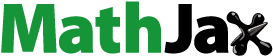
ABSTRACT
Increasing temperatures and sea levels, changing precipitation patterns and more extreme weather pose severe threats for vulnerable communities, ecosystems, and livelihoods in cities of developing countries. Realizing these threats has heightened scholarly inquiry on future risk trends of climate change and adaptation strategies in the countries of Global South and North. However, most studies are based on data of North America, Europe, and Asia. There is minimal documentation of adaptation strategies to mitigate the risk of extreme weather in cities of Sub-Saharan Africa. Hence, this study addresses this need by examining the factors influencing individual and household adaptation strategies to climate risk in Port Harcourt Metropolis, Nigeria. Data was collected from 384 randomly selected household heads in different residential densities of the city. Household socioeconomic and demographic attributes, awareness of climate change and factors influencing their adaptation strategies to climate risk were assessed using descriptive and inferential statistics. The study showed that adaptation strategies adopted were reactive rather than anticipatory and varied in magnitude according to the different residential densities. Recommendations emanating from the study include integrating and implementing climate change adaptation policies and embarking on a rigorous awareness campaign to ease households’ vulnerability and augment their climate change absorptive, adaptive, and transformative abilities in the city.
Introduction
Many cities across the world are facing enormous problems posed by climate change to their communities, populations and critical infrastructure (Pelling and Blackburn Citation2014; Sarker et al. Citation2020). Climate-related extreme events such as flooding, heat waves, storm surges and droughts are imposing heavy burden on societies (Mishra Citation2017; Cian et al. 2020). As a result of high population growth rate and proliferation of slums in Asia and Africa, cities in these continents have been identified as the two most vulnerable areas to the impacts of climate change (UN-HABITAT Citation2016; Lutz and Muttarak Citation2017). The major challenge of these regions and global communities in general centres on the capacity to implement appropriate actions that will mitigate and enhance the capacities of vulnerable communities to adapt to the threats and risks of climate change (Garschagen and Kraas Citation2010; Okunola Citation2019). This is further compounded by a number of epistemological and methodological shortcomings which have significantly affected future risk trends and adaptation needs (Silvast et al. Citation2020).
Despite the uncertainty surrounding the future risk of climate change, the complete picture of future risk trends and adaptation pathways is still unclear to the decision-makers (Berry et al. Citation2017; Clay and King Citation2019). This is attributed to the fact that the scientific method and tools to assess future risk have skewed towards assessing future climate hazards, resulting in an incomplete picture with limited validity and usability for adaptation planning (Birkmann et al. Citation2021; Garschagen et al. Citation2021). Therefore, a sound understanding of future risk trends is required for successful adaptation planning. The realization of this has led to the development and adoption of new adaptation pathways for assessing future risk.
Several scholars such as Li et al. (Citation2018), Okunola (Citation2019) and Huq et al. (Citation2020) have posited that the occurrence frequency and magnitude of future environmental and climate risk” may vary, owning to differences in the socioecological nature of hazards in different parts of the world. Most models (IPCC Citation2001; Hertel et al. Citation2010; Tejedor et al. Citation2021) however indicated that environmental and climate risks will be severe near the equator, hence, in Sub-Saharan Africa than in most other major regions on earth. By implication, Nigeria is highly vulnerable to the impacts of climate change like other countries in Sub-Saharan Africa (Haider Citation2019; Onwutuebe Citation2019). As noted by the World Bank (Citation2021), rural and urban communities in Nigeria are at risk of climate change because of increased temperatures, flooding, increased aridity, and soil erosion, particularly for poor and vulnerable groups. This necessitates the need for stakeholders to take into consideration emerging risks along with existing risks. In order to approach the problem appropriately, it is important to take into consideration local communities’ understanding of climate change, adaptation strategies as well as factors influencing their level of adaptation to climate change risks since they perceive climate as having a strong emotional, spiritual and physical dimension. (Wang et al. Citation2018).
Some notable studies have investigated individuals and households adaptation strategies to climatic events in the Global South and North (Kreibich et al. Citation2005, Citation2011; Botzen et al. Citation2013; Stojanov et al. Citation2016). For instance, Stojanov et al. (Citation2016) stated that the most common household adaptation to climate change in the North-Eastern part of the Czech Republic is the repair of damaged properties instead of implementing costly adaptation measures. The studies of Kreibich et al. (Citation2005) and Kreibich et al. (Citation2011) likewise divided household adaptation strategies into low-cost, medium and high cost adaptation strategies. The low-cost measures include assistance of neighbours affected by climate change events, gathering of information relating to precautionary measures and relocation of properties at risk from ground floor to safer locations (Dey et al. Citation2016; Okunola and Bako Citation2021). Furthermore, Kreibich et al. (Citation2011) stated that securing of flood embankments and barriers, adaptation of the interior (e.g. floor replacement) and flood insurance constitutes medium adaptation strategies to climate change effects. On the other hand, the high cost flood adaptation strategies entailed sealing important parts of the house, constructing small anti-flood walls on the surrounding lands or fortifying the cellar and foundations of the building and using solid and water-resistant materials (Daramola et al. Citation2016). This study also examined residents’ adaptation strategies to climate change effects and factors influencing their choice of strategies.
The studies of Grothmann and Patt (Citation2003) and Ekegren et al. (Citation2020) examined factors influencing household participation in recovering from major trauma. These authors established that perceived ability and self-motivation are major factors influencing individual and households adaptation to major catastrophic events. The authors further stated that self-motivated factor is at most times crucial to household or individual taking precautionary measures in order to cope and adapt to the climate change risks, without necessarily depending on external motivation to protect themselves against climatic risks. Likewise, Ihemezie et al. (Citation2018) stressed that house ownership, house type and income are major factors influencing household and individual adaptation to climate change effects in Leeds, United Kingdom. However, studies of this nature are limited in the West African Sub region and in particular, Port Harcourt, Nigeria.
Array of studies have been carried out on factors influencing household adaptation to climate change in sub-Saharan Africa (Belay et al. Citation2017; Yaméogo et al. Citation2018; Chete Citation2019). For instance, the study of Belay et al. (Citation2017) established that access to climate information and extension, markets and livestock production as well as income, household demography and farm size are major factors influencing farmers choice of adaptation decisions in the Central Rift Valley of Ethiopia. In a similar vein, Chete (Citation2019) in a study conducted in Osun state, Nigeria, identified access to credit and to extension agents, household size, farm income and access to climate change information as factors influencing farmers’ choice of adaptation methods to climate change. However, the focus of these studies was on factors influencing farmers decisions to climate change adaptation in rural areas of Sub-Saharan Africa.
Studies by authors such as Jabeen et al. (Citation2010) and Weru et al. (Citation2017) examined household resilience strategies to climate change effects in informal settlements of sub-Saharan African cities. These studies established that the provision of funds to low-income households to build better housing, barriers to prevent the entrance of floodwater into homes, house designs that keep down high temperatures food stores on top of high furniture or shelves and electrical wiring constitute major household resilience to climate change impacts in the informal settlement of Dhaka and Nairobi. However, these studies did not consider formal settlements of these cities. Therefore, this study intends to complement the literature by investigating how individuals and households adapt to these common extreme climatic events and factors influencing residents’ adaptation to climate change in informal and formal settlements of Port Harcourt, Nigeria.
Data and methods
2.1 Study Area
The study was conducted in Port Harcourt metropolis, a city in Niger Delta region of Nigeria. The metropolis is situated in River State on Longitude 6°55ʹE and Longitude 7°05ʹE and Latitude 4°55ʹN, and Longitude 6°55ʹE. It has an average altitude of 12 m above mean sea level. Port Harcourt metropolis is divided into two local government areas, Port Harcourt and Obio/Akpor ().
Figure 1. Map of River State showing Port Harcourt Metropolis.
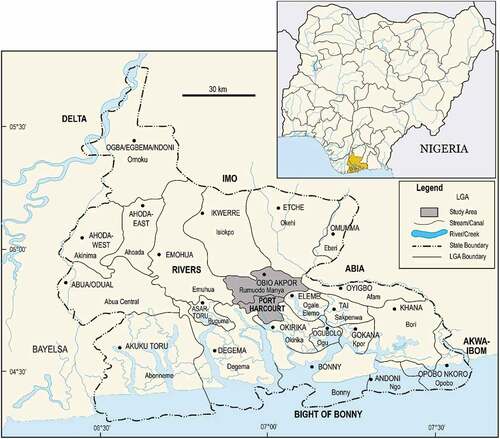
Over the years, Port Harcourt has recorded rapid growth in population and aerial spread like many other Nigerian cities and according to the 2006 census, it had a population of 1,005,904. Presently, the population is estimated to be around over 3 million (Christopher Citation2020). Physically, the city has grown from 15.54 square kilometres in 1914, to a metropolis covering 360 square kilometres in the 1980s, and it has grown to 397 square kilometres in 2021 (Johnson et al. Citation2021).
Climate in Port Harcourt metropolis is similar to that of southern Nigeria’s tropical savanna climate (Köppen climate classification “Aw”). From south to north, the metropolis experiences increased rainfall. Approximately 182 rainy days occur in Port Harcourt every year. The mean minimum temperatures in the metropolis are in the range of 17°C to 24°C while mean maximum monthly temperature ranges between 28°C and 33°C. Furthermore, the metropolis’s mean annual temperature is 26°C. According to Ekwe et al. (Citation2021), February through May are the hottest months in Port Harcourt metropolis. The study further stated that temperatures between dry and wet seasons in the metropolis rarely vary by more than 2°C.
Port Harcourt serves as the major commercial and administrative capital of the South-South geopolitical zone of Nigeria and has a large number of multinational firms as well as other industrial concerns, particularly business related to the petroleum industry. As a result, the city has experienced rapid population growth, political and physical expansion, and complexities in urban problems of various types. A general decline of social services (education and health) and of urban facilities (electricity and water supply, roads) affected Port Harcourt like other Nigerian cities (Amuwo et al. Citation2001) with several physical and social consequences. One of these consequences is occurrence of climate related disasters such as flood, storm among others (Greenwalt et al. Citation2020). According to NEMA (Citation2018), incidence of various disaster in Port Harcourt revealed that occurrence of climate related events are gradually becoming in similarity to that obtainable in Lagos and some other disaster prone cities of the world like Osaka, Beijing and Dhaka among others (UNISDR/UNESCAP Citation2012). it was recorded that in the year 2012, over 12,000 people were affected by the flooding in three districts of the city, while hundreds were rendered homeless (NEMA, Citation2018).
Conclusively, Nigeria towns and cities have been experiencing a lot of events directly or indirectly related to climate change (Ramli et al. Citation2019). Hence, communities in Port Harcourt metropolis with a similar residential pattern and seasonality were investigated to ascertain socioeconomic factors influencing residents’ adaptation to climate change.
2.2 Data Collection
Primary data were collected through administration of questionnaire to household heads in communities of different residential densities in Port Harcourt Metropolis. Three residential densities were purposively selected across the metropolis. These were Olive Crest Estate, Borokiri and King Jaja. The selected communities represent various socioeconomic groups situated in different geographic locations in Port Harcourt. For instance, King Jaja is located in the Southern fringe Port Harcourt Metropolitan area and dominated by low income earners. Borokiri can be categorized as middle-income community and dominated by residential and commercial activities. On the other hand, Olive Crest Estate is located in the eastern part of Port Harcourt city and dominated by high-income earners. A systematic sampling method was used to select respondents for the study based on the number of buildings identified in the three communities selected in the metropolis. A random sampling technique was used to select the first building in each community with subsequent units selected at every tenth building in the three communities. Consequently, a total number of 310, 138 and 112 buildings were selected in King Jaja, Borokiri and Olive Crest Estate respectively. In the case of storey buildings, first apartment on the ground floor were selected for the survey.
A total of 461 copies of questionnaire were distributed to the household heads in the three communities and a total of 384 copies of questionnaire were retrieved. This implied that 83.5% of the questionnaires distributed were retrieved comprising 229, 94 and 61 questionnaires in King Jaja, Borokiri and Olive Crest Estate respectively (). For surveys of this type, a response rate between 30% and 60% is considered adequate (Saunders et al. Citation2012). The first section of the questionnaire was designed to gather information about the respondents’ socioeconomic background. Others include respondents level of awareness to climate change and various methods of climate change adaptation measures in the study area.
Table 1. The questionnaires administered and retrieved in the selected study areas.
2.3 Data analysis
Data obtained was analysed based on the specific objectives of the study. For instance, respondents’ socioeconomic characteristics were analysed by both descriptive and inferential statistics such as frequency, percentages and one-way ANOVA. Information on respondents’ adaptation strategies to climate change effects in the study area was measured using one of the five Likert scales of Very Important (VI), Important (I), Just Important (JI), Not Important (NI) and Not at all Important (NA). The analysis of the responses was used to determine an index termed “Resident Response Index” (RRI). To arrive at RRI, the following steps were followed:
A weighted value of 5,4,3,2 and 1 was respectively attached to each rating of very important, important, just important, not important and not at all important
The summation of weighted value (SWV) for each adaptation strategy was obtained through addition of the product of the number of responses of each strategy and its respective weighted value attached to each rating.
The Mean Weighted Value (MWV) was arrived at by dividing SWV by the number of respondents.
The average level of importance attached to the adaptation measures in the three communities was obtained by the ratio of the sum of the MWV to all adaptation measures and the total number of adaptation measures rated. Thus
Where n = number of the respondents to rating, MD = Mean deviation was used in this research in describing the extent to which values were away from the arithmetic mean and MWV = Mean Weight Value for the study area
Multiple linear regression analysis was employed to determine socio-economic factors influencing level of climate change adaptation in the study area. A number of tests and modifications were carried out to ensure that data satisfy certain assumptions for linear regression. For instance, test for normality was carried out to assess the normality of the distribution of the data as well as other key assumptions to ensure the validity of the regression result. The result of the kolmogorov-Smirnov statistics indicated that 0.189 was not significant at 0.1500 (p-value > 0.05). This implied that the data used for linear regression analysis was normally distributed. The dependent variable for this study was household level of adaptation and this was collapsed to binary variables based on a subjective assessment of the most appropriate dichotomization. That is, Yes or No. Where yes is 1 and no is 0. Responses such as very important, important and just important were collapsed to 1, while not important and not at all important were dummied to 0.
This was subsequently regressed with a set of socio-economic variables such as age of respondents, monthly income, residential density, education level of respondents, household size, religion, gender, house type, house ownership and years lived in home. However, both residential density and religion of respondents were removed because of the multicollinearity problem of the two variables having similar effects with monthly income and household size on the dependent variable.
Hence, the multiple regression analysis is of the form: y = a+ b1x1+ b2x2+ b3x3+ b4x4+ b5x5+ b6x6+ b7x7 (2)
Where y assumed the dependent variable (level of adaptation strategies adopted by households in the study area). The predictors as used here are explained below:
X1 = age of respondents, X2 = average monthly income, X3 = education level of the respondents, X4 = household size, X5 = gender, X6 = house type, X7 house ownership, X8 = years lived in home.
3. Results and Discussion
3.1 Residents’ Socio-economic and Demographic Characteristics
Independent studies have established that there is a correlation between residents’ socio-economic, demographic attributes and response to environmental issues (Arcury and Johnson Citation1987; Seo and Mendelsohn Citation2008). Hence, this section discusses the compilation of the socioeconomic characteristics of the study’s respondents as presented in . Variables such as age, income, level of education, household size and house tenure are considered to determine their influence on residents’ response to climate change in Port Harcourt metropolis. Findings revealed that 69.8% of the residents were female while 30.2% were male. This affirmed the earlier assertion of Carlsson et al. (Citation2012) that women play a critical role in response to climate change due to their local knowledge of sustainable resource management at the household and community level. Also, the age of household head in the three identified residential densities of Port Harcourt Metropolis was grouped into three. These were: 19–30 years (the youths), 31–55 years (the young adults) and 56–65 (the adults) (Okunola Citation2019). It was evident from that majority (70.6%) of respondents in Port Harcourt metropolis were young adults. While youth and adult respondents accounted for 12.8% and 16.7% respectively. The implication of this according to Dahlgren and Whitehead (Citation2007) is that the reasoning level of mature adults with respect to climate change adaptation is expected to be high and thus facilitate their involvement in mitigation and adaption to climate change effects.
Table 2. Socioeconomic and demographic characteristic distribution of the respondents.
In this study, the income group for household heads was based on the income classification adapted from Ezeah (Citation2010) using the minimum wage implemented by the Federal Government of Nigeria as the benchmark (2019). Therefore, household heads who earn less than N30,000 (US$ 1 = 410,25 (Central Bank of Nigeria 2020)) monthly were regarded as low income earners, 30,000–150,000 were referred to as middle income earners, while the high-income earners earn above 150,000. Further findings from the analysis of average monthly income in the metropolis revealed the average monthly income of 38,176.00 K, 94,456.98k and 189,653.06k in the high, medium and low residential densities respectively. It was further shown that 34.5%, 8.5% and 3.5% of respondents in the high, medium and low residential densities respectively were in the low income bucket. Further analysis revealed that variation in income of respondents across the three residential densities was statistically significant (F = 183.484 and p = 0.000) at 95% confidence level. This implied that residents’ financial capacity in responding to climate change will vary in the three residential densities of the metropolis.
Findings on respondents’ level of education revealed that 38.3%, 46.1% and 15.6% of respondents in the high residential area had primary, secondary and tertiary education respectively. In the medium density, it changed to 21.6% for respondents that had primary education, 35.1% for secondary school holders and 43.3% for those that obtained tertiary education certificates. Furthermore, there was significant improvement in respondents’ level of education in the low residential density with 18.4% of primary school holders, 28.9% and 52.7% had secondary and tertiary education respectively. The study therefore established that there was a variation in the respondents’ educational status in the three identified residential densities.
Other identified indicator in relation to residents’ response to climate change is household size. In this regard, the Afon (Citation2007) and Okunola and Bako (Citation2021) household size classification was adopted and categorized into three. Household with 6 members and below, household that contained 7 to 10 members and household with more than 10 members. These were respectively regarded as small-, medium- and large-sized household size. From the summary presented in , low residential density had the largest proportion of respondents with small-sized household. This group accounted for 90.2% of the households within Port Harcourt metropolis. Households in the high and medium residential densities with small-sized household were 33.7% and 78.7% respectively. Also, the analysis of the households that were medium size revealed that the largest proportion was in the high residential density. This group accounted for 33.7% in Port Harcourt metropolis. Also, the difference in household size across the three residential densities was confirmed by result of the One-Way Analysis of variance (F = 4.304; p= 0.014 at 95% confidence level).
3.2 Spatial Variation in Adaptation to Climate Change Effects
In fulfilment of this study’s main purpose, which is to identify and assess factors influencing individual and household adaptation to climate change in the study area, the respondents were asked to identify and rank adaptation strategies adopted on a five-point Likert scale (1 to 5). As presented in , relocation from climate risk areas was the adaptation strategy with the highest rank (MD = 0.64), while complied with setback during property development was ranked least (MD = −0.43). Six of the eighteen adaptation measures were expressed as (positive) important, while twelve were expressed as (negative) not important. Adaptation measures such as repair and replace damaged property, cleaned or helped to maintain public drainage systems from waste, construction of more drainages system by government and community groups, relocation from climate risk areas, avoid haphazard bush burning and listening to information on climate change were adaptation measures with high level importance in the study area. It could be concluded from the results that respondents in the three residential densities relied more on coping measures to climate change effects than anticipatory measures. These findings corroborated the studies of Afon et al. (Citation2016) and Okunola (Citation2019) who asserted that response to climate change-related disasters in major cities of Nigeria is reactive rather than anticipatory. On the other hand, the three adaptation measures expressed to have lowest importance were: emplacement of storm surge barriers by government and community groups, provide efficient mechanisms for disaster risk reduction, use of windbreaks/shelter belts and restriction of land reclamation activities in newly developed areas by government. Furthermore, the average residents’ response index (RRI) for the study area was 3.67, while the RRI of the high, medium and low residential densities were 3.59, 3.69 and 3.72 respectively. These responses indicated that the intensity of responses to climate change effects was on the increase along the residential densities.
Table 3. Intra-urban responses to climate change in Port Harcourt metropolis.
3.3 Socio-economic factors influencing households level of adaptation to climate change
To determine socio-economic factors influencing households’ level of adaptation to climate change in the study area, multiple linear regressions analysis was employed and this presented in . The multiple regression coefficient R2 = 0.549 (see ), significant at 0.001, confirmed that 54.9% of the variance in the socio-economic factors influencing residents’ level of adaptation to climate change in the study area was explained by the model. The result also indicated that education, average monthly income, house type, house ownership and age of respondents were significant at 5% level of significance. This indicated that these variables are major drivers of household adaptation strategies to climate change in the study area. It could be concluded from the foregoing that households level of education, average monthly income, house type, house ownership and age are the major factors influencing residents adaptation to climate change effects in Port Harcourt, Nigeria.
Table 4. Result of multiple regression analysis on socioeconomic factors affecting adaptation strategies to climate change.
3.4 Discussion
The analysis of adaptation strategies adopted by households in the study area established that respondents adopted similar reactive or ex-post adaptations strategies which support them in coping with the effects of climate change in the study area. As a result, they relied on personal efforts more than government interventions in prevention and adaptation of climate change effects. According to Porter et al. (Citation2014), these coping strategies could be classified as coping responses. It is a change in behaviour that does not require a premeditated plan; it occurs spontaneously, sometimes after the impacts of climate change have been experienced. This finding echoes a previous study by Mertz et al. (Citation2009) that found coping response actions against extreme events of climate change are common among households in developing countries.
Furthermore, the study established that households’ choice of adaptation strategies to climate change effects in the study area are greatly influenced by their level of education, average monthly income, house type and house ownership. This indicated that formal education appears to play a greater role in determining people’s level of risk and their ability to adapt. This outcome further reinforces the findings of previous studies such as Wamsler et al. (Citation2012) and Alexander et al. (Citation2016) that education is a major factor that determines household’s or individual’s level of adaptation to climate change effects.
Strong evidence was revealed that age is significant in households’ choice of climate change adaptation strategies in the study area. This analysis of age as a factor for consideration in adaptation pathways highlights the necessity of paying attention to how the predominant age group of young adults in the residential densities of the study area can bring novel adaptation strategies to present and future climate risks. These findings thus substantiate earlier assertions by Ng’ang’a and Crane (Citation2020) that adaptation to climate change is a contingent process highly influenced by age because this factor shapes the positions from which residents negotiate, engage and evaluate different technical adaptation practices. However, family size, gender, number of years lived in in an area were found statistically insignificant factors to climate risk adaptation in the study area.Moreover, the study found that people who own their own homes tend to use more adaptation strategies to climate change than those who rent apartments. This assertion further substantiates the findings of previous studies such as Ihemezie et al. (Citation2018) which concluded that house owners are more likely to adopt more adaptation strategies as a protective measure against their property than tenants who have little stake or self-interest in the property. In line with this, level of household adaptation to climate change may likely be influenced by the type of house they live. It was discovered during interaction with respondents and field observation that households living in duplexes, high-rise buildings adopted more adaptation strategies compared to those that are living in bungalows and terraced houses. This might be attributed to the relatively low income of households living in bungalows and terraced houses as against households living in duplexes and high rise buildings who mostly fall under high income class. This finding further established the importance of income in households choice of adaptation strategies to climate change effects and corroborated the assertion of Uddin et al. (Citation2014) and Ihemezie et al. (Citation2018) who argue that households with high level of income are more likely to cope and adapt to the impacts of climate change.
This study also established that household adaptation strategies to climate risk had a positive and statistically significant effect on households’ income levels. This finding supports the assertion of Berhe et al. (Citation2017) and Ihemezie et al. (Citation2018) that individuals or households with higher monthly income are more likely to adopt more adaptation strategies, especially the more technical and financially demanding adaptation measures. The implication is that inequality in income level of individuals or households means that differences in vulnerability and exposure to climate change risk are likely to continue. In addition, adaptation responses may worsen existing inequalities or even create new ones. Generally, the study’s results confirm Ihemezie et al. (Citation2018) and Ng’ang’a and Crane (Citation2020) findings that socioeconomic status substantially affects respondents’ attitudes and behaviour regarding climate change adaptation.
4. Conclusion
This study provides an analysis to determine socio-economic factors influencing residents’ level of adaptation strategies to climate change effects. Understanding these factors is a step in the right direction towards strengthening households’ resilience to climate change risks. The study established that the strategies by residents in the different residential densities were similar and reactive rather than anticipatory. Relatedly, it was established that households’ choice of adaptation strategies to climate change effects in the study area are greatly influenced by their level of education, average monthly income, house type and house ownership. This demands that government and other relevant stakeholders provide adequate support and easy access to technical and financial resources so that residents can learn advanced adaptation techniques and how to adapt effectively. Therefore, building the capacity of the locals towards reducing climate risks and facilitating effective adaptations are essential. In addition, future policies need to address barriers to the adoption of advanced adaptation techniques at the community level.
More importantly, it is obvious that government support is needed to strengthen socio-economic status of individuals and households in order to evolve anticipatory adaptation to long-term climatic risk such as flood and storms in urban and rural communities across Nigeria. As a result, government and civil society organizations should advocate, empower, and implement policies enabling residents the opportunities to access, learn, and adopt advanced climate change adaptation techniques in the city.
Furthermore, the state and the federal government of Nigeria, development partners, and the private sector actors under the current climate variation should undertake their designated roles in climate change responses, measures and actions to implement context-specific policies to address impediments to the adoption of advanced climate change adaptation strategies across different residential densities in Port Harcourt and others with similar attributes. Furthermore, it is strongly recommended that stakeholders at all levels must assess, plan and implement climate risk reduction measures with the relevant communities to foster collaboration across sectors, addressing the immediate challenge of climate risks and increasing awareness. This would serve as a channel for teaching residents early to be anticipatory in response to climate change effects.
Highlights
Intensity of flood and heat are main indicators of climate change in Port Harcourt
Household adaptation strategies to climate risk are greatly influenced by their socioeconomic attributes
Sound understanding of future risk trends is required for successful adaptation planning
Implementation of context-specific policies to address impediments to the adoption of advanced climate change adaptation strategies
Acknowledgments
We would like to thank Munich Re Foundation through its “World Risk and Adaptation Futures: Social Protection” project including the financial support received in covering the APC charges for the publication of this paper.
Disclosure statement
The authors declare that they have no known competing financial interests or personal relationships that could have appeared to influence the work reported in this paper.
Data availability statement
The data will be provided upon a reasonable request.
References
- Afon AO. 2007. An analysis of solid waste generation in a traditional African city, the example of Ogbomoso, Nigeria. Environ Urban. 19(2):527–537. doi:10.1177/0956247807082834.
- Afon AO, Dada OT, Faniran GB. 2016. Socio-economic attributes of residents as drivers of adaptation to climate change effects in a nigerian traditional urban center. Arch Curr Res Int. 3(3):1–15. doi:10.9734/ACRI/2016/23740.
- Alexander M, Priest S, Mees H. 2016. A framework for evaluating flood risk governance. Environ Sci Policy. 64:38–47. doi:10.1016/j.envsci.2016.06.004.
- Amuwo K, Bach DC, Lebeau Y. 2001. Nigeria During The Abash Years (1993). Ibadan: IFRA.
- Arcury TA, Johnson TP. 1987. Public environmental knowledge: a statewide survey. J Environ Knowledge. 18(4):31–37.
- Belay A, Recha JW, Woldeamanuel T, Morton JF. 2017. Smallholder farmers’ adaptation to climate change and determinants of their adaptation decisions in the Central Rift Valley of Ethiopia. Agri Food Sec. 6(1):1–13. doi:10.1186/s40066-017-0100-1.
- Berhe M, Hoag D, Tesfay G, Tadesse T, Oniki S, Kagatsume M, Keske CM. 2017. The effects of adaptation to climate change on income of households in rural Ethiopia. Pastoralism. 7(1):1–15. doi:10.1186/s13570-017-0084-2.
- Berry P, Sánchez-Arcilla Conejo A, Betts R, Harrison PA (2017). High-end climate change in Europe: impacts, vulnerability and adaptation.
- Birkmann J, Sauter H, Garschagen M, Fleischhauer M, Puntub W, Klose C, Büter B, Göttsche F, Laranjeira K, Müller J. 2021. New methods for local vulnerability scenarios to heat stress to inform urban planning—case study City of Ludwigsburg/Germany. Clim Change. 165(1):1–20. doi:10.1007/s10584-021-03005-3.
- Botzen WJW, Aerts JCJH, van den Bergh JCJ. 2013. Individual preferences for reducing flood risk to near zero through elevation. Mitigat Adapt Strat Global Change. 18(2):229–244. doi:10.1007/s11027-012-9359-5.
- Carlsson F, Kataria M, Krupnick A, Lampi E, Löfgren Å, Qin P, Sterner T, Sterner T. 2012. Paying for mitigation: a multiple country study. Land Econ. 88(2):326–340. doi:10.3368/le.88.2.326.
- Chete OB. 2019. Factors influencing adaptation to climate change among smallholder farming communities in Nigeria. Afr Crop Sci J. 27(1):45–57. doi:10.4314/acsj.v27i1.4.
- Christopher OC. 2020. Cancer incidence in the Niger delta region of Nigeria; a population based review of Port Harcourt cancer registry. Nigerian Health J. 19(2):85–95.
- Clay N, King B. 2019. Smallholders’ uneven capacities to adapt to climate change amid Africa’s ‘green revolution’: case study of Rwanda’s crop intensification program. World Dev. 116:1–14. doi:10.1016/j.worlddev.2018.11.022.
- Dahlgren G, Whitehead M. 2007. Policies and strategies to promote social equity in health. Stockholm: Institute for Policy Studies.
- Daramola AY, Oni OT, Ogundele O, Adesanya A. 2016. Adaptive capacity and coping response strategies to natural disasters: a study in Nigeria. Int J Dis Risk Reduc. 15:132–147. doi:10.1016/j.ijdrr.2016.01.007.
- Dey MM, Gosh K, Valmonte-Santos R, Rosegrant MW, Chen OL. 2016. Economic impact of climate change and climate change adaptation strategies for fisheries sector in Solomon Islands: implication for food security. Marine Pol. 67:171–178.
- Ekegren CL, Braaf S, Ameratunga S, Ponsford J, Nunn A, Cameron P, Gabbe BJ, Gabbe BJ. 2020. Adaptation, self-motivation and support services are key to physical activity participation three to five years after major trauma: a qualitative study. J Physiother. 66(3):188–195. doi:10.1016/j.jphys.2020.06.008.
- Ekwe MC, Adamu F, Gana J, Nwafor GC, Usman R, Nom J, Aderoju OM, Adedeji OI, Halilu SA, Aderoju OM. 2021. The effect of green spaces on the urban thermal environment during a hot-dry season: a case study of Port Harcourt, Nigeria. Environment. Dev Sustain. 23(7):10056–10079. doi:10.1007/s10668-020-01046-9.
- Ezeah C (2010): Analysis of barriers and success factors affecting the adoption of sustainable management of Municipal Solid Waste in Abuja, Nigeria. A thesis submitted in fulfilment of the requirements of the University of Wolverhampton for the award of the degree of Doctorof Philosophy (PhD). Retrieved from: www.wlv.openrepository.com/wlv/bitstream Accessed 28 December 2018.
- Garschagen M, Doshi D, Reith J, Hagenlocher M. 2021. Global patterns of disaster and climate risk—an analysis of the consistency of leading index-based assessments and their results. Clim Change. 169(1):1–19. doi:10.1007/s10584-021-03209-7.
- Garschagen M, Kraas F (2010). Assessing future resilience to natural hazards–the challenge of capturing dynamic changes under conditions of transformation and climate change. In: Custer R, Sutter C, Ammann WJ (Eds.) Proceedings. International Disaster and Risk Conference, IDRC 2010. Davos, 30 May – 03 June 2010, pp. 209–213.
- Greenwalt J, Dede M, Johnson I, Nosa P, Precious A, Summers B (2020). ClimateChange adaptation and community development in Port Harcourt, Nigeria. African Handbook of Climate Change Adaptation, 1–28.
- Grothmann T, Patt A. 2003. Adaptive capacity and human cognition prepared for presentation at the open meeting of the global environmental change research Community, Montreal, Canada. Global Environ Change. 15:16–18.
- Haider H (2019). Climate change in Nigeria: impacts and responses. K4D Helpdesk Report 675. Brighton (UK): Institute of Development Studies.
- Hertel TW, Burke MB, Lobell DB. 2010. The poverty implications of climate- induced crop yield changes by 2030. Global Environ Change. 20(4):577–585. doi:10.1016/j.gloenvcha.2010.07.001.
- Huq ME, Shoeb AZM, Hossain MA, Fahad S, Kamruzzaman MM, Javed A, Sarven MS. 2020. Measuring vulnerability to environmental hazards: qualitative to quantitative. In: Fahad, Shah, Hasanuzzaman, Mirza, Alam, Mukhtar, Ullah, Hidayat, Saeed, Muhammad, Ali Khan, Imtiaz, Adnan, Muhammad editors. Environment, climate, plant and vegetation growth. Cham: Springer; p. 421–452.
- Ihemezie EJ, Onunka CN, Nnaji AP. 2018. Drivers and socioeconomic factors influencing individual and household adaptation to climate change: a case study of residents of Leeds, UK. Dev Agri Econom. 10(9):279–291. doi:10.5897/JDAE2018.0956.
- IPCC. 2001. Annex B: glossary of terms.
- Jabeen H, Johnson C, Allen A. 2010. Built-in resilience: learning from grassroots coping strategies for climate variability. Environ Urban. 22(2):415–431. doi:10.1177/0956247810379937.
- Johnson DV, Gobo AE, Ngerebara OD, Ekaka-a EN. 2021. Assessment and modelling of flash flood risk from extreme rainfall and soil permeability in port. Nigeria: Harcourt Metropolis.
- Kreibich H, Seifert I, Thieken AH, Lindquist E, Wagner W, Merz B. 2011. Recent changes in flood preparedness of private households and business in Germany. Reginol Environ Change. 11(1):59–71. doi:10.1007/s10113-010-0119-3.
- Kreibich H, Thieken AH, Petrow T, Müller M, Merz B. 2005. Flood loss reduction of private households due to building precautionary measures – lessons learned from the Elbe flood in August 2002. Natur Hazard Earth Syst Sci. 5(1):117–126. doi:10.5194/nhess-5-117-2005.
- Li S, Juhász-Horváth L, Pintér L, Rounsevell MD, Harrison PA. 2018. Modelling regional cropping patterns under scenarios of climate and socio-economic change in Hungary. Sci Total Environ. 622:1611–1620. doi:10.1016/j.scitotenv.2017.10.038.
- Lutz W, Muttarak R. 2017. Forecasting societies’ adaptive capacities through a demographic metabolism model. Nat Clim Chang. 7(3):177–184. doi:10.1038/nclimate3222.
- Mertz O, Halsnæs K, Olesen JE, Rasmussen, K. 2009. Adaptation to climate change in developing countries. Environ Manage. 43(5):743–752. doi:10.1007/s00267-008-9259-3.
- Mishra PK. 2017. Socio-economic impacts of climate change in Odisha: issues, challenges and policy options. J Clim Change. 3(1):93–107. doi:10.3233/JCC-170009.
- National Emergency Management Agency (NEMA) (2018) 2018 Annual Report, National Emergency Management Agency, Abuja (Nigeria)
- Okunola OH. 2019. Residents’ vulnerability analysis and explanation of development- induced disasters in a Sub-Saharan African city. Int J Dis Resil Built Environ. 11(1):71–84. doi:10.1108/IJDRBE-05-2019-0024.
- Okunola OH, Bako AI. 2021. Exploring residential characteristics as determinants of household adaptation to climate change in Lagos, Nigeria. Int J Dis Resil Built Environ. doi:10.1108/IJDRBE-06-2021-0060
- Onwutuebe CJ. 2019. Patriarchy and women vulnerability to adverse climate change in Nigeria. Sage Open. 9(1):2158244019825914. doi:10.1177/2158244019825914.
- Pelling M, Blackburn S. editors. 2014. Case studies: governing social and environmental transformation in coastal megacities. In: Megacities and the Coast. London: Routledge; p. 224–260.
- Porter JJ, Dessai S, Tompkins EL. 2014. What do we know about UK household adaptation to climate change? A systematic review. Clim Change. 127(2):371–379. doi:10.1007/s10584-014-1252-7.
- Ramli MF, Aris AZ, Jamil NR, Aderemi AA, Aderemi AA. 2019. Evidence of climate variability from rainfall and temperature fluctuations in semi-arid region of the tropics. Atmos Res. 224:52–64. doi:10.1016/j.atmosres.2019.03.023.
- Sarker MNI, Yang B, Lv Y, Huq ME, Kamruzzaman MM. 2020. Climate change adaptation and resilience through big data. Int J Adv Comput Sci Appl. 11(3):533–539.
- Saunders M, Lewis P, Thornhill A. 2012. Research methods for business students. Pearson. http://scholar.google.com/scholar_lookup?hl=en&publication_year=2012&author=M.+Saunders&author=P.+Lewis&author=A.+Thornhill&title=Research+methods+for+business+studies+students.
- Seo SN, Mendelsohn R. 2008. An analysis of crop choice: adapting to climate change in South American farms. Ecol Econom. 67(1):109–116. doi:10.1016/j.ecolecon.2007.12.007.
- Silvast A, Laes E, Abram S, Bombaerts G. 2020. What do energy modellers know? An ethnography of epistemic values and knowledge models. Energy Res Socl Sci. 66:101495. doi:10.1016/j.erss.2020.101495.
- Stojanov R, Duží B, Kelman I, Němec D, Procházka D. 2016. Household adaptation strategies to climate extremes impacts and population dynamics: case study from the Czech Republic. In: A, Milan, B, Schraven, K, Warner, N, Cascone editors. Migration, risk management and climate change: evidence and policy responses. Cham: Springer; p. 87–103.
- Tejedor E, Steiger NJ, Smerdon JE, Serrano-Notivoli R, Vuille M. 2021. Global hydroclimatic response to tropical volcanic eruptions over the last millennium. Proc Nat Acad Sci. 118(12). doi:10.1073/pnas.2019145118.
- Uddin MN, Bokelmann W, Entsminger JS. 2014. Factors affecting farmers‟ adaptation strategies to environmental degradation and climate change effects: a farm level study in Bangladesh. Climate. 2(4):223–241. doi:10.3390/cli2040223.
- UN-HABITAT (2016). The New Urban Agenda. Google Scholar. http://habitat3.org/wp-content/uploads/NUA-English.pdf (Accessed 30 July 2021).
- UNISDR/UNESCAP. 2012. Asia-Pacific disaster report 2012 – reducing vulnerability and exposure to disasters. Bangkok:UNESCAP.
- Wairimu Ng’Ang’a T, Crane TA. 2020. Social differentiation in climate change adaptation: One community, multiple pathways in transitioning Kenyan pastoralism. Environmental Science & Policy. 114:478–485. doi:10.1016/j.envsci.2020.08.010.
- Wamsler C, Brink E, Rentala O. 2012. Climate change, adaptation, and formal education: the role of schooling for increasing societies’ adaptive capacities in El Salvador and Brazil. Ecol Soci. 17(2). doi:10.5751/ES-04645-170202.
- Wang S, Leviston Z, Hurlstone M, Lawrence C, Walker I. 2018. Emotions predict policy support: why it matters how people feel about climate change. Global Environ Change. 50:25–40. doi:10.1016/j.gloenvcha.2018.03.002.
- Weru J, Njoroge P, Wambui M, Wanjiku R, Mwelu J, Chepchumba A, Wakesho T. 2017. The Akiba Mashinani Trust, Kenya: role of a local fund in urban development. New York City: International Institute for Environment and Development.
- World Bank (2021). Climate Risk Profile: the World Bank Group. [4 Oct 2021]. https://climateknowledgeportal.worldbank.org/sites/default/files/2021-07/15918-WB_Nigeria%20Country%20Profile-WEB.pdf
- Yaméogo TB, Fonta WM, Wünscher T. 2018. Can social capital influence smallholder farmers’ climate-change adaptation decisions? Evidence from three semi-arid communities in Burkina Faso, West Africa. Soc Sci. 7(3):33. doi:10.3390/socsci7030033.