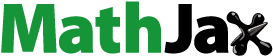
ABSTRACT
Aggressive driving behaviour has been related to the occurrence of risky situations and crashes. The current paper focuses on investigating individual characteristics as determinants of aggressive driving behaviour, with a specific focus on bicycle use. The analysis is based on a questionnaire survey conducted in a number of parking sites in the city centre of Thessaloniki, Greece. Aggressive driving was approximated as a latent variable with reported lane-changing behaviour, use of emergency lane on motorways and maximum speed in urban areas as its indicators. Sociodemographic characteristics and bicycle use were used as explanatory variables. The results showed that increase of aggressiveness had a positive relation to all indicators. Female respondents and older drivers were less likely to report aggressive behaviour. Also, bicycle users were less likely to be aggressive drivers compared to car drivers only. This finding shows that cycling could reduce aggressive behaviour and improve road safety.
Introduction
As mobility in modern societies is still highly dependent on motorized modes of transport, sustainability and driving safety are major issues to be resolved. Despite the decreasing trend of road casualties, the ratio of deceased drivers in road accidents remains considerably high (Commission Citation2015). Accidents can arise as a result of several factors which may involve vehicle attributes, topography of the road network, weather conditions etc. and last but not least driving behavior and human factors (Campbell et al. Citation2012; Janstrup Citation2017). The effect of the latter has been primarily investigated within the realm of the traffic psychology research field. In particular, studies focusing on road safety have confirmed that driving behavior is significantly affected by drivers’ attributes such as sociodemographic characteristics (e.g. gender, age, experience), attitudes, life stress, cognitive workload, fatigue, emotions etc. (Aberg Citation2001; Fountas et al. Citation2019a, Citation2019b; Fylan et al. Citation2006; Lonero and Clinton Citation1998; Wang et al. Citation2014). Relevant literature points out that negative emotions such as anger, come to the surface as a potential consequence of the extensive subjection of drivers to stressful situations and lead to aggressive driving behavior (Dula and Geller Citation2003; Hennessy and Wiesenthal Citation1999; Laagland Citation2005; Qu et al. Citation2016). Driving aggressiveness has been highlighted as a major cause of dangerous behavior (Kaysi and Alam Citation2000; Liu, Chang, and Yu Citation2012) and is mostly expressed within the form of excessive lane changing, tailgating and speeding, verbal and physical aggression, gestures or hostile behavior (Tasca Citation2000). The aforementioned actions are also related to the occurrence of hazardous situations, crashes, near misses, injuries, errors, violations etc. (Dahlen et al. Citation2012), while aggressive driving is also linked to higher emission levels (Hobeika, Jung, and Bae Citation2015; Rakha and Ding Citation2003).
The concept of aggressive driving behavior has been approximated from different perspectives and its definition is surrounded by a certain degree of ambiguity. The main difference among the various approaches concerns the intentionality underneath the observed behavior of a driver in terms of deliberately harming – physically or mentally – the others or not. Following the aforementioned distinction, several definitions are used to describe the occurring forms of aggressive driving behavior. One of the most common categorization groups aggressive driving behavior in road rage and driving aggression (Shinar Citation2017; Tasca Citation2000). The first type refers to hostile driver behavior (even criminal actions) that aim at assaulting or harming the others while the latter is mostly related to risky behavior that derives as a consequence of individual recklessness or irresponsibility (e.g. sensation seeking, time saving etc.). In a testimony before a Congressional subcommittee, Martinez (Citation1997) then director of the National Highway Traffic and Safety Administration (NHTSA) defined aggressive driving behavior as a situation where ‘an individual commits a combination of moving traffic offenses so as to endanger other persons or property’. This is a very inclusive definition referring both to risky driving (i.e., running red lights, weaving in traffic, speeding, tailgating, improper, or unsafe lane-changing) and violence (i.e., intentionally running a vehicle off the road, confronting a driver with a weapon, hand, and facial gestures, screaming, honking). Some similar definitions can also be found in Dula and Geller (Citation2003) where dangerous aggressiveness is classified in actions that intend to harm the others (physical, gestural, or verbal in nature acts), negative emotions such as anger or anxiety that are not necessarily expressed as aberrant actions but through other forms (gesturing, horn-honking etc.) and finally, risky behaviors irrelevant to any intention of assault (e.g. accelerating, weaving, tailgating, traffic light violations). In a parallel line to the aforementioned approach, Richer and Bergeron (Citation2012) distinguished aggressive and risky driving by reporting that the latter is not necessarily a result of anger or hostility. Finally, Neuman et al. (Citation2003)stated that driving aggressiveness can be considered as every type of risky behavior that involves recklessness, potential harmfulness, or accident occurrence, without examining whether it is an outcome of deliberate motivations.
Focusing on the emergence of driving aggression, negative emotions, and more specifically anger, is widely cited in literature as a staple source. The notion of driving anger is well established in the field of psychology, mainly adapting the trait-state anxiety theory (Cattell and Scheier Citation1961; Spielberger Citation2010) and incorporating it within the anger context (Deffenbacher, Stephens, and Sullman Citation2016; Deffenbacher et al. Citation2001). Moreover, there is a considerable body of literature that relates driving anger to aggressive driving such as hostile behavior (Deffenbacher et al. Citation2002), risk-taking (e.g. speeding, tailgating etc.) (Deffenbacher et al. Citation2003), increased number of lapses and errors (Berdoulat, Vavassori, and Sastre Citation2013; Kovácsová, Rošková, and Lajunen Citation2014; Sullman Citation2015) and violations (Lajunen, Parker, and Stradling Citation1998; Underwood et al. Citation1999). In a parallel approach, Zhang and Chan (Citation2016) related driving anger to five types of driving outcomes, namely, aggressive driving, risky driving, driving errors, near misses, and accidents. The aforementioned studies indicate a tightly bound – but not exclusive – relationship between anger and driving aggressiveness. However, driving aggressive or risky behavior is also triggered by other factors such as gender (Popescu Citation2015; Richer and Bergeron Citation2012; Vanlaar et al. Citation2008), age (Krahé and Fenske Citation2002; Lajunen and Parker Citation2001; Przepiorka, Blachnio, and Wiesenthal Citation2014), experience (Simons-Morton et al. Citation2017), personality (Dahlen et al. Citation2012; Ge et al. Citation2014; Krahé and Fenske Citation2002; Lajunen and Parker Citation2001; Przepiorka, Blachnio, and Wiesenthal Citation2014) or stress (Papadakaki et al. Citation2020). Also, Lennon and Watson (Citation2015) reported that the perception about the other drivers, in terms of whether they are intentionally aggressive, is related to the own aggressiveness. Finally, Shinar (Citation1998) mentioned that traffic congestion increases aggressiveness. This outcome was not nevertheless validated in a study conducted by Lajunen, Parker, and Summala (Citation1999) where such relationship did not occur however, other studies have shown impacts of congestion on aggressive driving behavior in post-congestion driving (Huang, Sun, and Zhang Citation2018; Li et al. Citation2020).
It is worth highlighting that within the context of the paper, as aggressive driving behavior was considered the definition of Martinez (Citation1997), – which has been also adopted by the NHTSA (American Automobile Association Citation2009; Royal Citation2004) – related to unsafe driving only, excluding any acts of rage and physical or verbal violence. Under this perspective, the study aims in investigating the relationship among specific types of aggressive behavior and its potential correlation with drivers’ characteristics, with a specific focus on bicycle use. In the existing literature, there is a number of studies that examine the differences in behavior, perceptions, and attitudes between drivers and cyclists/drivers toward cyclists and bicycle as a transport mode (Andronis et al. Citation2018; Johnson et al. Citation2014; Rissel et al. Citation2002; Wood et al. Citation2009) and vice versa (Kazemzadeh et al. Citation2021; Paschalidis et al. Citation2017), perceptions related to sharing the road (Chataway et al. Citation2014; Kaplan and Prato Citation2016; Paschalidis et al. Citation2016) or crash and conflict occurrence between vehicles and bicycles (Chen et al. Citation2018; Prati et al. Citation2020, Citation2018; Prato et al. Citation2016; Sloan et al. Citation2018). However, the effects of cycling on driving behavior, is not thoroughly examined. In a study by Fruhen and Flin (Citation2015), no significant differences between cycling and perceptions respect to risk-taking occurred. Also, Kaplan et al. (Citation2019) found that willingness to share the road as a cyclist or as a driver, correlates with pro-cycling social norms, positive attitudes toward cycling and toward cyclist behavior. In another study, De Angelis et al. (Citation2017)reported that negative attitudes toward cyclists influence the acceptance of an in-vehicle cyclist detection systems. Moreover, Møller and Haustein (Citation2017) suggested that cyclists scored significantly lower ratings in the driving anger expression inventory (DAX-short), however DAX-short mostly focuses on the aspect of anger expression rather than road behavior per se. In a study funded by BikeRight!, The University of Salford, and Transport for Greater Manchester (Sherriff Citation2017) the impact of cycling awareness training on heavy goods vehicles (HGV) drivers was investigated. The training part included a classroom-based theory module and a practical cycling module. The findings of the study suggest that this type of training had a positive impact on driver attitudes and behavior not only toward cyclists but overall. However, the results of the BikeRight! Study cannot be considered as a definite as another study conducted by Gitelman, Korchatov, and Elias (Citation2020) showed that young e-bikers can exhibit risky behavior that can endanger other road users, while also other studies have focused on risky cycling behavior (Federico Fraboni et al. Citation2016; Citation2018; Puchades et al. Citation2018). Most of the aforementioned studies do not clearly show a correlation between aggressive driving behavior and bicycle use, but they provide some indications with respect to a potential relationship between them that justify and could enable for further investigation.
In order to investigate this research objective, a questionnaire survey was developed and administered to drivers in parking locations in the city of Thessaloniki, Greece. The questions were related to sociodemographic characteristics, bicycle use, and specific cases of aggressive driving behavior. The remainder of the paper is organized as follows: The next section presents the undertaken research also including descriptive and inferential statistics analysis. This is followed by the section of model specification and interpretation of parameter estimates. The paper concludes with a discussion section of findings and implications.
Materials and methods
Description of the undertaken research
The objective of the study was to investigate whether car drivers who are also cyclists; active or inactive, are less prone in driving aggressively in comparison to car drivers who have no own cycling experience. For this purpose, a short supervised self-report questionnaire survey was conducted in the city of Thessaloniki in Greece (Konstantinidou Citation2013). Car drivers who completed the questionnaire were interviewed in a number of parking sites in the city center, adjacent to Egnatia Str., Agiou Dimitriou Str., Agias Sofias Str., Olympiados Str., Aristotelous Sqr., and Aristotle University of Thessaloniki campus area. In total, 300 questionnaires were collected in May 2013. In an earlier pilot study, no problems regarding the questionnaire were detected.
No data about the exact population of the car drivers in the city or the number of car drivers who are also cyclists were available. Therefore, no effort to generate a random or stratified sample was undertaken. Still, the city center of Thessaloniki is characterized by diversity in land use; residence, commercial, services, transportation, institutional, and public buildings, and recreational. Thus, it is expected that sampling bias was kept low and no driver category was severely underrepresented.
The questionnaire of the study consisted of seven items. These were driver’s gender, age, (car) driving experience, use, or former use of a bicycle as an alternate means of transportation, maximum driving speed in the city, lane-change behavior, and use of the emergency lane in highways. Consequently, the data were prepared for analysis. Data screening revealed that the data were free of missing values, outliers, and unengaged responses (Tabachnick, Fidell, and Ullman Citation2007). The description of the questionnaire items is shown in together with the descriptive statistics and frequencies of responses.
Table 1. Description and values of the variables.
In the context of the current study, the two survey items related to lane-change and emergency lane use behavior, together with a categorical variable about the maximum speed in urban areas, were considered as indicators of aggressive driving behavior. The use of the aforementioned items as indicators of aggressive driving behavior is based on types of behaviors considered as aggressive by the NHTSA (Royal Citation2004) but it is also consistent with existing literature findings. For instance, excessive lane-changing behavior has been considered as a characteristic of aggressive drivers (Alonso et al. Citation2019; Dula and Ballard Citation2003; Miles and Johnson Citation2003) while the same also holds for the use of the hard shoulder (Dula and Ballard Citation2003; Liu and Lee Citation2005; Shinar and Compton Citation2004). Finally, aggressive drivers are more likely to adopt speeding behavior (Kondyli and Elefteriadou Citation2012; Tasca Citation2000; Vanlaar et al. Citation2008).
The model of aggressive driving behavior
Aggressive driving behavior is not directly measured or observed thus, it was treated as a latent variable approximated via its effects on the three questions-indicators of aggressiveness, reported in Section 2.1, namely, lane-change behavior (I1), use of emergency lane (I2) and maximum speed in urban areas (I3). For convenience, the indicators of aggressive driving behavior will be reported following the aforementioned numbering. The variables derived from the first questionnaire section were used to explain the latent variable. The interpretation of indicator numbering is presented in .
Table 2. Latent variable indicators’ categories.
The model specification can be considered as an adaptation of a Rasch-type latent regression with manifest variables (Christensen et al. Citation2004; Wang and Wu Citation2011; Zwinderman Citation1991); however, the general latent variable approach of the current study stemmed from hybrid choice modeling approaches. As a recommendation, the reader is encouraged to peruse the work of (Abou-Zeid & Ben-Akiva, Citation2014), where the concept of latent variable is explained, while further notable and coherent references that also include case studies (Daly et al. Citation2012; Hess, Shires, and Jopson Citation2013; Mariel, Meyerhoff, and Hess Citation2013) are suggested. To be more concrete, the latent driving aggressiveness is defined as (EquationEquation 1(1)
(1) ):
where αn is the latent variable, Xn is a series of explanatory variables and β is a vector of parameters to be estimated. Finally, ηn is a normally distributed disturbance term of zero mean and variance. In order to address model identification issues, the variance of the disturbance term is usually fixed to unity. To obtain further information regarding driving aggressiveness, the latent variable is then used as an explanatory variable of the three indicators. For the binary indicator (I1), the regular logistic probability was used, that takes the form shown in EquationEquation 2
(2)
(2) :
where, δ is the value of the kth indicator of the nth individual, μk is a sample level constant of the indicator and ζk is the impact of the latent variable αn. The specification presented in EquationEquation 2(2)
(2) applies for binary indicators, however, I2 and I3 had three potential outcomes and thus, the aforementioned approach was not suitable. Usually, multiple-level indicators are of ordinal nature and the respective specification is the one used in Hess, Shires, and Jopson (Citation2013). This was not the case though in the current study, as the potential outcomes were not of a clearly ordinal nature. Moreover, regarding the indicator of maximum speed, the multinomial logit specification allowed for deriving different types of effects for each speed group, which would not be feasible if treating the variable as continuous as this would involve a single parameter only, or ordinal. Hence, the multinomial logit probability presented in EquationEquation 3
(3)
(3) was adopted (Train Citation2009):
To address model identification issues, the μk and ζk parameters for a given indicator value were fixed to zero. This value was considered as the reference case while the interpretation of the other two indicator values was derived with respect to the former, using alternative specific parameters. This approach becomes more definite in Section 3.2, where the parameter estimated are presented.
Model estimation involved the joint likelihood maximization of all indicators conditional on the latent variable. The unconditional log-likelihood function included integration over ηn as shown in EquationEquation 4(4)
(4) :
where, f(.) is the density of the disturbance term in the latent variable. The integral was approximated with simulation using 1000 Halton draws (Halton Citation1960). The model was estimated with the Simulated Maximum Likelihood approach using an adaptation of the Apollo package (Hess and Palma Citation2019), in R software.
Results
Descriptive and inferential statistics
The majority of the sample consisted of male drivers. Moreover, 34% of the sample was younger drivers (18–24 years old) and 46% was 25–54 years old while the remaining 20% was older drivers. Additionally, approximately 61% of the sample had driving experience of at least 6 years or more. With respect to bike use, almost 43% of the sample was current or former bicycle users, for trips in the city while 40% was private car uses exclusively. Participants were also asked regarding their maximum speed in the city. It is worth mentioning that previous research (Islam and Mannering Citation2020) has highlighted that slow drivers may also pose a risk for the surrounding traffic. In order to capture the potential relation of this behavior with the aggressive driving, this variable was further transformed to consider these effects. Given that respondents reported maximum speed at the speed limit and below only, an approximation of the operational V85 was calculated. The value for the 85th speed percentile was 50 km/h, same as the speed limit in urban areas. Any value below 50 km/h was considered as a lower speed category while another category was considered for maximum speed values above the speed limit. Hence, the initial maximum speed variable was recorded as follows: (a) below speed limit (<50 km/h), (b) speed limit (==50 km/h) and (c) above speed limit (>50 km/h). Approximately 61% reported a maximum speed above the speed limit in urban areas. With respect to the first of the two questions related to behavior in highways, 67.3% of the drivers reported lane change only when necessary while almost 33% mentioned constant lane changes. Finally, 67.7% of the sample reported use of emergency lane and 20% sometimes while the remaining 11.3% reported this type of behavior during congestion only.
Given the categorical and ordinal nature of the variables, cross-tabs chi-square and non-parametric tests used for the inferential statistics analysis. With this approach, it was aimed to derive further relationships between drivers’ aggressive behavior indicators and socio-demographic characteristics. The detailed cross-tabs and results of the inferential statistics tests are outlined in . For each of the aggressive driving behavior indicators considered in the analysis, the frequencies of the responses per category of respondents’ socio-demographic characteristics and transport modes used are presented. When these latter characteristics were of categorical nature, the cross-tabs Chi-Square test was used. For ordinal characteristics, it was applied either the Mann-Whitney U-test for binary indicators of aggressive driving behavior or the Kruskal–Wallis test for indicators that included more than two categories. The output of the former test provides the Mann-Whitney U and Wilcoxon test values together with a Z score that allows for the calculation of a p-value while the latter the test value together with the significance level.
Table 3. Inferential statistics results.
Results of the inferential statistics analysis showed that the youngest group of drivers reported the highest rate of constant lane-changing when driving on a highway. This is followed by the group of 55–64 years old while none of the drivers above 65 years mentioned constant lane-change behavior. These outcomes consist an indication that younger drivers may be more aggressive than the rest age groups. With respect to bike use, exclusive car drivers reported the highest rate of constant lane-change behavior, followed by car and motorcycle users. On the other hand, bike users reported the lowest constant lane-changing behavior. This finding may consist an indication that bike use can mitigate aggressive driving behavior.
Regarding the emergency lane use, the highest proportion of never using it when driving on a highway was reported by the drivers/cyclist group. On the other hand, the lowest proportion in the same question was observed for exclusive car users. The same drivers reported the highest proportions in ‘Sometimes’ and ‘Only during congestion’ responses. The next highest ratios for the ‘Sometimes’ response were observed for drivers that used all and motorcycle and car users respectively. Similarly to the lane-change item, bike use was linked to less aggressive driving behavior.
Finally, male drivers reported higher rates of driving above the speed limit in urban areas. Additionally, decrease in the age group was linked to higher proportions of violating the speed limit. Also, less experienced drivers reported higher speed limit violation. Although there might be some confounding between driving experience and age, this outcome may still be an indication that more experienced drivers are less prone to speed limit violation. As a final remark, it should be mentioned that bike users reported significantly lower speed limit violations compared to the rest drivers.
Parameter estimates
The parameter estimates of the model specification presented in the previous section are shown in . The Table is divided into two main parts where the parameters of the latent variable and the indicators are distinctly outlined. To assess model fit of the proposed (full) model, a second model (null model) was also estimated. The latter only included the μ and ζ parameters from however, no other explanatory variables were considered. As shown by the likelihood ratio (LR) test, the additional explanatory variables significantly improved model fit. Moreover, the full model had smaller AIC and BIC values compared to the null model.
Table 4. Parameter estimates of the model.
In order to further ensure that each explanatory variable significantly contributed, the model was estimated step-wise (starting from the null model) adding one additional new explanatory variable at a time. After each new estimation, the log-likelihood (LL) score of the new model was compared with the previous version using the Likelihood-ratio (LR) test. In brief, the test can be defined as:
where LLR is the LL value of the restricted model (the one with fewer variables) and LLU is the LL of the unrestricted model (the model that includes the extra variables). The resulting LR statistic is asymptotically χ2-distributed and is compared with a critical value which depends on the degrees of freedom (difference in estimated parameters). If the LR statistic exceeds that threshold value then the null hypothesis that both models perform equally is rejected. The results of the various likelihood ratio tests are presented in . In all cases, the null hypothesis is rejected at 95% level which implies that the models with additional variables have a significantly improved LL score.
Table 5. Likelihood ratio tests’ results.
Regarding the latent variable specification, a negative association occurred with respect to the gender dummy variable, showing that female drivers are less likely to exhibit aggressive driving behavior. With respect to the effect of age, results showed that all age groups were less likely to be related with aggressive driving behavior compared to the youngest drivers. Regarding the use of bicycle, all dummy variables (including motorcycle use) had a negative impact on aggressive behavior, compared to the reference group of exclusive private car use. This finding indicates that experience of using a transport mode that classifies a user as vulnerable compared to cars, may affect toward the adoption of less aggressive driving behavior. The strongest negative impact occurred for car and bicycle users followed by those using all the available examined modes. This outcome indicates that bike use is related to less aggressive reported behavior compared, to the use of motorized modes (car solely or car and motorcycle). Moreover, users of car/bicycle were less likely to report aggressive driving behavior compared to users of car/motorcycle/bicycle. This finding may suggest that cyclists without experience of using any other motorized vehicle than car are also less aggressive drivers. Overall, bike use was related to less reported aggressive driving behavior while the most aggressive behavior was reported by car drivers only which indicates potential positive effects of cycling on the reduction of aggressive driving behavior. Driving experience was tested as an explanatory variable but it did not have a significant effect.
The parameter estimates related to the indicators showed that the latent variable had a positive and statistically significant effect on all indicators. This outcome is consistent with the initial hypothesis that an aggressive driver is more likely to be associated with increased lane-changing, use of emergency lane and speed limit violation in urban areas. It is worth mentioning that, with respect to the use of emergency lane, the parameters were compared to the case of ‘never use the emergency lane’, and the impact of latent variable is stronger on the I22 value, while a larger value was expected for I21.
As a final step, the significance of the effects of the age and bike use parameters on the latent variable were further investigated, in pairwise comparisons. The values of the parameter estimates presented in refer to the difference with respect to the reference categories 18–24 years and car use only however, the significance among the rest of the parameters was not examined. In order to address this issue, the Delta Method was applied (Daly, Hess, and de Jong Citation2012). Following this approach, the standard errors and thus the t-ratios among the differences of all parameter estimates of interest can be calculated as a function of the parameter values and the covariance matrix of the estimates; however, the detailed illustration of the Delta Method is beyond the scope of the present work. The interested reader may refer to the cited paper of (Daly, Hess, and de Jong Citation2012) for further details. presents the parameter values and t-ratios (in brackets) of the pairwise comparisons regarding the age and bike use parameter estimates. The parameter values were derived as the difference of a parameter as presented in , minus the value of the parameter considered as reference level while the t-ratios were computed via the Delta Method.
Table 6. T-ratios derived from the delta method.
With respect to the age variable, the parameter estimates of the 65+ age group are significantly smaller both compared to the 25–54 and 55–64 age groups while no significant difference occurred in the comparison between the two aforementioned groups. Moreover, a significant difference occurred between the bicycle and car users and the motorcycle and car users’ parameters, indicating that the effect of the former had a significantly more negative effect on the latent variable. On the other hand, no significant difference occurred between the parameter of the latter with the users of all three transport means. Also, the t-ratio calculated for the difference between ‘All’ and ‘Bicycle and car’ users were above 1 but still not statistically significant. However, these findings pointed out a specific pattern with respect to the relation of mode use and driving aggressiveness. To be more concrete, the effect of using any other mode of transport, apart from private car, had a significantly negative effect on driving aggressiveness, as this has been defined in the current study. This had been followed by the motorcycle and car users which is a group of drivers that still do not use bicycle and had significantly different behavior from bicycle and car users but not from drivers with stated use of all means of transport. Moreover, the stated behavior of the two bicycle-related groups did not significantly differ either. These findings indicated that the use of other modes that bring drivers in more vulnerable positions may moderate their aggressiveness when driving and this effect is culminating for those who apart from car only use bicycle.
Marginal effects
This section illustrates the effects of the latent variable on the probability of obtaining each indicator. The purpose of this analysis was to provide some more detailed insights regarding the model interpretation. The probability values were calculated using the parameter estimates presented in . To that end, two different approaches were considered. In the first approach, marginal effects were calculated for each individual per independent variable and indicator category. The average values are presented in . It should be mentioned that since the actual effect on an indicator is the product of the parameter estimate of an explanatory variable, times the respective ζ parameter (), the marginal effects represent the combined effect of the two parameters simultaneously. This first approach considered sample average values for all the explanatory variables. For each individual, marginal effects were calculated as:
Table 7. Average marginal effects of independent variables.
where pj is the probability of a specific outcome for a given indicator of aggressive driving behavior and xk represents the value of a specific explanatory variable of interest. Finally, pm is used to represent the probability values for all outcomes of a specific indicator for a given individual.
In the second approach, the analysis focused on the effects of one variable at a time assuming all others being equal. To that end, for each of the explanatory variables of the latent variable, a sample average value was used, apart from the examined variable each time. Given that all explanatory variables were of categorical nature, the probability to obtain a specific indicator value was calculated for each level of the variables. For each indicator, the calculated probabilities refer to the values compared to the reference category, without presenting the latter. As expected, the probability values of the reference categories can be calculated if the values presented in the aforementioned Figures are subtracted from unity (The sum of all probabilities is one). It should be mentioned that only the significant variables have been included in the analysis.
The results presented in show that with respect to lane-change behavior, the age dummy for drivers above 65+ resulted in the highest increase in the probability of changing lane ‘when necessary’, compared to the ‘Constantly’ option. The next highest impact with respect to the ‘when necessary’ lane-changing behavior, occurred from the dummy variables related to bicycle use. This finding indicated that bike use had a stronger impact compared to age, gender, and the driver/motorcyclist category and supports the parameter interpretation presented in Section 3.2. Also, being a female driver resulted in an increase of the probability of this indicator as well. As expected, since lane-change was a binary indicator, the average marginal effects related to the ‘Constantly’ category had the exact opposite values to the ‘when necessary’ option. Similar trends were also observed regarding the emergency lane use indicator. The eldest group had the highest impact in the probability of the ‘Never’ option, followed by the two variables related to bike use. These variables were also related to the highest decreases in the probabilities of the ‘Sometimes’ and ‘Only during congestion options’. Gender also had a strong impact compared to most of the age-related variables and the driver/motorcyclist category. Finally, same findings also hold for the maximum speed indicator. With respect to the effects on the probability of the ‘Above the speed limit’ category, the strongest impact resulted from the eldest drivers dummy and after that the bike-use related variables. Overall, being a driver above 65 years favored the most less aggressive behavior followed by bike use. Moreover, being only cyclist and driver had a higher increase to the probabilities of options related to less aggressive driving behavior, compared to be a cyclist, motorcyclist, and driver at the same time. This outcome highlights the potential beneficial effects of having a cycling experience toward adoption less aggressive driving behavior. Being a female driver also had a stronger impact toward less aggressive driving compared to most of the age-related variables and being a driver/motorcyclist. The next paragraphs present the results of the second approach of the marginal effects analysis.
Lane-change behavior indicator
The marginal effects plots of the lane-change behavior indicator are presented in . As shown in , the probability of a male driver to provide a ‘Constantly’ response was higher compared to a female. Regarding age (), a decreasing trend to observe a ‘Constantly’ response occurred, as the age group increased. The difference was stronger for the 65+ which is an expected outcome given that, as shown in , the effect of the latter age group on the latent variable was significantly more negative compared to all other age groups. Finally, as shown in , the probabilities of bike use-related categories were smaller compared to the ones of non-bike users, indicating that bike users are less likely to be linked to excessive lane-change behavior. It should be mentioned that the magnitude of the presented probabilities is dependent on the sample average values used and thus would change if different values were used. However, the relative relationship between the categories of the independent variables would remain unchanged regardless which values would have been used as it is affected by the parameter estimates only.
Emergency lane use indicator
The analysis of the emergency lane use indicator is presented in . Given that this indicator consisted of three responses, each figure presents the probabilities of the ‘Sometimes’ and ‘Only during congestion’ responses, as compared to the reference ‘Never’ response. Regarding the effects of gender (), the same trend as in the lane-change indicator occurred, which is expected, given the model specification. The relative difference was higher for the ‘Only during congestion’ response compared to the ‘Sometimes’ as the probability calculated for a female respondent was less than half of a male. Once again, the probability values were always smaller compared to the reference category (which is not presented in ). The trends of the effects of age () and bike use () were similar to those of the lane-change indicator as well. Moreover, the values of the ‘Sometimes’ response were higher compared to the ‘Only during congestion’ in both cases.
Maximum speed indicator
The marginal effects plots related to speed limit violation are depicted in . With respect to gender (), the probability for a male driver to provide a response above the speed limit (>50 km/h) is almost 0.7 ceteris paribus. On the other hand, the probability for a female driver is slightly smaller than 0.5 (<50 km/h). Moreover, the probability of female drivers was higher for reporting maximum speed below the speed limit. Regarding age, there is a decreasing trend for >50 km/h response as the age group is increasing, while the largest difference was observed for the 65+ group. As expected, the opposite trend occurred for the below speed limit category. Finally, all others being equal, the probabilities of bicycle users to provide a > 50 km/h response were close to or smaller than 0.5. On the other hand, the same probability was above 0.6 for car and motorcycle users while it was almost 0.8 for car only users. The trends for responses below the speed limit were opposite however, with considerably smaller probability values.
Discussion
The current paper focused on the investigation of aggressive driving behavior. To that end, a short questionnaire survey was designed and administered to car drivers in a number of parking sites, in the city center of Thessaloniki, Greece. The survey itself was composed of questions related to sociodemographic characteristics, bicycle use and indicators of aggressive driving behavior. Given that the concept of aggressive behavior is not a readily measurable, it was treated as a latent variable that affects participants’ responses to the respective questions of aggressive driving behavior. Sociodemographic characteristics and bicycle use were then used as explanatory variables of the latent variable of aggressive driving behavior.
The parameter estimates of the model indicated that female drivers reported significantly less aggressive behavior, compared to male drivers. Moreover, respondents from the youngest group were significantly more likely to report aggressive behavior, compared to older drivers. These findings were consistent with previous literature comparing the relationship between aggressive driving and gender or age. With respect to transport mode use, car-only users were the most likely to report aggressive driving behavior, compared to the rest road users. Finally, it is worth mentioning that participants using bikes were less likely to report aggressive driving behavior. Results of marginal effects analysis showed that after the eldest drivers, bike use had the highest impact toward less aggressive driving behavior. This outcome suggests that cycling experience may be significantly related to safer driving behavior.
The findings of the present work require further investigation, as they are based on a simple and short survey which limited the possibility of deriving a wide range of findings. For instance, aggressive behavior might be expressed via some additional indicators to those used in the current study such as speed limit violation in the past (Beck, Wang, and Mitchell Citation2006; Vanlaar et al. Citation2008), rather than maximum speed in urban areas as examined in the current work. Moreover, other individual traits and characteristics might influence aggressive driving behavior, while the former could also be related to bike use. This complex set of relationships could be investigated via more comprehensive survey structures or different data collection approaches as interviews or focus groups. Also, it should be mentioned that the findings only show a correlation between bicycle use and decreased aggressive behavior, rather than a causal direction; this is a main limitation of the cross-sectional type of survey used. To the limitations of the study, it should be also added the potential presence of strategic bias or social desirability effects which can be sources of erroneous findings in all studies based on questionnaire surveys. To that, it should be added that in order to have representative results, this and similar questionnaire surveys should be administered in different cities in order to improve representativeness of the sample, as the indicators used to describe aggressive driving behavior may vary for individuals across different areas. In the current study, the results are based on a common understanding of these indicators by the respondents, based on analytical explanations provided during the data collection process by a trained student. The latter can be also extended in the explicit consideration of cyclist respondents to adequately capture the preferences of this group rather than the non-systematic approach that was followed in the current study. Also, in the current work, there was no distinction between active or inactive cyclists. Cycling frequency could potentially provide further insights with respect to the relation of bike use and aggressive driving. In terms of model specification, findings from previous studies (Islam, Alnawmasi, and Mannering Citation2020; Islam and Mannering Citation2020; Islam and Pande Citation2020; Mannering Citation2018; Mannering and Bhat Citation2014) have highlighted that the use of random parameters can improve model fit. Moreover, when the mean parameter can have both positive and negative values, the introduction of random heterogeneity in the effect of an explanatory variable can assist in capturing significant variance around an insignificant mean close to zero (Behnood and Mannering Citation2017). Omission of random heterogeneity in that case, would lead to a false assumption that this variable has no significant effect. Given the cross-sectional nature of the data in the current study, which may lead to less accurate recovery of heterogeneity (Hess and Train Citation2011) and also the significance levels of the results, the approach of random parameters was not followed.
The potential mitigating effect of bicycle use on aggressive driving could be exploited to reduce this type of behavior on the road. It is likely that drivers who are also cyclists express less aggressive behavior, as they have experienced the road environment from a vulnerable user perspective. For this reason, these drivers may adopt a more cautious and conservative driving style. This assumption is consistent with the results presented by Sherriff (Citation2017), with respect to the effects of cycling awareness training for HGV professional drivers. Based on this example and the findings of the present paper, it is likely that driver training incorporating elements of cycling could benefit and improve driving culture, leading to safer and more sustainable driving behavior that will benefit transportation systems with higher bicycle use and greener driving styles.
Author Contributions
Conceptualization, S.B., A.O.; Supervision, S.B., I.P., and E.P.; formal analysis, M.N.K., and E.P.; writing-original draft, E.P.; writing-review and editing, S.B., I.P., E.P., A.O., and M.N.K.
All authors have read and agreed to the published version of the manuscript.
Disclosure statement
No potential conflict of interest was reported by the author(s).
Data availability statement
Some or all data, models, or code that support the findings of this study are available from the corresponding author ([email protected]) upon reasonable request.
Additional information
Funding
References
- Aberg, L. 2001. “Attitudes.” In Traffic Psychology Today, edited by P. Barjonet, Boston, MA: Kluwer Academic Publishers.
- Abou-Zeid, M., and M. Ben-Akiva. 2014. “Hybrid Choice Models.” In Handbook of Choice Modelling, edited by Hess Stephane, and Daly Andrew, 383–412. Cheltenham, UK: Edward Elgar Publishing.
- Alonso, F., C. Esteban, L. Montoro, and A. Serge. 2019. “Conceptualization of Aggressive Driving Behaviors through a Perception of Aggressive Driving Scale (PAD).” Transportation Research. Part F, Traffic Psychology and Behaviour 60: 415–426. doi:10.1016/j.trf.2018.10.032.
- American Automobile Association. 2009. Aggressive Driving: Research Updating. Washington, DC: American Automobile Association Foundation for Traffic Safety.
- Andronis, K., N. Mavridis, A. Oikonomou, and S. Basbas2018. “An Analysis on Drivers’ Self-reported Questionnaire Responses, regarding Aggressive Driving, Attitude toward Cyclists and Personal Values.” Paper presented at the The 4th Conference on Sustainable Urban Mobility, Skiathos Island, Greece.
- Beck, K. H., M. Q. Wang, and M. M. Mitchell. 2006. “Concerns, Dispositions and Behaviors of Aggressive Drivers: What Do self-identified Aggressive Drivers Believe about Traffic Safety?” Journal of Safety Research 37 (2): 159–165. doi:10.1016/j.jsr.2006.01.002.
- Behnood, A., and F. Mannering. 2017. “The Effect of Passengers on driver-injury Severities in single-vehicle Crashes: A Random Parameters heterogeneity-in-means Approach.” Analytic Methods in Accident Research 14: 41–53. doi:10.1016/j.amar.2017.04.001.
- Berdoulat, E., D. Vavassori, and M. T. M. Sastre. 2013. “Driving Anger, Emotional and Instrumental Aggressiveness, and Impulsiveness in the Prediction of Aggressive and Transgressive Driving.” Accident Analysis & Prevention 50: 758–767. doi:10.1016/j.aap.2012.06.029.
- Campbell, J. L., M. G. Lichty, J. L. Brown, R. M. Richard, J. S. Graving, J. Graham, M. O'Laughlin, D. Torbic, and D. Harwood. 2012. Human Factors Guidelines for Road Systems (NCHRP Report 600) 2nd ed. Washington, DC: Transportation Research Board.
- Cattell, R. B., and I. H. Scheier. 1961. The Meaning and Measurement of Neuroticism and Anxiety. New York: Ronald Press.
- Chataway, E. S., S. Kaplan, T. A. S. Nielsen, and C. G. Prato. 2014. “Safety Perceptions and Reported Behavior Related to Cycling in Mixed Traffic: A Comparison between Brisbane and Copenhagen.” Transportation Research. Part F, Traffic Psychology and Behaviour 23: 32–43. doi:10.1016/j.trf.2013.12.021.
- Chen, J., Z. Li, W. Wang, and H. Jiang. 2018. “Evaluating bicycle–vehicle Conflicts and Delays on Urban Streets with Bike Lane and on-street Parking.” Transportation Letters 10 (1): 1–11. doi:10.1080/19427867.2016.1207365.
- Christensen, K. B., J. B. Bjorner, S. Kreiner, and J. H. Petersen. 2004. “Latent Regression in Loglinear Rasch Models.” Communications in Statistics-Theory and Methods 33 (6): 1295–1313. doi:10.1081/STA-120030150.
- Commission, E. 2015. “Road Safety in the European Union: Trends, Statistics and Main Challenges.” Technical Report.
- Dahlen, E. R., B. D. Edwards, T. Tubré, M. J. Zyphur, and C. R. Warren. 2012. “Taking a Look behind the Wheel: An Investigation into the Personality Predictors of Aggressive Driving.” Accident Analysis & Prevention 45: 1–9. doi:10.1016/j.aap.2011.11.012.
- Daly, A., S. Hess, and G. de Jong. 2012. “Calculating Errors for Measures Derived from Choice Modelling Estimates.” Transportation Research Part B: Methodological 46 (2): 333–341. doi:10.1016/j.trb.2011.10.008.
- Daly, A., S. Hess, B. Patruni, D. Potoglou, and C. Rohr. 2012. “Using Ordered Attitudinal Indicators in a Latent Variable Choice Model: A Study of the Impact of Security on Rail Travel Behaviour.” Transportation 39 (2): 267–297. doi:10.1007/s11116-011-9351-z.
- De Angelis, M., V. M. Puchades, F. Fraboni, L. Pietrantoni, and G. Prati. 2017. “Negative Attitudes Towards Cyclists Influence the Acceptance of an in-vehicle Cyclist Detection System.” Transportation Research. Part F, Traffic Psychology and Behaviour 49: 244–256. doi:10.1016/j.trf.2017.06.021.
- Deffenbacher, J. L., D. M. Deffenbacher, R. S. Lynch, and T. L. Richards. 2003. “Anger, Aggression, and Risky Behavior: A Comparison of High and Low Anger Drivers.” Behaviour Research and Therapy 41 (6): 701–718. doi:10.1016/S0005-7967(02)00046-3.
- Deffenbacher, J. L., R. S. Lynch, E. R. Oetting, and R. C. Swaim. 2002. “The Driving Anger Expression Inventory: A Measure of How People Express Their Anger on the Road.” Behaviour Research and Therapy 40 (6): 717–737. doi:10.1016/S0005-7967(01)00063-8.
- Deffenbacher, J. L., R. S. Lynch, E. R. Oetting, and D. A. Yingling. 2001. “Driving Anger: Correlates and a Test of state-trait Theory.” Personality and Individual Differences 31 (8): 1321–1331. doi:10.1016/S0191-8869(00)00226-9.
- Deffenbacher, J., A. Stephens, and M. J. Sullman. 2016. “Driving Anger as a Psychological Construct: Twenty Years of Research Using the Driving Anger Scale.” Transportation Research. Part F, Traffic Psychology and Behaviour 42: 236–247. doi:10.1016/j.trf.2015.10.021.
- Dula, C. S., and M. E. Ballard. 2003. “Development and Evaluation of a Measure of Dangerous, Aggressive, Negative Emotional, and Risky Driving 1.” Journal of Applied Social Psychology 33 (2): 263–282. doi:10.1111/j.1559-1816.2003.tb01896.x.
- Dula, C. S., and E. S. Geller. 2003. “Risky, Aggressive, or Emotional Driving: Addressing the Need for Consistent Communication in Research.” Journal of Safety Research 34 (5): 559–566. doi:10.1016/j.jsr.2003.03.004.
- Fountas, G., S. S. Pantangi, S. S. Ahmed, U. Eker, and P. C. Anastasopoulos. 2019a. “Factors Affecting Perceived and Observed Aggressive Driving Behavior: An Empirical Analysis of Driver Fatigue, and Distracted Driving.” US Department of Transportation. https://rosap.ntl.bts.gov/view/dot/62511
- Fountas, G., S. S. Pantangi, K. F. Hulme, and P. C. Anastasopoulos. 2019b. “The Effects of Driver Fatigue, Gender, and Distracted Driving on Perceived and Observed Aggressive Driving Behavior: A Correlated Grouped Random Parameters Bivariate Probit Approach.” Analytic Methods in Accident Research 22: 100091. doi:10.1016/j.amar.2019.100091.
- Fraboni, F., V. Marín Puchades, M. De Angelis, G. Prati, and L. Pietrantoni. 2016. “Social Influence and Different Types of red-light Behaviors among Cyclists.” Frontiers in Psychology 7: 1834. doi:10.3389/fpsyg.2016.01834.
- Fraboni, F., V. M. Puchades, M. De Angelis, L. Pietrantoni, and G. Prati. 2018. “Red-light Running Behavior of Cyclists in Italy: An Observational Study.” Accident Analysis & Prevention 120: 219–232. doi:10.1016/j.aap.2018.08.013.
- Fruhen, L. S., and R. Flin. 2015. “Car Driver Attitudes, Perceptions of Social Norms and Aggressive Driving Behaviour Towards Cyclists.” Accident Analysis & Prevention 83: 162–170. doi:10.1016/j.aap.2015.07.003.
- Fylan, F., S. Hempel, B. Grunfeld, M. Conner, and R. Lawton. 2006. “Effective Interventions for Speeding Motorists.” Contract Number PPAD 9: 031–133.
- Ge, Y., W. Qu, C. Jiang, F. Du, X. Sun, and K. Zhang. 2014. “The Effect of Stress and Personality on Dangerous Driving Behavior among Chinese Drivers.” Accident Analysis & Prevention 73: 34–40. doi:10.1016/j.aap.2014.07.024.
- Gitelman, V., A. Korchatov, and W. Elias. 2020. “Speeds of Young E-Cyclists on Urban Streets and Related Risk Factors: An Observational Study in Israel.” Safety 6 (2): 29. doi:10.3390/safety6020029.
- Halton, J. H. 1960. “On the Efficiency of Certain quasi-random Sequences of Points in Evaluating multi-dimensional Integrals.” Numerische Mathematik 2 (1): 84–90. doi:10.1007/BF01386213.
- Hennessy, D. A., and D. L. Wiesenthal. 1999. “Traffic Congestion, Driver Stress, and Driver Aggression.” Aggressive Behavior: Official Journal of the International Society for Research on Aggression 25 (6): 409–423. doi:10.1002/(SICI)1098-2337(1999)25:6<409::AID-AB2>3.0.CO;2-0.
- Hess, S., and D. Palma. 2019. “Apollo: A Flexible, Powerful and Customisable Freeware Package for Choice Model Estimation and Application.” Journal of Choice Modelling 32: 100170. doi:10.1016/j.jocm.2019.100170.
- Hess, S., J. Shires, and A. Jopson. 2013. “Accommodating Underlying pro-environmental Attitudes in a Rail Travel Context: Application of a Latent Variable Latent Class Specification.” Transportation Research Part D: Transport and Environment 25: 42–48. doi:10.1016/j.trd.2013.07.003.
- Hess, S., and K. E. Train. 2011. “Recovery of inter-and intra-personal Heterogeneity Using Mixed Logit Models.” Transportation Research Part B: Methodological 45 (7): 973–990. doi:10.1016/j.trb.2011.05.002.
- Hobeika, A. G., H. Jung, and S. Bae. 2015. “Contribution of Aggressive Drivers to Automobile Tailpipe Emissions under Acceleration and Braking Conditions.” Journal of Transportation Engineering 141 (2): 04014077. doi:10.1061/(ASCE)TE.1943-5436.0000736.
- Huang, Y., D. J. Sun, and L.-H. Zhang. 2018. “Effects of Congestion on Drivers’ Speed Choice: Assessing the Mediating Role of State Aggressiveness Based on Taxi Floating Car Data.” Accident Analysis & Prevention 117: 318–327. doi:10.1016/j.aap.2018.04.030.
- Islam, M., N. Alnawmasi, and F. Mannering. 2020. “Unobserved Heterogeneity and Temporal Instability in the Analysis of work-zone crash-injury Severities.” Analytic Methods in Accident Research 28: 100130. doi:10.1016/j.amar.2020.100130.
- Islam, M., and F. Mannering. 2020. “A Temporal Analysis of driver-injury Severities in Crashes Involving Aggressive and non-aggressive Driving.” Analytic Methods in Accident Research 27: 100128. doi:10.1016/j.amar.2020.100128.
- Islam, M., and A. Pande. 2020. “Analysis of single-vehicle Roadway Departure Crashes on Rural Curved Segments Accounting for Unobserved Heterogeneity.” Transportation Research Record 2674 (10): 146–157. doi:10.1177/0361198120935877.
- Janstrup, K. H. 2017. “Road Safety Annual Report 2017.” Paris, France: OECD/ITS.
- Johnson, M., J. Oxley, S. Newstead, and J. Charlton. 2014. “Safety in Numbers? Investigating Australian Driver Behaviour, Knowledge and Attitudes Towards Cyclists.” Accident Analysis & Prevention 70: 148–154. doi:10.1016/j.aap.2014.02.010.
- Kaplan, S., I. Mikolasek, H. Bruhova Foltynova, K. H. Janstrup, and C. G. Prato. 2019. “Attitudes, Norms and Difficulties Underlying Road Sharing Intentions as Drivers and Cyclists: Evidence from the Czech Republic.” International Journal of Sustainable Transportation 13 (5): 350–362. doi:10.1080/15568318.2018.1471556.
- Kaplan, S., and C. G. Prato. 2016. ““Them or Us”: Perceptions, Cognitions, Emotions, and Overt Behavior Associated with Cyclists and Motorists Sharing the Road.” International Journal of Sustainable Transportation 10 (3): 193–200. doi:10.1080/15568318.2014.885621.
- Kaysi, I., and G. Alam. 2000. “Driver Behavior and Traffic Stream Interactions at Unsignalized Intersections.” Journal of Transportation Engineering 126 (6): 498–505. doi:10.1061/(ASCE)0733-947X(2000)126:6(498).
- Kazemzadeh, K., R. Camporeale, C. D’Agostino, A. Laureshyn, and L. Winslott Hiselius. 2021. “Same Questions, Different Answers? A Hierarchical Comparison of Cyclists’ Perceptions of Comfort: In-traffic Vs. Online Approach.” Transportation Letters 13 (7): 531–539.
- Kondyli, A., and L. Elefteriadou. 2012. “Driver Behavior at freeway-ramp Merging Areas Based on Instrumented Vehicle Observations.” Transportation Letters 4 (3): 129–142. doi:10.3328/TL.2012.04.03.129-141.
- Konstantinidou, N. 2013. Investigation of Aggressive Driving Behaviour of Car Drivers with Cycling Experience. Diploma. Thessaloniki, Greece: Aristotle University of Thessaloniki.
- Kovácsová, N., E. Rošková, and T. Lajunen. 2014. “Forgivingness, Anger, and Hostility in Aggressive Driving.” Accident Analysis & Prevention 62: 303–308. doi:10.1016/j.aap.2013.10.017.
- Krahé, B., and I. Fenske. 2002. “Predicting Aggressive Driving Behavior: The Role of Macho Personality, Age, and Power of Car.” Aggressive Behavior: Official Journal of the International Society for Research on Aggression 28 (1): 21–29. doi:10.1002/ab.90003.
- Laagland, J. 2005. “How to Model Aggressive Behavior in Traffic Simulation.” Paper presented at the Twente Student Conference on IT, Enschede.
- Lajunen, T., and D. Parker. 2001. “Are Aggressive People Aggressive Drivers? A Study of the Relationship between self-reported General Aggressiveness, Driver Anger and Aggressive Driving.” Accident Analysis & Prevention 33 (2): 243–255. doi:10.1016/S0001-4575(00)00039-7.
- Lajunen, T., D. Parker, and S. G. Stradling. 1998. “Dimensions of Driver Anger, Aggressive and Highway Code Violations and Their Mediation by Safety Orientation in UK Drivers.” Transportation Research. Part F, Traffic Psychology and Behaviour 1 (2): 107–121. doi:10.1016/S1369-8478(98)00009-6.
- Lajunen, T., D. Parker, and H. Summala. 1999. “Does Traffic Congestion Increase Driver Aggression?” Transportation Research. Part F, Traffic Psychology and Behaviour 2 (4): 225–236. doi:10.1016/S1369-8478(00)00003-6.
- Lennon, A. J., and B. C. Watson. 2015. “A Matter of Style? Driver Attributional ‘Style’in Accounting for the Driving of Others as Protective or as Predisposing Drivers Towards Retaliatory Aggressive Driving.” Transportation Research. Part F, Traffic Psychology and Behaviour 30: 163–172. doi:10.1016/j.trf.2015.03.001.
- Li, G., W. Lai, X. Sui, X. Li, X. Qu, T. Zhang, and Y. Li. 2020. “Influence of Traffic Congestion on Driver Behavior in post-congestion Driving.” Accident Analysis & Prevention 141: 105508. doi:10.1016/j.aap.2020.105508.
- Liu, Y., G.-L. Chang, and J. Yu. 2012. “Empirical Study of Driver Responses during the Yellow Signal Phase at Six Maryland Intersections.” Journal of Transportation Engineering 138 (1): 31–42. doi:10.1061/(ASCE)TE.1943-5436.0000278.
- Liu, B.-S., and Y.-H. Lee. 2005. “Effects of car-phone Use and Aggressive Disposition during Critical Driving Maneuvers.” Transportation Research. Part F, Traffic Psychology and Behaviour 8 (4–5): 369–382. doi:10.1016/j.trf.2005.04.019.
- Lonero, L. P., and K. M. Clinton. 1998. Changing Road User Behavior: What Works, What Doesn’t. Toronto: PDE Publications.
- Mannering, F. 2018. “Temporal Instability and the Analysis of Highway Accident Data.” Analytic Methods in Accident Research 17: 1–13. doi:10.1016/j.amar.2017.10.002.
- Mannering, F. L., and C. R. Bhat. 2014. “Analytic Methods in Accident Research: Methodological Frontier and Future Directions.” Analytic Methods in Accident Research 1: 1–22.
- Mariel, P., J. Meyerhoff, and S. Hess 2013. “Using a Latent Variable Choice Model to Capture Heterogeneous Attitudes and Preferences toward the Landscape Externalities of Wind Power Generation.” Paper presented at the International Choice Modelling Conference, Sydney, AU.
- Martinez, R . 1997. Statement of the honorable Ricardo Martinez, M.D., Administrator National Highway Traffic Safety Administration before the Subcommittee on Surface Transportation Committee on Transportation and Infrastructure US House of Representatives, July, 17, 1997.
- Miles, D. E., and G. L. Johnson. 2003. “Aggressive Driving Behaviors: Are There Psychological and Attitudinal Predictors?” Transportation Research. Part F, Traffic Psychology and Behaviour 6 (2): 147–161. doi:10.1016/S1369-8478(03)00022-6.
- Møller, M., and S. Haustein. 2017. “Anger Expression among Danish Cyclists and Drivers: A Comparison Based on Mode Specific Anger Expression Inventories.” Accident Analysis & Prevention 108: 354–360. doi:10.1016/j.aap.2017.09.016.
- Neuman, T., R. Pfefer, K. Slack, K. Hardy, R. Raub, R. Lucke, and R. Wark. 2003. “Guidance for Implementation of the AASHTO Strategic Highway Safety Plan”. Volume 1: A Guide for Addressing Aggressive-Driving Collisions. Washington, DC: Transportation Research Board.
- Papadakaki, M., N. Stamou, S. Bessas, S. Lioliou, and J. Chliaoutakis. 2020. “Testing the Effectiveness of a mixed-method Pilot Intervention in Reducing Risky Driving Due to Aggression and Stress.” Safety 6 (1): 11. doi:10.3390/safety6010011.
- Paschalidis, E., S. Basbas, I. Politis, and M. Prodromou. 2016. ““Put the Blame on … Others!”: The Battle of Cyclists against Pedestrians and Car Drivers at the Urban Environment. A Cyclists’ Perception Study.” Transportation Research. Part F, Traffic Psychology and Behaviour 41: 243–260. doi:10.1016/j.trf.2015.07.021.
- Paschalidis, E., M. Prodromou, S. Basbas, and I. Politis. 2017. “Investigation of Cyclists’ Attitudes and Perceptions Towards Other Road Users: Evidence from a Case Study in Thessaloniki, Greece.” International Journal of Transportation 5 (2): 33–46. doi:10.14257/ijt.2017.5.2.03.
- Popescu, A. 2015. “Gender Differences in Aggressivity and Perceived Stress at Romanian Amateur Drivers.” International Journal of Traffic and Transportation Psychology 3 (1): 28–41.
- Prati, G., M. De Angelis, F. Fraboni, L. Pietrantoni, D. Johnson, and J. Shires. 2020. “Journey Attributes, E-Bike Use, and Perception of Driving Behavior of Motorists as Predictors of Bicycle Crash Involvement and Severity.” Transportation Research Record 2674 (12): 581–589.
- Prati, G., V. Marín Puchades, M. De Angelis, F. Fraboni, and L. Pietrantoni. 2018. “Factors Contributing to bicycle–motorised Vehicle Collisions: A Systematic Literature Review.” Transport Reviews 38 (2): 184–208. doi:10.1080/01441647.2017.1314391.
- Prato, C. G., S. Kaplan, T. K. Rasmussen, and T. Hels. 2016. “Infrastructure and Spatial Effects on the Frequency of cyclist-motorist Collisions in the Copenhagen Region.” Journal of Transportation Safety & Security 8 (4): 346–360. doi:10.1080/19439962.2015.1055414.
- Przepiorka, A. M., A. Blachnio, and D. L. Wiesenthal. 2014. “The Determinants of Driving Aggression among Polish Drivers.” Transportation Research. Part F, Traffic Psychology and Behaviour 27: 69–80. doi:10.1016/j.trf.2014.09.007.
- Puchades, V. M., L. Pietrantoni, F. Fraboni, M. De Angelis, and G. Prati. 2018. “Unsafe Cycling Behaviours and near Crashes among Italian Cyclists.” International Journal of Injury Control and Safety Promotion 25 (1): 70–77. doi:10.1080/17457300.2017.1341931.
- Qu, W., Q. Zhang, W. Zhao, K. Zhang, and Y. Ge. 2016. “Validation of the Driver Stress Inventory in China: Relationship with Dangerous Driving Behaviors.” Accident Analysis & Prevention 87: 50–58. doi:10.1016/j.aap.2015.11.019.
- Rakha, H., and Y. Ding. 2003. “Impact of Stops on Vehicle Fuel Consumption and Emissions.” Journal of Transportation Engineering 129 (1): 23–32. doi:10.1061/(ASCE)0733-947X(2003)129:1(23).
- Richer, I., and J. Bergeron. 2012. “Differentiating Risky and Aggressive Driving: Further Support of the Internal Validity of the Dula Dangerous Driving Index.” Accident Analysis & Prevention 45: 620–627. doi:10.1016/j.aap.2011.09.014.
- Rissel, C., F. Campbell, B. Ashley, and L. Jackson. 2002. “Driver Road Rule Knowledge and Attitudes Towards Cyclists.” Australian Journal of Primary Health 8 (2): 66–69. doi:10.1071/PY02029.
- Royal, D. 2004. “National Survey of Speeding and Unsafe Driving Attitudes and Behaviors: 2002.” 2, Findings.
- Sherriff, G. 2017. “‘Seeing It from a Cyclist’s Point of View Is Totally Different.’: Understanding the Impact of Cycling Awareness Training for HGV Drivers.”
- Shinar, D. 1998. “Aggressive Driving: The Contribution of the Drivers and the Situation.” Transportation Research. Part F, Traffic Psychology and Behaviour 1 (2): 137–160. doi:10.1016/S1369-8478(99)00002-9.
- Shinar, D. 2017. Traffic Safety and Human Behavior. Bingley, UK: Emerald Group Publishing.
- Shinar, D., and R. Compton. 2004. “Aggressive Driving: An Observational Study of Driver, Vehicle, and Situational Variables.” Accident Analysis & Prevention 36 (3): 429–437. doi:10.1016/S0001-4575(03)00037-X.
- Simons-Morton, B. G., J. P. Ehsani, P. Gershon, S. G. Klauer, and T. A. Dingus. 2017. “Teen Driving Risk and Prevention: Naturalistic Driving Research Contributions and Challenges.” Safety 3 (4): 29. doi:10.3390/safety3040029.
- Sloan, J., S. R. Nargesi, K. Bezboruah, and S. Mattingly. 2018. “Are Bicyclists and Pedestrians Victims or Villains When Struck by a Vehicle?” Journal of Transport & Health 9: S26–S27. doi:10.1016/j.jth.2018.05.084.
- Spielberger, C. 2010. “State-trait Anxiety Inventory.” In The Corsini Encyclopedia of Psychology, edited by Weiner I, and Craighead W, 1698–1699. Hoboken, NJ: John Wiley & Sons. doi:10.1002/9780470479216.
- Sullman, M. J. 2015. “The Expression of Anger on the Road.” Safety Science 72: 153–159. doi:10.1016/j.ssci.2014.08.013.
- Tabachnick, B. G., L. S. Fidell, and J. B. Ullman. 2007. Using Multivariate Statistics. Vol. 5. Boston, MA: Pearson.
- Tasca, L. 2000. A Review of the Literature on Aggressive Driving Research: Ontario Advisory Group on Safe Driving Secretariat. Ontario, Canada: Road User Safety Branch.
- Train, K. E. 2009. Discrete Choice Methods with Simulation. Cambridge: Cambridge university press.
- Underwood, G., P. Chapman, S. Wright, and D. Crundall. 1999. “Anger while Driving.” Transportation Research. Part F, Traffic Psychology and Behaviour 2 (1): 55–68. doi:10.1016/S1369-8478(99)00006-6.
- Vanlaar, W., H. Simpson, D. Mayhew, and R. Robertson. 2008. “Aggressive Driving: A Survey of Attitudes, Opinions and Behaviors.” Journal of Safety Research 39 (4): 375–381. doi:10.1016/j.jsr.2008.05.005.
- Wang, Y., L. Li, L. Feng, and H. Peng. 2014. “Professional Drivers’ Views on Risky Driving Behaviors and Accident Liability: A Questionnaire Survey in Xining, China.” Transportation Letters 6 (3): 126–135. doi:10.1179/1942787514Y.0000000019.
- Wang, W. C., and S. L. Wu. 2011. “The Random‐effect Generalized Rating Scale Model.” Journal of Educational Measurement 48 (4): 441–456. doi:10.1111/j.1745-3984.2011.00154.x.
- Wood, J. M., P. F. Lacherez, R. P. Marszalek, and M. J. King. 2009. “Drivers’ and Cyclists’ Experiences of Sharing the Road: Incidents, Attitudes and Perceptions of Visibility.” Accident Analysis & Prevention 41 (4): 772–776. doi:10.1016/j.aap.2009.03.014.
- Zhang, T., and A. H. Chan. 2016. “The Association between Driving Anger and Driving Outcomes: A meta-analysis of Evidence from the past Twenty Years.” Accident Analysis & Prevention 90: 50–62. doi:10.1016/j.aap.2016.02.009.
- Zwinderman, A. H. 1991. “A Generalized Rasch Model for Manifest Predictors.” Psychometrika 56 (4): 589–600. doi:10.1007/BF02294492.