ABSTRACT
A non-targeted workflow is reported for the isolation and identification of antimicrobial active compounds using bioassay-directed screening and LC coupled to high-resolution MS. Suspect samples are extracted using a generic protocol and fractionated using two different LC conditions (A and B). The behaviour of the bioactive compound under these different conditions yields information about the physicochemical properties of the compound and introduces variations in co-eluting compounds in the fractions, which is essential for peak picking and identification. The fractions containing the active compound(s) obtained with conditions A and B are selected using a microbiological effect-based bioassay. The selected bioactive fractions from A and B are analysed using LC combined with high-resolution MS. Selection of relevant signals is automatically carried out by selecting all signals present in both bioactive fractions A and B, yielding tremendous data reduction. The method was assessed using two spiked feed samples and subsequently applied to two feed samples containing an unidentified compound showing microbial growth inhibition. In all cases, the identity of the compound causing microbiological inhibition was successfully confirmed.
Graphical Abstract
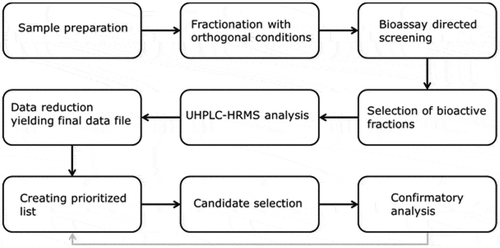
Introduction
Antibiotics are widely used in animal husbandry for the treatment and prevention of bacterial infections. Although their application as growth promoters is no longer allowed in the European Union (EU; Regulation EC 1831/2003 of the European Parliament and of the Council of 22 September 2003 on additives for use in animal nutrition Citation2003), this is still common practice in many other parts of the world. Antibiotics are often administered orally through feed and water at herd or flock level (Cromwell Citation2002). Environmental exposure of antibiotic residues can have great impact on the microbial populations and causes antimicrobial resistance development or persistence (Kümmerer Citation2003; Nõlvak et al. Citation2013; Zhu et al. Citation2013; Barra Caracciolo et al. Citation2015), which compromises the effectiveness of these drugs in both human and animal medicine. Therefore, responsible use of antibiotics in animal husbandry is essential, and comprehensive monitoring strategies are vital for enforcement.
A frequently used strategy for antibiotic residue monitoring comprises a fast effect-based initial microbiological screening method, followed by targeted confirmation of the suspect samples using LC-MS/MS (Pikkemaat Citation2009). However, the scope of such a targeted LC-MS/MS method is by definition limited. Consequently, when a compound causing the microbial growth inhibition is not within the scope of the targeted LC-MS/MS method; for example, if it is a disinfectant or a substance not registered for veterinary application, it will not be detected in such cases. Non-targeted analysis is needed to identify such ‘unknown’ antimicrobial compounds (Borràs et al. Citation2011).
Recent instrumental advances have led to the development of high-resolution MS (HRMS) capable of determining molecular masses with high mass accuracy, enabling full-scan non-targeted analysis (Nielen Citation2007; Brabander Citation2009). However, most applications so far using this technique are targeted approaches. Several multi-residue methods based on HRMS have been reported in the last few years for the analysis of antibiotics in various biological matrices. Peters (Citation2009) and Kaufmann et al. (Citation2011) have presented multi-residue screening methods covering ~100 veterinary drugs in egg, fish and tissue. Stolker et al. (Citation2008) presented an ultra-high performance LC method combined with time-of-flight mass spectrometry (UHPLC–TOF/MS) for screening and quantification of >100 veterinary drugs in milk. It was concluded that the technique is suitable for the analysis in one single method of both veterinary drugs and also other organic contaminants like pesticides, mycotoxins, and plant toxins (Boix et al. Citation2014). Some successfully validated full-scan HRMS methods have been reported for the analysis of a broad range of antibiotics (Kaklamanos et al. Citation2013) and antibiotics in combination with antifungal drugs (Chitescu et al. Citation2012) using the Fourier Transform (FT) Orbitrap MS. It was concluded that FT-Orbitrap MS has a high resolving power and provides a high mass accuracy, enabling qualitative and quantitative analysis in complex matrices such as animal feed and water at relevant concentration levels. León et al. (Citation2012) described a method for wide range screening of banned veterinary drugs in urine by UHPLC-HRMS analysis using Orbitrap MS.
Several papers have reported that HRMS is applicable for detecting unknown compounds (Le Bizec et al. Citation2009; Krauss et al. Citation2010; Zedda and Zwiener Citation2012). Zedda and Zwiener (Citation2012) proposed a general workflow comprising the determination of the elemental composition from an accurate mass, followed by the search of compound databases and additionally the use of physicochemical characteristics, like retention times (RT) and fragmentation products, for further elucidation of the identity. Krauss et al. (Citation2010) demonstrated the power of coupling LC and HRMS for identification of unknown compounds. The method presented in this paper is a bioassay-directed fractionation procedure. The method consists of a sample extraction, HPLC separation, non-targeted screening, automated exact mass filtering, and a library search for matching molecular formulas and structures, resulting in a list of tentative ‘known unknown’ compounds present.
Obviously, the biggest challenge in non-targeted HRMS identification is the selection of relevant signals out of the tremendous amount of data generated. Accurate data processing has become more feasible through the availability of software capable of automatically processing entire batches of data and simultaneously facilitating fast library searching for compound identification. Examples of useful software are MetAlign® (Lommen Citation2009, Citation2012; Lommen and Kools Citation2012) and commercially available software like Sieve (Thermo Scientific Citation2016) and Markerlynx Waters Citation2016. Even after software-mediated data reduction, the number of potentially relevant signals will often remain too large to handle. Therefore, additional strategies for the selection of relevant signals are essential. The strength and the novelty of the current strategy lies in the combination of two orthogonal LC fractionations, effect-based screening, and effective data handling. Our examples are based on an antimicrobial screening assay, but this can in fact be substituted by any effect-based bioassay. The result is a broadly applicable non-targeted approach that yields a limited selection of tentative candidates, followed by confirmatory analysis. We demonstrate that this procedure is capable of identifying ‘known unknown’ microbial growth inhibitors in feed, which, due to its complex composition, is considered to be one of the most difficult matrices for residue analysis.
Materials and methods
Ultra LC-MS grade water, methanol and acetonitrile (Actu-all chemicals, Oss, the Netherlands), formic acid 98–100% LC-MS grade, ammonia solution 25% and ammonium formate (Merck, Darmstadt, Germany) were used. Milli-Q water was prepared using a Milli-Q system at a resistivity of ≥18.2 MΩ cm–1 (Millipore, Billerica, MA). PEG average molecular weight 200, 300, and 600 were obtained from Sigma-Aldrich (St Louis, MI). PEG average weight 1000 and 1500 were obtained from Acros Organics (Geel, Belgium). The Exactive Orbitrap calibration standards for positive and negative ESI were obtained from ThermoFisher Scientific (San Jose, CA). External calibration standard: PEG standard of 50 µg/L. Didecyldimethylammonium chloride (DDAC) was obtained from Dr Ehrenstorfer LGC (Teddington, UK).
Approach
Routinely, samples of animal feed are analysed for the presence of antimicrobials using a growth inhibition assay (microbiological screening assay). Samples showing growth inhibition are subsequently analysed with targeted LC-MS/MS for confirmatory analysis. If this fails to yield a positive confirmation, the sample is classified as containing an ‘unidentified growth inhibitor’ and is subsequently forwarded into the procedure shown in .
Sample extracts are subjected to two separate HPLC fractionations utilising orthogonal conditions. Next, the bioassay-directed screening yielding the initial suspect sample, in this case a microbiological screening assay, is reapplied to select fractions containing the bioactive compound(s). These fractions are analysed by UHPLC-HRMS followed by data reduction and processing using MetAlign® software yielding the final data file (Excel). This final data file is ranked using several criteria (see data reduction), resulting in a prioritised list of tentative candidates. On basis of the determined molecular formula, ChemSpider (Royal Society of Chemistry 2016) searches literature for microbial active compounds. After selection of the most likely candidates from the tentative candidate(s) (see data reduction), confirmatory analysis is performed. If the proposed identity of the unknown bioactive compound is not confirmed (the unknown compound does not match with the selected tentative candidates), an additional list of tentative candidates will be selected for confirmatory analysis.
Sample preparation
A sample of 5 g animal feed was transferred into a polypropylene centrifuge tube and 10 mL of MeOH/water (1:1 v/v) added. The tube was then shaken for 15 min on a rotary tumbler. After centrifugation (3600 g, 15 min, 18°C), 2.5 mL of the upper layer was transferred to a 12 mL polypropylene tube. The extract was diluted with 2.5 mL MeOH/water (1:1 v/v). After mixing and centrifugation (3600 g, 5 min, 18°C), the extract was divided among four glass recovery vials (1.2 mL) and fractionated.
Fractionation
The fractionation system consisted of an Agilent 1200 series vacuum degasser, binary solvent pump, standard micro and preparative auto sampler, thermostatted column compartment, variable wavelength detector, and a preparative scale fraction collector.
After sample preparation, each sample was fractionated using two different separation conditions. Procedure A applies acidic solvents, pH 2 (solvent A, 0.2% formic acid; solvent B, 0.2% formic acid in ACN/H2O [9:1 v/v]). Procedure B applies slightly alkaline solvents; pH 8 (solvent A, 0.01% ammonium formate; solvent B, 0.01% ammonium formate ACN/H2O [9:1 v/v]). All other conditions were similar for both fractionations: the analytical column used was a Phenomenex (Torrance, CA) Kinetex EVO C18 (100 × 4.6 mm, 5 µm). The gradient applied was: 0–1.0 min, 10% B; 1.0–8.0 min, linear increase to 40% B; 8.0–15.0 min, linear increase to 100% B; 15.0–19.0 min, constant at 100% B; 19.0–20.0 min, linear decrease to 10% B; 20.0–30.0 min, equilibration at 10% B; operating at a flow of 0.4 mL min−1. The injection volume was 100 µL and the column temperature 30°C. During the first 25 min of a run, symmetrical time segmentation was applied: 0.5-min fractions (0.2 mL) were collected, yielding 50 fractions for each run. In total, one sample extract is injected 10 times to increase the total volume per fraction, yielding a total volume of 2 mL for each fraction. After collection, 5 mL MeOH was added to each 2 mL fraction followed by evaporation under a gentle nitrogen stream at 40°C until dry. Finally, the residue was dissolved in 500 µL H2O/MeOH (9:1, v/v). Each sample, therefore, results in 100 fractions (from the two systems) for subsequent analysis (A1–A50 and B1–B50).
Bioassay-directed screening
The microbial growth inhibition test was performed essentially as described in Pikkemaat et al. (Citation2008) with some modifications. Antibiotic medium no. 1 (BD Difco), supplemented with 100 mM phosphate buffer and 7.5 µg L−1 tylosin, and adjusted to pH 7.3, was inoculated with Micrococcus luteus (ATCC 9341). A volume of 25 mL of the inoculated agar was poured into a 120 × 120 mm bioassay plate and, after the agar had set, holes with a diameter of 9 mm were created to hold the 75 µL samples. Each of the fractions (50 per fractionation condition) was transferred to individual sample holes. Note that all fractions contain 10% MeOH; this does not affect the bacterial growth, as is evident from the fractions that do not contain antimicrobial compounds. After application of the fractions, the plates were incubated for 18–20 h at 30°C. The presence of growth-inhibiting compounds becomes visible as a clear zone (absence of bacterial growth) around the sample hole. For this procedure, any inhibition zone observed that is larger than a blank sample (which usually does not contain any inhibition zone) is considered relevant. If multiple sequential sample holes show inhibition, the fraction in the hole with the largest inhibition zone is selected. The fraction containing the active compound obtained from system A is called the bioactive fraction A; that obtained from system B, bioactive fraction B.
UHPLC-HRMS
The LC system used for HRMS analysis consisted of a Thermo Fisher Scientific (Dionex) (San Jose, CA, USA) Ultimate 3000 system with RS pump, RS auto-sampler and RS column compartment. The analytical column was a Waters Acuity UPLC BEH C18 (100 × 2.1 mm, 1.7 µm). The gradient was, for solvent A, 2 mM ammonium formate and 0.16% (v/v) formic acid; solvent B, 2 mM ammonium formate and 0.16% (v/v) formic acid in ACN/H2O (9:1 v/v): 0–1.0 min, at 0% B; 1.0–4.0 min, linear increase to 40% B; 4.0–10.0 min, linear increase to 100% B; 10.0–13.0 min, at 100% B; 13.0–13.5 min, linear decrease to 0% B; 13.5–16.0 min, at 0% B; operating at a flow of 0.4 mL min–1. The injection volume was 5 µL and the column temperature 40°C. Before injection the final extract was diluted three times by adding a solution of H2O/MeOH (9:1 v/v).
Detection was carried out using a Thermo Scientific Q-Exactive Orbitrap MS operating with a heated electrospray ionisation source in positive and negative mode. The tune operating parameters were: electrospray voltage ± 3.5 kV; sheath gas, 48 AU; auxiliary gas, 11 AU; Aux gas heater, 413°C; heated capillary, 256°C. The instrument was calibrated using a Pierce LTQ Velos ESI positive and negative calibration solution. The setting for the full scan analysis were: polarity, positive; scan range, 120 to 1200 m/z; resolution, 70,000; AGC target, 3,000,000; maximum IT, 200 ms.
The settings for targeted MS/MS, which is applied in the final confirmation phase, were the same as those described for full scan with the following exceptions: default charge, 2; resolution, 17,500; AGC target, 200,000; maximum IT, 100 ms; isolation window, 4 m/z; NCE, 50 ev.
Data reduction
MetAlign® is an in-house developed data processing tool in which the obtained HRMS data from the different bioactive fractions are reduced and converted to a final database file (Excel). The data generated using HRMS analysis were first baseline-corrected using cut-off criteria, signal-to-noise ratio, internal mass calibration, 1-point mass calibration, and subtraction of background signals (Lommen Citation2009, Citation2012; Lommen and Kools Citation2012; Lommen et al. Citation2012). For all signals, molecular formulas are suggested based on the exact mass obtained and the isotope pattern, including C, H, O, N, S, P, F, Cl, and Br.
Essentially, there are two possible approaches for data processing: the grouping approach and the singular approach (). The MetAlign® grouping approach is applied when both chromatography procedures yield bioactive fractions. First, a chromatogram is generated after m/z similarity processing of the chromatograms of bioactive fractions obtained from fractionations A and B; this is called the matching chromatogram. Next, chromatograms of blank fractions are subtracted from the matching chromatogram. Because different feed samples consist of very different commodities and additions, no other feed sample can function as a blank sample. Therefore, for the blank subtraction, fractions of the actual sample that show no bioactivity and which lie close to the bioactive fraction are selected. The matching procedure, including the blank subtraction yields a final data file (Excel). shows a simplified ideal situation in which one signal is left after blank subtraction; in reality, we obtain a list of relevant signals with an accompanying abundance, retention time, and exact mass. The MetAlign® singular approach is applied in case one of the fractionation procedures does not yield a bioactive fraction. In this case, no matching chromatogram can be generated. Blank fractions are directly subtracted from the positive bioactive fraction, yielding the final data file. Because MetAlign® is programmed to also investigate adduct ions, adduct ions are included in the final data file, which in some cases yields additional information on the identity of the compounds.
Candidate selection
The generated final data file is ranked using several criteria (see data reduction) resulting in a prioritised list of tentative candidates. A first criterion for selection is that the ppm error of the detected candidates should, under the chosen settings, be <1 ppm. Additional criteria for prioritisation are signal abundance, retention time, exact mass, and molecular composition of the proposed molecular formula. A good starting point is to prioritise signals with the highest abundance. However, note that the compound causing the effect in the bioassay could also be represented by minor signals due to a low concentration (combined with a high potency) or poor ionisation efficiency in MS. Therefore, this selection must be made with care, taking account of the combined results of the two orthogonal fractionation systems. Note that the obtained information on polarity of the unknown compound (obtained from the retention time in the two orthogonal fractionation systems), and whether it is an acid, neutral, or alkaline compound (obtained from the retention time difference in the two fractionation systems), must match the properties of the proposed molecular formulas. After ranking, a selection of tentative candidates is made for further investigation. This is performed using a direct ChemSpider link coupled to the proposed molecular formula. ChemSpider shows structures, and thus compound names, for the proposed molecular formulas ranked in order of decreasing number of citations in the literature. Proposed (first those with the highest number of citations) are reviewed for possible microbial active characteristics. Based on (1) the chemical structure in relation to the RT shift at different pH, (2) information on the activity of a compound, and (3) the number of references in ChemSpider, one or more possible candidates are selected for confirmatory analysis. Also, information on functional groups in the structure of the unknown compound can be obtained from the HR-MS fragment spectrum. Note that the identity of a compound can only be confirmed for commercially available compounds. However, with this approach, even compounds that have not been previously reported in the literature can be identified; but a more thorough investigation of the fragmentation pattern of such compounds is needed.
Confirmatory analysis
The identity of the compound is subsequently confirmed using commercially available reference standards. The sample and reference standards are analysed using LC-HRMS in targeted MS/MS mode to obtain fragmentation spectra (Berendsen et al. Citation2016). Besides a comparison of the spectra on the presence of all major fragments, the identity of the compound is confirmed using the criteria as established in CD 2002/657/EC (Commission Decision 2002/657/EC Citation2002), including RT and relative ion abundance. As a final check, the biological activity of the reference standard is confirmed in the initial effect-based bioassay.
Testing the procedure
The non-targeted method for the identification of antimicrobial compounds was tested using two samples, referred to as І and ІІ. Sample І was a spiked chicken feed with 2 mg kg−1 roxithromycin (a compound belonging to the macrolide class of antibiotics, but not registered as a veterinary drug) and sample ІІ, a cattle feed spiked with 40 mg kg−1 cryptotanshinone (a natural quinoid diterpene, first isolated from herb roots). The added compounds were known only to the expert preparing the samples and remained unknown to the analyst. Both samples were analysed according to the presented procedure, allowing identification of the added substances.
Demonstration of applicability
After successfully testing the procedure, it was applied to two true unknown samples, referred to as samples III and IV. Sample ІІІ was a commercially available spice mixture intended as a feed additive to improve animal growth. Sample IV was a pig feed sample. Both samples were encountered in routine analysis and clearly showed microbial growth inhibition. However, the presence of veterinary drug compounds was not confirmed using standard targeted MS analysis, but rather analysed according to the presented procedure, allowing confirmation of the identity of unknown active compounds in these samples.
Results and discussion
General
Our aim was to develop a non-targeted strategy for the analysis of a wide range of compounds showing antimicrobial activity. The method starts with a sample preparation that is essentially the same as for the initial microbiological effect-based bioassay, to ensure the bioactive compound is extracted and remains throughout further processing to avoid false negative results. Upon extraction, the sample is diluted without any further clean-up procedure. A key feature of the proposed strategy is the subsequent dual LC fractionation, which is performed in parallel using two different chromatographic conditions. Each condition will have its specific effect on both the retention of the compound of interest and the matrix compounds. Under different chromatographic conditions, the behaviour of the bioactive compound provides clues about the physicochemical characteristics of the compound(s) of interest. This approach ideally results in the compound of interest being present in two different fractions, both containing the active compound but with different matrix compounds. The bioactive fractions of both fractionation procedures are analysed using HRMS. MetAlign® software is applied to select the relevant signals taking advantage of the differences in matrix compounds introduced by the dual LC fractionation (), resulting in the final data file. The generated final data file is ranked using several criteria resulting a prioritised list of tentative candidates, taking into account the already obtained physicochemical information. If a reference standard is commercially available, the identity of the compound can be confirmed according to CD 2002/657/EC (Commission Decision 2002/657/EC, 2002). If no reference standard is available, no official confirmation can be obtained. However, a tentative identity can be determined on the basis of the exact mass, mass fragments in MS/MS and its chemical properties derived from the retention time observed in the two fractionating systems.
Several analytical columns with multiple separation mechanisms and different eluents were tested. The analytical columns were CSH C18, fluorophenyl, phenylhexyl, HSS C18 (all from Waters), cyano (Thermo Fisher Scientific), HILIC (Thermo Fisher Scientific), and EVO C18 (Phenomenex) with acidic, slightly alkaline, and neutral mobile phases. To optimise this protocol, model compounds from different classes of drugs (antibiotics, NSAIDs, anthelmintics, steroids, and β-agonists) were used (full data in supporting information S1). The optimal conditions were defined as those yielding the most extensive orthogonal separation and the smallest tentative candidate list after data reduction, while retaining the actual bioactive compound. The optimal result was obtained using a mobile phase with two different pH values (one acidic and one slightly alkaline) on a similar analytical column. The analytical column showing optimal resolution was a Phenomenex Kinetex EVO C18 (100 × 4.6 mm, 5 µm). This EVO column is applicable within a broad pH range and shows satisfactory peak shapes. Using two separation conditions with a different pH will cause a retention shift of weak acids and bases having a pKa between approximately 1 and 9. This does not only apply to the compound of interest, but also to background signals. In practice, this results in large differences in the ‘background’ LC profiles of the fractions in which the bioactive compound is present, allowing efficient data-reduction possibilities.
As a result of the method optimisation experiments, a general approach is proposed as outlined in the flowchart shown in . The bioassay-directed screening could theoretically yield three different outcomes: none of the fractions showing bioactivity, bioactivity in one or more fractions from only one of the fractionation conditions, or both conditions yielding fractions with bioactivity. If one or both of the applied conditions fails to yield a bioactive fraction, it is possible that the bioactive compound is not stable under these specific conditions, or the concentration of the compound has dropped below its effective threshold concentration. When both or one of the fractionation procedures yields bioactive fractions, the procedure is continued. If no relevant tentative candidate list can be obtained, or if the identity of the unknown bioactive compound cannot be confirmed, then additional HRMS analysis in negative ESI mode is recommended.
After confirmation of a biologically active substance, it cannot be fully excluded that other active substances are present in the sample unless all candidates are extensively studied or unless the concentration of the active compound is related to the observed activity in the bioassay. However, if other antimicrobial substances are present, they must have chemical properties related to the confirmed active compound with respect to its behaviour in the LC fractionation systems.
This procedure is clearly a very laborious one, which takes over a week. Clearly, it is not applied to all routine samples, but only in case a screening method demonstrates the presence of an antimicrobial substance that could not be confirmed using the routinely applied LC-MS/MS confirmatory approach.
Test samples
The non-targeted method for the identification of unknown bioactive compounds was tested using two samples.
Sample I (spiked chicken feed with 2 mg kg−1 roxithromycin)
For test sample I, the dual fractionation procedure yielded bioactivity in fractions 23 and 24 originating from fractionation procedure A and in fractions 41 and 42 obtained with procedure B. This corresponds with an increased retention time of about 10–15 min when alkaline chromatographic conditions are applied, implying that the compound is more charged under acidic conditions compared with basic conditions. It is most likely a weak base. For data reduction, fractions 21 and 26 (respectively, 39 and 44 for acidic and alkaline fractionation) were selected as blanks.
To demonstrate the effect of the two orthogonal fractionations in combination with the grouping approach, the grouping and singular approaches were compared. The singular approach resulted in 90 unique masses for the acidic fractionation, while the grouping approach yielded a final data file with a total of 14 unique masses, illustrating the strength of the presented approach. The final data file was prioritised and further investigation was performed using a direct ChemSpider link coupled to the proposed molecular formulas. Investigation of m/z 837.5314 resulted in roxithromycin as first hit in ChemSpider (ranked in order of highest number of citations in literature). Roxithromycin is a macrolide antibiotic, being an anti-infective, and was selected as a possible candidate. The assumption based on the outcome of the dual fractionation regarding the charging of the bioactive compound complies with the physical properties of roxithromycin (pKa at 2.3 and 9.1): indeed, roxithromycin is ionised under acid conditions, resulting in a decreased RT compared with alkaline chromatographic conditions. Additionally, the analytical standard of roxithromycin was tested in the microbial growth inhibition test, producing the same pattern as the sample. No confirmatory analysis was applied, as the observation was found to be correct: roxithromycin was indeed the compound added to the feed sample.
Sample II (cattle feed spiked with 40 mg kg−1 cryptotanshinone)
Fractionation of sample ІІ showed similar retention times for both chromatographic conditions, yielding bioactivity in fractions 36 and 37 for both procedures. This implies that the compound does not have pKa values between 1 and 9. For data reduction, fractions 34 and 39 were selected as the blanks. Applying the two MetAlign® approaches yielded 299 unique masses with the singular approach, while the grouping approach yielded a total of 73 unique masses, again (although to a lesser extent than for test sample I) showing the strength of the grouping approach. Apparently, many matrix compounds shifted away from the compound of interest in the chromatogram as a result of the two fractionation conditions. The final file of unique masses for sample II was substantially longer compared with the result of sample I. This is caused essentially by the fact that the elution of the bioactive compound is unaffected by the different chromatographic conditions, causing a larger overlap in co-eluting compounds. The final data file was first ranked based on the abundance and further investigation was performed using a direct ChemSpider link coupled to the proposed molecular formulas. The three most abundant ions included both cryptotanshinone (m/z 297.1484) and tanshinone (m/z 295.1328). Both were indicated as the first hit in ChemSpider (ranked in order of highest number of references in literature). Tanshinones are hydrophobic active compounds isolated from danshen (Shi et al. Citation2005) and are active as broad-spectrum bactericides (Hur et al. Citation2015). Cryptotanshinone and tanshinone were selected as possible candidates. The observation that the retention of the active compound(s) in the LC column was not affected by different pH conditions complies with the physical properties of cryptotanshinone and tanshinone, which are uncharged in both acidic and basic conditions. Additionally, the analytical standard of cryptotanshinone (as this was available in the laboratory) was tested in the microbial growth inhibition test, resulting in the same pattern as the sample. No confirmatory analysis was applied, as the observation was found to be correct: cryptotanshinone was indeed the compound added to the feed sample. This case demonstrates that even with a relatively long tentative candidate list, successful identification is possible.
Application of the procedure
After successful testing of the procedure, it was applied to two true unknown samples.
Sample III (unknown)
According to the label, the product comprised a spice mixture intended to be used as a feed additive. The sample showed bacterial growth inhibition in a microbiological screening assay, no positive identification could be obtained with traditional targeted MS analysis.
Fractionation
After fractionation and microbiological effect-based bioassay, the bioactive fractions and the associated blank fractions were selected for HRMS analysis. The selected fraction from the acidic chromatographic system (system A) was A29 with blank fractions A25 and A35. The selected bioactive fraction from the alkaline chromatographic system (system B) was B33 with blank fraction B25. It was observed that the bioactive compound eluted over several fractions, forcing the selection of blank fractions relatively far away from the selected bioactive fraction. The fact that the bioactivity in the sample eluted over several fractions could result from a compound with a poor peak shape in the LC system or indicate the presence of a mixture of bioactive compounds with comparable structures. The latter was later confirmed.
Data reduction
The obtained HRMS data were reduced using the grouping MetAlign® approach, yielding the final data file. When both MetAlign® approaches were applied, the singular approach resulted in 1323 unique masses, whereas the grouping approach yielded 128 unique masses. The final data file, after applying the grouping approach, was prioritised and further investigation was performed using a direct ChemSpider link coupled to the proposed molecular formulas. The most prominent signals (masses with high intensity) were mainly observed around RT 10–11 min in the LC-HRMS chromatogram.
Compound selection and identification
When reviewing the most prominent signals (masses with high intensity), it was observed that most were highly related and could be clustered in three groups (): Group 1 containing Cx, Hy, and N; Group 2 containing Cx, Hy, N, and O; and Group 3 containing C x, Hy, N, and O2. Investigation of these molecular formulas using ChemSpider yielded a list of many different potential compound identities for each of these molecular formulas.
Table 1. Tentative candidate list for sample III; the numbers correspond to the figures in the supporting information.
To gain more insight in the structure of the 10 highest-ranked candidates (), these compounds were fragmented using HRMS in targeted MS/MS mode. The fragment spectra of these compounds are presented in supporting information S2. From the fragmentation spectra of the individual candidates it was concluded that the selected candidates were very similar in structure. For all these candidates, the observed fragments were short alkanes and quaternary ammonium compounds (QACs). QACs are positively charged ions with the structure (NR4)+ containing alkyl or aryl R groups. It was therefore concluded that the bioactive compound(s) most likely comprised a range of QACs.
QACs are widely used for a range of purposes, including biocides, pesticides, herbicides, disinfectants, and additives for technical applications. The literature shows that quaternary ammonium salts can have antimicrobial properties, resulting in growth inhibition (Tischer et al. Citation2012; Jennings et al. Citation2014). Among the molecular structures obtained from the ChemSpider search, the majority were indeed structures of QACs. For m/z 326.378, DDAC was assigned as a possible identity by ChemSpider. As an example, identity confirmation was focused on this compound because it is a common biocide reported to be an antimicrobial, and because its reference standard is commercially available. The identification and confirmation of DDAC is presented below in more detail. The other compounds were only tentatively identified on basis of their MS/MS fragment spectra (see online resource 1). The results are presented in . The identity of these compounds can be confirmed using a commercially available reference standard. Because the reactivity of QACs in collision-induced dissociation (CID) has been extensively reported (Seto et al. Citation1999, Citation2001), the identity of these compounds might also be elucidated with extensive investigation (Sultan and Gabryelski Citation2006) if no reference standard is available; this is beyond this paper’s scope. The presence of a mixture of active compounds can complicate the procedure; but even in such a case, this procedure can be successful, as is demonstrated here.
DDAC confirmation
Targeted MS/MS analysis of the original sample focusing on m/z 326.378, yielded the spectrum presented in ). The molecular ion at m/z 326.378 is clearly observed and also two previously reported product ions were observed: m/z 186.2216 and m/z 57.0702 (Cao et al. Citation2014). After selecting DDAC as a likely candidate for causing the antimicrobial effect, a DDAC analytical reference standard was prepared and fragmented to unequivocally confirm the identity of DDAC in the feed sample. ) shows the spectrum of targeted MS/MS analysis of the 2 mg L−1 DDAC reference standard.
Figure 3. Result of the targeted MS/MS analysis of m/z 326.3781 in (a) the bioactive fraction of sample III and (b) a DDAC reference standard solution (2 mg L−1).
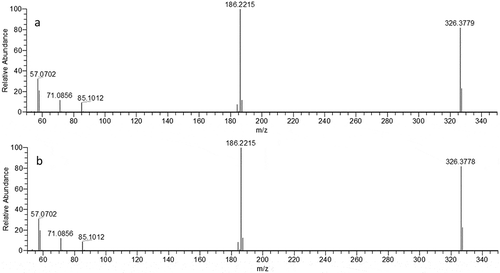
When the targeted MS/MS chromatograms of the sample and the reference standard are compared, the chromatographic peaks show the same retention time, similar fragmentation spectrum and similar ratios of the fragment ions. The results were evaluated according to Commission Decision 2002/657/EC (2002), which provides criteria for the evaluation of confirmatory analysis including retention time and ion ratio deviation. These findings firmly support the identity of the unknown antimicrobial active compound in the sample to be DDAC.
The total fragmentation pattern was also evaluated. shows the structure of DDAC and its tentative product ions. All major product ions of the DDAC reference standard were also observed for the unknown compound, and all product ions could be identified and correspond to the DDAC structure. Overall, it was concluded that these fragmentation results confirm DDAC being among the compound(s) responsible for the antimicrobial activity in sample III. These results also match with previously reported findings (Cao et al. Citation2014). The properties of DDAC comply with the observed shift in RT in the fractionation systems. In conclusion, DDAC could be assigned as the most likely identity of the compound, with m/z 326.378.
To even further support the confirmation, the analytical standard of DDAC was tested in the microbial growth inhibition test resulting activity against the same bacteria as the sample. Because the amount of DDAC in the sample was not determined, we cannot determine whether the antimicrobial activity is caused solely by DDAC.
Finally, the likelihood of DDAC being present in herbal mixtures was assessed based on publicly available information. The European Food Safety Authority (EFSA) published a report (2013) on the monitoring of DDAC and reported positive food samples. Concentrations of DDAC ranged from 0.06 to 14.4 mg/kg in milk, fruits and nuts, and other plant products like oilseeds, pulses, hops, spices, tea, coffee, and sugar plants (European Food Safety, 2013) Since DDAC was observed in many different plant materials, it seems reasonable to expect to find DDAC in a spice sample. Unfortunately, data on DDAC transfer after oral intake by animals are lacking, so we do not know to what extent a herb mixture containing DDAC being part of an animal’s diet will result in residues in animal food products (European Food Safety, 2014).
Sample IV (unknown)
According to the label, the sample was a pig feed. The sample extract showed bacterial growth inhibition in the microbiological screening assay, but no positive identification was obtained with the targeted MS/MS analysis for veterinary antibiotics. The presented procedure for untargeted analysis was applied to this sample in order to identify the compound responsible for the antimicrobial activity. Fractionation resulted in a RT shift, showing lower RT for the alkaline condition compared with the acidic run. The grouping approach resulted in 194 hits, showing linolenic acid as the first candidate based upon its signal abundance, which a commonly used feed additive in pig production (Palmquist Citation2009; Čítek et al. Citation2015). Linolenic acid is negatively charged under alkaline conditions and neutral under acidic conditions, resulting a decreased retention time under alkaline LC conditions compared with acidic conditions, consistent with the observations. Furthermore, antibacterial activity of long-chain unsaturated fatty acids has been reported (Zheng et al. Citation2005). The presence of linolenic acid in the sample was positively confirmed using LC-MS/MS by comparison with a commercially available reference standard (Sigma-Aldrich), and the activity of linolenic acid against the specific bacteria was confirmed by the microbial test.
In summary, a novel strategy for the detection and identification of unknown bioactive compounds in complex samples is presented. This approach is a combination of a generic extraction, an effect-based screening, two parallel fractionations, LC-HRMS, and intelligent software processing. The main improvement compared with previously presented procedures is the use of two parallel, orthogonal fractionation procedures to isolate the bioactive compound. By matching the two fractions containing the bioactive compound, data are significantly reduced, allowing effective signal selection for identification purposes. We have demonstrated that, using this approach, it is possible to identify unknown antimicrobial compounds in feed. Showing the successful application of the method using a matrix as complex as feed, it is anticipated that application to other matrices should be fairly straightforward. Moreover, using the appropriate bioassay-directed screening, the presented approach is applicable to any kind of unknown bioactive compound.
tfac-2017-218-File003.docx
Download MS Word (232.4 KB)tfac-2017-218-File002.docx
Download MS Word (21.3 KB)tfac-2017-218-File001.docx
Download MS Word (51.9 KB)Acknowledgment
This research was funded by the Dutch Ministry of Economic Affairs under its statutory tasks. We thank Dr Arjen Lommen for his assistance using MetAlign®.
Disclosure statement
No potential conflict of interest was reported by the authors.
Supplemental material
Supplemental data for this article can be accessed here.
Additional information
Funding
References
- Barra Caracciolo A, Topp E, Grenni P. 2015. Pharmaceuticals in the environment: biodegradation and effects on natural microbial communities. A review. J Pharm Biomed Anal. 106:25–36.
- Berendsen BJA, Meijer T, Wegh R, Mol HGJ, Smyth WG, Armstrong Hewitt S, Van Ginkel L, Nielen MWF. 2016. A critical assessment of the performance criteria in confirmatory analysis for veterinary drug residue analysis using mass spectrometric detection in selected reaction monitoring mode. Drug Test Anal. 8:477–490.
- Boix C, Ibáñez M, Sancho JV, León N, Yusá V, Hernández F. 2014. Qualitative screening of 116 veterinary drugs in feed by liquid chromatography-high resolution mass spectrometry: potential application to quantitative analysis. Food Chem. 160:313–320.
- Borràs S, Companyó R, Granados M, Guiteras J, Pérez-Vendrell AM, Brufau J, Medina M, Bosch J. 2011. Analysis of antimicrobial agents in animal feed. TrAC, Trends Anal Chem. Aug;30:1042–1064.
- Brabander HFD. 2009. Residue analysis: future trends from a historical perspective. J Chromatogr A. 1216:7964–7976.
- Cao H, Kang M, Chen Z, Li L, Cui M, Chen M, Zhang H, Wang Y. 2014. Determination of five quaternary ammonium compounds in foodstuffs using high performance liquid chromatography-tandem mass spectrometry. Anal Methods. 6:4790–4796.
- Royal Society of Chemistry, 2015. Chemspider search and share chemistry. May 5. Available from: //www.chemspider.com/
- Chitescu C, Oosterink E, Jong J, Stolker A. 2012. Accurate mass screening of pharmaceuticals and fungicides in water by U-HPLC–Exactive Orbitrap MS. Anal Bioanal Chem. 403:2997–3011.
- Čítek J, Stupka R, Okrouhlá M, Vehovský K, Brzobohatý L, Šprysl M, Stádník L. 2015. Effects of dietary linseed and corn supplement on the fatty acid content in the pork loin and backfat tissue. Czech J Anim Sci. 60:319–326.
- European Commission Decision. 2002. 2002/657/EC. Off J Eur Comm L 221, 8–36.
- Cromwell GL. 2002. WHY AND HOW ANTIBIOTICS ARE USED IN SWINE PRODUCTION. Anim Biotechnol. 13:7–27. 2013 Mar 24.
- European Food Safety A. 2013. Evaluation of monitoring data on residues of didecyldimethylammonium chloride (DDAC) and benzalkonium chloride (BAC). EFSA Supporting Publications. 10:n/a-n/a.
- European Food Safety A. 2014. Reasoned opinion on the dietary risk assessment for proposed temporary maximum residue levels (MRLs) of didecyldimethylammonium chloride (DDAC) and benzalkonium chloride (BAC). EFSA J. 12:n/a-n/a.
- Hur JM, Shim JS, Jung HJ, Kwon HJ. 2015. Cryptotanshinone but not tanshinone IIA inhibits angiogenesis in vitro. Exp Mol Med. 37:133–137.
- Jennings MC, Ator LE, Paniak TJ, Minbiole KPC, Wuest WM. 2014. Biofilm-eradicating properties of quaternary ammonium amphiphiles: simple mimics of antimicrobial peptides. ChemBioChem. 15:2211–2215.
- Kaklamanos G, Vincent U, Von Holst C. 2013. Analysis of antimicrobial agents in pig feed by liquid chromatography coupled to orbitrap mass spectrometry. J Chromatogr A. 1293:60–74.
- Kaufmann A, Butcher P, Maden K, Walker S, Widmer M. 2011. Development of an improved high resolution mass spectrometry based multi-residue method for veterinary drugs in various food matrices. Anal Chim Acta. 700:86–94.
- Krauss M, Singer H, Hollender J. 2010. LC–high resolution MS in environmental analysis: from target screening to the identification of unknowns. Anal Bioanal Chem. 397:943–951.
- Kümmerer K. 2003. Significance of antibiotics in the environment. J Antimicrob Chemother. 52:5–7. Jul 1.
- Le Bizec B, Pinel G, Antignac J-P. 2009. Options for veterinary drug analysis using mass spectrometry. J Chromatogr A. 1216:8016–8034.
- León N, Roca M, Igualada C, Martins CPB, Pastor A, Yusá V. 2012. Wide-range screening of banned veterinary drugs in urine by ultra high liquid chromatography coupled to high-resolution mass spectrometry. J Chromatogr A. 1258:55–65.
- Lommen A. 2009. Metalign: interface-driven, versatile metabolomics tool for hyphenated full-scan mass spectrometry data preprocessing. Anal Chem. 81:3079–3086.
- Lommen A. 2012. Plant metabolomics. In: Nigel WH, Robert DH, editors. Plant metabolomics. New York (NY): Springer; p. 229–253.
- Lommen A, Kools H. 2012. MetAlign 3.0: performance enhancement by efficient use of advances in computer hardware. Metabolomics. 8:719–726. Aug 1.
- Lommen A, Van Der Kamp HJ, Kools HJ, Van Der Lee MK, Van Der Weg G, Mol HGJ. 2012. metAlignID: a high-throughput software tool set for automated detection of trace level contaminants in comprehensive LECO two-dimensional gas chromatography time-of-flight mass spectrometry data. J Chromatogr A. 1263:169–178.
- MarkerLynx [Internet]. 2016. Waters. Jul 15. Available from: http://www.waters.com/waters/en_US/MarkerLynx-/nav.htm?cid=513801
- Nielen MWF. 2007. Screening and confirmation criteria for hormone residue analysis using liquid chromatography accurate mass time-of-flight, Fourier transform ion cyclotron resonance and orbitrap mass spectrometry techniques. Anal Chim Acta. 586:122–129.
- Nõlvak H, Truu M, Tiirik K, Oopkaup K, Sildvee T, Kaasik A, Mander T, Truu J. 2013. Dynamics of antibiotic resistance genes and their relationships with system treatment efficiency in a horizontal subsurface flow constructed wetland. Sci Total Environ. 461-462:636–644.
- Palmquist DL. 2009. Omega-3 fatty acids in metabolism, health, and nutrition and for modified animal product foods. Prof Anim Scientist. 25:207–249.
- Peters RJB. 2009. Multi-residue screening of veterinary drugs in egg, fish and meat using high-resolution liquid chromatography accurate mass time-of-flight mass spectrometry. J Chromatogr A. 1216:8206–8216.
- Pikkemaat MG. 2009. Microbial screening methods for detection of antibiotic residues in slaughter animals. Anal Bioanal Chem. 395:893–905.
- Pikkemaat MG, Dijk SO-V, Schouten J, Rapallini M, van Egmond HJ. 2008. A new microbial screening method for the detection of antimicrobial residues in slaughter animals: the Nouws antibiotic test (NAT-screening). Food Control. 19:781–789.
- European Commission Regulation (EC) No 1831/2003 of the European Parliament and of the Council of 22 September 2003 on additives for use in animal nutrition. 2003. Off J Eur Comm L 268, 29–43.
- Seto C, Grossert JS, Waddell DS, Curtis JM, Boyd RK. 1999. Studies of mixed-site and charge-site-remote fragmentations of quaternary ammonium ions. II: effects of chain length12. Int J Mass Spectrom. 188:27–38.
- Seto C, Grossert JS, Waddell DS, Curtis JM, Boyd RK. 2001. Effects of ionization mode on charge-site-remote and related fragmentation reactions of long-chain quaternary ammonium ions. J Am Soc Mass Spectrom. 12:571–579.
- Shi Z, He J, Yao T, Chang W, Zhao M. 2005. Simultaneous determination of cryptotanshinone, tanshinone I and tanshinone IIA in traditional Chinese medicinal preparations containing Radix salvia miltiorrhiza by HPLC. J Pharm Biomed Anal. 37:481–486.
- Stolker AAM, Rutgers P, Oosterink E, Lasaroms JJP, Peters RJB, Rhijn JA, Nielen MWF. 2008. Comprehensive screening and quantification of veterinary drugs in milk using UPLC–ToF-MS. Anal Bioanal Chem. 391:2309–2322.
- Sultan J, Gabryelski W. 2006. Structural identification of highly polar nontarget contaminants in drinking water by ESI-FAIMS-Q-TOF-MS. Anal Chem. 78:2905–2917.
- Thermo Scientific SIEVE Software for Differential Expression Analysis [Internet]. 2016. Thermo Scientific. Jul 15. Available from: http://www.thermo.com/eThermo/CMA/PDFs/Various/File_53659.pdf
- Tischer M, Pradel G, Ohlsen K, Holzgrabe U. 2012. Quaternary ammonium salts and their antimicrobial potential: targets or nonspecific interactions? ChemMedChem. 7:22–31.
- Zedda M, Zwiener C. 2012. Is nontarget screening of emerging contaminants by LC-HRMS successful? A plea for compound libraries and computer tools. Anal Bioanal Chem. 403:2493–2502.
- Zheng CJ, Yoo J-S, Lee T-G, Cho H-Y, Kim Y-H, Kim W-G. 2005. Fatty acid synthesis is a target for antibacterial activity of unsaturated fatty acids. FEBS Lett. 579:5157–5162.
- Zhu YG, Johnson TA, Su JQ, Qiao M, Guo GX, Stedtfeld RD, Hashsham SA, Tiedje JM. 2013. Diverse and abundant antibiotic resistance genes in Chinese swine farms. Proc Natl Acad Sci U S A. 110:3435–3440.