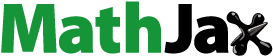
ABSTRACT
The paper reports on an ex-ante evaluation of the nationwide scale up of two pilot cash transfer programmes in Uganda. We use panel data to estimate consumption elasticities of child health status and school enrolment. They provide the main parameters of a micro-simulation model predicting cash transfer effects on human capital accumulation and feedback effects on consumption. Quantifying year on year costs and direct and indirect benefits, we track the annual trend in rates of return. The findings indicate important gains in child health, schooling, and income growth associated with programme participation. The rates of return improve over the medium term but remain negative after 10 years. These findings underline the need to regard cash transfers as longer-term social investment instead of short-term costs.
1. Introduction
A wide array of experimental and non-experimental evidence shows that improved education and health investments can lead to positive long-term outcomes (Glewwe and Kremer Citation2006; Glewwe and Miguel Citation2007; Psacharopoulos Citation1985, Citation1994; Psacharopoulos and Patrinos Citation2004; Strauss and Thomas Citation2007; Peet, Fink, and Fawzi Citation2015). In addition to the intrinsic values of health and education, the economic value gained by encouraging investments in human capital is potentially large. Yet, liquidity constraints can hinder the human capital investments of poor households (Kremer, Brannen, and Glennerster Citation2013). Social cash transfer programmes are now widely used to alleviate the financial burden that constrains households in their decision to invest in children’s human capital.
Unconditional cash transfers allow recipients to boost investments in human and physical capital, to smooth consumption and to engage in more risky but productive activities (Fiszbein et al. Citation2009). The existing evidence focuses on the positive impact of these programmes on human capital, especially of younger individuals. Numerous impact evaluation studies have carefully shown that cash transfers to vulnerable households can have large effects on the education and health outcomes of children in beneficiary households (Haushofer and Shapiro Citation2016; Kremer, Brannen, and Glennerster Citation2013). Case study evidence from Uganda is no exception to this. A recent evaluation indicated that the two cash transfer programmes considered in this paper enhanced household welfare. Beneficiary households reported higher consumption expenditures and used part of the transfers on health and education related expenditures and investments in productive assets (Merttens et al. Citation2016).
However, despite promising evidence on short-term impacts on a broad range of outcomes, we know little about the evolution of effects of cash transfers in the medium- and long-term. To date, evaluation of the longer term effects of small-scale programmes providing low-level benefits in Sub Saharan Africa is missing. In this paper, we simulate the ex-ante year-on-year returns to two cash transfer programs in Uganda’s by applying a cost-benefit framework.
In 2010 the government of Uganda decided to establish a national social protection system with the goal of reducing chronic poverty and improving the life chances of poor men, women and children. As one of its main pillars this included the Social Assistance Grant for Empowerment (SAGE). Two design options were piloted between 2011 and 2015 to test their impacts and cost-effectiveness in achieving the SAGE goals to tackle chronic poverty and to grant access to key services such as healthcare and education. The design options include the Senior Citizen Grant (SCG), a universal social pension targeted at persons aged 65 and above, and the Vulnerable Family Grant (VFG), which is targeted at vulnerable households with restricted access to the labour market and high dependency ratios. Both programs were piloted in different regions and there was no overlap in programme participation. While the design options differ fundamentally, they share the same SAGE outcome objectives.
In this paper, we report on an ex ante assessment of the returns to education from household investments in education and child health following the national rollout of the SCG and the VGF, based on a micro-simulation approach. Methodologically, our approach is in line with dynamic discrete choice models (Todd and Wolpin Citation2010), in which we model behavioural responses to cash benefits in terms of child health and education investments and the returns to programme induced human capital investments. Similar approaches have been used to estimate education choices in Mexico with PROGRESA (Attanasio, Meghir, and Santiago Citation2011; Todd and Wolpin Citation2008) and for hypothetical transfer programmes in Cambodia (Mideros, Gassmann, and Mohnen Citation2016).
As we are relying on non-experimental data, identification of programme effects and modelling behavioural responses to programmes is challenging. Yet, we do not aim to present exact point estimates of programme returns. Instead, we seek to illustrate the year-by-year evolution of returns through human capital investments and to assess whether these indirect returns matter in a cost benefit analysis.
Hence, our paper makes the following contributions: first, we apply a longer-term perspective and assess the expected programme returns over a period of 10 years using a dynamic micro-simulation model; second, applying a cost-benefit framework, we test whether and when the monetary returns to human capital investments exceed the operational costs of these programmes; third, the paper contributes to the evidence in East Africa by studying the case of Uganda using nationally representative data instead of focussing on pilot regions.
The remainder of the paper is structured as follows: the background section reviews the existing literature and describes the social transfer programmes of interest, followed by a discussion of the analytical framework, the micro-simulation model and the data. Thereafter, we discuss the semi-elasticities and present the micro-simulation results. In the last sections, we discuss the model limitations followed by concluding remarks.
2. Background
Cash transfer programs raise current consumption levels, but they also strive for breaking the intergenerational transmission of poverty (Barrientos Citation2013). This can be achieved by increasing investments of households in both human and physical capital that will lead to higher productivity and labour market outcomes.
Despite a growing body of evidence on the effects of cash transfer programs (Bastagli et al. Citation2018) few studies focus on the long-term effects of these programs (defined as 10 years or more) (Molina-Millán et al. Citation2019). This is in part because the expansion of these programmes is very recent, but also because experimental studies are normally designed with a short-term window. The handful of studies available focus on older programs in Latin America such as in Honduras (Ham and Michelson Citation2018), Mexico (Parker and Vogl Citation2018; Fernald, Gertler, and Neufeld Citation2009), Nicaragua (Barham, Macours, and Maluccio Citation2013), Ecuador, and Colombia (García et al. Citation2012). Moreover, these long-term evaluations based on experimental methods suffer from high attrition rates that significantly decrease both the internal and external validity (Molina-Millán et al. Citation2019).
Two recent papers present mid-term results of cash transfers in the East-Africa region. Handa et al. (Citation2018) find positive consumption impacts of Zambia’s Child Grant Programme three years after its implementation. On average consumption increases, exceed the amount of transfers received by 67%, thereby confirming the presence of strong multiplier effects. Examining an unconditional cash transfer programme in Malawi two years after the program had stopped, Baird, McIntosh, and Ozler (Citation2016) find positive impacts on anthropometric indicators of children of adolescent beneficiaries.
To assess the potential long-term effects of these programs, simulations and structural models offer the best solution where simulations also allow to test different scenarios and policy designs (Bourguignon and Spadaro Citation2006; Todd and Wolpin Citation2010). Modelling both costs and benefits for different designs, simulations help policy makers to estimate whether a policy is worth implementing. This technique can be used at both the planning stage of programs but also in their scaling-up phase. For example Bourguignon et al. (Citation2003) simulated the effects of Bolsa Escola in Brazil but just for one period. In a similar fashion, Kakwani, Soares, and Son (Citation2006) look at simulations for a set for African countries, but considering just the short-term direct effects of an income transfer. Filipski and Taylor (Citation2012) look at the case of Ghana and Malawi on production and welfare. Long-term simulations include the article by Mideros, Gassmann, and Mohnen (Citation2016) for the case of Cambodia, and Peruffo and Ferreira (Citation2017) for the case of Brazil. Our study does this for the context of Uganda. This is a novelty for Uganda and Sub Saharan Africa as a whole. The only other comparable long-term study for the region by Brent (Citation2013) does not, in fact, include a micro simulation, but just modelling using parameters from existing studies.
Uganda, a landlocked low-income country in sub-Saharan Africa, has made notable progress in terms of poverty reduction. Between 2005 and 2012 poverty rates decreased from 31.1 percent to 19.7 percent (Etang Ndip and Tsimpo Citation2017). The Expanding Social Protection Program (ESP) was set up to establish a national social protection system as a core element in the national planning and budgeting process. The ESP was approved by the Cabinet in 2010 on a pilot basis and initially funded by international development partners. Its flagship programme SAGE consisted initially of two types of social transfers: the Senior Citizens Grant (SCG) and the Vulnerable Family Grant (VFG). Both provide bi-monthly cash transfers of 50.000 Ugandan Schilling, about 15% of the average consumption per capita according to UNPS data, to their beneficiaries.
The SCG was targeted to all people above the age of 64Footnote1. According to ESP costing scenarios for a national scale-up of the SCG, the administrative programme costs for a national implementation are estimated to be 7.1% of the transfer payments. The VFG was designed to target poor and vulnerable households with restricted labour capacities and high dependency ratios using the same transfer values and frequencies as the SCG. A set of indicators was used to develop an eligibility score that ranks households according to their approximated vulnerability.Footnote2 The highest scoring 15% of households in each district were entitled to receive the cash transfer. If present in a beneficiary household, adult women were selected to be the actual recipients of the transfers. The administrative costs are estimated at around 22.8% of the programme payments for the case of a national roll out of the programme (Merttens et al. Citation2016). The government of Uganda has recently announced that the VFG will be discontinued after the pilot phase, while the SCG will be scaled up reaching an additional five districts per year covering 55 districts by the end of the fiscal year 2019/20.
3. Analytical framework
Our framework connects household decisions regarding child health and schooling. We follow a simple model developed by Glewwe and Miguel (Citation2007): there are two time periods denoted by the subscripts 1 and 2 that refer to pre-school age and school age of a child. Skills obtained in school at the end of the second period are a function of child health H in the first and second period, parent’s educational investments EI (monetary and time), child’s innate ability α, school characteristics SC and years of schooling YS in period 2. The subscripts pr refer to production functions.
In this structural skills production function, all variables have a positive effect on school outcomes in period 2. For example, child health in the first period has a positive effect on schooling outcomes in period 2 holding everything else constant. Parents’ utility is a function of consumption C in period one and two, child health in both periods, and child’s school attainment in period two.
Parents maximise their utility function conditional to a budget constraint (function of inputs, prices p and the discount rate r), the skills production function
, and child health
in period 1 and 2, which is a function of medical inputs M, the local health environment HE, and the innate health predisposition
. The subscript C refers to consumption of children.
Based on the maximisation problem, the demand functions for the endogenous variables can be derived and the demand for child’s academic skills as a function of households’ budget, environment, and tastes can be formulated as follows:Footnote3
Where and
describe parental general tastes for child health and schooling. Equation 6 suggests that a small transfer, such as SAGE transfers, has a positive income effect on skills obtained in period 2 if the budget constraint is binding. First, transfers raise the demand for educational inputs, which has a positive effect on
Secondly, transfers increase the demand for medical inputs which has a positive effect on
and
and thus a positive effect on
through improved child health.
Following the analytical framework cash transfers are expected to increase the demand for child health inputs and the demand for schooling (directly and indirectly through improved child health). We assume that transfers are equally distributed among household members so that a transfer to elderly household members benefits the whole household. However, the effects depend on whether the benefits are allocated in period one or two. In period two, parents cannot go back and change their investment decisions in child health. In this case, the short-term effect of SAGE on schooling works only through the education investments effect and the immediate effect of child health in period two on schooling decisions. Because of data constraints (discussed in the Data section), we only consider the programme effects on child health before children enter school (period 1) and not during their time in school (period 2). This implies that programmes require several years to unfold their full potential through child health in period one and the immediate education investments in period two. Therefore, we simulate the SAGE effects over a period of 10 years to also account for medium-term programme effects. Yet, even 10 years may still be too short-termed to see the complete programme effects through human capital investments realised.
Increased school attainment induced by SAGE transfers further increase household incomes through higher earnings from improved education. The conventional return to education suggests that more educated individuals earn higher wages as they become more productive. However, positive returns to education have also been reported for self-employed farm and non-farm earnings, which is important in a context of a large informal sector in Uganda (Appleton Citation2001; Jolliffe Citation2004). We refer to direct programme effects as those induced directly by SAGE cash transfers and indirect effects as those generated by programme induced investments in child health, schooling and returns to schooling.
4. Micro-simulation
We simulate the effects as outlined in the conceptual framework over 10 years on the national level separately for each programme. That means that we simulate the effects not only in the pilot regions where the SCG and VFG are or have been implemented, but for the hypothetical case of a countrywide implementation. The advantage of the simulation approach is that it facilitates an ex-ante assessment of the programme returns looking beyond the effects found in the pilot regions and over a longer period of time, which would not be possible with an ex-post approach. Yet, simulations are a simplification of reality that hinge on assumptions and the results need to be interpreted in light of these assumptions. Thus, the aim of this exercise is not to present point estimates of the programme returns, but to present an assessment of the scale of returns that could be generated through programme induced human capital investments.
The dynamic simulation model incorporates the average child health and education responses and returns to education. In the first step, we quantify the relationships between household incomes, child health, education and returns to education, which then feed the micro-simulation model in the second step. That is, based on the estimates we predict how an increase in household incomes through SAGE affects child health and schooling indicators. We must rely on non-experimental methods to quantify the coefficients, but it should be noted that the internal validity of the estimation models is not a pre-condition for accurate predictions. To validate the elasticities, we compare the coefficients to existing evidence. The details of the regression analysis are presented in Section 6.
Based on the VFG and SCG eligibility criteria, we simulate two separate scenarios assigning programme transfers either to individuals above age 64 (SCG) or to the top 15% in each district in terms of the eligibility score (VFG). Therefore, we assume that the programme benefits are strictly allocated following the targeting formula and are completely taken up. The programme transfers are equally distributed among household members.Footnote4 As both SAGE design options target different populations, we test whether income changes are then associated with different responses to health and education investments that would need to be accounted for in the simulation model; but we do not find statistically significant differences (see Section 6).
The simulation procedures are the same in each period as outlined in Figure A1 in the technical Annexe. First, eligible households receive the programme transfers, which increase their disposable income. Based on that, the model predicts pre-school children’s health, the likelihood of school enrolment, and the likelihood of school continuation (separately for primary, secondary, and tertiary school age). Subsequently, the educational attainment is updated if individuals completed an additional year of schooling. This allows us to assess how an increase in income could affect our education and child health indicators. In the third step, we simulate the returns to higher education attainments according to the estimates discussed in Section 6. Programme induced changes in educational outcomes are compared to a control scenario without the programme transfers. In that regard, the indirect benefits can be formulated as follows:
Where refers to the expected outcomes of the program and
to the expected outcomes in the absence of the programmes. The new income levels are calculated as the sum of the previous income level plus the direct effect (transfers) and the indirect benefits (returns to education). The difference in income in a scenario with and without the programmes reflects the overall benefits
of the programmes in our model.
In the last step, the micro-simulation includes a demographic module accounting for demographic changes over time. We incorporate WHO mortality rate projections by age and sex to probabilistically determine deaths and new-borns of women in each period. The simulated population increases at an average rate of 2.8% per period, which is close to the observed annual population growth of around 3% in the last 5 years. The demographic development in the model is the same for control and programme scenarios, as the data does not allow estimating mortality and fertility rates as a function of incomes. The demographic module ensures that individual eligibility is adjusted within the simulation horizon. At the end of each period, the demographic changes are realised. In the next period households need to qualify again for the programme transfers and only the educational benefits are carried over to the next period’s income. This procedure is performed for 10 periods.Footnote5
Similar to the programme benefits, the costs can also be separated into direct and indirect sources. The direct costs refer to programme transfers and operational costs. The costs of sending boys and girls to school (fees, uniforms etc.) are implicitly incorporated in increased household expenditures. From a policy maker’s perspective there could be indirect costs associated with the public provision of education, or, given a fixed education budget, a decrease in the quality of (and returns to) education for example due to increasing class sizes. In addition, there could be distortionary cost of raising money to finance the provision of additional schooling, which could further increase the costs for the public. These indirect costs are important, but difficult to quantify. We only consider the programme related operational and administrative costs, something that needs to be born in mind in the interpretation of the results.
5. Data
We use the Uganda National Panel Survey (UNPS), a nationally representative panel household survey. The sampling was based on the 2005 Socio-Economic and Community Survey. As the questionnaire design has changed and several key variables were not collected in 2005, our analysis uses the four waves from 2009 to 2013. In total, the sample includes observations of 82,718 individuals. For the analysis, we focus on households with at least two observations over time.Footnote6
5.1. Descriptive statistics
presents the descriptive statistics of the main outcome variables. The income variable is at the core of our analysis. However, the income reports seemed incomplete, with a considerable number of negative incomes.Footnote7 This is at least partly related to missing observations and changes in the questionnaire design across waves. Therefore, we decided to use consumption to approximate household incomes. As households do not necessarily consume the complete transfers, we apply a propensity to consume of 80% in the main results following Angelucci and Attanasio (Citation2009).Footnote8 Household consumption aggregates at 2005 prices are provided in the data set. The average monthly consumption per capita in 2005 prices summed up to around 37.000 UGS (approximately 47 US$). Crop farming was the main income source followed by incomes from non-agricultural enterprises, livestock and wage earnings and other household incomes (transfers, rents etc.). The data shows that real household consumption was on average highest in 2009 and dropped markedly in 2010/11 and 2011/12.
We are interested in the programme effects on household education investments. School enrolment is often erratic and the line between drop-outs and low attendance is blurred (Glewwe and Kremer Citation2006). As the frequency of school participation is not covered in the data, we use a binary variable that describes whether household members are currently enrolled in school and the grade they are attending. However, the panel structure of the data allows us to analyse how changes in income affect school dropouts. School enrolment rates of school age children and youths (6–24) remained relatively constant at about 77% on average in all waves with the lowest enrolment rates reported in 2013. Despite that, school attainment of adults above 16 with completed schooling was highest in 2013 with an average of 6 years of schooling, nearly half a year higher than in 2009.
Besides education, we consider the indirect effects of the programme on child health. As we are interested in the proximate effect of an income increase on child health, we use a binary variable for child underweight classified as a weight-for-age z-score below −2. Weight-for-age is a more contemporaneous measure of the nutritional status and better suited to capture short-term changes in income than height-for-age, which covers the aggregate of health investments over a longer period (Duflo Citation2000, Citation2003; Haddad et al. Citation2003; Strauss and Thomas Citation2007).Footnote9 We regard the likelihood of underweight in the simulation to approximate severe health problems. The data set includes weight and height information for about 63% of all children up to age 4 in the sample. shows that child underweight at age 4 was about 12% on average and fell from 14% in 2009 to 10% in 2013.
Table 1. Descriptive statistics of the main outcome variables.
With regards to demographics, the Ugandan population is relatively young, with an average age of 21 years. The typical household is composed primarily of school age children and youths (6–24) where 85% of households with an old age person also include school age family members, while 57% include children under 6. Looking at the other side of the relationship, 44% of households with school age individuals and 39% of households with children also include an old age member.Footnote10 Given the intra-household sharing of income, old-age transfers like the SCG also reach younger individuals.
6. Elasticities
In order to estimate the coefficients for the micro-simulation model, the relations between income, child health and education need to be quantified. Therefore, we estimate the effect of an increase in income on child health, school enrolment and school continuation. As both SAGE design options target different populations, we also tested whether the semi-elasticities are different for households with an elderly (above 65) or a female head. We also test if household with members above 65. That is, we analysed whether changes in incomes of households with potential SCG beneficiaries are associated with different responses, compared to VFG ones, to health and education investments that would need to be accounted for in the simulation model. The estimation results of these models are presented in Table A9, A10 and A11 in the Technical Annexe. In none of the models we find statistically significant interaction effects, which is why we use the same semi-elasticities in the simulation of both programmes. Note, however, that in all predictions we condition on a range of socio-demographic variables. Lastly, in the simulations we don’t model program related changes in intra household decision making and refer to the Limitations section for a discussion of possible implications.
6.1. Indirect effects: child health
To quantify the effect of income on child health, we regress a binary variable underweight on the logarithm of consumption per capita
and a set of additional regressors
.Footnote11 The selection of control variables mainly follows a study by Haddad et al. (Citation2003) in which the authors analyse income effects on pre-school Weight-for-Age z-scores in 12 developing countries. As we are interested in the short-run effect of incomes on child health, we control for the local health infrastructure and the household specific sanitation endowment.Footnote12 In addition, we control for household characteristics including the level of education of the household head, size and composition of the household and individual characteristics including age, sex, and orphan-hood status and include regional and time fixed effects, which can be presented in a simplified form as follows:
In the presented models we specify linear models as we are mainly interested in the marginal effects (Angrist and Pischke Citation2008) and because of the difficulties with binomial two stage least square (2SLS) random effects models.Footnote13 In the second step, we estimate individual fixed effects models that avoid unobserved heterogeneity bias through genetic predisposition and other time invariant factors. Lastly, we use 2SLS models to account for potential measurement error of consumption reports and the potential simultaneity of time allocated to income generating activities and childcare. Therefore, we instrument household consumption using various household assets and durable goods as instruments for consumption. Similar instruments have been used in the literature as their prevalence is correlated with household consumption, but they have no direct bearing on child health (Haddad et al. Citation2003; Mideros, Gassmann, and Mohnen Citation2016).
The estimated consumption semi-elasticities are displayed in . For clarity, we only present the coefficients of interest in the main text and present the complete estimation tables including all control variables and the first stage of the 2SLS in a separate technical Annexe. The results show a negative effect of consumption on the likelihood of underweight of children between age 1 and 4 in the random effects models. The consumption coefficient of the fixed effects model is not significant. The exogeneity of consumption is rejected by the Wald test of exogeneity for the random effects model but not for the fixed effects model. With or without accounting for the endogeneity of consumption, estimating a fixed effects model leads to a change in sign and a non-significant consumption coefficient. The F-test of the first stage estimation is slightly below the 10% critical Stock-Yogo value, which could lead to a poor performance of the estimator. Therefore, we use the specification of column 3 for the micro-simulation model.
Table 2. Consumption effect on child underweight (age 1–4).
The coefficient suggests that a 10% increase in consumption decreases the likelihood of child underweight by 1 percentage point (pp). Our estimate seems to be in line with the mean coefficients of all 12 countries analysed in Haddad et al. (Citation2003). Their results for Sub Saharan Africa include Kenya, South Africa, and Mozambique indicating a (large) income elasticity ranging from 0.08 in South Africa to 0.41 in Kenya when estimating by 2SLS, but using weight-for-age z-scores instead of underweight. Similarly, Bengtsson (Citation2010) finds that in Tanzania child weight, particularly of female children, is sensitive to income changes. The authors find that a 10% decrease in income result in a 0.5 kg decrease in the body weight of females up to age 4.
6.2. Indirect effects: schooling
Following our research framework, we measure the quantity of education as completed years of schooling. In the first step, we estimate the effect of household consumption and underweight at age 4 (
) on the likelihood of school enrolment (
. Therefore, we explore the panel data to test how underweight at age 4 affected the school enrolment in the following data waves. Given that our data covers a time span from 2009 to 2013, we estimate the likelihood of school enrolment up to age 8. However, modelling the link between health and enrolment is complex and there are many confounders that affect education and child health investments. As underweight at age 4 is determined before school enrolment decisions are taken, there is less of an identification problem here (Glewwe and Jacoby (Citation1994). We use proxy variables to control for confounders of school enrolment (
) as far as the data allows. Therefore, we include the education level of the household head, access to schools in the community, as well as household composition, time and regional fixed effects and other household characteristics. As underweight at age 4 is time invariant, we are not able to estimate the effects of underweight at age 4 with a fixed effects model. As in the previous models, we furthermore present 2SLS models instrumenting household consumption using land ownership and household’s durables.
After estimating the income and child health effects on school enrolment, we estimate how income affects school continuation. That means we test how changes in income affect the likelihood of school dropouts. Other than the estimation of school enrolment, we do not account for child health effects as information on underweight at age 4 is not available for older children and the panel structure is not longitudinal enough to capture the health effects on higher education levels. Yet, in the simulations child health is implicitly – though only partially – incorporated through the effect on first time school enrolment, which affects subsequent schooling decisions. For the estimations, we use a similar approach as outlined in Equation (3) separately for primary, secondary, and tertiary education ages. However, estimating the likelihood of school continuation is subject to a number of challenges. Simultaneity arises if school enrolment is a substitute for child work, which could lead to a downward bias of the estimated coefficient (Grimm Citation2011). Typical concerns with unobserved heterogeneity include child’s ability and a time variant correlation of household incomes and opportunities to obtain a job that requires a certain degree of education. Therefore, we additionally estimate individual fixed effects models and 2SLS models using household assets and durables as instruments for consumption as described in the previous section.
The estimated effects of income and underweight on school enrolment are displayed in . Being underweight at age 4 was associated with a 14 pp decrease in the likelihood of school enrolment between age 5 and 8. At the same time, we find no effect of consumption on the likelihood of school enrolment. Based on the applied instrumental variables the exogeneity of consumption is not rejected by the Wald test of exogeneity. We thus use specification 1 for the simulation model. These results are in line with a study on the effect of child health on delayed enrolment in Ghana finding that lower Height-for-Age z-scores increased delayed enrolment significantly whereas household income had no effect on delayed enrolment (Glewwe and Jacoby Citation1994).
Table 3. Consumption and underweight effect on school enrolment (age 5–8).
In , we present the consumption effects on school continuation of previously enrolled children. The baseline random effects model shows a positive and significant effect of consumption on school continuation. The results hold when estimating individual fixed effects models and for the sub-samples of primary, secondary, and tertiary age individuals. 2SLS models do not indicate any significant consumption effects on school continuation; Wald tests of exogeneity do not reject the exogeneity of consumption based on the applied instrumental variables (see column 5–7), which is why we use the OLS specifications of columns 2 to 4 for the simulations.Footnote14
Table 4. Consumption effect on school continuation (age 6–24).
The results imply that an increase of 10% in household consumption increases the probability to stay in school by 0.1 pp between the age of 5 and 12, by 0.2 pp between the age of 13 and 18, by 0.6 pp between the age of 19 and 24. The relatively small effect of consumption on school continuation could be related to the introduction of free primary education in Uganda in 1997. Deininger (Citation2003) showed that free primary education reduced the effect of household incomes on enrolment decisions significantly and balanced the strong gender bias towards male enrolment rates. The success of public education policies could explain why the consumption coefficients are small compared to findings in other countries (Grimm Citation2011; Kremer, Brannen, and Glennerster Citation2013; Mideros, Gassmann, and Mohnen Citation2016). However, this would also imply that there is less scope for the cash transfer programmes to increase education outcomes in Uganda.
6.3. Returns to education
Increased school enrolment and improved child health lead to higher educational attainment, which eventually generates returns to education. The conventional pathway suggests that individuals that are more educated earn higher wages as they become more productive. Non-experimental studies typically use the Mincerian framework (Appleton Citation2001; Card Citation2001; Girma and Kedir Citation2005; Kingdon and Soderbom Citation2007; Psacharopoulos and Patrinos Citation2004) where log wages are regressed on years of schooling ( and work experience
:
However, there are several problems encountered in estimating returns to education in Uganda; firstly, only a relatively small fraction of households receives a wage salary (about 14% of working age adults reported wages from employed work). Positive returns to education have also been reported for self-employed farm and non-farm earnings (Appleton Citation2001; Jolliffe Citation2004). We use consumption as approximation for income and thus consider all forms of household incomes. Therefore, we regard the allocative effect of education on consumption and estimate the effect of the maximum level of school attainment in the household on consumption.Footnote15
Secondly, endogeneity problems could lead to biased coefficients. This could for example come from workers’ unobserved ability systematically correlated with both years of education and the dependent earnings, or systematic measurement error in consumption reports. Several scholars have dealt with endogeneity problems by estimating returns to education using two-stage least squares models (Card Citation2001; Kerr and Quinn Citation2010; Leyaro, Morrissey, and Owens Citation2010; Rankin, Sandefur, and Teal Citation2010). We follow this approach using access to primary and secondary schools on the community level as instruments for educational attainment as has been suggested in the literature (Glewwe and Miguel Citation2007). Restricted access to schools increases the cost of schooling, which is expected to be negatively related to school attainment. On the other hand, the accessibility of schools is expected to have no direct effect on incomes, qualifying it as instrument for the maximum and mean level of schooling in the household. In we show the estimated coefficients of the maximum school attainment in households on log consumption.
Table 5. Effect of school attainment on HH consumption.
The results of the random effects models indicate that an additional year of the maximum level of schooling is associated with a 5% increase in consumption. The effect more than doubles if we estimate the model by 2SLS with access to primary and secondary schools in the community as instruments for school attainment.Footnote16 Wald tests of exogeneity suggest that maximum school attainment should be treated as endogenous in our specification. In the fixed effects estimations the coefficients are still positive but drop markedly. As in previous estimations, our instruments vary little over time. The F-statistic of the first stage estimation with fixed effects is very low (2.19) indicating that the estimation suffers from weak identification. Therefore, we prefer the specification of column 2. As robustness check, we estimate the effect of school attainment on individual wages (column 5) with a Heckman selection model, which suggests an increase of 17% in hourly wages for an additional year of education, which is larger compared to our preferred model. This is not surprising as returns to education have been found to be lower for farm earnings than for employed work (Appleton Citation2001; Jolliffe Citation2004). Earlier estimates on the returns to education in Uganda found that four years of primary education increase the agricultural production by about 7%)and that wages by about 7%-8% for each additional year of schooling (Appleton Citation2001).
7. Micro-simulation results
We present the simulation results as relative changes of VFG and SCG, respectively, compared with the control scenario without any transfers (see Equation 7). To calibrate the model, we use data from 2011, to avoid any contamination of the simulations by the ‘real’ pilot study that was rolled-out in 2013. We fit the simulated data of the programme effects per period using local mean smoothing techniques. We use Nadaraya–Watson Epanechnikov kernel estimators with a rule of thumb bandwidth of 1.5 to fit the outcome variables per period. As we only observe outcomes once per period, we select a bandwidth larger than the optimal bandwidth minimising the mean integrated squared error (MISE) using least square cross validation.Footnote17 Furthermore, we present confidence intervals for the individual or household level variation in outcomes.
7.1. Child health
shows the simulated programme effects on child underweight at age 4. For the VFG, the likelihood of child underweight decreases along the 10 periods compared to the control scenario without transfers. The SCG also reduces the likelihood of child underweight, but the effect diminishes over time. After 10 periods, the effects of both programmes converge to an annual reduction in child underweight of 0.2 pp. The initial differences between SCG and VFG are mainly driven by different programme sizes. While about 60% of households have at least one member above the age of 65 and would thus receive SCG, by definition only 15% of households would be assigned VFG transfers. The diminishing effect of SCG over time can be explained with demographic changes. In the simulations, the share of households with individuals over 65 and children of age 4 is decreasing over time. Disregarding the demographic module leads to a slightly decreasing likelihood of child underweight over time.
7.2. Education
shows the simulated programme effects on school enrolment of children between age 5 and 8 and the subsequent likelihood of school continuation for the age bracket between 6 and 24. The effect is largest for the SCG and increases over time. However, after 10 periods the likelihood of first time school enrolment only increases by slightly less than 0.02 pp compared with the control scenario. As Uganda has introduced free primary education for many years now, the results could reflect that there is little scope for the programmes to further increase school enrolment rates.
Figure 2. Programme effects on school enrolment (6–9; left) and school continuation (age 6–24; right). (local mean smoothing with bandwidth of 1.5).
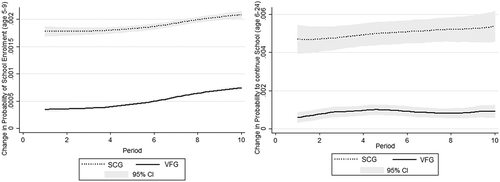
The effects on school continuation of all previously enrolled children and youth between age 6 and 24 range between 0.5 pp (SCG) and 0.1 pp (VFG) after 10 periods. The effects marginally increase over time (SCG) or remain stable (VFG) throughout the 10 periods of the simulation.
The combined effects on school enrolment and continuation increase school attainment measured as completed years of schooling. The effect is relatively low, but increases over time resulting in a difference of slightly more than 0.01 years of schooling in the SCG scenario after 10 periods (see figure 3). The figure seems relatively small, but it has to be noted that it refers to all individuals with completed schooling, which reflects about one third of the population of roughly 39 million Ugandans, which translates into a very large absolute number of additional years of schooling that the programmes could generate. As with the previous results, the effect is significantly larger for SCG compared with VFG due to the larger number of programme beneficiaries and the targeting of VFG to vulnerable families with orphans and disabled children, who are unlikely to attend schools even with monetary support.
7.3. Returns to education
In the last step, we examine the monetary returns to increased school attainment. The monetary returns to education per year are close to zero in the beginning but increase steadily to about 100 UGS (SCG) and 20 UGS (VFG) per capita in period 10. However, comparing the education returns to the programme transfers still shows a negative balance after 10 periods. To account for the different cost structures, presents the rate of return (RoR) of both programmes. The net present value of direct and indirect benefits generated through education returns are compared to the net present value of the operational costs of the programme as follows:
Figure 4. Monetary returns to education (local mean smoothing with bandwidth of 1.5) and rates of return (line graph).
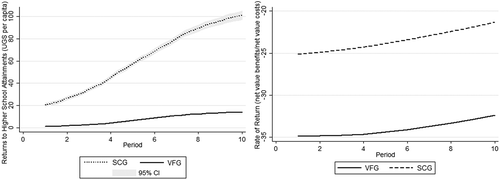
Benefits and costs are discounted with a discount rate of 10%.Footnote18 The results show that both programmes do not generate positive returns through education. However, we see an increase of around 3pp-4pp of both programmes over time due to the returns to education. The results suggest that the SCG performs better in terms of the RoR, which is due to the lower operational costs of the programme.
The negative RoR’s could be related to the fact that only 80% of the programme transfers are used for consumption and thus 20% of the transfers are regarded as unproductive costs in the analysis. Using a propensity to consume of 100% instead, the RoR turns positive after 13 periods for the SCG but remain negative after 20 periods for the VFG. Given by the fact that they also use a propensity to consume of 100%, these results are in line with Mideros, Gassmann, and Mohnen (Citation2016). As robustness check, we furthermore used the lower and upper bound predictions of the estimations in the simulations. To illustrate this, Figure A6 in the technical Annexe displays the results for the RoR using the lower and upper bound coefficients of the returns to education () in the simulation model. After ten periods, the results of the upper and lower bounds differ by around one pp compared with the average predictions of the returns to education, but the overall implications of the findings still hold.
8. Limitations
Several data constraints influenced our methodological choices. Erratic income reporting lead us to use consumption per capita as welfare measure. While this is common practice in a developing country context – especially when measuring poverty (Deaton Citation2005) – our elasticity estimates relied on assumptions regarding the propensity to consume. We tested the robustness of our results by using a range of different parameters found in the literature. These different model predictions broadly support the main results of our study. A further limitation of the study is that the data only contain anthropometric information of children under 5. Because of the longitudinal data structure, we can track the consequences of undernutrition at age four in consecutive years, but we cannot model the immediate effects of transfers on undernutrition of older children and adults. Lastly, we do not model transformative effects that could be generated by empowering women and elderly, which could increase their intra-household bargaining power. Several papers have found support for such transformative effects of cash transfers (Attanasio and Lechene Citation2002; Angelucci and Attanasio Citation2013; Attanasio and Lechene Citation2014). However, the extent to which these effects help to achieve program objectives remains unclear and the empirical evidence on the effects of targeting on intra-household bargaining power is mixed. On one hand side, cash transfers targeted to specific household members can increase their control over household resources (Almås et al. Citation2018), but on the other hand, while targeting cash transfers to women in the household aims to increase their resource control, in practice other household members may take over control over these resources or reallocate other resources (Roy et al. Citation2015). To explore whether income transfers could lead to different responses when targeted to women and elderly household members, we estimated the models with interaction terms for households led by women or members over 65. Even though the results are only indicative, we find no significant differences in the income elasticities (see technical Appendix table 10 and 11). Therefore, we decided to use the same semi-elasticties in the simulation model.
It is important to note that due to data limitations our model only considers monetary returns through increased levels of education. Other indirect effects such as for example returns to improved adult health, increased agricultural investments, and improved risk management are missing and could change the estimated rates of return significantly. Even for education effects, we cannot consider important aspects such as the quality of schooling. Studies have found that the universal education policies in Uganda increase enrolment but the quality of education is still poor (Chapman, Burton, and Werner Citation2010; Huylebroeck and Titeca Citation2015). The effect of improved education on better health, or the effects of improved health on mortality rates and thus programme costs, cannot be modelled with the available household data.
9. Conclusions
In this paper, we performed an ex-ante assessment of the effects of two cash transfer programmes in Uganda in terms of child health, educational attainment, and the indirect monetary returns of these programmes looking beyond the short-term effects. The objective of the study is to analyse the evolution of returns to programme induced human capital investments and the weight of these indirect returns in a cost benefit analysis.
After 10 years, the simulation predicts a decrease of 1.5 pp in child underweight and an increase in school attainment of 0.01 years of schooling of all adults compared with a scenario without the cash transfer programmes. Despite positive returns to education, the programme costs still exceed these indirect benefits after 10 periods leading to negative rates of return. Comparing the SCG programme, which is universally targeted at people above 64, with the proxy-means tested VFG programme shows that the universal programme leads to larger effects and less negative rates of return. This is mainly due to the higher pay-outs for the SCG programme, the targeting of VFG to vulnerable families with orphan and disabled children, who are less likely to achieve higher school attainment even with monetary support, and the three times larger administrative costs of the VFG.
Despite several limitations, the study helps to understand the magnitude of the programme induced returns to human capital. The results show how the effects add up over time and how indirect effects of the programme can further fuel promotional and transformational effects. From a utilitarian perspective, the negative rates of return could suggest to rather invest in schooling infrastructure with potentially higher rates of return as compared to scaling up the SCG and the VFG. Given Uganda’s significant efforts in promoting education for all, the results could indicate that there is limited additional scope for the analysed programmes to increase school enrolments. However, from a poverty reduction perspective – which is ultimately the focus of SAGE – a scale-up could have a sustainable effect on beneficiary households through human capital investments and increasing returns over time. Programme rates of return could be also improved in several ways. For example, a scale up could of the programme could generate higher returns for the same costs due to economics of scale. Moreover, the returns could be even higher than estimated as, given the alarmingly high youth unemployment rates, the programmes could facilitate the transition into the labour market through the increased human capital.
technicalannex.docx
Download MS Word (107.5 KB)Acknowledgments
The research reported in this paper is part of the research agenda of the Knowledge Platform Inclusive Development Policies and funded by the Dutch Ministry of Foreign Affairs throughNWO-WOTRO.
Disclosure statement
No potential conflict of interest was reported by the authors.
Supplementary material
Supplemental data for this article can be accessed here
Additional information
Funding
Notes on contributors
Stephan Dietrich
Stephan Dietrich is Assistant Professor at UNU-MERIT. His research focuses on individual and household level responses to economic shocks and public policies to protect vulnerable populations.
Daniele Malerba
Daniele Malerba is a Research Fellow at the German Development Institute (DIE) and Honorary Fellow at the University of Manchester. His research focuses on social protection and poverty alleviation, and their links with climate mitigation.
Armando Barrientos
Armando Barrientos is Professor Emeritus of Poverty and Social Justice at the Global Development Institute, University of Manchester.
Franziska Gassmann
Franziska Gassmann is Professor of Social Protection and Development at Maastricht University and Professor Poverty and Social Protection at Bonn-Rhein-Sieg University of Applied Science. Her research studies social protection policies and the measurement of poverty and vulnerability using primarily empirical methods on the basis of large household surveys.
Pierre Mohnen
Pierre Mohnen is Professor at Maastricht University and Professorial Fellow at UNU-MERIT. He does empirical research on R&D, innovation, productivity, growth, development and policy evaluations.
Nyasha Tirivayi
Nyasha Tirivayi is a Research Fellow at the United Nations University (UNU-MERIT). She holds a PhD in Public Policy from Maastricht University. Her research focus is on impact evaluations of policies and programs in the fields of social protection, health and agriculture.
Notes
1. With the exception of Karamoja district, where – due to extreme poverty and reduced life expectancy – the age criterion for enrolment is lowered to 60 years.
2. The weights of the VFG eligibility score are presented in table A1 in a separate technical Annexe.
3. See Glewwe and Miguel (Citation2007) for a more detailed discussion of the (conditional) demand functions.
4. Existing evidence advocates against the alternative assumption that pensions are not shared within households (e.g. Woolard and Klasen Citation2005). In the simulation, we need to assume that transfers do not change households’ internal decision-making. VFG transfers are (preferably) allocated to female household members and SCG to the elderly, which could strengthen their decision making power within the household leading to a different use of the transfer as compared with other incomes (see e.g. Angelucci and Attanasio (Citation2013)). However, for simplicity we assume that households use incomes interchangeably.
5. As it is too complex to model the formation of new households such as, for example members moving out to form new families, we only simulate the mid-term effects over 10 periods in order to avoid excessively large households over time.
6. This is to ensure that random and fixed effects models are based on the same sample.
7. We aggregated all household income sources including employed and non-employed work, farm incomes, non-farm enterprises, and other household incomes such as rents, interest rates, and inheritances. More than 15% reported negative or no incomes in all 4 waves.
8. It has to be noted that the propensity to consume assumption has important implications for the results. Using a propensity to consume of 100% leads to a significant positive upward shift in the results.
9. Trying to capture the full meaning of health seems impossible due to the multi-dimensional aspects and factors that make up health. Anthropometric indicators have been the workhorse in the health economic literature and have been shown to be correlated with a wide array of current and future health outcomes (Strauss and Thomas Citation2007).
10. Descriptive statistics and definitions of the control variables used in the analysis can be found in the technical Annexe Table A2.
11. We use the natural logarithm of consumption in regard to the log-normal distribution of the consumption variable in the data. For the simulations, we apply a Duan’s smearing factor to retransform log consumption predictions to level values (Duan Citation1983).
12. In the long run households with higher incomes can choose to invest in the health environment through own investments (sanitation or drinking water) or let investments be made on behalf of them at the community level.
13. Whenever possible we compared the presented results with the marginal effects evaluated at the means of probit models. We found only small differences.
14. Including the inverse Mills ratio in the estimations to correct for the selection of children into school enrolment yields similar coefficients (probit random effects models; results not presented).
15. As robustness check, we estimated the returns to education using the mean years of schooling and got very similar results.
16. Note that the number of observations drop markedly in the 2SLS model as the community information on access to schools has many missing observations.
17. Findings do not change markedly using bandwidths between 1–2 in steps of 0.25 as robustness check (not presented). In addition, several probabilistic elements (mortality, fertility, propensity of school enrolment etc.) in the simulation model can lead to a variation in the simulated outcomes. Figure A7 in the technical Annexe displays the variation in outcomes due to the probabilistic elements after iterating the simulation model for 25 times.
18. The discount rate was set based on the inflation rate of 6.5% in 2012–2013 plus a net interest rate of 3.5%.
References
- Almås, I., A. Armand, O. Attanasio, and P. Carneiro. 2018. “Measuring and Changing Control: Women’s Empowerment and Targeted Transfers.” The Economic Journal 128 (612): F609–F39. doi:10.1111/ecoj.12517.
- Angelucci, M., and O. Attanasio. 2009. “Oportunidades: Program Effect on Consumption, Low Participation, and Methodological Issues.” Economic Development and Cultural Change 57: 479–506. doi:10.1086/596616.
- Angelucci, M., and O. Attanasio. 2013. “The Demand for Food of Poor Urban Mexican Households: Understanding Policy Impacts Using Structural Models.” American Economic Journal: Economic Policy 5 (1): 146–205.
- Angrist, J. D., and J.-S. Pischke. 2008. Mostly Harmless Econometrics: An Empiricist’s Companion. Princeton university press. Princeton.
- Appleton, S. 2001. Education, Incomes and Poverty in Uganda in the 1990s.
- Attanasio, O., and V. Lechene. 2002. “Tests of Income Pooling in Household Decisions.” Review of Economic Dynamics 5 (4): 720–748. doi:10.1006/redy.2002.0191.
- Attanasio, O., and V. Lechene. 2014. “Efficient Responses to Targeted Cash Transfers.” Journal of Political Economy 122 (1): 178–222. doi:10.1086/674968.
- Attanasio, O. P., C. Meghir, and A. Santiago. 2011. “Education Choices in Mexico: Using a Structural Model and a Randomized Experiment to Evaluate Progresa.” The Review of Economic Studies 79 (1): 37–66. doi:10.1093/restud/rdr015.
- Baird, S., C. McIntosh, and B. Özler. 2016. “When the Money Runs Out: Do Cash Transfers Have Sustained Effects on Human Capital Accumulation?”.
- Barham, T., K. Macours, and J. A. Maluccio. 2013. “More Schooling and More Learning? Effects of a Three-year Conditional Cash Transfer Program in Nicaragua after 10 Years.” IDB Working Paper Series.
- Barrientos, A. 2013. Social Assistance in Developing Countries. Cambridge University Press. Cambridge.
- Bastagli, F., J. Hagen-Zanker, L. Harman, V. Barca, G. Sturge, and T. Schmidt. 2018. “The Impact of Cash Transfers: A Review of the Evidence from Low- and Middle-income Countries.” Journal of Social Policy 48 (3): 569–594. doi:10.1017/S0047279418000715.
- Bengtsson, N. 2010. “How Responsive Is Body Weight to Transitory Income Changes? Evidence from Rural Tanzania.” Journal of Development Economics 92 (1): 53–61. doi:10.1016/j.jdeveco.2009.01.002.
- Bourguignon, F., and A. Spadaro. 2006. “Microsimulation as a Tool for Evaluating Redistribution Policies.” The Journal of Economic Inequality 4 (1): 77–106. doi:10.1007/s10888-005-9012-6.
- Bourguignon, F., H. G. Francisco, Ferreira, and P. G. Leite. 2003. “Conditional Cash Transfers, Schooling, and Child Labor: Micro-Simulating Brazil’s Bolsa Escola Program.” The World Bank Economic Review 17 (2): 229–254. doi:10.1093/wber/lhg018.
- Brent, R. J. 2013. “A Cost-benefit Framework for Evaluating Conditional Cash-transfer Programs.” Journal of Benefit-Cost Analysis 4 (2): 159–180. doi:10.1515/jbca-2012-0014.
- Card, D. 2001. “Estimating the Return to Schooling: Progress on Some Persistent Econometric Problems.” Econometrica 69: 1127–1160. doi:10.1111/1468-0262.00237.
- Chapman, D. W., L. Burton, and J. Werner. 2010. “Universal Secondary Education in Uganda: The Head Teachers’ Dilemma.” International Journal of Educational Development 30 (1): 77–82. doi:10.1016/j.ijedudev.2009.08.002.
- Deaton, A. 2005. “Measuring Poverty in a Growing World (or Measuring Growth in a Poor world).” The Review of Economics and Statistics 87 (1): 1–19. doi:10.1162/0034653053327612.
- Deininger, K. 2003. “Does Cost of Schooling Affect Enrollment by the Poor? Universal Primary Education in Uganda.” Economics of Education Review 22 (3): 291–305. doi:10.1016/S0272-7757(02)00053-5.
- Duan, N. 1983. “Smearing Estimate: A Nonparametric Retransformation Method.” Journal of the American Statistical Association 78 (383): 605–610. doi:10.2307/2288126.
- Duflo, E. 2000. “Child Health and Household Resources in South Africa: Evidence from the Old Age Pension Program.” The American Economic Review 90 (2): 393–398. doi:10.1257/aer.90.2.393.
- Duflo, E. 2003. “Grandmothers and Granddaughters: Old‐age Pensions and Intrahousehold Allocation in South Africa.” The World Bank Economic Review 17 (1): 1–25. doi:10.1093/wber/lhg013.
- Etang Ndip, A., and C. Tsimpo. 2017. Beyond Income Poverty: Nonmonetary Dimensions of Poverty in Uganda. World Bank. Washington.
- Fernald, L. C. H., P. J. Gertler, and L. M. Neufeld. 2009. “10-year Effect of Oportunidades, Mexico’s Conditional Cash Transfer Programme, on Child Growth, Cognition, Language, and Behaviour: A Longitudinal Follow-up Study.” The Lancet 374 (9706): 1997–2005. doi:10.1016/S0140-6736(09)61676-7.
- Filipski, M., and J. Edward Taylor. 2012. “A Simulation Impact Evaluation of Rural Income Transfers in Malawi and Ghana.” Journal of Development Effectiveness 4 (1): 109–129. doi:10.1080/19439342.2012.649542.
- Fiszbein, A., N. Schady, F. H. G. Ferreira, M. Grosh, N. Keleher, P. Olinto, and E. Skoufias. 2009. Conditional Cash Transfers: Reducing Present and Future Poverty. Washington, DC: World Bank.
- García, A., O. L. Romero, O. Attanasio, and L. Pellerano. 2012. “Impactos De Largo Plazo Del Programa Familias En Acción En Municipios De Menos De 100 Mil Habitantes En Los Aspectos Claves Del Desarrollo Del Capital Humano.” Informe final. Unión temporal Econometría y SEI.
- Girma, S., and A. Kedir. 2005. “Heterogeneity in Returns to Schooling: Econometric Evidence from Ethiopia.” The Journal of Development Studies 41: 1405–1416. doi:10.1080/00220380500187026.
- Glewwe, P., and E. A. Miguel. 2007. “The Impact of Child Health and Nutrition on Education in Less Developed Countries.” Handbook of Development Economics 4: 3561–3606.
- Glewwe, P., and H. Jacoby. 1994. “Student Achievement and Schooling Choice in Low-income Countries: Evidence from Ghana.” Journal of Human Resources 29: 843–864. doi:10.2307/146255.
- Glewwe, P., and M. Kremer. 2006. “Schools, Teachers, and Education Outcomes in Developing Countries.” Handbook of the Economics of Education 2: 945–1017.
- Grimm, M. 2011. “Does Household Income Matter for Children’s Schooling? Evidence for Rural Sub-Saharan Africa.” Economics of Education Review 30 (4): 740–754. doi:10.1016/j.econedurev.2011.03.002.
- Haddad, L., H. Alderman, S. Appleton, L. Song, and Y. Yohannes. 2003. “Reducing Child Malnutrition: How Far Does Income Growth Take Us?” The World Bank Economic Review 17 (1): 107–131. doi:10.1093/wber/lhg012.
- Ham, A., and H. C. Michelson. 2018. “Does the Form of Delivering Incentives in Conditional Cash Transfers Matter over a Decade Later?” Journal of Development Economics 134: 96–108. doi:10.1016/j.jdeveco.2018.05.007.
- Handa, S., L. Natali, D. Seidenfeld, G. Tembo, and B. Davis. 2018. “Can Unconditional Cash Transfers Raise Long-term Living Standards? Evidence from Zambia.” Journal of Development Economics 133: 42–65. doi:10.1016/j.jdeveco.2018.01.008.
- Haushofer, J., and J. Shapiro. 2016. “The Short-term Impact of Unconditional Cash Transfers to the Poor: Experimental Evidence from Kenya.” The Quarterly Journal of Economics 131: 1973–2042. doi:10.1093/qje/qjw025.
- Huylebroeck, L., and K. Titeca. 2015. “Universal Secondary Education (USE) in Uganda: Blessing or Curse? the Impact of USE on Educational Attainment and Performance.” In L’Afrique Des Grands Lacs: Annuaire 2014-2015/reyntjens, f.[edit.]; Et Al., 349–372. Antwerp: University Press. Antwerp.
- Jolliffe, D. 2004. “The Impact of Education in Rural Ghana: Examining Household Labor Allocation and Returns on and off the Farm.” Journal of Development Economics 73 (1): 287–314. doi:10.1016/j.jdeveco.2003.02.002.
- Kakwani, N., F. Soares, and H. H. Son. 2006. “Cash Transfers for School-Age Children in African Countries: Simulation of Impacts on Poverty and School Attendance.” Development Policy Review 24 (5): 553–569. doi:10.1111/j.1467-7679.2006.00347.x.
- Kerr, A., and S. Quinn. 2010. Returns to Education in Tanzania: exploiting a natural experiment, 2010.
- Kingdon, G., and M. Soderbom. 2007. Education, skills and labor market outcomes: evidence from Ghana.
- Kremer, M., C. Brannen, and R. Glennerster. 2013. “The Challenge of Education and Learning in the Developing World.” Science 340 (6130): 297–300. doi:10.1126/science.1235350.
- Leyaro, V., O. Morrissey, and T. Owens. 2010. “Food Prices, Tax Reforms and Consumer Welfare in Tanzania 1991–2007.” International Tax and Public Finance 17: 430–450. doi:10.1007/s10797-010-9139-7.
- Merttens, F., E. Sindou, A. Lipcan, L. Pellerano, M. Binci, S. Ssewanyana, S. Neema, et al. 2016. “Uganda - Social Assistance Grants for Empowerment Programme 2014, Endline: Impact After Two Years of Programme Operations.”
- Mideros, A., F. Gassmann, and P. Mohnen. 2016. “Estimation of Rates of Return on Social Protection: Ex Ante Microsimulation of Social Transfers in Cambodia.” Journal of Development Effectiveness 8 (1): 67–86. doi:10.1080/19439342.2015.1025815.
- Molina-Millán, T., T. Barham, K. Macours, J. A. Maluccio, and M. Stampini. 2019. “Long-Term Impacts of Conditional Cash Transfers: Review of the Evidence.” The World Bank Research Observer 34 (1): 119–159. doi:10.1093/wbro/lky005.
- Parker, S. W., and T. Vogl. 2018. Do conditional cash transfers improve economic outcomes in the next generation? Evidence from Mexico. No. w24303. National Bureau of Economic Research.
- Peet, E. D., G. Fink, and W. Fawzi. 2015. “Returns to Education in Developing Countries: Evidence from the Living Standards and Measurement Study Surveys.” Economics of Education Review 49: 69–90. doi:10.1016/j.econedurev.2015.08.002.
- Peruffo, M., and P. C. Ferreira. 2017. “The Long-term Effects of Conditional Cash Transfers on Child Labor and School Enrollment.” Economic Inquiry 55 (4): 2008–2030. doi:10.1111/ecin.12457.
- Psacharopoulos, G. 1985. “Returns to Education: A Further International Update and Implications.” Journal of Human Resources 20: 583–604. doi:10.2307/145686.
- Psacharopoulos, G. 1994. “Returns to Investment in Education: A Global Update.” World Development 22 (9): 1325–1343. doi:10.1016/0305-750X(94)90007-8.
- Psacharopoulos, G., and H. A. Patrinos. 2004. “Returns to Investment in Education: A Further Update.” Education Economics 12 (2): 111–134. doi:10.1080/0964529042000239140.
- Rankin, N., J. Sandefur, and F. Teal. 2010. “Learning & earning in Africa: Where are the returns to education high?” In.
- Roy, S., J. Ara, N. Das, and A. R. Quisumbing. 2015. ““Flypaper effects” in Transfers Targeted to Women: Evidence from BRAC’s “Targeting the Ultra poor” Program in Bangladesh.” Journal of Development Economics 117: 1–19. doi:10.1016/j.jdeveco.2015.06.004.
- Strauss, J., and D. Thomas. 2007. “Health over the Life Course.” Handbook of Development Economics 4: 3375–3474.
- Todd, P. E., and K. I. Wolpin. 2008. “Ex Ante Evaluation of Social Programs.” Annales d’Economie Et De Statistique 263–291. doi:10.2307/27917248.
- Todd, P. E., and K. I. Wolpin. 2010. “Structural Estimation and Policy Evaluation in Developing Countries.” Annual Review of Economics 2 (1): 21–50. doi:10.1146/annurev.economics.102308.124345.
- Woolard, I., and S. Klasen. 2005. “Determinants of income mobility and household poverty dynamics in South Africa.” Journal of Development Studies 41, no. 5 (2005): 865–897.