Abstract
We provide a ‘how to’ guide to undertake systematic reviews of effects in international development, by which we mean, synthesis of literature relating to the effectiveness of particular development interventions. Our remit includes determining the review's questions and scope, literature search, critical appraisal, methods of synthesis including meta-analysis, and assessing the extent to which generalisable conclusions can be drawn using a theory-based approach. Our work draws on the experiences of the International Initiative for Impact Evaluation's (3ie's) systematic reviews programme.
1. Introduction
Our skills should be reserved for the evaluation of policies and programs that can be applied in more than one setting… The lack of this knowledge makes us incompetent estimators of programme impacts, turning out conclusions that are not only wrong, but are often wrong in socially destructive ways.
(Campbell Citation1979, p. 84)
What is the evidentiary basis for trends in development policy? For example, microcredit has grown rapidly in the last three decades, being promoted by both official development agencies and non-governmental organisations (NGO). Microcredit is said to lift people out of poverty and empower women.
However, evidence to support such claims is often anecdotal. For example, a typical NGO website presents a very positive impact: ‘By helping a mother buy a sewing machine to start a tailoring business or a father buy seeds to plant a vegetable garden, small loans enable people in poverty to earn an income and provide for their families … Each successful business feeds a family, employs more people and eventually helps empower a whole community’.Footnote 1
Other claims are based on single studies, rather than a systematic critical appraisal of the whole literature. For example, in commentary that seems to be based on the World Bank impact evaluation of microcredit in Bangladesh (Pitt and Khandker Citation1998), Muhammad Yunus (Citation2005) has argued ‘impact studies done on the Grameen Bank by independent researchers find that 5 per cent of borrowers come out of poverty every year, children are healthier, education and nutrition levels are higher, housing conditions are better, child mortality has declined by 37 per cent, the status of women has been enhanced, and the ownership of assets by poor women, including housing, has improved dramatically’.
And where statements draw on a range of studies, it is not clear whether these statements are truly representative of the literature. The website of the Microfinance Gateway summarises the evidence on impact as follows: ‘Microcredit can provide a range of benefits that poor households highly value including long-term increases in income and consumption … Women participants in microcredit programs often experience important self-empowerment … there is a strong indication from borrowers that microcredit improves their lives’.Footnote 2
In contrast to the above claims, there have been three recent systematic reviews addressing various aspects of the question of whether microcredit is effective, which paint a very different picture. Stewart et al. (Citation2010) conclude that in sub-Saharan Africa ‘some people are made poorer, and not richer, by microfinance, particularly micro-credit clients’ (p. 6), and urge decision-makers to ‘be cautious about offering clients continuing loans’ (p. 7).Footnote 3 Duvendack et al. (Citation2011) argue ‘all impact evaluations of microfinance suffer from weak methodologies and inadequate data [which] can lead to misconceptions about the actual effects of a microfinance programme’ (p. 4) and ultimately that ‘it remains unclear under what circumstances, and for whom, microfinance has been and could be of real, rather than imagined, benefit to poor people’ (p. 76). Focusing on women's empowerment, Vaessen et al. (Citation2012) argue that ‘from those studies deemed comparable and of minimum acceptable quality, we can conclude that overall the effect of microcredit on women's control over household spending is weak’.
The case of microfinance illustrates the importance of systematic reviews. That is, studies which synthesise all the existing high-quality evidence using transparent methods to give the best possible, generalisable statements about what is known.Footnote 4 The field of systematic review and meta-analysis originates in US social science (Glass Citation1976) and has been proven useful for policy and practice in the biomedical field, particularly under the auspices of the Cochrane Collaboration since 1993 (Higgins and Green Citation2011). With the advent of groups such as the Campbell Collaboration in 1999, these tools are being widely applied in the social science field including latterly in international development.
The emphasis on the word ‘systematic’ distinguishes a systematic review from a conventional literature review (Cooper and Hedges Citation1994). Thus, a systematic review has a clear protocol for systematically searching defined databases over a defined time period, with transparent criteria for the inclusion or exclusion of studies, as well as the analysis and reporting of study findings. A systematic review of effects may also involve meta-analysis – that is, the statistical pooling of summative information on study effect sizes.Footnote 5 Finally, to better understand differences in findings by context, theory-based systematic reviews will use an explicit theory of change, collecting data on outcomes along the causal chain.
However, applying systematic review methodology to socio-economic interventions in low- and middle-income countries (LMICs), called here ‘development interventions’, presents new opportunities and challenges. The sources of data, methods of analysis commonly used in primary studies and greater degree of substantive heterogeneity in contexts justify specific guidance for reviewers working in this emerging area of evidence synthesis. In this article, we draw on International Initiative for Impact Evaluation's (3ie's) experience in funding, conducting or managing many of the 100 new systematic reviews, which have been undertaken of social and economic development interventions to date. We draw on and adapt existing approaches to reviews beginning in Section 2 with a discussion of review scoping and question setting, followed by guidance on search (Section 3), critical appraisal (Section 4), data collection (Section 5), synthesis (Section 6) and assessing ability to generalise findings using a theory-based approach (Section 7). Section 8 concludes.
2. Setting the question
A good answer needs a good question. The main issue in setting the question is the breadth of the question. We would all like to know the answer to the question ‘how do we end global poverty and achieve world peace?’ but it is rather broad for a systematic review! Rather, a systematic review will be most successful when the methodology is applied to a clearly defined research question on issues where a review seems sensible. For example, a review in medicine will often ask a narrow question such as ‘the effects of magnesium sulphate for the treatment of eclampsia and pre-eclampsia in maternal health’ (Duley et al. Citation2003). A current criticism of many of the systematic review questions development researchers have attempted to answer is that they are too broad, which inevitably leads to challenges (Mallett et al. Citation2012).
Among reviewers, this debate is known as lumping versus splitting (Gøtzsche Citation2000; cited in Grimshaw et al. Citation2003). ‘Splitters’ contend that it is only appropriate to compare studies which are very similar in terms of design, population, intervention characteristics and outcome; in addition, broad reviews are more cumbersome to manage and time consuming.Footnote 6 On the other hand, ‘lumpers’ argue that broader reviews allow policy relevance since they compare a range of interventions to attain a common goal, allowing policy-makers to select the most effective intervention relevant to their context. Ideally, the study will assess the most cost-effective intervention, though the primary studies rarely have the cost data for this to be possible. Moreover, broadening review scope also enables generalisability to be assessed across a wider range of contexts, study populations and behaviours (Shadish et al. Citation2002, Grimshaw et al. Citation2003) (see also Section 7).
A related issue is whether to set the question around an outcome – ‘what are the effects of community-based intervention packages on neonatal and maternal mortality?’ (Lassi et al. Citation2012) – or an intervention – ‘what are the impacts of daycare programmes?’ (Leroy et al. Citation2012). Splitters would further delimit by combining the two, so for example, ‘what are the impacts of daycare programmes on children's cognitive development?’. Lumpers would then argue that daycare can have a broader range of benefits in developing social skills, and so should not be assessed on their impact on cognitive development alone.
There are also arguments around lumping or splitting evidence from ‘developed’ (high-income) and ‘developing’ country (low- and middle-income) contexts, and within the latter, by geographical region, or context such as ‘fragile’ states. The main argument for splitting is that many interventions in developing countries are different from those in high-income countries, due to the factors relating to extreme poverty, more limited access to basic services, poorer quality of service provision, weaker governance and so on. There may indeed be cases where programmes for, and circumstances facing, particularly marginalised groups in high-income countries are comparable with those in lower-income contexts, and so this evidence might be justifiably included; examples are the proposed reviews by Kristjansson et al. (Citation2012) on early childhood feeding programmes and by Coren et al. (Citation2012) on interventions for street children.
However, even if programmes appear similar, programme effectiveness is likely to differ from that in high-income countries, which may result in either a greater impact (higher returns from a low base) or a lesser one (weaker implementation or lack of response from intended beneficiaries). Another way of stating this is that results may differ due to either ‘absolute’ differences between the developed and the developing countries (for example, background prevalence) or ‘relative’ (differential response due genetic, literacy or feasibility issues) (Oxman et al. Citation2009). This consideration usually favours full splitting, or at least sensitivity analysis according to contextual moderators, as both the reviews cited above are proposing.
Within international development, rigorous impact evaluations are still thin on the ground for many interventions. This fact tends to support lumping over splitting as questions that are too tightly defined will result in empty, or near-empty, reviews. Better to cover a larger range of interventions and outcomes, even if most of those are empty, so the argument goes, since at least more terrain has been mapped. When there is more evidence, the case for splitting is stronger. Thus, there are many primary studies of conditional cash transfers (CCTs), so there are separate reviews for different outcomes, including health and nutrition (Gaarder et al. Citation2010), child stunting (Manley et al. Citation2012), education (Baird et al. Citation2012) and economic outcomes (Kabeer et al. Citation2012).
A useful way to break down the component parts of the review question is through the ‘PICO’ acronym – population, intervention, comparator and outcome (Higgins and Green Citation2011). For example, ‘Behaviour change interventions [intervention] to prevent HIV [outcome] among low-income girls and women living in low and middle income countries [population]’ (McCoy et al. Citation2009). The title omits, as many do, the comparator which may be a no treatment control, a placebo (though this is uncommon for social interventions) or the existing or an alternative treatment. In addition, for economic and social policy interventions, study design is also usefully included in this framework; the PICOS framework thus forming the basis of the study selection criteria of the review (Petticrew and Roberts Citation2006).Footnote 7
3. The search strategyFootnote 8
The literature search provides the ‘raw material’ of a systematic review, and full text access to a range of databases is therefore essential. Developing a comprehensive search strategy is a specialised skill and all review teams should include or consult an information specialist. A comprehensive search should cover both published and unpublished papers, and so avoid publication bias by which null, and possibly negative, findings are less likely to be published. The search should cover published and unpublished sources of literature in three main areas: electronic database searches, screening and hand-searches and literature snowballing.
Electronic searches should cover key bibliographic databases which are (1) multidisciplinary, such as Web of Science and Google Scholar; (2) specific to international development, including the Joint Libraries of the World Bank and IMF (JOLIS) database, the British Library of Development Studies (BLDS) and ELDIS (Institute of Development Studies) and 3ie's database of impact evaluations; (3) specific to social sciences, both general and discipline-specific, such as Social Science Research Network (SSRN), IDEAS/Repec and Econlit for economics, PsycInfo for behavioural studies, ERIC for education and (4) subject-specific, for example, LILACS for Latin American health publications, and the ALNAP evaluative reports database if the question relates to humanitarian interventions, and Medline and EMBase for health.Footnote 9 These databases ensure coverage of literature published in academic journals (Web of Science, Social Science Research Network (SSRN), Econlit, PsycInfo, ERIC, Medline and EMBase) as well as literature published elsewhere, such as in working papers (IDEAS/Repec, Google Scholar and 3ie) or in books and reports (BLDS, ELDIS, JOLIS and ALNAP).
Many studies are identified through the screening of websites of key development and research agencies, such as the World Bank's Documents and Reports database, publications of independent evaluation departments of multilateral development banks and bilateral development agencies (such as the UK's Department for International Development (DFID) or USAID), the 3ie Impact Evaluations DatabaseFootnote 10 and the websites of the Abdul Latif Jameel Poverty Action Lab (J-PAL), Innovations for Poverty Action (IPA) and so on. In addition, hand-searching in libraries enables the search to sweep up studies, which are poorly indexed. This involves the hand-searching of key journals and of library shelves.
In addition to publication bias, ideally language bias should be avoided (for example, by using LILACS, which emphasises health publications in Spanish and Portuguese). Avoiding this bias is usually interpreted to mean that the exclusion criteria cannot rule out studies on grounds of the language in which they were written. A truly comprehensive search would also include search terms in other languages, notably Spanish and Portuguese in international development, because of the sizeable body of primary studies in Latin America, as, for example, implemented by Cirera et al. (Citation2012) in a review on free trade zones.
Search strategies usually base their keywords on PICO. ‘Methods filters’ are sometimes used to ensure that the search results are restricted to studies of interests. In the health field, these filters are more straightforward as study titles are more descriptive of study design and content. Unfortunately, methods filters used in the health field often will not work for the social sciences as a wide variety of terms – such as ‘intervention’, ‘evaluation’, ‘effectiveness’, outcomes if specified, and so on – are used to describe studies and may not appear in the title or abstract of the papers (and therefore will not retrievable in many databases). In most cases, therefore, it will be wise not to include methods terms in searches (see Brunton et al. Citation2012).
Petticrew and Roberts (Citation2006) point out that given the problems in searching social science literature, reference snowballing is especially important. Snowballing involves reviewing and pursuing references in identified papers, including primary studies and existing reviews, and using these sources to build up (that is, snowball) a larger body of evidence. Snowballing includes both bibliographic back-referencing (reviewing references of included studies) and citation tracking (reviewing references in which the included study has been cited). For example, both Web of Science and Google Scholar can be used to identify citations to particular studies, and this can be a good adjunct to conventional database searching. Given publication delays in the social sciences, the search should also necessarily incorporate contacting key experts in the field for information on recent or on-going studies.
Indeed, to identify studies quickly, reviewers may want to conduct snowballing initially, prior to electronic database searching. Having a few key relevant papers at hand before searching is a good way of identifying or ‘pearl harvesting’ key terms to include in a draft search strategy (Sandieson Citation2006). Searching is an iterative process, and as it progresses, additional terms may become apparent which can then be added to the strategy. Key papers may also be searched for in databases to identify subject headings or descriptors applied to them, which can then be included in a strategy in addition to title or abstract words, so taking advantage of both more restrictive ‘controlled language’ approaches to subject indexing and unrestrictive ‘natural language’ methods.Footnote 11
The results of the search should be clearly documented (Moher et al. Citation2009). presents an example search flow from a review on interventions to reduce incidence of female genital mutilation and cutting (Berg and Denison Citation2012). A typical review goes from several thousand or so papers identified in the initial search (often many more in social science literature), to just a couple of hundred for which an abstract or full-text screening review is conducted, and then a dozen or less included effectiveness studies. These figures give the impression that a lot of evidence is being thrown away.
Figure 1. PRISMA flow diagram of literature review process for studies on female genital mutilation and cutting in Africa.
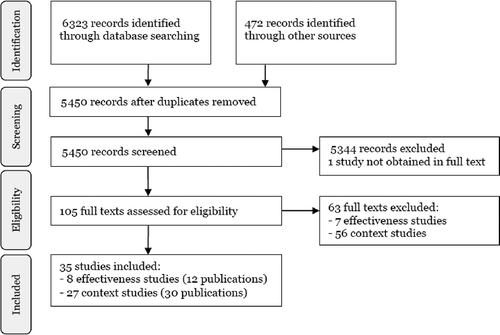
The exclusion of the first several thousand papers should not be an issue. Reviews cast the net very widely to ensure studies are not missed, and so pick up a lot studies that are not actually evaluations of the intervention or outcome of interest. The real issue is at the next stage, going from 100 down to 12. These studies are relevant evaluations, which generally get excluded on grounds of study design. The theory-based approach propounded by 3ie does require that studies without credible designs are excluded from the synthesis of causal effects. However, analysis of the rest of the causal chain requires other types of evidence. And this evidence is thin in studies that are included because of rigorous impact evaluation designs. Hence, there is a need to turn to evidence in the other studies excluded at that final stage, as shown in the additional context studies included in Berg and Denison (Citation2012). However, there is not yet agreement as to what constitutes credible evidence to allow additional studies to be included for this purpose (see Snilstveit Citation2012).
4. Quality assessment
Systematic reviews use clear inclusion criteria, which are ideally set a priori in a protocol. Inclusion criteria are usually not only based on the PICO characteristics, but also include some minimum quality thresholds. Quality assessment of studies thus proceeds in two stages: firstly, inclusion or exclusion of studies based on relevance and study design characteristics, and secondly, a detailed critical appraisal to determine validity of included study designs, based on ‘risk of bias’ evaluation criteria. In effect, in terms of study design, this process determines inclusion of studies based on ‘potential’ bias according to the planned study design, and risk of bias in ‘implementation’ of that design. Hence, it could happen that some excluded studies are more valid than the included low-quality studies. It is not clear how often this problem occurs in practice, nor whether it is particularly important to the findings in a review which is reported appropriately.Footnote 12 The results of the quality assessment – ideally, conducted by at least two reviewers working independently – should be reported for each study and taken into consideration in the synthesis.
4.1. Setting appropriate study design inclusion and exclusion criteria
In a review of effects, establishing criteria for eligible studies means including the types of studies that are considered appropriate and valid for making causal inferences. However, determining which quantitative study designs are valid for causal inference, and which can provide only associational evidence, has been widely debated. In this section, we focus on cases of ‘large n’; that is, studies which have a sufficient number of assignment units to which statistical methods of causal inference can be applied (see White and Phillips Citation2012). Even when statistical techniques are possible, some questions may require reviewers to draw on methods not covered here, such as cross-country regression analysis and computable general equilibrium models. For example, Cirera et al. (Citation2011) conduct meta-regression analysis of studies examining effects of trade reform on employment and government revenue.
Most authors in the quantitative ‘evidence movement’ (for example, Rubin Citation1974, Cook et al. Citation2008, Duflo et al. Citation2008, Shadish et al. Citation2008, Higgins and Green Citation2011, Gertler et al. Citation2011) have agreed that, where an experimental approach based on randomised allocation to the intervention is possible and appropriately designed and implemented, it will usually be the most valid method of causal inference. While randomised control trials (RCTs) are probably the most famous example of causal studies, a range of other quasi-experimental techniques will usually also allow valid causal inference when well implemented (see also Duvendack et al. Citation2012). Quasi-experimental methods are by definition based on non-random allocation and can be applied to assignment rules based on a cut-off on a continuous scale such as a test score or poverty index (regression discontinuity design (RDD)), some other form of exogenous variation (natural experiments) or self-selected assignment determined by programme planners or by beneficiaries themselves (Shadish et al. Citation2002); methods commonly used to identify causation among self-selected groups in international development include instrumental variables (IVs), difference-in-differences (DIDs), propensity score matching (PSM) (Ravallion Citation2008) and, to a lesser extent, interrupted time series (ITS) (Shadish et al. Citation2002).
presents a decision flow for experimental and quasi-experimental designs according to the types of data available to which each method can be appropriately applied. The designs are ordered roughly according to a priori credibility, with more credible designs such as RCTs, RDDs and natural experiments at the top, and less credible designs based on cross-sectional or pre-test/post-test (before versus after, without comparison group) data at the bottom.Footnote 13 Thus, evaluation designs based on knowledge about allocation rules which are external to participants are usually considered more credible than others (Shadish et al. Citation2002, Hansen et al. Citation2011). In the majority of cases, however, assignment rules are not observed and must be modelled. In the case of regression-based techniques, some unobservable characteristics (for example, ability or attitude to risk) can be controlled for, in the case of credible IV both time-varying and time-invariant sources, whereas in the case of double differences (DID and fixed effects panel data regression) time-invariant sources only.
In contrast, single difference (SD) estimation, applied to either cross-section or pre-test/post-test data, is not usually able to control for either. The credibility of matching methods such as PSM for cross-sectional data depends on the extent that unobservable characteristics are correlated with variables which can be observed (for example, socio-economic status, demographic characteristics, location and so on); matching is, however, often preferred to normal SD regression estimation since by construction it includes observations in the region of common support (see Heckman et al. Citation1998).Footnote 14
Due to the logic of confounding, the use of a control or comparison group which receives no (or a different) intervention is usually a key to dealing with the attribution of an effect to the programme, though is not a sufficient condition due to selection bias. Nonetheless, and subject to appropriate risk of bias assessment, the reviewer may want to consider the inclusion of pre-test/post-test (reflexive control) designs when assessing changes in outputs (or outcomes immediately resulting from the intervention) along the causal chain. An example would be beneficiary time-savings resulting from provision of a new amenity like a public water source (White Citation2008).
So, where does this discussion leave us with respect to which types of studies to include in a systematic review, and where to draw the methodological line for inclusion in the review? There are two approaches to inclusion: set the quality threshold bar low a priori, or include only studies judged to be of ‘low risk of bias’. The reviews on microcredit highlighted at the beginning of the paper (Duvendack et al. Citation2011, Stewart Citation2012, Vaessen et al. Citation2012) indicate the value added of reviews based on higher quality evidence; examining the excluded studies' list indicates that there are many microfinance evaluations which did not meet standards of the review, despite all including far broader evidence than RCTs alone. Recent reviews of high quality evidence have also reached different conclusions from reviews based on broader evidence. For example, a recent review of nutritional interventions (Masset et al. Citation2012) found that existing evaluations of agricultural programmes like home gardens were not sufficiently powered to assess the nutritional outcomes they were trying to measure; these results contrasted with the positive effects suggested in a previous review which included lower quality evidence including pre-test/post-test studies (Berti et al. Citation2003).
The Cochrane Collaboration currently recommends setting the bar at RCTs and quasi-RCTs (Higgins and Green Citation2011).Footnote 15 Setting the bar high does, however, mean that empty reviews can be common in a field of study where rigorous evaluation does not exist. The benefits of including broader evidence are to provide more detailed information on where existing studies fall down, and where new primary studies are required, as Koehlmoos et al. (Citation2011) showed in their update of an empty Cochrane review (Koehlmoos et al. Citation2009) on social franchising (a means of creating contracted networks of non-state providers) of health care. The small number of available studies in many international development reviews of effects tends to suggest inclusiveness of study design. The advantage is that this allows researchers to draw on a broader range of evidence, thus avoiding discarding potentially useful information which can be drawn on to inform further evaluation research. The clear disadvantage is the increased potential for including evidence which may be ‘wrong’. Therefore, if broader inclusion is adopted, the important point is that implications for policy and practice should be reported appropriately based on ‘risk of bias’ categories.Footnote 16 In the case of 3ie's meta-analysis of water, sanitation and hygiene interventions (Waddington and Snilstveit Citation2009), we did not find big differences in effects compared with Fewtrell et al. (Citation2005) original analysis, despite the latter including additional high risk of bias effects based on self-selected intervention groups; however, sensitivity analysis by study quality did suggest some differences in moderator effects, particularly in terms of sustainability of impacts.
4.2. Critical appraisal
Inclusion decisions based on design should be followed by detailed critical appraisal of the study. All studies are subject to a range of biases which affect internal validity, statistical conclusion validity, construct validity and external validity (Shadish et al. Citation2002). This section tackles internal validity and statistical conclusion validity, and we discuss external validity further in Section 7.Footnote 17
Internal validity refers to extent to which a causal claim is valid, while statistical conclusion validity assesses whether the effect has been estimated in a precise and unbiased manner. The validity of all study designs depends not only on the design itself but on the execution of the strategy. Thus, design-based assessment is necessary but insufficient to assess causal validity (Littell et al. Citation2008). For example, usually sound methodological designs such as RCTs can have methodological problems in implementation (for example, contamination, problems with the way randomisation was conducted, or non-random attrition rates, and so on) that may require us to interpret results cautiously. Similarly, poorly designed or implemented quasi-experiments will not generate good causal evidence. As argued by Hansen et al. (Citation2011), quasi-experimental designs such as RDD, IV, DID and PSM are more accurate – as measured by the deviation from the result produced by an RCT – the greater the available information on rules determining programme placement and selection.Footnote 18
Risk of bias tools address and test the specific assumptions underpinning the validity of causal attribution methods, using transparent evaluation criteria. While a large number of tools exist to assess risk of bias,Footnote 19 we are not aware of any that enables appropriate evaluation of the quasi-experimental designs discussed here including among those reported in the extensive review by Deeks et al. (Citation2003). We have therefore developed a list of criteria to assess consistently internal validity in social experiments and quasi-experiments, together with evaluation questions relevant to each study design (Hombrados and Waddington Citation2012), summarised in
Table 1. Internal validity appraisal categories for social experiments and quasi-experiments
The categories of bias which undermine causal attribution – selection bias, confounding and group equivalence, spill-overs and reporting biases – are broadly equivalent for experimental and quasi-experimental studies. However, the questions to operationalise the evaluation criteria differ, and the evaluation questions used to assess them are notably more difficult to apply for quasi-experiments. While the validity of all methods of identification, including RCTs, relies on both statistical and qualitative judgement, the evaluation of quasi-experiments requires advanced statistical knowledge.
Selection bias is addressed through the method of assignment or counterfactual identification; for quasi-experiments, this includes assessing different counterfactual identification mechanisms, such as discontinuity assignment, exogeneity of the instrument or the approach to statistical matching. Group equivalence assesses the success of execution of the method, and how well the studies control for external confounders and other factors which may invalidate group equivalence during the process of implementation of the programme, such as non-random attrition. Spill-over effects are particularly important where an intervention can have large externalities (such as the impact of a sanitation improvement on communicable diseases) or is provided in intangible form (such as information) and may be assessed according to the degree of geographical or social separation between groups, for example. Remaining criteria address problems arising due to Hawthorne effects and reporting biases. Since quasi-experimental studies provide greater opportunities for arbitrarily selecting methods, particularly when designed and conducted retrospectively using observational survey data, outcome and analysis reporting biases are disaggregated. Other biases which affect all study designs include bias due to expectations,Footnote 20 courtesy bias, recall bias and other biases in data collection and analysis, and coherence of the results, together with the adequacy of reporting these factors.
For all included studies, reviewers should report each category of bias, such as by using the Cochrane Collaboration's traffic lights reporting tables (Higgins and Green Citation2011, Chapter 8). However, to enable sensitivity analysis, it can be useful to provide an overall decision rule based on an appropriate weighting of the categories. These categories may differ depending on the type of intervention (see Higgins et al. Citation2011). Score-based weighting schemes are not recommended (Deeks et al. Citation2003), but it is useful to determine an overall ‘risk of bias’ associated with each effect size, for example, based on minimum acceptable bias reported on particular categories. GRADE provides a means of determining risk of bias without scoring.Footnote 21
The final internal validity category relates to statistical significance, which refers to the estimate of dispersion (statistical significance) of the effect; this includes a number of factors, though here we discuss statistical power and unit of analysis errors only. The power of a study is defined as the probability of detecting a significant effect size of a given magnitude. Among other factors it depends on the study sample size and the magnitude of the effect size. The results from underpowered studies need to be interpreted with caution, particularly when the analysis yields non-significant results. In the latter situation, it is not possible to determine whether the intervention truly has no effect or whether this result is due to the study's lack of ability to detect an effect because of insufficient sample size. The aggregation of studies in a meta-analysis can partly account for the problems related with lack of power of included studies (Borenstein et al. Citation2009). The assessment of statistical power can include either an ex post calculation of the power of the study for the point estimate of the effect, or report the power of the study to detect pre-established significant effects' magnitudes (for example, the power of the study to detect a significant 10 per cent, 20 per cent and 50 per cent impact effect on the outcome).Footnote 22 For an example in the context of a systematic review, see Masset et al. (Citation2012).
Unit of analysis error arises when the unit at which the intervention is implemented and the unit of data analysis differ, for example, when the intervention is delivered at a cluster level (for example, village or household), but the analysis is carried out at the individual level and no attempt is made to control for clustering in the analysis. The idea behind the unit of analysis error lies in the assumption that individuals within the same clusters are likely to be more similar in their response than individuals across clusters. In such a case, the observations within clusters cannot be considered independent from one another, and therefore, the effective sample size is smaller than the total sample size. A bias is introduced if clustering is not taken into account in meta-analysis: the analysis yields narrower confidence intervals than the true confidence intervals, increasing the risk of type I error as well as the weight of the study in a meta-analysis, thus biasing the pooled effect.
Although the unit of analysis problem has been mainly analysed in the context of cluster randomised trials, it can be also a matter of concern in quasi-experimental studies in which treatment allocation is clustered (Calhoun et al. Citation2008).Footnote 23 If assessment suggests that unit of analysis is indeed different from the unit of treatment assignment, the reviewers must assess whether the authors have taken clustering into account in the analysis (for example, using multilevel model, variance components analysis, cluster level fixed effects or generalised estimating equations).Footnote 24 For those studies with relevant risk of unit of analysis error, corrections may be applied to the standard errors (SEs) and confidence intervals of those studies. Adjusted SEs for those studies with relevant risk of unit of analysis error can be estimated as follows:
where m is the number of observations per cluster and ICC is the intra-cluster correlation coefficient, which is an estimate of the relative variability within and between clusters. Since the data for estimating the ICC are often unavailable, it may be necessary to approximate ICCs based on studies reporting them on the same or a similar subject.Footnote 25
5. Data collection and effect size calculation
All reviews should collect extensive data from each study on populations, interventions (and co-interventions), comparison conditions, outcomes, contextual factors and other effect moderators, the codebook for data collection being presented in the study protocol. In this section, we focus on calculation of effect sizes based on reported outcome data. These data should be collected for all relevant outcomes reported (both positive and negative), relevant sub-groups (for example, by gender or age) and where studies include multiple follow-ups, time periods too. A theory-based approach will collect outcomes data reported along the causal chain, from outputs and intermediate outcomes, to ‘endpoint’ or final outcomes (see Section 7).
Quantitative data on outcomes should be converted into effect sizes. An effect size is a statistical measure of the change in outcomes in the intervention group, over the comparison group. A good effect size estimate should be comparable across studies – that is, independent of units of measurement – and only reflect effect magnitude for each study, and not other factors such as sample size (Lipsey and Wilson Citation2001, see also Duvendack et al. Citation2012). Studies included in a systematic review often use a range of different metrics for expressing the effect size, which means that systematic review authors commonly have to recalculate effect sizes from individual studies, transforming them into a common metric.
The type of metric being used depends on the type of outcome variable being measured. For continuous outcomes like income, we usually calculate the standardised mean difference (SMD), which measures the size of the intervention effect in terms of the number of standard deviations in the outcome variable. In the case of dichotomous outcomes like school attendance – that is, when the outcome of interest is a categorical variable that can only take the numerical value of 0 or 1 – we calculate the risk ratio (RR) or odds ratio, which measures the ratio between two proportions, the dichotomous outcome level in the treatment group to the dichotomous outcome level in the comparison group.
Two issues are worth noting here. The first relates to the use of relative (standardised mean and ratio) estimates of effect size versus use of absolute mean and risk differences. Usually, standardised and ratio estimates are preferred when making comparisons across contexts – as in cases when interventions are conducted under high versus low prevalence of the problem, or among different disadvantaged groups – since they factor in differences in baseline conditions (Higgins and Green Citation2011, Chapter 12.5). An example is achieving the final mile in universal primary education: the required interventions are likely to be different in a situation in which only 60 per cent of children are regularly attending school from that in which we are trying to reach the final 5–10 per cent, and the comparison of relative effectiveness across contexts is therefore best described using a standardised mean or ratio estimate which takes into account the starting point (for an example in education, see Petrosino et al. Citation2012). However, communicating findings to decision-makers is often best expressed using natural frequencies (or in healthcare, numbers needed to treat), which are derived from the absolute difference. When presenting findings, it can therefore be useful to present results in terms of relative and absolute effects and discuss the implications of differences in absolute or relative effects for different contexts.
A second issue relates to whether we should calculate effect sizes at all, given their computational difficulty, particularly for the quasi-experimental designs discussed above. In addition to facilitating statistical synthesis, the main benefit of calculating the effect size is that it enables assessment of the magnitude of the average treatment effect, and therefore the ‘policy significance’ of the intervention. The methods for calculating the effect size from experimental studies are well known (see Borenstein et al. Citation2009). provides formulae for calculating SMD and RR effect sizes and their SEs for studies that use statistical matching and regression analysis (see also Lipsey and Wilson Citation2001). While just because something is difficult does not mean that it is not worth doing, teams need to ensure they have the advanced statistical expertise to incorporate a wide range of quasi-experimental designs in their reviews.
Table 2. Formulae for calculation of effect size and its SE for studies using parallel group or matching, and regression analysis
A more relevant problem limiting calculation of effect sizes is that it may simply be not possible to do so for SMD effect sizes based on the information reported in primary literature in the social sciences. The usual options available are to attempt to obtain information by contacting the study authors, or to calculate other effect size estimates such as response ratios for continuous variables.Footnote 26 In some cases where there are insufficient study-level comparisons to necessitate the extraction of effect sizes and meta-analysis, it is possible that the computational burdens could outweigh the benefits (for an example, see Leroy et al. Citation2012). However, the benefits of meta-analysis, where possible and appropriate, are substantial, as discussed in the next section.
6. Synthesising the evidence
Synthesis of evidence on effects may be either quantitative, using the statistical technique of meta-analysis, or narrative.Footnote 27 Meta-analysis is usually promoted over other methods of synthesising quantitative summary findings on the grounds that it improves statistical power, enabling reviewers to overcome sampling errors in individual studies (Cohn and Becker Citation2003). According to Chalmers (Citation2005, p. 234), ‘when it is both possible and judged appropriate … meta-analysis can reveal “reconcilable differences” among studies, and avoid the play of chance resulting in misleading inferences about the effects of interventions’.
All scientific evidence is uncertain or probabilistic in nature. By enabling quantification of an average effect, together with the likely range of that effect in different contexts based on a confidence interval, meta-analysis also improves the review's policy relevance. It allows the overall effect size and the variance from different studies to be calculated, thereby giving a strong indication of the likely impact of a policy intervention, as well as information on who is likely to benefit from the intervention and who will not. In effect, it can provide policy-makers with an indication of both the ‘signal’ and the ‘noise’ that is associated with a policy intervention, enabling comparison of effectiveness (and when evidence is available cost-effectiveness) of different interventions.
The traditional and, in international development, still most common, method of quantitative synthesis is ‘vote-counting’ or ‘goal-scoring’ – that is, adding up the number of studies finding a positive, negative and no impact. The category with the greatest number of studies is sometimes ‘assumed to give the best estimate of the direction of the true [effect]’ (Light and Smith Citation1971, p. 433; cited in Hunter and Schmidt Citation2004, p. 446).
Simply put, vote counting is inappropriate and can lead to misleading conclusions. Consider the two 95 per cent confidence intervals in (coefficients are SMDs), which are from studies reporting impacts of school feeding on school attendance (Petrosino et al. Citation2012). These two studies appear very consistent, with virtually identical point estimates. However, a significance-based vote-counting approach based on the 95 per cent confidence intervals would simply state that the two studies yield inconsistent results, a statement that seems less sound if we learn that the total sample size for study one was 350 and study two was 5000.Footnote 28 The heart of the problem is that vote counting relies upon ‘one study, one vote’ taking account of neither the magnitude of the effect size nor its precision. While vote counting by statistical significance may attempt to account for precision, it still fails to take into account the magnitude of effect (Littell et al. Citation2008).Footnote 29 Meta-analysis, which is the statistical pooling of effect sizes weighted by a measure of sample size (usually, the inverse of the variance) from studies identified for inclusion in the review, does enable these factors to be controlled.
Table 3. Effect sizes for two school feeding programmes
Meta-analysis pools the findings from different studies to provide a single point estimate with increased statistical power, together with the likely range of effects based on the associated confidence interval (Cohn and Becker Citation2003). The increase in power can mean that a collection of studies with positive but insignificant findings can be pooled to yield a significant estimate; this may be particularly beneficial for ‘rare’ outcomes such as maternal mortality.Footnote 30 Indeed, as shown in , when we pool the results of the inconclusive studies using meta-analysis, we estimate a significant positive effect of the intervention overall. Equally, meta-analysis can increase our confidence in a null-effect by narrowing down the confidence interval around zero sufficiently to conclude that, if there is an effect, its magnitude is too small to be of ‘policy significance’ (Greenhalgh Citation2001).
Meta-analysis can be used to generalise from the sample of studies based on different assumptions about the distribution of effects. The assumption of fixed effect meta-analysis is that the underlying treatment effect is common across all studies. Hence, differences in study findings in fixed effect meta-analysis are assumed to be due to sampling error (chance) only (Riley et al. Citation2011). In contrast, random effects meta-analysis estimates the average effect across studies, allowing for differences due to both chance and other factors which affect estimates. We refer to some of these factors as sources of ‘programme heterogeneity’, such as the study location, characteristics of the population receiving the intervention or the intensity of the intervention received or its length. Others relate to ‘methodological heterogeneity’ of the study design, including whether the treatment effect is estimated over population sub-groups (as in a local average treatment effect (LATE), estimator, for example).Footnote 31 The random effects confidence interval is therefore wider than that estimated in a fixed effect meta-analysis, reflecting the additional uncertainty around the estimate.
Cohn and Becker (Citation2003, p. 250) argue that due to these properties, ‘random effects analyses may permit generalisations that extend beyond the studies included in a review’, assuming the included studies are sufficiently homogeneous. In systematic reviews of socio-economic interventions, we usually expect heterogeneity to arise from other sources, as well as chance alone, necessitating the use of random effects models on a priori grounds, together with moderator analysis based on variables representing possible sources of heterogeneity.Footnote 32 When sufficient studies are available to enable it, we can explore heterogeneity in a multivariate context using meta-regression analysis; see Hunter (Citation2009) for a demonstration in the context of household water treatment efficacy trials.
Meta-analysis also enables the quantification of publication bias. presents funnel plots (Egger et al. Citation1997) for studies examining the effects of farmer field schools extension on agricultural yields. The plots aim to provide a graphical depiction of publication bias, based on the rationale that small studies are more likely to be unreported than large studies (note that the y-axis showing the SE, corresponding to sample size, is inverted with large studies measured at the top).
Figure 3. Funnel plots showing small study bias in studies assessing effects of farmer field schools on agricultural yields.
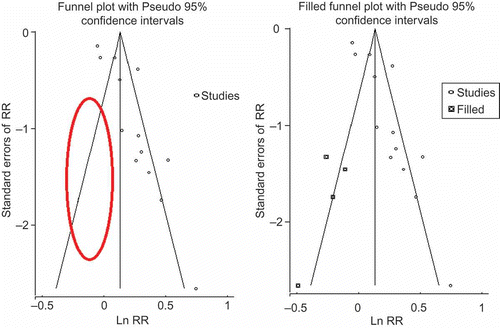
The asymmetry in the plot, as highlighted by the lack of small sample studies which report findings below the average effect at the vertical line, suggests evidence for publication bias. Imputation of missing studies, using ‘trim-and-fill’ analysis (Duval and Tweedie Citation2000) suggests where we might expect those unpublished studies to appear and the re-estimated pooled effect accounting for these studies. In this case, the average pooled effect is reduced in magnitude by one-third.Footnote 33
However, it is not always appropriate to undertake statistical meta-analysis, and when it is possible the limitations of the approach should be acknowledged. Where reviews are broad in scope, involving a range of different interventions, it makes little sense to estimate pooled effects, though this practice is surprisingly common. However, even for reviews covering a discrete intervention, where the evidence suggests that the sources of heterogeneity are as severe to produce the opposite effect to that desired, or to produce particularly large effects, it may not be appropriate to conduct meta-analysis across all, or indeed any, studies (Higgins and Green Citation2011). Important differences in the comparison condition – no treatment versus alternate treatment – are a good example where we might expect this to occur, as was deemed the case in a review of behaviour change interventions to combat HIV (McCoy et al. Citation2009). Another case might be where despite similar intervention–comparator combinations evidence suggests important differences in implementation fidelity (Snilstveit Citation2012, discusses an approach to examine this). Differences in study design and risk of bias status necessitate sensitivity analysis at the very least and may invalidate cross-study comparisons altogether. Given the expected heterogeneity for development interventions, the extent to which reviewers go on to estimate average effects across all studies using meta-analysis is likely to be an evidence-based judgement, which inevitably includes how different are the individual study results.
Whether or not meta-analysis is performed the results should be discussed narratively, along the causal chain. Where meta-analysis is not sensible or possible, the narrative synthesis should describe the primary studies and attempt to arrive at some ‘over-arching theory that reconciles the findings’ (Hunter and Schmidt Citation2004, p. 445). Littell et al. (Citation2008) describe some of the approaches, cautioning that the intended objectivity of the systematic review approach should be retained through transparent decision rules, including on how to weight studies in the synthesis. Narrative syntheses that do not distinguish between null findings that result from low power, and null findings that reflect a genuine absence of treatment effects of policy relevant magnitudes, should also be avoided. However, in the worst case scenarios, reviews which provide only a narrative will produce no more than a summary of each included study, the resulting lack of synthesis being of limited use to policy decision-makers.
In conclusion, where statistical meta-analysis is not possible or appropriate, reviewers should take sample size and magnitude of effect into consideration when interpreting findings, by always presenting the direction, magnitude and statistical significance of findings, using effect sizes where calculable, together with information about the sample size and risk of bias. Ex post power calculations can help ensure that studies are not underpowered for the desired magnitude of effect (Masset et al. Citation2012). Most importantly, a synthesis rather than just a summary should be carried out, with evidence weighed transparently based on explicitly stated and critically chosen criteria.
7. Generalising evidence from systematic reviews using a theory-based approach
The main benefit of a single study, its context specificity, is also its weakness when attempting to draw more generalisable conclusions. Many evaluation studies are conducted over relatively small samples, in a single geographic location, or under conditions which may not closely resemble those of programme implementation – for example, participants may be volunteers, or provided the intervention at zero cost, or subject to repeated observation, and so on.
By synthesising information from multiple studies conducted in different contexts and across different groups of beneficiaries, systematic reviews do provide a stronger basis for generalising findings than single studies (Cooper and Hedges Citation1994). Indeed, Petticrew and Roberts (Citation2006, p. 149) argue that a systematic review ‘itself provides a test of generalisability’, since where findings are similar across a range of different conditions this increases the confidence that findings are transferable to other settings.
Nevertheless, a major challenge for systematic reviews is in assessing the extent to which the findings from such studies are relevant across a range of different populations and, in particular, to ‘real world’ contexts. While issues relating to the ability to generalise findings are the case for all systematic reviews of evidence – whether relating to health care, education, social welfare, crime and justice and so on – the wide diversity of settings (and, for the moment, relatively small collection of high quality impact evidence) does suggest this is particularly relevant for many reviews in international development. ‘Generalisability’ can refer to a number of concepts such as ‘external validity’ in terms of whether the study has relevance to other contexts and in particular to ‘real world’ programmes, ‘transferability’ of findings to another setting, or ‘applicability’ in terms of whether the intervention process could be implemented in that setting. As indicated in , reviews are capable of assessing external validity, but will not usually be motivated towards assessing transferability or applicability unless the methods of analysis are set up to do so explicitly.
Table 4. Generalising evidence using systematic reviews
Systematic reviews do not commonly assess external validity, and a brief review of guidelines and text books on systematic review methodology reveals limited guidance on methods authors should use to deal with it. These sources do note, however, that variations in effects across setting, population or intervention characteristics can inform judgement about the conditions under which different findings are likely to be applicable (Petticrew and Roberts Citation2006). Authors should therefore explicitly discuss the results with reference to the range of settings of included studies and explore possible relationships between study characteristics and findings (CRD Citation2008). In addition, where studies include effect estimates for sub-groups (for example, boys and girls), these will ideally be reported in separate analyses (for an example for education studies, see Petrosino et al. Citation2012).Footnote 34
Use of an explicit theory of change, and collecting data on outcomes along the causal chain, not just on ‘endpoint’ outcomes, can be useful in attempts to explain effect size heterogeneity. A programme theory can set out hypotheses about the characteristics of contexts, populations and interventions likely to affect findings (Anderson et al. Citation2011), which can then be tested empirically. Both contributions in this issue by Leroy et al. (Citation2012) on daycare and Stewart et al. (Citation2012) on microcredit present theories of change. Drawing on a systematic review of effects by Kristjansson et al. (Citation2006), Greenhalgh et al. (Citation2007) used a theory-based approach to examine school feeding interventions. Examples of consolidated theory-based systematic reviews include Waddington and Snilstveit (Citation2009) on water and sanitation, King et al. (Citation2011) on community-driven development and Lassi et al. (Citation2012) on community health. Berg and Denison (Citation2012) also draw on theory to synthesise both quantitative and qualitative evidence on interventions to prevent female genital mutilation and cutting in Africa.
Finally, the external validity of primary studies themselves can be assessed, and incorporated into the analysis. The external validity of primary studies depends, among others, on whether the intervention was implemented in controlled (efficacy) or ‘real-world’ (effectiveness) settings, and by context (time and space), sampling frame, and duration of intervention and follow-up (Bracht and Glass Citation1968). The tool presented in Valentine and Cooper (Citation2008) contains questions for assessing a study's external validity. Theory can also be insightful in determining the external validity of evidence collected under more controlled settings. For example, Waddington and Snilstveit (Citation2009) drew on Rogers' (Citation2005) Diffusion of Innovations to explore effectiveness and sustainability of water, sanitation and hygiene interventions; presents these characteristics.
Table 5. Factors underlying adoption of interventions
8. Conclusion
Conducting systematic reviews of effects in the field of international development has presented several challenges: great heterogeneity in context and intervention design, but a paucity of primary studies which are able credibly to address causality. If systematic reviews are to be a useful tool for international development, we have shown how we believe the methodology should be adapted for the types of programme evaluations which are now commonly used in international development.
The first attempts at applying systematic review methodology have rightly focused on establishing good review practice. But that practice also needs to evolve to produce studies which are both rigorous and relevant and so have the potential to inform policy and improve lives. This article has summarised the key stages, processes and methods of systematic reviews of effects, with particular attention to their application to international development interventions, programmes and policies. We conclude that the continued and expanded use of systematic reviews in international development will raise the quality of evidence to support both policy and practice.
Acknowledgements
The authors thank the established communities of practice in systematic review and meta-analysis from whom we learn, and to the emerging community in international development from whom we draw inspiration. The authors also thank the external reviewers and our colleagues at 3ie, Annette Brown, Eric Djimeu and Shari Krishnaratne, for their comments. All errors are the responsibility of the authors.
Notes
1. Opportunity International, Australia, website: http://www.opportunity.org.au/What-We-Do/Our-Philosophy.aspx?gclid=CMO1uaem960CFQV66wodTHCOqw [Accessed 30 January 2012].
2. http://www.microfinancegateway.org/p/site/m/template.rc/1.26.12263/ [Accessed 30 January 2012].
3. This issue contains a version of the review by Stewart et al. (Citation2012).
4. It is also an example of where synthesis of rigorous evidence contradicts the received wisdom. There are many examples of reviews which show that development interventions do work – such as Petrosino et al. (Citation2012) on education enrolment interventions and Lassi et al. (Citation2012) on community-based health interventions – and how to make them work better, such as King et al. (Citation2011) on community-driven development.
5. In addition to questions about programme effects, there are different types of systematic review and methods of synthesis for a range of policy relevant questions. See Lavis (Citation2009) and Snilstveit et al. (Citation2012).
6. Indeed, two of 3ie's broadest studies – on interventions to increase education enrolments, and the impact of microfinance on empowerment – have both run more than two years over schedule.
7. Other variants exist, such as ‘time’ in the case of PICOT (see Haynes et al. Citation2005).
8. Hammerstrøm et al. (Citation2010) provide a comprehensive guide to searching for Campbell Collaboration reviews.
9. A useful guide to databases in the field which cover LMICs is available from the Cochrane EPOC Group: http://epocoslo.cochrane.org/lmic-databases. ‘Database finder’ guides from major university libraries can also be useful, for example, http://www.library.tufts.edu/resourceDB/.
11. For those new to database searching a short guide to the basic principles is available in Eyers (Citation1998).
12. For example, the empirical literature suggests that a cross-sectional (SD) regression study can be more valid than a poorly implemented instrumental variables (IV) regression. If we set the bar on study design to include all IVs and exclude all cross-section regression studies, regardless of risk of bias assessment, we may therefore end up excluding less invalid studies than some of the included. However, cross-section regression studies would very rarely, if ever, be considered to be any less than of ‘high risk of bias’ status, and sensitivity analysis should ensure that the review results are not weighted according to the results of any low quality IVs. On the other hand, setting the bar for inclusion based on ‘risk of bias’ rather than on ‘study design’ would imply a considerable amount of resources (assessing risk of bias of every single study design) for marginal studies and would not change the review's implication for policy, which should in any case be based only on sufficiently credible causal analysis.
13. We have identified one guidelines document from the UK Medical Research Council (Craig et al. Citation2011) which covers quasi-experimental designs, referring to them as ‘natural experiments’; in contrast, we use ‘natural experiment’ to refer to those designs where there is natural exogenous variation in treatment, which in social science literature is usually based on geographical variation.
14. NICE (Citation2005) further distinguishes cross-sectional studies from case–control and cohort studies, which are more commonly used in epidemiology, based on data in which outcomes and exposures are not measured contemporaneously.
15. Cochrane's Effective Practice and Organisation of Care Group (EPOC) extends inclusion to controlled before and after and interrupted time series studies.
16. If the bar is set sufficiently low to include evidence which might be considered correlational or associational, the review findings must be interpreted through clear separation of higher quality causal and associational evidence, and implications for policy should not be drawn from the latter.
17. Construct validity – or the relevance of the study to the constructs we are interested in measuring – also warrants assessment, for example, according to the appropriateness of intervention and outcomes characteristics (see Valentine and Cooper Citation2008). See also the discussion of construct validity in Vaessen's et al. (Citation2012) review on microcredit and women's empowerment.
18. Duvendack et al. (Citation2012) provide a fuller review of within-study comparisons.
19. See Higgins and Green (Citation2011) for medical experiments and Coalition for Evidence-Based Policy (Citation2010) for social experiments. For tools covering non-randomized studies, see EPHPP (n.d.), EPOC (n.d.), NICE (Citation2009); see also Petticrew and Roberts (Citation2006, p. 135) and Deeks et al. (Citation2003) for an extensive list. For a good example of a comprehensive risk of bias tool which assesses a fuller range of validity sources, see Valentine and Cooper (Citation2008).
20. Expectation effects may confound the causal mechanisms embodied in the particular intervention (Scriven Citation2008). However, social interventions usually require behaviour change from participants, and expectations may form an important mechanistic component in the process of behaviour change. Therefore, isolating expectation effects (such as placebo effects) from other causal mechanisms may be less relevant. However, factors relating to motivation of those being observed (Hawthorne effects) can still be of major concern in trials.
22. Power analysis for risk ratios and SMDs can be performed with the sampsi command in Stata. Details on the formulae for these calculations are available in Fleiss et al. (Citation2003).
23. For clustered quasi-experimental studies based on regression estimation, the unit of analysis error arises when, conditional on the covariates and characteristics controlled for, the observations within clusters cannot be considered independent one from each other. That is, when the covariates and methods used in the regression do not fully account for the differences between individuals across clusters. The validity of regression analysis is based on the assumption of independence of the error term across observations conditional on the covariates. If this condition is not fulfilled, the regression framework yields a biased result. Therefore, in a regression analysis, the existence of unit of analysis error not taken into account in the analysis would not only cause the size of the confidence intervals to be underestimated but also a biased treatment effect.
24. For cluster randomised studies, see Higgins and Green (Citation2011, Chapter 16.5).
25. The Health Research Unit provides ICCs for different interventions and outcomes http://www.abdn.ac.uk/hsru/research/delivery/behaviour/methodological-research/. Unfortunately, ICCs are not yet widely available in a database for development interventions.
26. For continuous outcomes, if the data reported or obtainable from the authors are not sufficient to estimate SMD, it may be necessary to estimate response ratios, which offer greater possibilities both for estimation and comparability across study designs (for example, in making comparisons across SD and double difference estimates). Response ratios measure the proportionate change in the outcome between the intervention and the control group (Borenstein et al. Citation2009). The formula is the same as that for calculating risk ratios (see ).
27. Synthesis of qualitative data is discussed in the article by Snilstveit et al. (Citation2012).
28. Total effective sample sizes according to the unit of randomisation, which was at school (cluster) level, are reported as 10 (Jacoby et al. Citation1996) and 50 (Vermeersch and Kremer Citation2004). Note also that Jacoby et al. are reporting the effects of school feeding on children approximately 11 years old, while Vermeersch and Kremer are reporting the effects of pre-school feeding on children of 4 years of age.
29. While statistically correct methods of vote-counting, both those based on statistical significance and those based on the direction and/or magnitude of effect, have been developed (for example, Hedges and Olkin Citation1980), the key assumption of these methods is that ‘the population effect size... does not vary across studies’ (that is, the fixed effect assumption) (Hunter and Schmidt Citation2004, p. 453); this means that they are unlikely to be valid for most social interventions. They are also less efficient estimators producing wider confidence intervals than meta-analysis.
30. Similarly, individual studies may have insufficient power to detect significant effects in sub-group analysis. If these sub-groups are present in the primary studies, however, then meta-analysis is better able to analyse the heterogeneity of impact.
31. There is a debate around the comparability (external validity) of effect sizes based on use of different treatment effect estimators such as intention-to-treat, average treatment effect on the treated (ATET) or LATEs that may need to be explored in heterogeneity analysis (see Duvendack et al. Citation2012).
32. Statistical tests for heterogeneity such as I-squared, which measures the percentage of variability in effects that is likely due to between study heterogeneity rather than chance, should be reported alongside meta-analysis findings. However, the meta-analysis model should be chosen a priori, and test statistics such as I-squared, Tau and Q used to diagnose the extent to which we can trust the pooled estimate, or whether further moderator analyses by sub-groups of studies are required (Borenstein et al. Citation2009). The consequence of using fixed effect meta-analysis inappropriately is to underestimate confidence intervals, and thus increase chance of Type I error.
33. Given difficulties in accurately assessing asymmetry by visual inspection, reviewers are recommended to rely on statistical tests and report the results of ‘trim and fill’ even when visual inspection is not conclusive. Examination of publication bias can also be done in the absence of meta-analysis, using Eggers’ statistical test for asymmetry based on small study effects. In our example, Eggers test provides support for asymmetry at high levels of significance (p < 0.000). The response ratio (RR) effect size (95% confidence interval) estimated by ‘trim and fill’ analysis in the example here is 1.14 (1.05–1.24) as compared to 1.22 (1.11–1.34) from the original meta-analysis. These figures correspond to a reduction in average effect size by 8 percentage points from a 22 per cent increase in yields for field school graduates over the comparison group, to 14 per cent; confidence intervals are statistically significant in both cases and overlap.
34. Analysis of qualitative data can also be useful where this is available (see Snilstveit Citation2012, this issue for a methodological discussion).
References
- Adi , Y. 2007 . Systematic review of the effectiveness of interventions to promote mental wellbeing in primary schools , Coventry : University of Warwick .
- Anderson , L.M. 2011 . Using logic models to capture complexity in systematic reviews . Research synthesis methods , 2 ( 1 ) : 32 – 42 .
- Baird, S., et al., 2012. Effectiveness and cost effectiveness of conditional cash transfers versus unconditional cash transfers in improving education (Protocol). Campbell systematic reviews [online]. http://campbellcollaboration.org/lib/project/218/ (http://campbellcollaboration.org/lib/project/218/) (Accessed: 15 September 2012 ).
- Berg, R. and Denison, E., 2012. Interventions to reduce the prevalence of female genital mutilation/cutting in African countries. Campbell systematic reviews [online], 9. www.campbellcollaboration.org/lib/download/2101/ (http://www.campbellcollaboration.org/lib/download/2101/) (Accessed: 30 June 2012 ).
- Berti , P. , Krasevec , J. and Fitzgerald , S. 2003 . A review of the effectiveness of agricultural interventions in improving nutrition outcomes . Public health nutrition , 7 : 599 – 609 .
- Borenstein , M. 2009 . Introduction to meta-analysis , Chichester : Wiley .
- Bracht , G. and Glass , G. 1968 . The external validity of experiments . American educational research journal , 5 ( 4 ) : 437 – 474 .
- Brunton , G. , Stansfield , C. and Thomas , J. 2012 . “ Finding relevant studies ” . In An introduction to systematic reviews , Edited by: David , G. , Sandy , O. and James , T. London : Sage .
- Calhoun , A. 2008 . Addressing the unit of analysis in medical care studies. A systematic review . Medical care , 46 ( 6 ) : 635 – 643 .
- Campbell , D.T. 1979 . Assessing the impact of planned social change . Evaluation and program planning , 2 : 67 – 90 .
- Centre for Reviews and Dissemination . 2008 . Systematic reviews: CRD's guidance for undertaking reviews in health care , York : Centre for Reviews and Dissemination, University of York .
- Chalmers , I. 2005 . If evidence-informed policy works in practice, does it matter if it doesn't work in theory? . Evidence & policy , 1 ( 2 ) : 227 – 242 .
- Cirera, X., Lakshman, R., and Spratt, S., 2012. The impact of export processing zones on employment, wages and labour conditions in developing countries (Protocol) [online]. New Delhi: 3ie. http://www.3ieimpact.org/en/evidence/systematic-reviews/details/212/ (http://www.3ieimpact.org/en/evidence/systematic-reviews/details/212/) (Accessed: 15 June 2012 ).
- Cirera , X. , Willenbockel , D. and Lakshman , R. 2011 . What is the evidence of the impact of tariff reductions on employment and fiscal revenue in developing countries? , London : EPPI-Centre, Social Science Research Unit, Institute of Education, University of London . Technical report
- Coalition for Evidence-Based Policy, 2010. Checklist for reviewing a randomized controlled trial of a social program or project, to assess whether it produced valid evidence [online]. http://coalition4evidence.org/wordpress/wp-content/uploads/Checklist-For-Reviewing-a-RCT-Jan10.pdf (http://coalition4evidence.org/wordpress/wp-content/uploads/Checklist-For-Reviewing-a-RCT-Jan10.pdf) (Accessed: 30 January 2012 ).
- Cohn , L.D. and Becker , B.J. 2003 . How meta-analysis increases statistical power . Psychological methods , 8 ( 3 ) : 243 – 253 .
- Cook , T. , Shadish , W. and Wong , V. 2008 . Three conditions under which experiments and observational studies produce comparable causal estimates: new findings from within-study comparisons . Journal of policy analysis and management , 27 ( 4 ) : 724 – 750 .
- Cooper , H. and Hedges , L. , eds. 1994 . Handbook of research synthesis , New York : Russell Sage Foundation .
- Coren , E. 2012 . Interventions for promoting reintegration and reducing harmful behaviour and lifestyles in street-connected children and young people (Protocol) . Cochrane database of systematic reviews , 4 : CD009823 doi: 10.1002/14651858.CD009823
- Craig, P., et al., 2011. Using natural experiments to evaluate population health interventions: guidance for producers and users of evidence [online]. London: Medical Research Council. http://www.mrc.ac.uk/Utilities/Documentrecord/index.htm?d&=MRC008043 (http://www.mrc.ac.uk/Utilities/Documentrecord/index.htm?d&=MRC008043) (Accessed: 15 July 2012 ).
- Deeks , J. 2003 . Evaluating non-randomised intervention studies . Health technology assessment , 7 ( 27 ) : 1 – 173 .
- Duley , L. , Gulmezoglu , A.M. and Henderson-Smart , D.J. 2003 . Magnesium sulphate and other anticonvulsants for women with pre-eclampsia . Cochrane database of systematic reviews , 2 : CD000025
- Dulfo , E. , Glennerster , R. and Kremer , M. 2008 . “ Using randomization in development economics research: a toolkit. In: T. Paul Schultz ” . In Handbook of development economics , Edited by: Strauss , J. Vol. 4 , Amsterdam : North-Holland .
- Duval , S. and Tweedie , R. 2000 . A nonparametric “trim and fill” method of accounting for publication bias in meta-analysis . Journal of the american statistical association , 95 ( 449 ) : 89 – 98 .
- Duvendack, M., et al., 2011. What is the evidence of the impact of microfinance on the well-being of poor people? [online]. London: The EPPI-Centre, Social Science Research Unit, Institute of Education, University of London. http://eppi.ioe.ac.uk/cms/Default.aspx?tabid=3178 (http://eppi.ioe.ac.uk/cms/Default.aspx?tabid=3178) (Accessed: 30 January 2012 ).
- Duvendack , M. 2012 . Assessing ‘what works’ in international development: meta-analysis for sophisticated dummies . Journal of development effectiveness , 4 ( 3 ) : 456 – 471 .
- Effective Practice and Organisation of Care Group (EPOC), Undated. Suggested risk of bias criteria for EPOC reviews [online]. http://epocoslo.cochrane.org/sites/epocoslo.cochrane.org/files/uploads/Suggested%20risk%20of%20bias%20criteria%20for%20EPOC%20reviews.pdf (http://epocoslo.cochrane.org/sites/epocoslo.cochrane.org/files/uploads/Suggested%20risk%20of%20bias%20criteria%20for%20EPOC%20reviews.pdf) (Accessed: 7 March 2012 ).
- Effective Public Health Practice Project (EPHPP), Undated. Quality assessment tool for quantitative studies [online]. http://www.ephpp.ca/PDF/Quality%20Assessment%20Tool_2010_2.pdf (http://www.ephpp.ca/PDF/Quality%20Assessment%20Tool_2010_2.pdf) (Accessed: 7 March 2012 ).
- Egger , M. 1997 . Bias in meta-analysis detected by a simple, graphical test . British medical journal , 315 : 629 – 634 .
- Eyers, J.E., 1998. Searching bibliographic databases effectively. Health policy and planning [online], 13, 339–342. http://heapol.oxfordjournals.org/content/13/3/339.full.pdf+html (http://heapol.oxfordjournals.org/content/13/3/339.full.pdf+html) (Accessed: 1 April 2012 ).
- Fewtrell , L. 2005 . Water, sanitation, and hygiene interventions to reduce diarrhoea in less developed countries: a systematic review and meta-analysis . Lancet infectious diseases , 5 : 42 – 52 .
- Fleiss , J.L. , Levin , B. and Paik , M.C. 2003 . Statistical methods for rates and proportions , 3rd , Chichester : Wiley .
- Gaarder , M. , Glassman , A. and Todd , J. 2010 . Conditional cash transfers and health: unpacking the causal chain . Journal of development effectiveness , 2 ( 1 ) : 6 – 50 .
- Gertler, P., et al., 2011. Impact evaluation in practice [onlilne]. Washington, DC: The World Bank. http://siteresources.worldbank.org/EXTHDOFFICE/Resources/5485726-1283444590513/IEP_001-262_final.pdf (http://siteresources.worldbank.org/EXTHDOFFICE/Resources/5485726-1283444590513/IEP_001-262_final.pdf) (Accessed: 1 April 2012 ).
- Glass , G.V. 1976 . Primary, secondary and meta-analysis of research . Educational researcher , 10 : 3 – 8 .
- Gøtzsche , P.C. 2000 . Why we need a broad perspective on meta-analysis . British medical journal , 321 : 585 – 586 .
- Greenhalgh , T. 2001 . How to read a paper: the basics of evidence based medicine , London : BMJ Books .
- Greenhalgh , T. , Kristjansson , E. and Robinson , V. 2007 . Realist review to understand the efficacy of school feeding programmes . British medical journal , 335 : 858 doi: 10.1136/bmj.39359.525174.AD
- Grimshaw , J. 2003 . Systematic reviews of the effectiveness of quality improvement strategies and programmes . Quality and safety in health care , 12 : 298 – 303 .
- Hammerstrøm, K., Wade, A., and Klint Jørgensen, A.-M., 2010. Searching for studies: a guide to information retrieval for Campbell Systematic Reviews. Campbell systematic reviews [online] 2010 (Supplement 1). www.campbellcollaboration.org/lib/download/969/ (http://www.campbellcollaboration.org/lib/download/969/) (Accessed: 1 April 2012 ).
- Hansen, H., Klejntrup, N., and Andersen, O., 2011. A comparison of model-based and design-based impact evaluations of interventions in developing countries. FOI Working Paper 2011/16 [online]. http://okonomi.foi.dk/workingpapers/WPpdf/WP2011/WP_2011_16_model_vs_design.pdf (http://okonomi.foi.dk/workingpapers/WPpdf/WP2011/WP_2011_16_model_vs_design.pdf) (Accessed: 1 April 2012 ).
- Haynes , R.B. 2005 . Clinical epidemiology: how to do clinical practice research , Philadelphia , PA : Lippincott, Williams, Wilkins .
- Heckman , J. 1998 . Characterizing selection bias using experimental data . Econometrica , 66 ( 5 ) : 1017 – 1098 .
- Hedges , L.V. 1981 . Distribution theory for glass's estimator of effect size and related estimators . Journal of educational statistics , 6 : 107 – 128 .
- Hedges , L.V. and Olkin , I. 1980 . Vote counting in research synthesis . Psychological bulletin , 88 : 359 – 369 .
- Hedges , L.V. and Olkin , I. 1985 . Statistical methods for meta-analysis , Orlando , FL : Academic Press .
- Higgins , J.P.T. 2011 . The cochrane collaboration's tool for assessing risk of bias in randomized trials . British medical journal , 2011 ( 343 ) : d5928
- Higgins, J.P.T. and Green, S., eds., 2011. Cochrane handbook for systematic reviews of interventions. Version 5.1.0 (updated March 2011). The Cochrane Collaboration [online]. http://www.cochrane-handbook.org/ (http://www.cochrane-handbook.org/) (Accessed: 30 January 2012 ).
- Hombrados , J.G. and Waddington , H. 2012 . Internal validity in social experiments and quasi-experiments: an assessment tool for reviewers , Mimeo : 3ie .
- Hunter , P.R. 2009 . House-hold water treatment in developing countries: comparing different intervention types using meta-regressions . Environmental science & technology , 43 ( 23 ) : 8991 – 8997 .
- Hunter , J.E. and Schmidt , F.L. 2004 . Methods of meta-analysis: correcting error and bias in research findings , London : Sage .
- IDCG (Campbell International Development Coordinating Group), 2012. Protocol and review Guidelines, The Campbell Collaboration [online]. http://www.campbellcollaboration.org/artman2/uploads/1/Campbell_International_Development_Group_Protocol_and_Review_Guidelines_Mar2012.pdf (http://www.campbellcollaboration.org/artman2/uploads/1/Campbell_International_Development_Group_Protocol_and_Review_Guidelines_Mar2012.pdf) (Accessed: 1 April 2012 ).
- Jacoby , E. , Cueto , S. and Pollitt , E. 1996 . Benefits of a school breakfast programme among Andean children in Huaraz, Peru . Food and nutrition bulletin , 17 ( 1 ) : 54 – 64 .
- Kabeer , N. , Piza , C. and Taylor , L. 2012 . What are the economic impacts of conditional cash transfer programmes: a systematic review of the evidence , London : EPPI-Centre, Social Science Research Unit, Institute of Education, University of London .
- Keef , S.P. and Roberts , L.A. 2004 . The meta-analysis of partial effect sizes . The British journal of mathematical and statistical psychology , 57 ( 1 ) : 97 – 129 .
- King , E. , Samii , C. and Snilstveit , B. 2011 . Interventions to promote social cohesion in sub-Saharan Africa . Journal of development effectiveness , 2 ( 3 ) : 336 – 370 .
- Koehlmoos , T.P. 2009 . The effect of social franchising on access to and quality of health services in low- and middle-income countries . Cochrane database of systematic reviews , 1 : CD007136 doi: 10.1002/14651858.CD007136.pub2
- Koehlmoos , T. 2011 . Social franchising evaluations: a scoping review , London : EPPI-Centre, Social Science Research Unit, Institute of Education, University of London .
- Kristjansson, E.A., et al., 2006. School feeding for improving the physical and psychosocial health of disadvantaged students. Campbell systematic reviews [online] 2006, 14. doi: 10.4073/csr.2006.14. Available from:[Accessed 1 June 2012]. Also published in Cochrane database of systematic reviews, 2007 (1), CD004676. doi: 10.1002/14651858.CD004676.pub2 http://campbellcollaboration.org/lib/project/23/ (http://campbellcollaboration.org/lib/project/23/)
- Kristjansson, E.A., et al., 2012. Preschool feeding programmes for improving the health of disadvantaged infants and young children (Protocol). Campbell systematic reviews [online]. http://campbellcollaboration.org/lib/project/102/ (http://campbellcollaboration.org/lib/project/102/) (Accessed: 1 June 2012 ).
- Lassi , Z.S. , Haider , B.A. and Bhutta , Z.A. 2012 . Community-based intervention packages for reducing maternal and neonatal morbidity and mortality and improving neonatal outcomes . Journal of development effectiveness , 4 ( 1 ) : 151 – 187 . Shorter version published in Cochrane database of systematic reviews, 11, CD007754. doi: 10.1002/14651858.CD007754.pub2
- Lavis, J., 2009. How can we support the use of systematic reviews in policy making? Plos medicine [online], 6 (11), 1–6. http://www.plosmedicine.org/article/info%3Adoi%2F10.1371%2Fjournal.pmed.1000141 (http://www.plosmedicine.org/article/info%3Adoi%2F10.1371%2Fjournal.pmed.1000141) (Accessed: 30 January 2012 ).
- Leroy , J.L. , Gadsden , P. and Guijarro , M. 2012 . The impact of daycare programs on child health, nutrition and development in developing countries: a systematic review . Journal of development effectiveness , 4 ( 3 ) : 472 – 496 .
- Light , R.J. and Smith , P.V. 1971 . Accumulating evidence: procedures for resolving contradictions among different research studies . Harvard education review , 41 : 429 – 471 .
- Lipsey , M.W. and Wilson , D.B. 2001 . Practical meta-analysis , Thousand Oaks : Sage Publications .
- Littell , J. , Corcoran , J. and Pillai , V. 2008 . Systematic reviews and meta-analysis , New York : Oxford University Press .
- Mallett , R . 2012 . The benefits and challenges of using systematic reviews in international development research . Journal of development effectiveness , 4 ( 3 ) : 445 – 455 .
- Manley, J., Gitter, S., and Slavchevska, V., 2012. How effective are cash transfer programmes at improving nutritional status? A rapid evidence assessment of programmes’ effects on anthropometric outcomes [online]. London: EPPI-Centre, Social Science Research Unit, Institute of Education, University of London. http://www.dfid.gov.uk/R4D/Output/190438/Default.aspx (http://www.dfid.gov.uk/R4D/Output/190438/Default.aspx) (Accessed: 15 July 2012 ).
- Masset , E. 2012 . Effectiveness of agricultural interventions that aim to improve nutritional status of children: systematic review . British medical journal , 2012 ( 344 ) : d8222
- McCoy , S.I. , Kangwenda , R.A. and Padian , N. 2009 . Behavior change interventions to prevent HIV infection among women living in low and middle income countries: a systematic review . AIDS and behavior , 14 ( 3 ) : 469 – 482 .
- Moher , D. 2009 . Preferred reporting items for systematic reviews and meta-analyses: the PRISMA statement . PLoS medicine , 6 ( 6 ) : e1000097 doi: 10.1371/journal.pmed1000097
- National Institute for Health and Clinical Excellence (NICE), 2005. Guideline development methods: information for National Collaborating Centres and guideline developers [online]. London: National Institute for Clinical Excellence. www.nice.org.uk/niceMedia/pdf/GDM_Allchapters_0305.pdf (http://www.nice.org.uk/niceMedia/pdf/GDM_Allchapters_0305.pdf) (Accessed: 1 May 2012 ).
- NICE, 2009. Quality appraisal checklist – quantitative intervention studies. In: Methods for the development of NICE public health guidance [online]. http://www.nice.org.uk/media/2FB/53/PHMethodsManual110509.pdf (http://www.nice.org.uk/media/2FB/53/PHMethodsManual110509.pdf) (Accessed: 30 September 2011 ).
- Oxman , A.D. 2009 . SUPPORT Tools for evidence-informed health policymaking (STP) 10: taking equity into consideration when assessing the findings of a systematic review . Health research policy and systems , 7 ( Suppl. 1 ) : S10 doi: 10.1186/1478-4505–7–S1–S10
- Petrosino, A., et al., 2012. The effects of K-12 school enrolment policies in developing nations. Campbell systematic reviews [online]. The Campbell Collaboration. http://campbellcollaboration.org/lib/project/123/ (http://campbellcollaboration.org/lib/project/123/) (Accessed: 1 June 2012 ).
- Petticrew , M. and Roberts , H. 2006 . Systematic reviews in the social sciences: a practical guide , Oxford : Blackwell Publishing .
- Pitt , M.M. and Khandker , S.R. 1998 . The impact of group-based credit programs on poor households in Bangladesh: does the gender of participants matter? . Journal of Political Economy , 106 ( 5 ) : 958 – 996 .
- Ravallion , M. 2008 . “ Evaluating anti-poverty programs ” . In Handbook of development economics , Edited by: Schultz , T. Paul and Strauss , J. Vol. 4 , Amsterdam : North-Holland .
- Riley , R. , Higgins , J. and Deeks , J. 2011 . Interpretation of random effects meta-analysis . British medical journal , 342 : 549
- Rogers , E.M. 2005 . Diffusion of innovations , 5th , New York : The Free Press .
- Rubin , D. 1974 . Estimating causal effects of treatments in randomized and nonrandomized studies . Journal of educational psychology , 66 ( 5 ) : 688 – 701 .
- Sandieson , R. 2006 . Pathfinding in the research forest: the pearl harvesting method for effective information retrieval . Education and training in development disabilities , 41 ( 4 ) : 401 – 409 .
- Scriven , M. 2008 . A summative evaluation of RCT methodology: & an alternative approach to causal research . Journal of multidisciplinary evaluation , 5 ( 9 ) : 11 – 24 .
- Shadish , W. , Clark , M. and Steiner , P. 2008 . Can nonrandomised experiments yield accurate answers? A randomized experiment comparing random and nonrandom assignments . Journal of the American statistical association , 103 ( 484 ) : 1334 – 1344 .
- Shadish , W. , Cook , T. and Campbell , D. 2002 . Experimental and quasi-experimental designs for generalized causal inference , Boston : BROOKS/COLE CENGAGE Learning .
- Snilstveit , B. 2012 . Systematic reviews: From ‘bare bones’ reviews to greater policy relevance . Journal of development effectiveness , 4 ( 3 ) : 388 – 408 .
- Snilstveit , B. , Oliver , S. and Vojtkova , M. 2012 . Narrative approaches to systematic review and synthesis of evidence for international development policy and practice . Journal of development effectiveness , 4 ( 3 ) : 409 – 429 .
- Stewart, R., et al., 2010. What is the impact of microfinance on poor people? A systematic review of evidence from sub-Saharan Africa. Technical report [online]. London: EPPI-Centre, Social Science Research Unit, University of London. http://eppi.ioe.ac.uk/cms/Default.aspx?tabid=2965 (http://eppi.ioe.ac.uk/cms/Default.aspx?tabid=2965) (Accessed: 30 January 2012 ).
- Stewart , R. , van Rooyen , C. and de Wet , T. 2012 . Purity or pragmatism? Reflecting on the use of systematic review methodology in development . Journal of development effectiveness , 4 ( 3 ) : 430 – 444 .
- Vaessen, J., et al., 2012. The effects of microcredit on women's control over household spending in developing countries. Campbell systematic reviews [online]. http://campbellcollaboration.org/lib/project/178/ (http://campbellcollaboration.org/lib/project/178/) (Accessed: 1 June 2012 ).
- Valentine , J. and Cooper , H. 2008 . A systematic and transparent approach for assessing the methodological quality of intervention effectiveness research: the study design and implementation assessment device . Psychological methods , 13 ( 2 ) : 130 – 149 .
- Vermeersch, C. and Kremer, M., 2004. School meals, educational achievement, and school competition: evidence from a randomized evaluation. World Bank Policy Research Working Paper No. 3523 [online]. Available from:or http://dx.doi.org/10.2139/ssrn.667881 http://ssrn.com/abstract=667881 (http://ssrn.com/abstract=667881) (Accessed: 15 June 2012 ).
- Waddington , H. and Snilstveit , B. 2009 . Effectiveness and sustainability of water, sanitation, and hygiene interventions in combating diarrhoea . Journal of development effectiveness , 1 ( 3 ) : 295 – 335 .
- Waddington, H., et al., 2012. Farmer field schools for improving farming practices and farmer outcomes in low- and middle-income countries: a systematic review. Campbell systematic reviews [online]. http://campbellcollaboration.org/lib/project/203/ (http://campbellcollaboration.org/lib/project/203/)
- Wang , S. , Moss , J.R. and Hiller , J.E. 2006 . Applicability and transferability of interventions in evidence-based public health . Health promotion international , 21 ( 1 ) : 76 – 83 . doi: 10.1093/heapro/dai025
- White , H. 2008 . What works in water supply and sanitation: lessons from impact evaluation , Washington, DC : Independent Evaluation Group (IEG), World Bank .
- White, H. and Phillips, D., 2012. Addressing attribution of cause and effect in small n impact evaluations: towards an integrated framework. Working Paper 15, International Initiative for Impact Evaluation [online]. New Delhi: 3ie. http://www.3ieimpact.org/media/filer/2012/06/29/working_paper_15.pdf (http://www.3ieimpact.org/media/filer/2012/06/29/working_paper_15.pdf) (Accessed: 15 July 2012 ).
- Yunus, M., 2005. Eliminating poverty through market-based social entrepreneurship. Global Urban Development Magazine [online], 1 (1). http://www.globalurban.org/Issue1PIMag05/Yunus%20article.htm (http://www.globalurban.org/Issue1PIMag05/Yunus%20article.htm) (Accessed: 30 January 2012 ).