Akiva Citation1996; Adriana Bernardino et al., Citation1993; Drucker and Khattak Citation2000; Pouri and Bhat Citation2003; Shabanpour et al. Citation2018; Yap and Tng Citation1990). For example, Bernardino et al. (Bernardino and Ben-Akiva Citation1996; Adriana Bernardino et al., Citation1993) showed that women are more likely than men to telecommute because of childcare. Moreover, Pouri et al. (Pouri and Bhat Citation2003) investigated the impact of age on telecommuting and found that older people are less likely to adopt it. The literature has also revealed the importance of education, income, having prior experience, and job category in determining telecommuting choice and frequency (Bagley and Mokhtarian Citation1997; Bernardino and Ben-Akiva Citation1996; Adriana Bernardino et al., Citation1993; Drucker and Khattak Citation2000; Shabanpour et al. Citation2018). Commute features have been frequently stated as a determinant of telecommuting frequency. The commute time has been seen to be positively correlated with telecommuting frequency (Bagley and Mokhtarian Citation1997). Shabanpour et al. (Shabanpour et al. Citation2018) investigated the impact of vehicle ownership as a key factor on telecommuting frequency and found that since having a private vehicle makes commuting easier, it decreases telecommute frequency.
Telecommuting during the pandemic and after
The literature on telecommuting during the pandemic and after is limited but growing (Balbontin et al. Citation2021; Beck, Hensher, and Wei Citation2020a; Beck and Hensher Citation2020a; Conway et al. Citation2020; Hensher, Beck, and Wei Citation2021; Javadinasr et al. Citation2022; Maria Barrero Nicholas Bloom Steven Davis et al. Citation2021; Matson et al. Citation2021b, Citation2021a; Nguyen Citation2021a; Salon et al. Citation2021b, Citation2021a; Shamshiripour et al. Citation2020). Nguyen (Citation2021a) investigated the influential factors on telecommuting behavior in Vietnam during and after the pandemic. In another study in Vietnam, Nguyen and Armoogum (Citation2021) explored the gender-based differences in perceptions and attitudes toward telecommuting. Baert et al. (Citation2020) studied perceptions toward telecommuting in Belgium.
Beck and Hensher (Beck and Hensher Citation2020a, Citation2020b) conducted an online survey at the beginning of the pandemic studied how people’s travel patterns and activities including telecommuting have changed in Australia. Using the wave 1 of the survey, Beck and Hensher (Citation2021) developed an ordered logit model to measure the number of days telecommuting in a day. Based on the second wave, they (Beck, Hensher, and Wei Citation2020a) modeled the impact on telecommuting frequency due to the slow relaxing of restrictions in Australia. Balbontin et al. (Citation2021) studied telecommuting behavior in Australia, South America, and South Africa and estimated the weekly frequency of it during the pandemic. The U.S. National Bureau of Economic Research conducted the most extensive survey to study the persistence of telecommuting after the pandemic and found %20 of full workdays will be supplied from home in post-pandemic (Maria Barrero Nicholas Bloom Steven Davis et al. Citation2021). Studies conducted during the pandemic mostly focused on understanding employees’ perceptions and attitudes toward telecommuting behavior as the effects of these factors on preferences for the continuity of telecommuting have been highlighted since COVID-19.
Proposed hypotheses
The majority of pre-pandemic studies frequently discussed how telecommuting affects employees’ productivity (Baruch Citation2001; Collins and Moschler Citation2009; Manoochehri and Pinkerton Citation2003). However, a few of the during the pandemic studies examined the effects of productivity on employees’ preferences toward telecommuting (Beck, Hensher, and Wei Citation2020b; Nguyen and Armoogum Citation2021). A positive relationship between the level of productivity and telecommuting frequency has been found in the literature indicating that the more productive employees are at home, the more frequent they telecommute. Nguyen and Armoogum (Citation2021) discussed productivity by looking into how the difficulty of focusing at home affects perceptions toward telecommuting.
Productivity varies across different groups of demographics. Female employees perceive the home environment as more distracting than males (Plaut Citation2006). The Household’s number of bedrooms affects productivity by accommodating a dedicated and comfortable workplace. An early survey conducted in Singapore indicated that single women with dedicated home workspace are more likely to telecommute. The study highlighted the importance of a comfortable and not distracting workspace at home as well as the significant effects of childcare on telecommuting adoption (Yap and Tng Citation1990).
Positive impacts of the number of children or the presence of young children in the household on telecommuting adoption frequently discussed in the pre-pandemic literature (A Bernardino et al., Citation1993; Bernardino and Ben-Akiva Citation1996; Yen and Mahmassani Citation1997). However, a few of recent studies found that childcare can decrease the productivity of parent employees at home, resulting in their lower willingness to continue telecommuting (Baert et al. Citation2020; Nguyen Citation2021b; Nguyen and Armoogum Citation2021). Feeling isolation, another inhibitor of productivity, is the other commonly mentioned factor influencing employees’ preferences toward telecommuting (Baruch Citation2001; Collins and Moschler Citation2009; Manoochehri and Pinkerton Citation2003). The possible conflicts of work and personal life boundary, a determinant of productivity, also discussed in the literature (Collins and Moschler Citation2009; Vyas and Butakhieo Citation2020) negatively influencing telecommuting frequency. Furthermore, the availability of required equipment or technology at home affecting telecommuting productivity has been pointed out frequently in previous studies (A Bernardino et al., Citation1993; Maria Barrero Nicholas Bloom Steven Davis et al. Citation2021).
Every crisis rises risk perception and hazard acceptance. People’s need for security grows as their fear of COVID-19 and personal risk perception grows (Kohler et al. Citation2021). Perić, Dramićanin, and Conić (Citation2021) showed that risk perception negatively affects travel intentions in Serbia during COVID-19 pandemic. Nguyen and Armoogum (Citation2021, Citation2021) found that fear of COVID-19 is a strong positive factor influencing perceptions toward telecommuting during the pandemic. Beck and Hensher (Citation2021) revealed that majority of employers and employees show some concern about the risk of COVID-19 in their workplace.
Research gaps and contribution
There are some research gaps exist in both pre and during the pandemic literature. In the pre-pandemic literature, a few studies explored the effects of perceptual factors, such as productivity on employees’ preferences toward telecommuting. Moreover, much has changed since the pre-pandemic time, when many people did not have the option or did not want to telecommute. However, the pandemic forced many employees and employers who could telecommute to do so. During the pandemic, literature mostly examined the short-term effects of COVID-19 on telecommuting as they were conducted at the beginning of the pandemic. However, people have experienced many changes in the 2 years since the pandemic, including more understanding about the virus, mandate lifting, vaccination arrival, all of which have raised hopes and resulted in changes in perceptions and preferences. The ever-changing nature of the pandemic requires a panel study to capture the evolution of people’s activity-travel behavior for the long-term future. Furthermore, many of the during the pandemic studies understand the changes in telecommuting behavior based on a data descriptive method rather than characterizing. Characterizing the long-term impacts of the pandemic on telecommuting necessitates looking beyond the individual characteristics, job features, and household factor effects. We need to understand the effects of changes in some behaviors. Risk perception and employees’ perceptions of their telecommuting productivity can be among the most significant behavioral changes brought on by the COVID-19 pandemic.
This article investigates the long-term impact of the COVID-19 pandemic on telecommuting frequency while accounting for (1) risk perception associated with exposure to the COVID-19 (referred to as ‘risk perception’) at two times during the pandemic, and (2) perceived productivity at home (referred to as ‘productive telecommuting’). Furthermore, we explore the underlying factors that lead to the persistence of telecommuting after the pandemic. To do so, we conducted a comprehensive multi-wave activity-travel behavior survey in the U.S. from April 2020 to May 2021. We develop a panel Generalized Structural Equation Model (GSEM). Then we investigate the relationships between productivity, risk perception, and telecommuting behavior during and after the pandemic.
The contributions of this study are fourfold: first, to the best of our knowledge, this is the first study using multi-wave nationwide data in the U.S. to measure the COVID-19 impact on telecommuting behavior during and post-pandemic era. Another notable contribution of this study is taking into account ‘new telecommuters’ who started telecommuting at the beginning of the pandemic. This provides a unique opportunity to investigate attitudes and concerns toward telecommuting based on real-life experiences. Furthermore, we characterize how risk perception associated with crises like the COVID-19 pandemic affects employees’ preferences toward telecommuting. Fourth, we illustrate how telecommuting behavior changes as people get familiar with the crisis, and risk perception might change.
Data
Survey
The datasource of this study is obtained from an extensive nationwide survey in the United States that we conducted to investigate the effects of the COVID-19 pandemic on activity-travel behavior (the Future Survey). The COVID Future survey was distributed in two waves containing 8,723 responses in wave 1 and 3111 respondents in wave 2. Waves 1 and 2 were administered via the Qualtrics online platform from April to October 2020, and November 2020 to May 2021, respectively. The collected data contains three sets of features: (1) socio-demographic characteristics such as age, gender, race, annual household income, job category, location of residence, along with household-related information such as household structure and the number of vehicles in the household; (2) activity-travel behavior information such as individuals’ commuting and telecommuting, online and in-person shopping, and online and in-person learning behaviors and attitudes; (3) Health-related information, such as whether individuals or their household members were infected with COVID-19 and whether they were vaccinated or intended to get vaccinated. Other works that use the data can be found in (Chauhan et al. Citation2021; Conway et al. Citation2020; Javadinasr et al. Citation2022; Salon et al. Citation2021b).
To analyze telecommuting behavior evolution during the pandemic and its future once the pandemic has ceased to be a concern, we need to focus on those who can judge their telecommuting behavior before the pandemic, during two waves of the survey, and after the pandemic. Therefore, we need to narrow down our sample to those who were employed before and during the pandemic and expect themselves to be employed in the post-pandemic. Furthermore, we seek to confirm the effects of perceived productivity at home on preferences toward telecommuting, which requires studying those who have done so during the pandemic. The final sample includes 583 employees who telecommuted at least some of the time during the pandemic. illustrates the spatial distribution of the final sample.
Analytical framework
The developed panel GSEM model in this study estimates (1) how ‘new telecommuters’ and ‘continuing telecommuters’ perceive their telecommuting to be productive; (2) how they perceive the risk of COVID-19 exposure at two different times during the pandemic, and (3) how their productivity and risk perception affect their preferences for continuing telecommuting during and after the pandemic. To estimate productivity latent construct and telecommuting frequency at two waves of the survey and post-pandemic future, which are outcomes, we extract data from the following questions. (i) Did the respondent telecommute at the time of the survey? If so, at the time of the survey, how many days in the past 7 days they telecommuted? (ii) If telecommuted, how their productivity has changed compared to before the pandemic? The respondent reported the change in their productivity through a 5-point Likert scale (i.e. significantly lower, some lower, …). (iii) If productivity changed, they select the reasons for the change in their productivity from the given factors. Hence, productivity decrease/increase factors are binary in nature. (iv) At the time of the survey, how frequently did they expect to telecommute in the future when COVID-19 is no longer a concern are asked? The respondent reported their expectation through categories of ‘never,’ ‘a few times a year,’ ‘a few times a month,’ ‘once a week,’ ‘a few times a week,’ and ‘every day.’
Estimations revealed that thresholds for ‘a few times a year’ and ‘once a week’ in telecommuting expectation outcome are insignificant. Therefore, to improve the model, we merged ‘a few times a year’ and ‘never’ categories into one, namely ‘never.’ In addition, we considered ‘a few times a month’ and ‘once a week’ categories together as a single category, namely ‘a few times a month.’ Hence, the telecommuting frequency is modeled through four categories in the developed GSEM framework: (1) never, (2) a few times a month, (3) a few times a week, and (4) every day. To ensure consistency in telecommuting frequency classification, the frequency during the pandemic is classified as follows. First, we classify those employees who did not telecommute in the past 7 days as the ‘never’ category. Following that, those who reported telecommuting only 1 day were assigned to the ‘a few times a month’ category. Third, those who telecommuted between 2 and 4 days fall into the ‘a few times a week’ category. The remaining respondents who telecommuted 5 or more days in the past 7 days are classified as having an ‘every day’ frequency.
The descriptive analysis of the data provides intriguing insights into how telecommuting behavior has changed during the pandemic and the projection of the post-pandemic future. As shown in (a), the findings indicate that 45% of employees who experienced telecommuting for the first time during the pandemic expect to continue it frequently (i.e. a few times a week or every day) after the pandemic. About 79% of those who telecommuted every day before the pandemic are expected to do so in the future. represents the changes in telecommuting frequency during the pandemic. Nearly 54% of employees who never had telecommuted at wave 1 experienced it a few times a week or every day during wave 2. It is also shown that only 5% of employees who frequently telecommuted during wave 1 reduced the frequency to never at wave 2.
In the risk perception data collection, people were asked to tell us how much they perceive the risk of exposure to COVID-19 when they shop at a grocery store, ride public transit, take a taxi or ride-hailing service, travel in an airplane, and send their children to school. They rated their risk perception for each activity using a 5-point Likert scale (i.e. extremely low risk, low risk, …). The descriptive analysis of data shown in , reveals that the distribution of risk perception levels for different activities skewed to the right in wave 2 compared to wave 1. This indicates that the proportion of those who were extremely concerned about risk in wave 1 decreased in wave 2. With a decrease in risk perception, we observe a decrease in the proportion of everyday telecommuting, meaning a decrease in telecommuting frequency indicating the interaction between risk perception and telecommuting behavior.
We assume that perceived productivity at home did not significantly change during the pandemic between two waves of the survey. The logic behind the assumption is that there was a couple of months between the two waves of the survey, which is probably too short for perceived productivity at home to significantly change. Our analysis of the productivity distribution shown in illustrates a difference of less than 5% between two waves in all levels of change in productivity, supporting the assumption. However, to test the validity of our assumption, we estimated the model considering two latent constructs for perceived productivity to capture the changes in productivity during the pandemic. The results revealed that measurement variables which are productivity decreased/increased reasons are significant in estimating only one of the productivity latent constructs. This indicates that productivity at wave 1 and productivity at wave 2 are not significantly different to incorporate both in the model. We used wave 2 productivity decreased/increased reasons since (1) we aim to use the most recent information to accurately estimate perceived productivity at home. (2) Some of the significant productivity decreased/increased reasons, such as feeling sad/depressed were collected only in the second wave of the survey. presents summary statistics for the significant factors of the data used in the model estimation.
Table 1. Summary statistics of the significant explanatory variables in the final model (Sample size = 583 observations).
Method
This study aims to capture how telecommuting frequency changes over time during and after the pandemic while also risk perception might change. This section explains the interrelationship between three outcome variables, three latent constructs, and observed determinants (as outlined in) in a panel Generalized Structural Equation Modeling (GSEM) framework using a balanced panel dataset. A balanced panel dataset contains a sample of N individuals observed at T times (i.e. ). This study’s balanced panel data source includes 583 individuals, all of whom were observed twice (i.e. waves 1 and 2 of the survey). In addition, respondents reported their telecommuting frequency before the pandemic and the expected frequency of continuing it in the post-pandemic future.
In the panel/longitudinal structure of GSEM, we aim to estimate the relationship between and within the observed and latent variables repeated at different times. The outcomes are defined as ordinal outcomes with logit links, which captured through a four-point scale. Stata 16 is employed to build and estimate the framework. The following is the formulation of the developed panel GSEM model with ordinal outcomes and logit links (Acock, Citation2013; Bollen and Noble Citation2011; Williams, Allison, and Moral-Benito Banco de España Madrid Citation2018). The GSEM model breaks down into two parts: the latent variable model and the measurement model. The latent variable model is:
where,
is the vector of the latent endogenous variable for individual
,
is the vector of intercepts,
is the matrix of coefficients reflecting the potential impacts of the latent endogenous variables
on each other,
is the vector of latent exogenous variables,
is the matrix of coefficients indicating the potential impacts of the latent exogenous variables
on the latent endogenous variables
,
is the vector of observed exogenous variables for individual
,
is the matrix of coefficients representing the potential impacts of the observed exogenous variables
on the latent endogenous variables
, and
is the random disturbances.
Exogenous variables are those that are not described by other variables in the model. In contrast, endogenous variables are those that are directly explained by other variables in the model. Latent exogenous variables () are hypothesized to be normally distributed in which
is the covariance matrix,
). We assume that random disturbances have a normal distribution,
), where
is the covariance matrix of the random disturbances (
). The latent variable model captures the study hypotheses about how telecommuting productivity and perceived risk of exposure to COVID-19 affects preferences toward telecommuting behavior during and after the pandemic and how preferences change as risk perception changes.
The measurement model connects the latent variables to the observed variables. In the panel structure of the measurement model, the outcome variable at time (
) is estimated by the independent variable at time
(
) the dependent variable at time
(
) and latent-constructs (
). Here, we model time-variant dependent variable and latent construct, yet the independent variables including socio-demographics, job features, and commute trip characteristics before the pandemic are time-invariant. Therefore, to estimate the outcome variable at time
(
) we use time-invariant independent variable (
) and latent construct (
) (Jöreskog and Sörbom Citation2018). EquationEquation (2)
(2)
(2) and (Equation3
(3)
(3) ) represent the formulation of the developed measurement model.
where,
and
are the unobservable time-invariant fixed effect,
is the time-variant error term,
and
capture all unobserved factors across individuals on
and
, respectively,
is the matrix of coefficients indicating the potential impacts of the observed exogenous variables on the observed endogenous variables (i.e. outcome),
is the matrix of coefficients indicating the potential impacts of the observed endogenous variables at time
on the observed endogenous variables at time
,
is the matrix of coefficients indicating the potential effects of the endogenous latent variables (
) on the observed endogenous variables
),
is the matrix of coefficients reflecting the potential effects of the exogenous latent variables
on the observed exogenous variables (
,
Consider as the ordinal outcome variable,
as the number of discrete values that
can take (i.e.
in this study), and
as the utility of latent variables that varies across individual
.
is also known as the linear prediction of
which is transformed into the discrete values
through the thresholds
. The probability that a random outcome variable
has the value
is given by:
where represents the primary stochastic constituent of
. The transformation of the linear prediction of
(i.e.
), to the mean value of
(i.e.
), is described by a logit link function as follows:
or equivalently:
Results
In this section, we present the findings of this study. As stated earlier, this study investigates how COVID-19 has reshaped telecommuting behavior and how the future looks in the long term while exploring the influential factors. We apply a panel generalized structural equation model (GSEM). The model incorporates three latent constructs, including productive telecommuting and risk perception at two different times during the pandemic: survey wave 1 (i.e. April to October 2020) and survey wave 2 (i.e. November 2020 to May 2021). displays the structure of the developed GSEM framework. The model captures telecommuting frequency at three times: two waves of the survey and after the pandemic. In addition to latent constructs and observed determinants, telecommuting frequency at each time is predicted by the telecommuting frequency at the previous time. The final estimation results are presented in .
Figure 4. The generalized structural equation modeling structure.
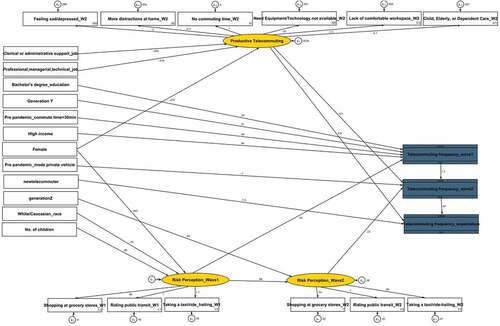
Table 2. Estimation results of the generalized structural equation model.
The results reveal the significant impact of productivity on telecommuting frequency during and after the pandemic at 85% and 90% confidence levels, respectively. In addition, COVID-19 risk perception is shown to significantly affect telecommuting frequency during the pandemic, at waves 1 and 2 of the survey at 85% and 90% confidence levels, respectively. Furthermore, the COVID-19 risk perception in the model has an indirect and positive effect on telecommuting behavior expectations after the pandemic. The ‘Productive telecommuting’ latent variable is estimated by six observed perceptual factors, while the ‘COVID-19 risk perception’ latent constructs at waves 1 and 2 of the survey are estimated by three. The coefficient of one of the perception factors is constrained to one to adjust the scale of latent variables.
The findings suggest that saving the time and energy spent on daily commutes for work-related activities leads employees to perceive telecommuting as productive, which is in line with the literature (Maria Barrero Nicholas Bloom Steven Davis et al. Citation2021). In contrast, a distracting environment and lack of comfortable workspace at home inhibit employees from being productive. In addition, child, elderly, or dependent care, need equipment/technology not available at home, feeling sad or depressed are negatively associated with employees’ perceptions toward productive telecommuting. The positive and strong correlation between productivity telecommuting frequency during the pandemic, at wave 2, and after the pandemic emphasizes the importance of designing ‘workable homes’ when planning for the future. Workable homes have a convenient workspace and the appropriate environment to telecommute productively in it.
The results show the significance of gender and job category in employees’ perceptions toward their telecommuting productivity. Females are more vulnerable to the low levels of productive telecommuting. The potential explanation is that females traditionally feel more responsible than males for housework, such as childcare, housekeeping, and other household activities (Bailyn Citation1989; Plaut Citation2006). In terms of job category impacts, the findings indicate that employees with professional, managerial, or technical jobs are less likely to have productive telecommuting. The same is shown for clerical or administrative work. This could be due to these employees’ type of job responsibilities, which cannot be performed efficiently at home. Professional, managerial, or technical jobs involve supervision and control of the production process, which might require the employee’s presence in the office (Shabanpour et al. Citation2018). Moreover, the clerical or administrative support job category includes daily office responsibilities, which are difficult to be accomplished at home. These two groups of employees are essential to the office and may be required to be present in order to communicate with others in person. This is also in line with the literature. Sassen (Citation2001) discusses that globalization generates an increased need for face-to-face interaction because of the command-and-control requirements of large enterprises.
The sub-model estimating COVID-19 risk perception at waves 1 and 2 of the survey reveals that employees who are concerned about the risk of exposure to COVID-19 telecommute more frequently during the pandemic. This is also consistent with previous studies, which show that as COVID-19 risk perception increases, going-out self-restriction increases (Parady, Taniguchi, and Takami Citation2020). The significant impact of risk perception at wave 2 highlights the importance of including the contributing factors to risk perception when exploring the effects of COVID-19 on telecommuting behavior. Regarding panel effects, risk perception at wave 1 of the survey is positively and significantly associated with risk perception at wave 2. This indicates that those who were concerned about the risk of exposure to COVID-19 at public places or facilities still perceived the risk at wave 2.
The results indicate gender, race, age, and the number of children as the underlying factors of risk perception. Females are more concerned about being exposed to COVID-19 than males, as evidenced by the literature (Abdelrahman Citation2020; Niño et al. Citation2021; Rana et al. Citation2021). It is demonstrated that White/Caucasian people are less averse to the risk of COVID-19 exposure. Generation Z is also shown to perceive lower levels of risk. Niño et al. (Citation2021) also showed that risk perception significantly differs across different age groups and different races. In terms of indirect effects, white people and Generation Z are not likely to telecommute frequently. The findings reveal a positive correlation between the number of children in the household and risk perception indicating that the likelihood of avoiding COVID-19 exposure increases with the number of children in the household.
Education and income are the other important factors that positively and directly contribute to telecommuting frequency. This could be because high-educated, and high-income employees may have higher ranks in their companies, thus having more bargaining power with their employers (Mannering and Mokhtarian Citation1995; Peters, Tijdens, and Wetzels Citation2004; Shabanpour et al. Citation2018). In addition, the findings demonstrate that age is another important indicator of telecommuting frequency. According to the findings, Generation Y or millennials who are between 25 and 40 years old are positively correlated with telecommute frequency. The potential explanation is that generation Y might be more familiar with working with ICT devices; therefore, they are more comfortable with telecommuting.
The contribution of commute trip features to telecommuting frequency is also explored. The findings indicate the positive correlation between a long commute trip (i.e. more than 30 minutes) and telecommuting frequency, also mentioned in previous studies (Mokhtarian and Meenakshisundaram Citation2010; Shabanpour et al. Citation2018). Employees who commute with their private vehicles are not expected to telecommute frequently. This could be because having a private vehicle makes the commute easier, and as a result, decreases telecommuting frequency.
In terms of the panel effects, the results reveal that telecommuting behavior during wave 1 of the survey (i.e. April to October 2020) is positively associated with behavior at wave 2 (i.e. November 2020 to May 2021). The same pattern can be seen between the frequency of telecommuting at wave 2 of the survey and the future, indicating that employees who frequently telecommuted during the pandemic, at wave 2, are inclined to do so after the pandemic. This could be due to the experience employees gained during the pandemic, which makes them familiar and comfortable with telecommuting.
Interestingly, the findings reveal that ‘new telecommuters’ who started telecommuting during the pandemic with no prior experience are not likely to continue it frequently in the post-pandemic future. Although new telecommuters might perceive their home environment to be convenient to telecommuting, they may consider their home environment as distracting and thus be less productive at home, which reduces their willingness to telecommute frequently (Cummins Citation2020).
The marginal effect analysis, shown in , also suggests that new telecommuters do not prefer to continue it frequently after the pandemic. As illustrated in , being a new telecommuter reduces the ‘every day’ frequency by up to 29%. The analysis, on the other hand, finds that ‘new telecommuter’ has a positive effect on the lowest frequency category (i.e. ‘never’), which is as high as 25%. Similarly, new telecommuters positively impact the ‘a few times a month’ category, which can be counted as infrequent telecommuting. And they negatively affect the ‘a few times a week’ category, which can be considered frequent telecommuting. However, the impacts on ‘a few times a month’ are not as high as those of ‘never.’ Likewise, new telecommuters do not affect the ‘a few times a week’ category as much as the ‘every day’ frequency.
Policy implications
In this section, we discuss the findings from the standpoint of policymakers and planners. The findings of this study point to two crucial factors that influence telecommuting frequency during and after the pandemic: (1) perceived productivity and (2) risk perception. The first idea for policy implication can be inferred from the effects of employees’ perceptions toward their home environment and telecommuting benefits (e.g. no commuting time) on their productivity. Lack of comfortable workspace, more distractions at home, childcare, and feeling depressed are all productivity inhibitors originating from home environment characteristics and interactions.
To understand the future of telecommuting, we should first consider how convenient it is for employees. The convenience of telecommuting can be derived from how much employees perceive their workspace at home to be comfortable and the availability of required equipment/technology. Businesses can make telecommuting more convenient by providing the devices needed at home as much as it is possible. However, it is prohibitively expensive or impossible to bring the technology needed to employees’ homes in some job categories. The findings also show that the job category influences productive telecommuting. This could indicate that some job-related tasks are inconvenient and inefficient to complete at home.
Employees’ perception of distraction in their home environment is another major determinant of productivity. Although household interactions might cause most distractions at home, part of it could be due to the workability of the home environment. Workable homes, where employees have dedicated workspace and a productive environment, provide telecommuting opportunities in normal situations or during a similar crisis to the COVID-19 pandemic (Allam and Newman Citation2018). Given that workable homes increase the likelihood of telecommuting, one could argue that having workable home environments allows for better continuity of teleworking with less chance of disruption, potentially increasing the resiliency of such telecommuters.
The second idea regarding policy implications is related to one of the productivity inhibitors: feeling depressed or sad. Some employees experience depression when telecommuting since they feel isolated at home. Engagement in teamwork and connectivity to other employees make telecommuters productive and happy at home. In today’s modern world, the ease of using instant messages and chat services has made them the dominant way of communication. The video-integrated chat services are highly required to make telecommuters feel connected to other employees as much as their in-office colleagues do. Therefore, frequent virtual calls and video conferences should be considered a key strategy of the telecommuting program. As a full-time telecommuter in the marketing director position at Pancheros Mexican Grills restaurants, Reid Travis emphasizes the importance of video-integrated services by stating that video-integrated chat services have saved his company’s culture (D’Angelo Citation2020). Furthermore, having dedicated virtual happy hours or fun talks can also help telecommuters feel more connected to the office. Travis suggests businesses make sure that their telecommuters feel like they are a part of the team, which will improve their productivity (D’Angelo Citation2020).
The third policy implication idea concerns the significant effects of child, elderly, or dependent care on employee productivity. Childcare or other household chores have affected many women and some men employees’ telecommuting productivity. The different potential amount of engagement in household chores, including childcare, between the couples can significantly affect women’s business participation, resulting in gender inequality (Del Boca et al. Citation2020). Along with other policies, the Italian government has implemented two national guidelines to assist families in maintaining a work-life balance. First, offering an extra period of parental leave to families with children younger than 12. Second, offering a babysitter voucher. The extra paternal leave period is up to 30 days with 30% of the parents’ salaries. According to the data released by the Italian National Social Security in May 2020, 76% of requests for extra parental leave have been submitted by women employees. This suggests that women may be more involved in childcare and face greater pressure to maintain a work-life balance (Del Boca et al. Citation2020).
Risk perception is one of the strong predictors of telecommuting behavior during the pandemic. Employees who perceive a higher risk of COVID-19 exposure telecommute more frequently. Risk perception is significantly correlated with telecommuting frequency as long as COVID-19 is a concern for most people. However, people may perceive the risk of COVID-19 exposure as decreasing as they become more familiar with the COVID-19 crisis. This is also in line with the literature indicating that familiar crises are perceived as less risky than unknown and novel crises (Siegrist, Luchsinger, and Bearth Citation2021; Siegrist, Keller, and Kiers Citation2005).
Getting know how to manage COVID-19 crisis, businesses trying to prepare safe and healthy workplaces. According to Harvard Business Review (Citation2021), a few employers have moved to return their employees to offices since they believe in better collaboration with face-to-face interaction and higher productivity at offices. Forcing employees to get back to offices ignores their perceived risk of exposure to COVID-19 at workplaces, which threatens both their physical and mental health. Falco et al. (Citation2021) have shown that the perceived risk of exposure to COVID-19 at workplace positively affects emotional exhaustion, which is negatively correlated with communication and decision-making. At this point, employees with high-risk perception might involve in activities to protect themselves from being infected and continuously concern about safety-related events resulting in lower productivity in offices. Risk perception varies different socio-demographic groups. We found that gender, race, and age are significant predictors of risk perception.
The fourth policy implication idea is about the persistence of telecommuting after the pandemic is no longer a concern. The stickiness to telecommuting is affected by both employees’ and employers’ preferences and intentions. Evidence by Bagley and Mokhtarian (Citation1997) suggests that employers who support their employees in telecommuting play a crucial role in boosting its frequency. As mentioned earlier, many businesses confirmed that they have a positive experience with telecommuting during the pandemic, motivating them to do so after the pandemic (Business Insider Citation2020; CNN Citation2020; Courtney Citation2021). Meanwhile, the findings of this study, in line with the literature (Beck, Hensher, and Wei Citation2020a), show potential shifts in preferences toward telecommuting after the pandemic. Such potential shifts are illustrated for several groups: (1) Generation Y; (2) employees who spent long commute times before the pandemic; (3) High-income; and (4) high-educated employees.
Telecommuting experience significantly affects the persistence of telecommuting after the pandemic. Employees who started telecommuting during the pandemic are not inclined to do so frequently at post-pandemic. This implies that a thorough analysis of employees’ preferences toward their future telecommuting behavior requires accounting for their previous experiences. However, the findings show the positive effects of telecommuting behavior during the pandemic on the expectations toward telecommuting frequency after the pandemic. This implies that employees who telecommute during the pandemic prefer to do so more frequently in the post-pandemic future than they did before the pandemic. Altogether, given that most businesses experienced during the pandemic, a potential shift in preferences toward telecommuting after the pandemic can be expected.
Conclusion
This study explored the long-term impacts of the COVID-19 crisis on telecommuting behavior. Meanwhile, it accounted for (1) changes in COVID-19 risk perception during the pandemic (referred to as ‘risk perception’), and (2) employees’ perceptions toward their productivity at home (referred to as ‘Productive telecommuting’). Moreover, we investigated the influential factors in the persistence of telecommuting after the pandemic.
The results reveal the significance of perceived productivity at home and risk perception in telecommuting continuity during and after the pandemic. According to the results, a dedicated and comfortable workspace at home and the absence of a child, elderly, or dependent care can help telecommuters better focus on their work. The results also indicate the availability of required equipment and technology at home as a productivity driver allowing some work tasks to be completed at home. One of the determinants of productive telecommuting is feeling depressed or sad, particularly at this time, during a pandemic, when health, economic, and social crises have virtually affected everyone’s life. Depression can cause one to lose motivation to work, resulting in lower productivity at home. In addition, employees consider the absence of daily commuting time as a factor that improves telecommuting productivity, which could help them save their time and energy to work on their job-related tasks. Besides, job category and gender have been identified as factors influencing perceived telecommuting productivity.
The findings introduce the significant effects of risk perception on telecommuting behavior during the pandemic. Employees who perceive a risk of COVID-19 exposure in public places or facilities, such as grocery stores, transit, taxis, and ride-hailing services, telecommute more frequently. Increased perceived risk leads to increased self-isolation, thus, frequent telecommuting. Gender, race, age, and household structure all play a role in determining how employees perceive risk. The results also illustrate that risk perception at wave 1 significantly and positively affects risk perception at wave 2. The same pattern is shown between telecommuting frequency during the pandemic and the post-pandemic future. Furthermore, education, income, previous telecommuting experience, and trip features such as commuting mode and time are shown to impact telecommuting frequency directly.
This study can be expanded in some directions. First, we have considered the work from home class of telecommuting. Other forms of telecommuting can also be considered in future studies to obtain a more comprehensive framework. Second, we have not considered the preferences of essential employees (e.g. healthcare personnel, manufacturing, construction, etc.). Such employees reported in the survey that they could not telecommute due to their job categories. However, the daily progress of technology may provide the telecommuting opportunity for such employees; thus, in future studies, the preferences of these employees should be considered. Third, the source data of this study is limited in a way. Each wave of the survey of the data took 6 months. Within this period, some behaviors might have changed, which we could not capture in our study.
Acknowledgments
This research was supported in part by the National Science Foundation (NSF) RAPID program under grants no. 2030156 and 2029962 and by the Center for Teaching Old Models New Tricks (TOMNET), a University Transportation Center sponsored by the U.S. Department of Transportation through grant no. 69A3551747116, as well as by the Knowledge Exchange for Resilience at Arizona State University. This COVID-19 Working Group effort was also supported by the NSF-funded Social Science Extreme Events Research (SSEER) network and the CONVERGE facility at the Natural Hazards Center at the University of Colorado Boulder (NSF Award #1841338) and the NSF CAREER award under grant no. 155173. Any opinions, findings, and conclusions, or recommendations expressed in this material are those of the authors and do not necessarily reflect the views of the funders.
Disclosure statement
No potential conflict of interest was reported by the author(s).
Additional information
Funding
References
- Abdelrahman, M. 2020. “Personality Traits, Risk Perception, and Protective Behaviors of Arab Residents of Qatar during the COVID-19 Pandemic.” International Journal of Mental Health and Addiction 1–12. doi:10.1007/S11469-020-00352-7.
- Acock, A. C. 2013. Discovering Structural Equation Modeling Using Stata. Stata Press books.
- Ahrendt, D., J. Cabrita, E. Clerici, J. Hurley, T. Leoncikas, M. Mascherini, S. Riso, and E. Sandor. 2020. “Living, Working and COVID-19.” Living, working and COVID-19 , COVID-19 series, Publications Office of the European Union, Luxembourg. pp. 80. ISBN: 978-92-897-2117-2
- Allam, Z., and P. Newman. 2018. “Redefining the Smart City: Culture, Metabolism and Governance.” Smart Cities 1: 4–25. doi:10.3390/SMARTCITIES1010002.
- Asgari, H., X. Jin, and A. Mohseni. 2014. “Choice, Frequency, and Engagement.” Transportation Research Record Transportation Research Board 2413: 101–109. doi:10.3141/2413-11.
- Baert, S., L. Lippens, E. Moens, P. Sterkens, and J. Weytjens, 2020. The COVID-19 Crisis and Telework: A Research Survey on Experiences, Expectations and Hopes.
- Bagley, M. N., and P. L. Mokhtarian. 1997. “Analyzing the Preference for non-exclusive Forms of Telecommuting: Modeling and Policy Implications.” Transportation (Amst) 24: 203–226. doi:10.1023/A:1004924601411.
- Bailyn, L. 1989. “Toward the Perfect Workplace? Commun.” Communications of the ACM 32: 460–471. doi:10.1145/63334.63338.
- Balbontin, C., D. A. Hensher, M. J. Beck, R. Giesen, P. Basnak, J. A. Vallejo-Borda, and C. Venter. 2021. “Impact of COVID-19 on the Number of Days Working from Home and Commuting Travel: A cross-cultural Comparison between Australia, South America and South Africa.” Journal of Transport Geography 96: 103188. doi:10.1016/J.JTRANGEO.2021.103188.
- Baruch, Y. 2001. “The Status of Research on Teleworking and an Agenda for Future Research.” International Journal of Management Reviews 3: 113–129. doi:10.1111/1468-2370.00058.
- Beck, M. J., and D. A. Hensher. 2020a. “Insights into the Impact of COVID-19 on Household Travel and Activities in Australia – The Early Days of Easing Restrictions.” Transport Policy 99: 95–119. doi:10.1016/j.tranpol.2020.08.004.
- Beck, M. J., D. A. Hensher, and E. Wei. 2020a. “Slowly Coming Out of COVID-19 Restrictions in Australia: Implications for Working from Home and Commuting Trips by Car and Public Transport.” Journal of Transport Geography 88: 102846. doi:10.1016/j.jtrangeo.2020.102846.
- Beck, M. J., and D. A. Hensher. 2020b. “Insights into the Impact of COVID-19 on Household Travel and Activities in Australia – The Early Days of Easing Restrictions.” Transport Policy 99: 95–119. doi:10.1016/J.TRANPOL.2020.08.004.
- Beck, M. J., D. A. Hensher, and E. Wei. 2020b. “Slowly Coming Out of COVID-19 Restrictions in Australia: Implications for Working from Home and Commuting Trips by Car and Public Transport.” Journal of Transport Geography 88: 102846. doi:10.1016/J.JTRANGEO.2020.102846.
- Beck, M. J., and D. A. Hensher, 2021. INSTITUTE of TRANSPORT and LOGISTICS STUDIES Insights into Working from Home in Australia in 2020: Positives, Negatives and the Potential for Future Benefits to TRANSPORT and Society.
- Bernardino, A., M. Ben-Akiva, and I. Salomon. 1993. “Stated Preference Approach to Modeling the Adoption of Telecommuting.” Transportation Research Record 1413. Transportation Research Board. ISSN: 0361-1981.
- Bernardino, A., and M. Ben-Akiva. 1996. “Modeling the Process of Adoption of Telecommuting: Comprehensive Framework.” Transportation Research Record: Journal of the Transportation Research Board 1552: 161–170. doi:10.1177/0361198196155200122.
- Bollen, K. A., and M. D. Noble, 2011. Structural Equation Models and the Quantification of Behavior. Proceedings of the National Academy of Sciences 108, no. Supplement 3 (2011): 15639-15646. doi: 10.1073/PNAS.1010661108
- Brooking Institution, 2020. Telecommuting Will Likely Continue Long after the Pandemic [WWW Document]. https://www.brookings.edu/blog/up-front/2020/04/06/telecommuting-will-likely-continue-long-after-the-pandemic/ ( accessed 4.28.21).
- Business Insider, 2020. Twitter Tells Employees They Can Work from Home Permanently [WWW Document].
- Chauhan, R. S., D. Capasso da Silva, D. Salon, A. Shamshiripour, E. Rahimi, U. Sutradhar, S. Khoeini, A. (Kouros) Mohammadian, S. Derrible, and R. Pendyala. 2021. “COVID-19 Related Attitudes and Risk Perceptions across Urban, Rural, and Suburban Areas in the United States.” Findings 1–7. doi:10.32866/001c.23714.
- CNN, 2020. These Companies Plan to Make Working from Home the New Normal. As in Forever [WWW Document].
- Collins, J. H., and J. J. Moschler. 2009. “The Benefits and Limitations of Telecommuting.” Definition Acquis Review Journal 16(1). Defense Acquisition University Press.
- Conway, M. W., D. Salon, D. C. D. Silva, and L. Mirtich. 2020. “How Will the COVID-19 Pandemic Affect the Future of Urban Life? Early Evidence from Highly-Educated Respondents in the United States.” Urban Science 4 (4): 50. doi:10.3390/URBANSCI4040050.
- Courtney, E., 2021. 23 Companies Switching to Long-Term Remote Work | FlexJobs [WWW Document]. https://www.flexjobs.com/blog/post/companies-switching-remote-work-long-term/ (accessed 4.21.21).
- Cummins, E., 2020. Why You Can’t Stay Focused while Working from Home - Vox [WWW Document]. Vox News. https://www.vox.com/the-highlight/21317485/work-from-home-coronavirus-covid-19-zoom-distraction-animal-crossing (accessed 4.4.22).
- D’Angelo, M., 2020. How to Create an Effective Telecommuting Policy - Businessnewsdaily.com [WWW Document]. https://www.businessnewsdaily.com/7749-create-telecommuting-policy.html (accessed 8.24.21).
- DeFilippis, E., S. M. Impink, M. Singell, J. T. Polzer, and R. Sadun. 2020. “Collaborating during Coronavirus: The Impact of COVID-19 on the Nature of Work.” National Bureau of Economic Research Working Paper Series. 27612. doi:10.3386/W27612.
- Del Boca, D., N. Oggero, P. Profeta, and M. Rossi. 2020. “Women’s and Men’s Work, Housework and Childcare, before and during COVID-19.” Review of Economics of the Household 184 (18): 1001–1017. doi:10.1007/S11150-020-09502-1.
- Drucker, J., and A. J. Khattak. 2000. “Propensity to Work from Home: Modeling Results from the 1995 Nationwide Personal Transportation Survey.” Transportation Research Record: Journal of the Transportation Research Board 1706: 108–117. doi:10.3141/1706-13.
- The Economist, 2020. People are Working Longer Hours during the Pandemic | The Economist [WWW Document]. https://www.economist.com/graphic-detail/2020/11/24/people-are-working-longer-hours-during-the-pandemic (accessed 3.15.22).
- Falco, A., D. Girardi, L. Dal Corso, M. Yıldırım, D. Converso, and D. Unger. 2021. “The Perceived Risk of Being Infected at Work: An Application of the Job demands–resources Model to Workplace Safety during the COVID-19 Outbreak.” PLoS One 16: e0257197. doi:10.1371/JOURNAL.PONE.0257197.
- Harvard Business Review, 2021. Don’t Force People to Come Back to the Office Full Time [WWW Document]. https://hbr.org/2021/08/dont-force-people-to-come-back-to-the-office-full-time (accessed 2.21.22).
- Hensher, D. A., M. J. Beck, and E. Wei. 2021. “Working from Home and Its Implications for Strategic Transport Modelling Based on the Early Days of the COVID-19 Pandemic.” Transportation Research Part A: Policy and Practice 148: 64–78. doi:10.1016/J.TRA.2021.03.027.
- Hjorthol, R. J. 2013. “Teleworking in Some Norwegian Urban Areas—Motives and Transport Effects.” Urban Geography 27: 610–627. doi:10.2747/0272-3638.27.7.610.
- ILO, 2021. Teleworking Arrangements during the COVID-19 Crisis and beyond Paper Prepared for the 2 Nd Employment Working Group Meeting under the 2021 Italian Presidency of the G20 International Labour Organization (ILO).
- Javadinasr, M., T. B. Magassy, E. Rahimi, M. (Yalda) Mohammadi, A. Davatgari, A. (Kouros) Mohammadian, R. S. Chauhan, et al. 2022. “Observed and Expected Impacts of COVID-19 on Travel Behavior in the United States: A Panel Study Analysis.”
- Jöreskog, K. G., and D. Sörbom. 2018. “Recent Developments in Structural Equation Modeling.” Journal of Marketing Research 19(4): 404–416. doi:10.1177/002224378201900402.
- Koenig, B. E., D. K. Henderson, and P. L. Mokhtarian. 1996. “The Travel and Emissions Impacts of Telecommuting for the State of California Telecommuting Pilot Project.” Transportation Research Part C: Emerging Technologies 4: 13–32. doi:10.1016/0968-090X(95)00020-J.
- Kohler, H., A. Bäuerle, A. Schweda, B. Weismüller, M. Fink, V. Musche, A. Robitzsch, et al. 2021. “Increased COVID-19-related Fear and Subjective Risk Perception regarding COVID-19 Affects Behavior in Individuals with Internal high-risk Diseases.” Journal of Primary Care & Community Health 12. doi:10.1177/2150132721996898.
- Mahmassani, H. S., J.-R. Yen, R. Herman, and M. A. Sullivan. 1993. “Employee Attitudes and Stated Preferences toward Telecommuting: An Explanatory Analysis.” Transportation Research Record 1413. ISSN 0361-1981, ISBN 0-309-05560-1.
- Mannering, J. S., and P. L. Mokhtarian. 1995. “Modeling the Choice of Telecommuting Frequency in California: An Exploratory Analysis.” Technological Forecasting and Social Change 49: 49–73. doi:10.1016/0040-1625(95)00005-U.
- Manoochehri, G., and T. Pinkerton. 2003. “Managing Telecommuters : Opportunities and Challenges.” American University Business Law Review 21(1): 9.
- Maria Barrero Nicholas Bloom Steven Davis, J. J., J. Maria Barrero, N. Bloom, and S. J. Davis. 2021. “Why Working from Home Will Stick.” National Bureau of Economic Research Working Paper Series 28731. doi:10.3386/W28731.
- Matson, G., S. McElroy, G. Circella, and Y. Lee. 2021a. UC Davis Policy Briefs Title Telecommuting Rates during the Pandemic Differ by Job Type, Income, and Gender Permalink. https://escholarship.org/uc/item/5f46r97r Publication Date.
- Matson, G., S. McElroy, Y. Lee, and G. Circella, 2021b. UC Davis Research Reports Title Longitudinal Analysis of COVID-19 Impacts on Mobility: An Early Snapshot of the Emerging Changes in Travel Behavior Publication Date.
- Mokhtarian, P. L. 1991. “Telecommuting and Travel: State of the Practice, State of the Art.” Transportation (Amst) 18: 319–342. doi:10.1007/BF00186563.
- Mokhtarian, P. L., S. L. Handy, and I. Salomon. 1995. “Methodological Issues in the Estimation of the Travel, Energy, and Air Quality Impacts of Telecommuting.” Transportation Research, Part A 29: 283–302. doi:10.1016/0965-8564(94)00029-A.
- Mokhtarian, P. L., and K. V. Varma. 1998. “The trade-off between Trips and Distance Traveled in Analyzing the Emissions Impacts of center-based Telecommuting.” Transportation Research Part D: Transport and Environment 3: 419–428. doi:10.1016/S1361-9209(98)00018-2.
- Mokhtarian, P. L., and R. Meenakshisundaram. 2010. “Patterns of Telecommuting Engagement and Frequency: A Cluster Analysis of Telecenter Users.” Prometheus 20(1): 21–37. doi:10.1080/08109020110110907.
- Nguyen, M. H., and J. Armoogum. 2021. “Perception and Preference for Home-Based Telework in the COVID-19 Era: A Gender-Based Analysis in Hanoi.” Vietnam Sustainability 13: 3179. doi:10.3390/su13063179.
- Nguyen, M. H. 2021a. “Factors Influencing home-based Telework in Hanoi (Vietnam) during and after the COVID-19 Era.” Transportation (Amst) 1–32. doi:10.1007/s11116-021-10169-5.
- Nguyen, M. H. 2021b. “Factors Influencing home-based Telework in Hanoi (Vietnam) during and after the COVID-19 Era.” Transp 1–32. doi:10.1007/S11116-021-10169-5.
- Nilles, J., P. Gray, and J. L. Nilles. 1975. Telecommuting - a Possible Transport Substitute. undefined. Archives of Pathology 99 398–400.
- Nilles, J. M. 1988. “Traffic Reduction by Telecommuting: A Status Review and Selected Bibliography.” Transportation Research Part A: General 22: 301–317. doi:10.1016/0191-2607(88)90008-8.
- Niño, M., C. Harris, G. Drawve, and K. M. Fitzpatrick. 2021. “Race and Ethnicity, Gender, and Age on Perceived Threats and Fear of COVID-19: Evidence from Two National Data Sources.” SM - Population Health 13. doi:10.1016/J.SSMPH.2020.100717.
- Parady, G., A. Taniguchi, and K. Takami. 2020. “Travel Behavior Changes during the COVID-19 Pandemic in Japan: Analyzing the Effects of Risk Perception and Social Influence on going-out self-restriction.” Transportation Research Interdisciplinary Perspectives 7: 100181. doi:10.1016/J.TRIP.2020.100181.
- Perić, G., S. Dramićanin, and M. Conić. 2021. “The Impact of Serbian Tourists’ Risk Perception on Their Travel Intentions during the COVID-19 Pandemic.” European Journal of Tourism Research 27: 2705–2705. doi:10.54055/EJTR.V27I.2125.
- Peters, P., K. G. Tijdens, and C. Wetzels. 2004. “Employees’ Opportunities, Preferences, and Practices in Telecommuting Adoption.” Information & Management 41: 469–482. doi:10.1016/S0378-7206(03)00085-5.
- Plaut, P. O. 2006. “The intra-household Choices regarding Commuting and Housing.” Transportation Research Part A: Policy and Practice 40: 561–571. doi:10.1016/j.tra.2005.10.001.
- Pouri, Y. D., and C. R. Bhat. 2003. “On Modeling Choice and Frequency of Home-Based Telecommuting.” Transportation Research Record: Journal of the Transportation Research Board 1858: 55–60. doi:10.3141/1858-08.
- Rana, I. A., S. S. Bhatti, A. B. Aslam, A. Jamshed, J. Ahmad, and A. A. Shah. 2021. “COVID-19 Risk Perception and Coping Mechanisms: Does Gender Make a Difference?” International Journal of Disaster Risk Reduction 55: 102096. doi:10.1016/J.IJDRR.2021.102096.
- Salon, D., M. W. Conway, L. Mirtich, A. Costello, E. Rahimi, A. (Kouros) Mohammadian, R. S. Chauhan, et al. 2021a. “The Effects of the COVID-19 Pandemic on Telecommuting in the United States.”
- Salon, D., M. W. Conway, D. C. D. Silva, R. S. Chauhan, S. Derrible, A. (Kouros) Mohammadian, S. Khoeini, et al., 2021b. The Potential Stickiness of pandemic-induced Behavior Changes in the United States. The Proceedings of the National Academy of Sciences 118: e2106499118. doi: 10.1073/PNAS.2106499118
- Sassen, S. 2001. The Global City: New York, London, Tokyo (Princeton Paperbacks). 2nd ed. ed. Princeton N.J: Princeton University Press.
- Shabanpour, R., N. Golshani, M. Tayarani, J. Auld, and A. (Kouros) Mohammadian. 2018. “Analysis of Telecommuting Behavior and Impacts on Travel Demand and the Environment.” Transportation Research Part D 62: 563–576. doi:10.1016/j.trd.2018.04.003.
- Shamshiripour, A., E. Rahimi, R. Shabanpour, and A. (Kouros) Mohammadian. 2020. “How Is COVID-19 Reshaping activity-travel Behavior? Evidence from a Comprehensive Survey in Chicago.” Transportation Research Interdisciplinary Perspectives 7: 100216. doi:10.1016/j.trip.2020.100216.
- Siegrist, M., C. Keller, and H. A. L. Kiers. 2005. “A New Look at the Psychometric Paradigm of Perception of Hazards.” Risk Analysis 25: 211–222. doi:10.1111/J.0272-4332.2005.00580.X.
- Siegrist, M., L. Luchsinger, and A. Bearth. 2021. “The Impact of Trust and Risk Perception on the Acceptance of Measures to Reduce COVID-19 Cases.” Risk Analysis 41: 787–800. doi:10.1111/RISA.13675.
- Vyas, L., and N. Butakhieo. 2020. “The Impact of Working from Home during COVID-19 on Work and Life Domains: An Exploratory Study on Hong Kong.” Policy design and practice 4(1): 59–76. doi:10.1080/25741292.2020.1863560.
- Washington Post, 2020. The Economy and Life May Be Reshaped by the Coronavirus Pandemic - the Washington Post [WWW Document]. https://www.washingtonpost.com/business/2020/03/21/economy-change-lifestyle-coronavirus/ (accessed 4.28.21).
- WHO, 2021. Weekly Epidemiological Update on COVID-19 - 20 July 2021 [WWW Document]. https://www.who.int/publications/m/item/weekly-epidemiological-update-on-covid-19—20-july-2021 (accessed 7.22.21).
- Williams, R., P. D. Allison, and E. Moral-Benito Banco de España Madrid. 2018. “Linear Dynamic panel-data Estimation Using Maximum Likelihood and Structural Equation Modeling.” The Stata Journal: Promoting Communications on Statistics and Stata 18: 293–326. doi:10.1177/2378023117710578.
- Yap, C. S., and H. Tng. 1990. “Factors Associated with Attitudes Towards Telecommuting.” Information & Management 19: 227–235. doi:10.1016/0378-7206(90)90032-D.
- Yen, J.-R., and H. S. Mahmassani. 1997. “Telecommuting Adoption: Conceptual Framework and Model Estimation.” Transportation Research Record: Journal of the Transportation Research Board 1606: 95–102. doi:10.3141/1606-12.
- Zhu, P., and S. G. Mason. 2014. “The Impact of Telecommuting on Personal Vehicle Usage and Environmental Sustainability.” International Journal of Environmental Science and Technology 11: 2185–2200. doi:10.1007/s13762-014-0556-5.