ABSTRACT
Multimodal interactions that incorporate technology use can support rich new forms of educational dialogue that highlight differences between participants’ perspectives and make reasoning processes more explicit. Learning with technology is mediated by digitally represented knowledge artefacts that participants construct together and manipulate through dialogue. However, there is a need for research into the methods by which researchers and practitioners can understand the learning taking place in such complex environments where verbal and nonverbal interactions with technology are inter-connected and entangled with activities and material learning resources. This paper addresses that need. It offers a critical discussion of the underlying theory and presents a new 13-category coding scheme, ‘Tech-SEDA’. This is the first public version of a systematic, research-informed and practical tool for analysing verbal and nonverbal dialogic communication at the micro- or meso- level across diverse educational settings. Application is illustrated with examples of dialogic interaction across diverse technology contexts by learners located in classrooms or remotely – including online discussions, 3-dimensional modelling in augmented reality, software apps on tablets, Knowledge Forum, Scratch programming, interactive whiteboards, and more. The paper considers the methodological issues arising in capturing dynamic multimodal dialogue and in identifying the learning taking place.
1. Introduction
Research interest is increasing in the area of educational dialogue and the mediating role that digital technologies can play in supporting it across a wide range of settings – inside and outside classrooms. The term dialogue here refers to interaction characterised by building cumulatively on others’ ideas to jointly construct knowledge (e.g. Alexander Citation2020), and critically but respectfully challenging and evaluating multiple perspectives and reasons (Bakhtin Citation1981; Kim and Wilkinson Citation2019; Mercer and Littleton Citation2007). Learners play an active role in their own learning, within a supportive culture of mutual trust and support for risk taking (e.g. Kershner Citation2020). This characterisation is underpinned by both dialogism in which participants position themselves relatively to others and explore difference (Bakhtin Citation1981; Wegerif Citation2007) and a sociocultural perspective of situated knowledge creation within social learning communities (Vygotsky Citation1978) in which tools and objects mediate individuals’ and collaborative learning (e.g. Stahl Citation2005).
Technology mediation concerns the role of a technology tool in shaping the nature of the communication between participants (e.g. Blake et al. Citation2013), including opening up and resourcing new dialogic spaces for learning together (Wegerif Citation2007). The focus on educational technologies is driven by their increasing pervasiveness globally and by their potential affordances (perceived – supporting or constraining – action possibilities: Gibson Citation1979) for learning-focused dialogue. A seminal scoping review of 72 studies across 18 countries (Major et al. Citation2018) identified how digital technology promotes exposure to alternative perspectives, opportunities for reflective dialogues, and dialogue that scaffolds peers’ understanding. In particular, many technologies offer affordances for fostering rich new, multimodal forms of dialogue, beyond talk. Modes are sets of diverse semiotic resources for representation and communication, including image, gesture, writing, speech, and so on (Kress Citation2010).
Approaches to analysing patterns of dialogic interactions within and across multiple modes that include digital tool use are under-developed. This paper addresses the gap. Its first objective is to characterise and illustrate some of the main forms of technology mediation of dialogue and the theoretical concepts that can aid researchers in analysing how these support learning (Section 2). The second is to present and illustrate application of ‘Tech-SEDA’, an evolving new scheme for coding (i.e. systematically categorising) technology-mediated dialogic interactions across educational contexts (Section 3). The third objective is to identify how Tech-SEDA and complementary tools can mitigate the key methodological challenges and considerations in multimodal analysis (Section 4). The discussion throughout is illustrated with examples from the literature and my own and colleagues’ research over the years in order to explicate both the contributions and limitations of the coding tool. The pioneering works of Norris (Citation2004), Goodwin (Citation2018) and others have made extensive contributions towards methods for representing multimodal communication and cooperative action, and some researchers have extended these methods to analysing tech-mediated interaction (e.g. Samuelsson, Price, and Jewitt Citation2022). However, this is believed to be the first effort towards a systematic scheme for analysing multimodal dialogic communication at the micro- or meso-level; it can feed into macro-level analysis too. Critical discussion is interspersed throughout the paper, then implications and future developments are suggested (Section 5). (Note that although Tech-SEDA is introduced in Section 3, its codes that are referred to or illustrated in the article are highlighted throughout in purple italic font, beginning in Section 2).
2. Theoretical underpinnings
This section describes how technology use can mediate learning through affording opportunities to co-construct digital knowledge artefacts through multimodal dialogue.
2.1. The role of artefacts
In sociocultural theories of learning, human cognition is inextricably connected to artefacts by externalisation and internalisation processes (Ley Citation2019; Wartofsky Citation1979). The chief mechanism proposed here for collaborative learning through tech-mediated dialogue is learners, or teachers and learners, together creating and manipulating ‘digital knowledge artefacts’ (Hennessy Citation2011). These are provisional, interim records of the past and ongoing, collective activity. They externalise and embody the dialogue and thinking process, and hence the evolving knowledge and ideas as they are negotiated and cumulatively co-constructed over time. This affordance of provisionality of digital knowledge artefacts (including reversibility) is linked to the conceptions of ‘improvable’ objects of inquiry (Bereiter Citation2002; Scardamalia and Bereiter Citation2014; Wells Citation1999) and of dialogue as unfinalisable (Bakhtin Citation1981).Footnote1 To illustrate, the character limit in the ‘TalkWall’ microblogging environment (studied in Norway and England) encourages unfinished formulations and hence encourages risk taking in testing ideas – characterising a supportive culture for dialogue (Frøytlog and Rasmussen Citation2020; Omland and Rødnes Citation2020).Footnote2 These joint reference objects illuminate learning trajectories (Hennessy Citation2011). Teachers (and researchers) can gain insight into students’ preconceptions and emergent (mis)understandings through their explicit reasoning (Pifarré Citation2019; Rødnes et al. Citation2021; Sedlacek and Sedova Citation2017).
Artefacts provide symbolic, physical recordsFootnote3 of collaborative activity and ideas that can remain visible during subsequent activity (Muukkonen, Lakkala, and Hakkarainen Citation2005; Wartofsky Citation1979), extending the dialogic space beyond a single session.Footnote4 On the interactive whiteboard (IWB), for instance, archiving the activity, including any annotations or modifications, allows knowledge artefacts to be revisited later, manipulated, reflected on, commented and built on (Hennessy Citation2011; Wells Citation1999). Cognitive resources can be connected and shared across different learning activities; for example, referring back to small group discussion outcomes during whole-class dialogue (Frøytlog and Rasmussen Citation2020). Publicly highlighting students’ posts in the public arena gives their ideas status and shifts the responsibility for knowledge building to them (Rødnes et al. Citation2021). Shared knowledge artefacts ‘materialise’ the interaction between individual expertise and communal knowledge (Paavola and Hakkarainen Citation2014; Säljö Citation1995). Online written contributions can become materialised objects for further oral discussion and engagement with others’ ideas (Pifarré Citation2019; Pifarré and Li Citation2018) – within a ‘material-dialogic space’ (Cook et al. Citation2019, 228). Unlike transient oral texts, asynchronous online discussions, for example, create opportunities for thoughtful listening and reflective speaking (Lipponen Citation2002).
Co-constructing, juxtaposing and comparing digital knowledge artefacts supports learning through dialogue by making the difference or agreement between perspectives more salient, laying them open to collective critical scrutiny and reflection and highlighting their strengths and omissions (Hennessy Citation2011). The visibility and permanence of contributions in a forum or displayed on a virtual wall ‘provid[e] a visual multi-voicedness’ (Rødnes et al. Citation2021, 10). illustrates how the technology affordances of highlighting (yellow) and provisionality (repositioning at the bottom of the wall) can be exploited to focus learners’ attention on investigating the difference from other contributions (inviting coordination).Footnote5 In Ye and Pennisi’s (Citation2022) study, knowledge construction mainly occurs when online discussion participants are reading and commenting on others’ posts since they start to explore dissonance among ideas, potentially challenging them. Ultimately, learners – including new groups – integrate external useful information into their own understanding. They modify the proposed synthesis (coordination), agreeing a newly constructed meaning or problem solution (Stahl, Koschmann, and Suthers Citation2014).Footnote6 This can be adapted and validated in new situations (Ley Citation2019; Paavola and Hakkarainen Citation2014).
Figure 1. Talkwall activity 1.
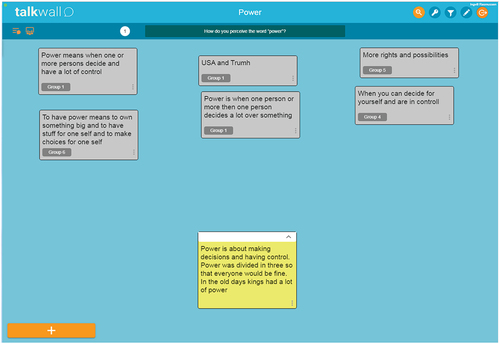
2.2. Multimodal dialogue and entanglement
Analysing tech-mediated dialogue involves examining the multiple, complementary modalities of meaning making (Lemke Citation1999) through which learners collaboratively construct their knowledge artefacts. This includes the temporal sequencing and spatial location of communication modes. Location is used in to organise the modes identified as key across the studies discussed in this article and capturable using Tech-SEDA. The modes can be distributed among multiple participants (remotely or co-located: Goodwin Citation2018).
Figure 2. Modes of tech-mediated dialogueresearchers may want to capture these episodes and artefacts too (Hennessy Citation2020).”Footnote42
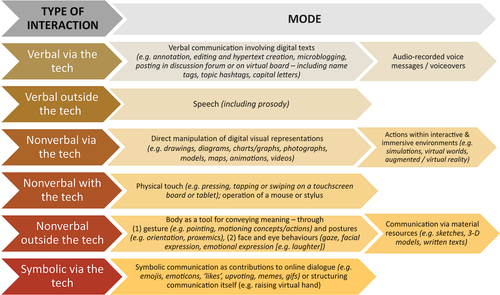
The modes can occur separately or in combination and inter-relationship (as in multimedia presentations and stories).Footnote7 They may be sequential but are often either simultaneous or partially overlapping.Footnote8 Importantly, these diverse semiotic resources are interlinked, continually reshaping the communication and mutually elaborating each other (‘intertwined semiosis’: Goodwin Citation2018, 227) as they are compiled into new, locally relevant multimodal packages. In the example in , individual contributions posted on a Google JamboardFootnote9 [verbal via tech] are subsequently discussed orally by the group [verbal outside tech], generating two common themes. Learners’ talk [verbal outside tech] overlaps with their repositioning of contributions [nonverbal via tech] to form two thematic clusters sorted under overarching theme labels; talk is hence important here (and commonly) in shaping the artefacts, which are ‘talked into being’ (Floriani Citation1993, 242).
Building on the work on multimodality, a helpful theoretical lens informing the analysis of methodological issues in this paper is the socio-materialist notion of entanglement, whereby technology is construed as one of multiple interdependent aspects of a complex learning environment, alongside humans, pedagogical activities and digital and/or other material learning resources (Fawns Citation2022; Hodder Citation2012). Drawing on Barad’s (Citation2007) ‘new materialist’ perspective, in which the boundary between meaning and matter is undefined and they are entangled, a dialogic space and engagement with a technology and other resources in the specific sociocultural environment are likewise interconnected and affect each other (Cook et al. Citation2019; Hetherington and Wegerif Citation2018). The blurred boundary between the material and dialogue is illustrated by Cook et al. through an extended example of learners iteratively repositioning posts on a shared class ‘feed’ in Talkwall, categorising their daily actions as sustainable or unsustainable (); online contributions were shaped by oral contributions and vice versa, including verbal and nonverbal challenges (e.g. one student repositioning his peer’s feed indicated his disagreement).Footnote10
Figure 4. Talkwall activity 2.
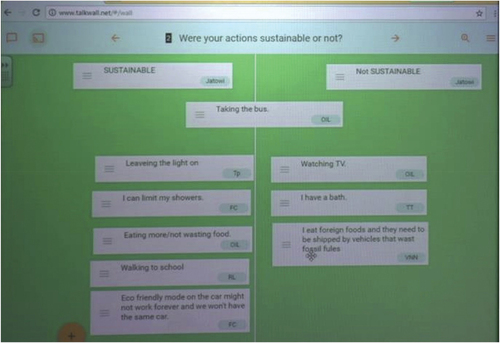
Another, unusual example comes from a New Zealand study of vocational students studying electrical engineering (Simpson Citation2022). Sharing digital photographs of their circuit boards under construction () with their peer group through Facebook Messenger in order to troubleshoot was a highly effective way of students explaining the concepts and questions they were struggling with. Here, seeking assistance with the developing artefact was a form of invitation to build on ideas. The dialogic space in the physical workshop extended into the digital space: ‘images of students’ work became dialogic tools as points of shared knowledge, inquiry, and reflection’ (Simpson Citation2022, 135). In sum, use of tech within learning entanglements provides opportunities for – and can shape – dialogue and meaning making, and vice versa.
Figure 5. Circuit board photograph (Simpson Citation2022).
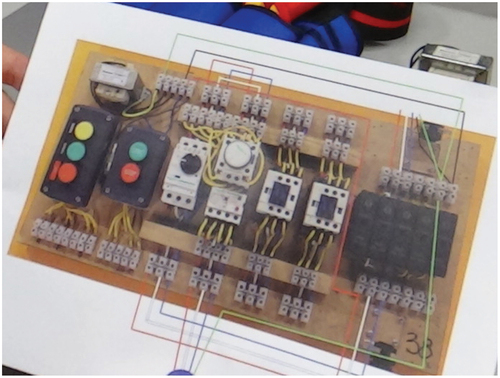
Nevertheless, the notion of technology and other materials having an active voice within the dialogue (Cook et al. Citation2019; Hetherington and Wegerif Citation2018) needs cautious interpretation. Technology has no agency nor intrinsic dialogicality of its own, and its affordances for dialogue are pre-determined neither by the teacher nor the tool’s functionality (Jeong and Hmelo-Silver Citation2016). Affordances (and constraints) are fluid and emerge through interaction with technology in situ within a specific learning context; researchers need to examine the enacted affordances (Major et al. Citation2018). The effective mediating role of a technology, and the pathway that enactment of its affordances for dialogue takes, depend heavily on:
learner factors – understandings, agency and their social configuration (Simpson Citation2022);
teacher factors – (a) understanding of key technology affordances for dialogue (Amodia-Bidakowska Citation2020; Jeong & Hmelo Citation2016) and (b) dialogic intentions and actions to facilitate and scaffold learning (e.g. Hmelo-Silver, Lakkala, and Hakkarainen Citation2005; Warwick et al. Citation2020), including setting cultural expectations for collective accountability (Kumpulainen and Lipponen Citation2010; Michaels, O’Connor, and Resnick Citation2008), for example obliging learners to contribute posts (Rødnes et al. Citation2021) or, conversely, dedicating time to review and respond to peers’ contributions without adding (Hay Citation2022);
pedagogical design – including structure/openness of the activity (Boyd and Rubin Citation2006; Hetherington and Wegerif Citation2018) – e.g. whether it involves collaboration towards a joint artefact;
learning ethos – a supportive culture of conjecture, trial and error (e.g. exploiting the provisionality affordance), and learners’ readiness to contribute (‘talk culture’: Alexander Citation2018, 22);
technology designer’s intentions and choices.Footnote11
These factors collectively set the scene, shaping technology use and the nature of dialogue taking place around/through it. While some opportunities afforded by technology mediation can occur without it, technology’s presence can facilitate new forms of learning through dialogue and hence alter the teacher’s own mediating role, for instance towards one of devising and facilitating appropriate activities to optimally exploit the specific technology’s affordances. However, the factors do add to the methodological complexity of analysing multimodal dialogue holistically.
3. Methods for analysing multimodal dialogue
This is a rich and fast-developing field. This section summarises methods available that can be used alone or in combination and then contributes a new tool that can be used alongside.
3.1. Overview of methods
Data capture and analytic methodsFootnote12 available to help identify the supportive affordances of the particular technology and context, and to analyse the process dynamics and outcomes of multimodal interaction taking place, include:
Audio/video recordings, screenshots, screen recordings or automatic server-log data capture;
Automatically capturing chronology of events through timestamps enhances most methods, especially showing relationships between dialogue in different modes;
Specific metrics, e.g. numbers, types, lengths and temporal distribution of contributions, number of posts viewed or responded to (Wise et al. Citation2012), listening reflectivity (revisiting earlier posts, predicting richer argumentation: Wise, Hausknecht, and Zhao Citation2014), active participation levels (names, click logs and visualisationsFootnote13: Paulus (Citation2005); Stahl et al (Citation2014);
Multimodal learning analytics offering predictive modelling of learning outcomes (Cukurova, Giannakos, and Martinez‐Maldonado Citation2020; Emerson et al. Citation2020; Olsen et al. Citation2020);
Using a coding scheme to systematically categorise transcripts or videos of interaction sequences – including interactions with artefacts – in order to identify specific functional dialogue elements (see 3.2);
Mixing qualitative and quantitative analysis methods, including frequency counting after coding, sociocultural discourse analysis (Mercer, Citation2004) combining quantification (e.g. concordance analysis) with in-depth qualitative analysis, and network analyses;
Contribution quality can be coded or rated for clarity, criticality, relevance, reference to discussion, evidence, etc. (Paulus Citation2005; Wise, Zhao, and Hausknecht Citation2014).
Thematic analysis (e.g. of affordances or student engagement in inquiry), with/without critical incident analysis illuminating how/when multimodal dialogue moves thinking forward.
Interviewing participants, often using stimulated recall or think-aloud protocols, can help to access thinking and reduce inference (see 4.1), as can combinations of the research methods listed above.
Coding is the main focus here because it is a demonstrably powerful and prevalent tool in non-technology contexts that permits: efficient searching and reducing large datasets to highlight key dialogue markers, and useful comparisons across settings and timepoints (Hennessy et al. Citation2020). While coding has its roots in qualitative analysis approaches (for example, multiple codes may be simultaneously applicable), mixed-method approaches are increasingly popular. Hennessy, Bouton and Asterhan (Citationin press) outline several available approaches. Besides (a) capturing and integrating qualitative and quantitative data, (b) multi-level approaches combine analytic units of different grain sizes (e.g. turn, episode and lesson), (c) coding can be bottom-up and then top-down, or vice versa, and (d) frameworks for analysing disciplinary knowledge can be integrated with coding the dialogic functions and forms of turns.
Section 3.2 describes how the need for a coding scheme specific to multimodal dialogue was addressed. Coding can be applied to any communication modes including interactions with or captured within artefacts. Nonverbal contributions of images or symbols and direct manipulations of artefacts (e.g. grouping contributions: ) can themselves be coded for dialogic function; Sections 3.2 and 3.3 offer several illustrations. In some cases, gestures and oral contributions can only be understood in relation to an interaction with technology or to a visual artefact (as in , where interpreting and coding the child’s invitation for reasoning ‘Why do you think that could eat that?’ depends on seeing the indicated food chain components). The artefacts serve a pivotally important role in rendering the externalised dialogue, decision-making and reasoning processes, and substantive (disciplinary) knowledge under construction, available to researchers for analysis and interpretation.Footnote14 Coding can inform or be used alongside the other methods listed above plus several further methods that are particularly helpful in integrating dialogue data to create thick, multimodal descriptions, as illustrated in 3.3 and 4.4: discussion thread analyses, multimodal analysis tables, chronological event maps, interactional ethnography and network analyses.
3.2. Development and application of tech-SEDA
Tech-SEDA allows researchers to explore questions such as the added value of particular technologies in supporting learning through dialogue, how tech mediation supports specific dialogue forms (e.g. whether revisiting artefacts supports more ‘reference back’ and synthesis of others’ ideas), and which forms are productive for learning. It builds on previous coding frameworks developed by our team, beginning with the fine-grained (33-category) SEDA (the Cam-UNAM Scheme for Educational Dialogue Analysis [SEDA] developed by Hennessy et al. Citation2016). SEDA was theoretically informed through a comprehensive review of relevant studies (themselves based on decades of classroom observation and teacher report) and established coding schemes (e.g. Michaels, O’Connor, and Resnick Citation2008; Wells and Arauz Citation2006) followed by iteratively developing and empirically testing categories that synthesised the core elements of productive dialogue from the studies. Despite variation in application, the broad themes underlying the resulting codes are prominent within the literature internationally. SEDA was adapted by Howe et al. (Citation2019) and condensed into a larger-grained (12-category) scheme (CDAS). These schemes were adapted further to create Tech-SEDA, through selecting and combining 13 codes found to be pertinent to tech-mediated multimodal interaction ().
Figure 6. Tech-SEDA categories.
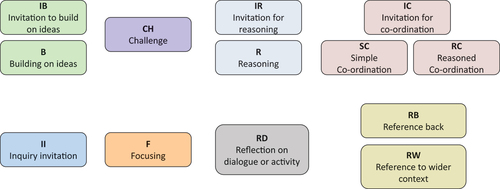
Like SEDA, Tech-SEDA was iteratively developed over several years through (a) reviewing the literature on technology affordances for dialogue and sociocultural theory concerning the role of artefacts in learning, and (b) hands-on workshops and discussions with colleagues in the field during multiple rounds of coding episodes, this time aiming to pinpoint the most pertinent elements of tech-mediated dialogue. Outliers were reconciled through progressively reformulating categories. After applying CDAS to multiple examples of technology-mediated dialogue from the group’s research, 10 codes remained applicable and useful,Footnote15 but to ensure comprehensiveness, dialogic elements potentially missing for multimodal contexts were also explicitly sought. One original fine-grained SEDA code that emerged as particularly pertinent was Focusing; a term originally used by Wood (Citation1994) to denote a form of scaffolding where the teacher draws attention to critical task features which might not yet be understood, it is now applied to exploiting the features of some technologies to spotlight or zoom in on specific objects, guiding learners by directing their attention in a particular direction (Hennessy Citation2011). A further code, Reflect on dialogue or activity, was derived from collapsing three related, fine-grained SEDA codes, in order to capture the metacognition deemed important for learning in the literature (and already included in another scheme devised for practitioners by the T-SEDA Collective Citation2023: the Toolkit for Systematic Educational Dialogue Analysis). Collaboration with colleagues in Hong Kong working on knowledge building with the ‘Knowledge Forum’ (KF: see Glossary of technologies) environment identified a gap when applying earlier Tech-SEDA versions to their lesson exemplars, giving rise to a third additional code, Inquiry invitation – reflecting the predominant stimulus for dialogic inquiry (Tong and Chan Citation2020; Yang, van Aalst, and Chan Citation2019).Footnote16
While the Tech-SEDA category names and definitions are adapted from its predecessor schemes, all applications are related to tech-mediated dialogue. Since the scheme needs to apply across diverse contexts, a wide range of technology examples is used to illustrate it; these reflect the data at hand from the researchers in our network contributing to the scheme. Examples include online discussions, 3-dimensional modelling in augmented reality, software apps on tablets, Knowledge Forum, Scratch programming, interactive whiteboards: see Appendices A and B. The premise was that the principles of dialogue remain the same when technology tools are used, but they manifest themselves differently, in particular when artefacts are co-constructed and multimodal forms of dialogue are present. The code definitions capture both verbal (not necessarily oral) and nonverbal communications, although applying a dialogue code needs to reach a certainty threshold level that is harder to reach without any language. Nonverbal modes of communication and action with/via the technology environment may well support identification of the dialogic act, however. For example, some online dialogue activities include nonverbal forms of building on and challenge as learners reposition others’ posts (as in Cook et al. Citation2019), along with verbal examples of these plus reasoning, as illustrated by the coding applied in to Hay’s (Citation2022) example from a group of teenage students in England.Footnote17 In Tech-SEDA, such examples are integrated with any verbal dialogue captured, however when using the scheme for analysis, a multimodal analysis table (see 4.4.1) may well juxtapose the modes in two or more separate columns. Pifarré (Citation2019, 10) uses two columns in her illustration of similar repositioning in the synchronic shared space cacoo.com. revisualises her example of Spanish students reviewing, annotating and grouping related ideas posted, to show temporal sequencing and application of Tech-SEDA coding: focusing, simple coordination, building on and challenge.Footnote18
Figure 7. Padlet discussion of ‘was odysseus a good leader?’ (Hay Citation2022).
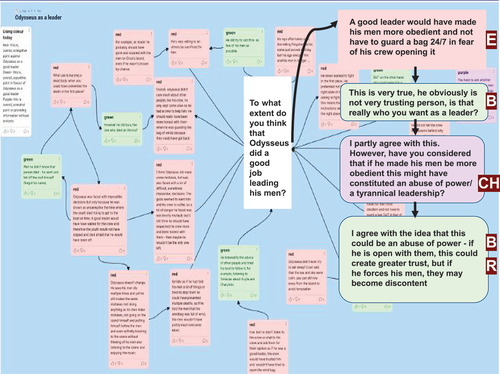
Figure 8. Brainstorm on selecting a playground wall design theme, adapted (with consent) from Pifarré (Citation2019), excerpt 1.
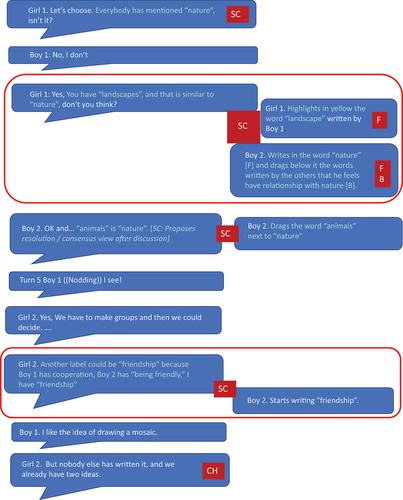
An earlier publication discussed in depth some key methodological considerations in developing systematic coding schemes for analysing educational dialogue (Hennessy et al. Citation2020); these are revisited here in order to clarify the parameters and robustness of Tech-SEDA for potential users. The scope of Tech-SEDA is broad in terms of applications spanning varied educational levels (primary, secondary, tertiary), different subject areas, and interaction by pairs, groups or a whole class (including between teachers). In essence, as with its underpinning schemes, the scope is propositional or invitational dialogic acts, any of which in principle can be by learners and/or educators. The level of granularity at which codes are specified is moderate. However, Tech-SEDA distinctively focuses entirely on technology use settings. When dialogue is multimodal, the researcher chooses which information (from which communication modes) to code.
Systematically analysing interaction requires criteria for segmentation, determining the unit of analysis. The Ethnography of Communication (Hymes Citation1972; Saville-Troike Citation2003) proposed a useful foundational system of nested analysis levels. Micro-level ‘Communicative Acts’ (CA) are identified by their interactional function and embedded within meso-level ‘Communicative Events’ (CE), where participants, participant structure (class, group or dyad), purpose, task, orientation and/or general topic remain constant (see ). In turn these are elements of broader, macro-level ‘Communicative Situations’ (CS), namely the general communication context (e.g. a lesson or lesson sequence).
Figure 9. Hierarchical and nested categories in the Ethnography of Communication.
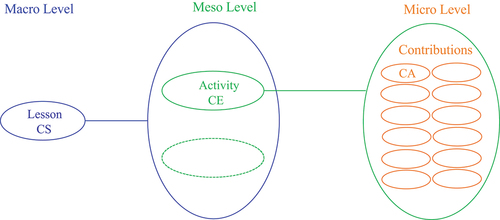
In research on verbal dialogue the unit varies but it is typically the turn or utterance (Hennessy et al. Citation2020). Meso-level units related to Hymes’ criteria for CE are also commonly employed (Kumpulainen and Rajala Citation2017; Nystrand et al. Citation2003; Sedova, Sedlacek, and Svaricek Citation2016; Wells and Arauz Citation2006). Some instead define chunks by a particular time window (e.g. 10-minute lesson segments: Schindler et al. Citation2021) or a specific number of turns/lines (Lefstein, Snell, and Israeli Citation2015; Shaffer Citation2017). Levels can be combined (Soter et al. Citation2008). Tech-SEDA likewise can be used in these various ways. Micro-level coding of individual turns is illustrated in . Bigger chunks make it easier to interpret individual contributions as parts of the larger collaborative discourse and activity (Stahl Citation2002), however, and this is likely to be more fruitful in multimodal contexts. illustrates meso-level coding of multimodal interaction too: three instances of simple coordination each span more than one contribution and mode. Two of these (circled in red) span participants too; in each case, Boy 2 enacts what a peer suggests orally. Note that while the examples in this article depict post priori coding, selected codes could alternatively be applied to live observation as supported by our templates for tallying or time sampling,Footnote19 although this is impractical with multiple modes and/or codes.
Validity of coding schemes is a neglected but important consideration, to determine whether the categories represent key constructs that the relevant research community would recognise. Here, construct validity was maximised through having rigorously mapped the codes in its predecessor schemes onto research literature theorising, operationalising within other schemes, or empirically demonstrating the most productive dialogue forms. Theoretical underpinnings of 12 Tech-SEDA codes are detailed in our three earlier publications (cited above). The final code, Inquiry invitation, has a very strong theoretical foundation in the collaborative knowledge building literature (e.g. Yang, van Aalst, and Chan Citation2019). For example, the discourse coding framework developed by Yang et al. (Citation2016) based on knowledge-building inquiry principles clearly resonates with prototypical dialogue elements and thus our coding schemes (Explanation, Explanation-seeking, Elaboration, Metacognitive statement, Metacognitive question, Synthesising notes, Negotiating a fit with community ideas – including (dis)agreement). However, Tech-SEDA’s focus only on technology use has added an extra validation layer through application to tech-mediation contexts, including coding relevant published or donated external examples.Footnote20 The empirical trialling and refinement served to maximise ecological validity.
Inter-coder reliability was tested through the author and an assistant independently coding seven dialogic episodes from two projects, yielding an overall Cohen’s kappa coefficient of 0.817 and a range of 0.722–1.00 for individual codes (excluding RC and RW where frequencies were too low to calculate kappa).
3.3. Charting knowledge building in online discussion and inquiry environments
Discussion environments afford dialogic interaction because it is easy to not only comment on and cite another’s post, but also to quote it by pasting excerpts of their earlier message within the current post, or by responding explicitly to their specific ideas (reference back, then building on or challenging). Following discussion threads to see how much quoting takes place allows researchers to examine the ‘dialogic resonance’ that activates affinities across utterances (Du Bois Citation2014, 359). This method can capture micro-level incremental knowledge-building activity over many weeks; in KF, inquiry thread analysis includes how participation is distributed within each thread and how ideas are improved and integrated across threads (Yang et al. Citation2016). In Muukkonen et al’.s (Citation2005) experimental study, analysis showed that none of the 1893 ideas logged by the 17 Finnish psychology students in the non-technology condition referred to another participant’s contributions, although they met weekly. In the tutored-technology condition, by contrast, 10.7% of the 1,353 ideas posted by 17 students using the Future Learning Environment (FLE) included direct quotes from other students’ posts, asking for clarifications (inviting building on) and requesting explanations (inviting reasoning). Tracing the deepening interlinked threads highlighted how theories and problems were reformulated, understanding increased, and participants evaluated the collaborative process (reflection on dialogue) or built on the content-specific issues of preceding messages.Footnote21
The analysis can be conducted manually, as in the example in Footnote22 from a recent practitioner professional development course led by the author. Yellow highlighting denotes the parts of contributions that go on to be quoted, revoiced or interpreted (building on/reference back) in the segments in blue. Individual and group trajectories can be elicited. Discussion thread analysis can be combined with other methods including dialogue coding: Tech-SEDA codes applied are shown on the right side. Seven different codes apply to just four posts, illustrating the dialogue’s richness. The reflection on the preceding dialogue and provocative question in the third post are followed in the final post by reasoned coordination – rare in classroom talk (e.g. Howe et al. Citation2019), yet a pivotal element of dialogue for learning that developing digital artefacts in a discussion forum like this may encourage and portray. Together, the discussion thread analysis and dialogue coding indicate the ‘speaking quality’: posts that are clearly expressed and argued, critical, and connected, generating insight and understanding (Rovai Citation2007).
4. Methodological challenges in analysing multimodal dialogue using tech-SEDA
This section outlines some key challenges that Tech-SEDA can address, and, acknowledging the limitations of any one tool, suggests how complementary multimodal and multilevel approaches may also be used.
4.1. Level of inference in interpreting nonverbal modes
In tech-mediated dialogue there is often less need for oral explanation/interaction and more nonverbal interaction (e.g. drawingFootnote23 or touch actions on a screen), longer pauses and less talk than in contexts without technology (Hennessy Citation2020; Jordan and Henderson Citation1995; Samuelsson, Price, and Jewitt Citation2022).Footnote24 The inference level is thus likely to be higher and confidence in coding lower. Visibly explicit contributions that are more easily categorised using Tech-SEDA include annotated digital objects and posts to a discussion (‘speaking’ or externalising ideas: Wise, Hausknecht, and Zhao Citation2014), whereas ‘listening’ (taking in others’ externalisations by accessing their contributions: Wise et al.) is largely invisible in any dialogue mode. Despite lacking verbal clues to the participant’s intention, nonverbal actions and responses to them are chained, as in talk; what precedes each and what is anticipated to follow signify its meaning (Alexander Citation2020; Bakhtin Citation1981).
In particular, validity is higher where an oral contribution shapes an action’s meaning (e.g. before/after moving an object, learners discuss repositioning, as in ). Who is in control also plays a role in the interpretation. In one science lesson observed by Warwick et al. (Citation2010) where three primary school children created a food chain on an interactive whiteboard (),Footnote25 the somewhat dominating boy executed his own suggested actions while orally explaining (reasoning) but not always awaiting agreement from the two girls. The children verbally and non-verbally challenged each other’s positioning: one girl undid the boy’s action, replacing a frog picture with a caterpillar. After his further, oral challenge, they jointly removed the caterpillar and continued a dialogue around co-constructing the chain (e.g. the boy invited reasoning as he gestured towards two of the organisms: ‘Why do you think that could eat that?’).
Lower inference of verbal contributions via the tech is even more radically reduced when the environment builds in highly structured scaffolding for making collaborative idea development and metacognitive reflection explicit. Some predominantly text-based environments require learners to label their contributions using a drop-down menu, helpfully rendering them visible to the researcher (albeit unnaturalistic). Coercing particular interaction patterns and negotiation of joint meaning (as with Negotiation Tool [NTool]: Beers et al. Citation2005; Kirschner et al. Citation2008) thereby structures the analysis.Footnote26 KF includes scaffolds to help learners track, synthesise and metacognitively reflect on trajectories of idea development [reflection on dialogue], e.g. ‘My theory’ and ‘I need to understand’ (encouraging students to pose questions to others, i.e. inquiry invitation), and ‘What we understand now’ (Yang et al. Citation2016).
A number of built-in tools additionally support ‘meta-discourse’ whereby learners periodically review and evaluate their collaborative knowledge‐building discourse, including identifying critical incidents and changes in thinking, ultimately connecting a ‘rise-above’ note to selected, related ‘reference notes’. An example of such a note is:
We [initially] thought design can solve problems but it has diverse purposes and meanings, such as the meaning of the design process, the purpose of conveying designers’ ideas. Artifacts design is one of the ways for designers to express their ideas.Footnote27
Meta-discourse links directly with Tech-SEDA codes: reasoned coordination and reflection on dialogue. The scaffolds support researchers in analysing the diversity of ideas engaged with through reading others’ posts (breadth of listening) and the levels of response to and interpretation of others’ ideas, or depth of listening (Wise et al. Citation2012).
Learners’ reflections are recorded in the system for researchers’ easy access, although any other data forms still need manual integration. Innovative and sophisticated analytic tools in the Knowledge Building Data Analytics Center (KBDAC) dashboard inform both researchers and learners, including identifying which scaffolds have been used and how many of an individual’s notes have been read, modified and/or built on. Each note is pre-labelled with a theory-building scaffold (including ‘build-on’ and ‘this theory cannot explain … ’ used to challenge a peer’s theory, or ‘a better theory’ used to justify an alternative [reasoning]), again reducing the researcher inference level. While the default set of scaffolds largely maps onto Tech-SEDA codes, they are customisable. Options could be built into or generated bottom-up within other tech environments too, as in Chan et al’.s (Citation2023) study of pre-service teachers in Hong Kong devising dialogic prompts (e.g. ‘Can you explain more? [invitation for reasoning]; ‘I agree with you, but I also think…’ [challenge]) to scaffold their Moodle discussion forum reflection tasks.
Symbolic representations likewise help to make dialogic interaction explicit. The students in Hay’s aforementioned (Hay Citation2022) study developed an innovative set of emojis to add to Padlet posts in order to build a trusting culture and soften the impact of cognitive challenges. This in turn added extra information for the researcher concerning participants’ nuanced intentions () and generated sub-categories of challenge during online learning to address in more depth the research focus of developing critical engagement with others’ ideas.Footnote28 Structure and focusing provided by tasks and teachers/facilitators (e.g. ) can also serve to reduce inference in Tech-SEDA coding. For instance, colour coding of post-it notes could be used by learners on a virtual board to signify whether their statements are building on or challenging existing posts.Footnote29 In Liu’s (Citation2023) study, an innovative Chinese primary teacher asked her class to show how they were learning from peers’ ideas that differed from their own by adding voice messages to explain what each step meant in the peer’s number sentences in the zoomabc environment (invitation to build on).
Figure 12. Emojis to accompany challenges (Hay Citation2022).
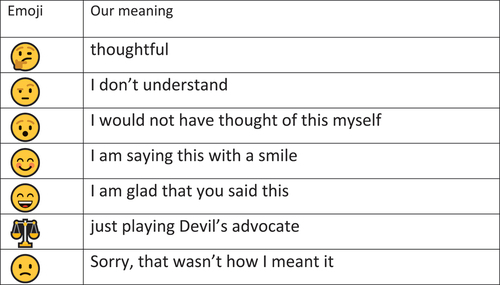
4.2. Challenge of interpreting multiple, entangled interaction modes
Tech-SEDA is designed for analysing dynamic multimodal dialogue as it progresses, including how interaction with tech shapes the subsequent actions and interactions outside as well as via the tech. Some applications illustrate how direct action with the tech such as touch may, like talk, significantly change the artefact and interactions between participants moment-by-moment (McGann, De Jaegher, and Di Paolo Citation2013; Samuelsson, Price, and Jewitt Citation2022). Moreover, gestures can convey the learner’s thinking (Goldin‐Meadow Citation2009) and aspects of meaning (either concrete objects/actions or more abstract concepts: Janin Citation2023) that are present or absent in other modes. They constitute a semiotic modality in their own right (Goodwin Citation2003, Citation2018), orchestrating the talk, digital resources and participation into a coherent whole (Fernández-Cárdenas and Silveyra-De La Garza Citation2010).Footnote30 Gaze and how teachers distribute their focus of visual attention among students – encouraging responses to questions and opening space for discussion – have also proven pivotal in some studies (Muhonen et al. Citation2020; Olsen et al. Citation2020).
Video recording is invaluable here. Some researchers first review their video recordings without sound to focus on the embodied aspects of interaction, then layer on the verbal communication (Wilmes and Siry Citation2021). However, the semiotic material layers are not additive but transformative (Goodwin Citation2018). The inextricably entangled communication modes create a rich experience that could be considered more than the sum of its parts, but making sense of these different semiotic resources and their role in learning requires iterative analysis. This makes the researcher’s job much more demanding than where dialogue is mainly talk-based (Samuelsson, Price, and Jewitt Citation2022). Modes may become more or less salient or relevant in the ebb and flow of dialogue, triggering attention switching and sometimes missed communication (Thorne and Hellermann Citation2022). This continually reshapes dialogic activity (Goodwin Citation2018), making it unpredictable. Given the blurred boundaries between dialogue and digital and physical material resources, the challenge is how to analyse parallel or intertwined interaction modes to determine the nature and direction of influences. In the interaction in , the latter are fairly easy to infer from the contributions’ content and timing, however when some technologies are used asynchronously, ascertaining which contributions students are looking at is impossible unless they add comments (Hay Citation2022). Moreover, while many technologies including Padlet allow export of a time-stamped record of contributions, they do not show when modifications, repositioning, links or comments were added. Screen recordings are needed to capture the real-time action sequence.Footnote31 Finally, tech-mediated interaction lag time can disrupt the activity (Thorne and Hellermann Citation2022), confounding the analysis.
4.3. Entanglement means that selecting the unit of analysis becomes complex
Nonverbal interactions with digital tools and artefacts can themselves each be conceptualised as an ‘utterance’ or ‘turn’ in dialogue (Jordan and Henderson Citation1995; Samuelsson, Price, and Jewitt Citation2022; Tomasello Citation2003), as in the above example of repositioning another’s post on a virtual board (challenge).Footnote32 The notion of a ‘composite utterance’ (Enfield Citation2009) combining multiple information sources, especially speech, gesture and gaze, is also helpful though, since each mode can be enriched by its co-occurrence with the other.Footnote33 However, temporal sequencing is again clearly all-important and even within one mode, contributions may appear sequentially (e.g. in an online discussion forum) or synchronously (e.g. on a virtual wall or board like Padlet, Talkwall, Miro or Jamboard).Footnote34 In multimodal dialogue, the turn boundaries are often much less easily distinguishable. For example, one learner may instruct a peer to manipulate an app on a tablet; timings of the speech turns and nonverbal action turns may not match. In , we saw that some written contributions and nonverbal actions coincided with associated speech turns and others took place in between or overlapping them.
The challenges outlined (4-1-4.3) have several implications for researchers who must decide which granularity level(s) to use and which modes to analyse – together or alone. Coding CA in modes selectively or separately can help to disentangle the modes, yet coding in one mode (e.g. artefacts such as contributions to a drawing on a display board or computer) using another (e.g. associated talk or gesture) to inform the interpretation decreases inference. The temporal dimension of chained or overlapping multimodal contributions additionally means that (as with analysing talk alone) researchers must interpret any CA in light of those preceding and surrounding it, and often looking across modes. It may be far easier and more fruitful to use the meso-level CE in many multimodal contexts so as to capture all relevant information. Pifarré (Citation2019), for instance, identifies episodes in terms of phases (‘facets’) of computer-supported co-creation in a STEAM project. She finds that students’ movement between phases – for example, from generating ideas through brainstorming and information gathering to fusing ideas through grouping and labelling them – can be signified by different nonverbal actions (e.g. gaze and sketching in the shared digital space). The most meaningful interaction unit and which modes to include depend on research purpose and context, as always, and also on the number of modes and whether they overlap. Some trial and error may be apt to explore the implications of available options.
It should be noted that capturing the degree to which cultural norms and protocols for dialogue are successfully developed (Hofmann and Ruthven Citation2018) necessitates moving towards a more macro-level analysis and one that includes the affective, social and ethical characteristics of classroom communication, alongside cognitive elements. For instance, sentiment analysis uses natural language processing and computational linguistics to identify emotional state (Ilic Citation2020), allowing us to go beyond binary classifications of ‘likes’ etc.; however, application to dialogic interaction, and multimodal sentiment analysis, are both undeveloped.Footnote35 Rating scales are particularly useful in distinguishing authentic and superficial student involvement. Our own team used a (macro-level) 3-point scale to rate students’ active participation across a whole lesson, finding high ratings significantly associated with subject learning gains when combined with particular forms of dialogue – building on and challenge (Howe et al. Citation2019). Rating scales can also be fruitfully applied to CEs in digital environments: for example, Lee et al. (Citation2006) rated depth of inquiry and depth of explanation.
4.4. Multimodal, multilevel analysis
Various techniques can be used to process Tech-SEDA coded data further in ways that address both challenges outlined in 4.2 and 4.3, including capitalising on the benefits of integrating dialogue data across different analysis levels.
4.4.1. Multimodal analysis table
Data captured from different verbal and nonverbal communication modes are commonly and usefully displayed in different columns in a multimodal analysis table. These may include images of digital artefacts or embodied actions and gestures represented with de-identified frames extracted from videoFootnote36 ().
Figure 13. Simple multimodal analysis table (S. M. Bridges Citation2020).
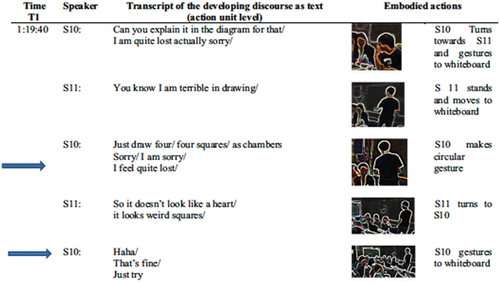
Additional columns may include dialogue codes (e.g. Tech-SEDA: , column 9), analytic commentary, themes, affordances or critical incidents identified, subject vocabulary used, or other learning markers. Integrating the outcomes across the different analyses often produces an interpretative narrative that spans the whole session (CS), enriching coding and offering a holistic picture of how technology use mediates the dialogue.
Figure 14. Sample full multimodal analysis table.Footnote43
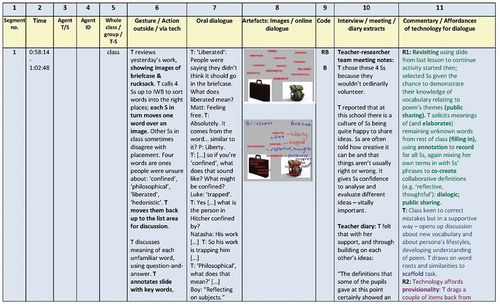
4.4.2. Event time plots
Multimodal layers can alternatively be represented in parallel along a horizontal timeline (Jordan and Henderson Citation1995). Event time plots can graphically illustrate the frequency, density and collocation of specific (Tech-SEDA) codes. They visually chart progression and shifting activity trajectories, relating these to learning (Falloon Citation2013; Lefstein, Snell, and Israeli Citation2015; Nystrand et al. Citation2003). In multimodal transcription, spatial layout highlights relationships between participants and activity modes, and overlapping modes (Cowan Citation2014; Wohlwend et al. Citation2017).Footnote37 Selected video stills make salient information about the interaction that would otherwise require wordy description, such as gaze or proxemics – distance between participants and from relevant objects (e.g. ).
4.4.3. Network analysis methods
Socio-semantic network analysis methods powerfully represent patterns of connection between coded acts or participants in dialogue. Epistemic network analysis (ENA: Shaffer Citation2017) is a quantitative ethnographic method combining qualitative analysis with statistical modelling of connections made by participants between an expressed idea and those in its recent temporal context.Footnote38 Researchers can create discourse pattern visualisations and test for learning gains (e.g. Cao Citation2022; Mochizuki et al. Citation2022). This innovative tool can integrate (Tech-SEDA coded) verbal and nonverbal communication, as in : in a secondary school art and design lesson (Gomez Citation2021),Footnote39 verbally proposing new ideas (SG.CON) and building on ideas (SG.ELAB) by students in the high creativity student group (red network) were strongly linked to drawing out ideas or a plan – explaining ideas (nonverbal reasoning) to peers while co-constructing artefacts using augmented reality software.Footnote40
Figure 16. Designing a 3D model of school library furniture using grib 3D (Gomez Citation2021).
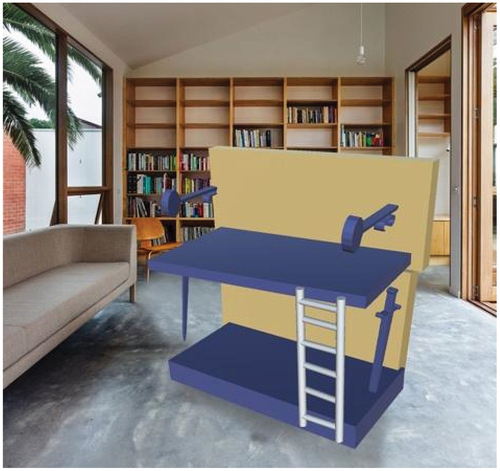
Figure 17. Epistemic network comparing high and low creativity groups (Gomez Citation2021).
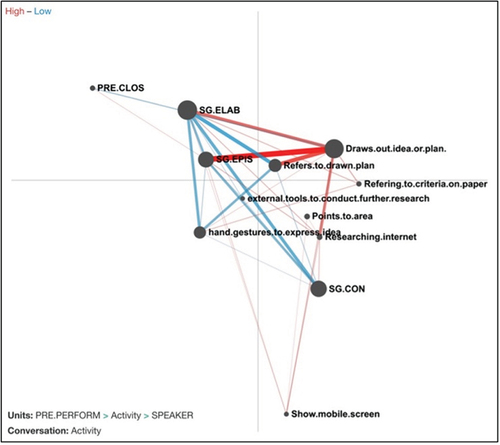
ENA is underpinned by social network analysis (SNA) which can represent technology mediation of the complex dialogic relationships between participants. KF tools include Knowledge Building Discourse Explorer (KBDeX), a SNA tool (Oshima, Oshima, and Saruwatari Citation2020) showing who referred back to and built upon whose ideas and the strength of connections between topics, including measuring collective responsibility (cf. Michaels, O’Connor, and Resnick Citation2008). Researchers can examine change (knowledge building) over time as ideas become visibly joined into more complex discourse networks (reasoned coordination) as in . SNA could be used after coding with Tech-SEDA as a macro-level measure of participation equity. It might combine verbal and nonverbal communicative acts.
Figure 18. Network changes in knowledge forum (C. Chan et al. Citation2019).
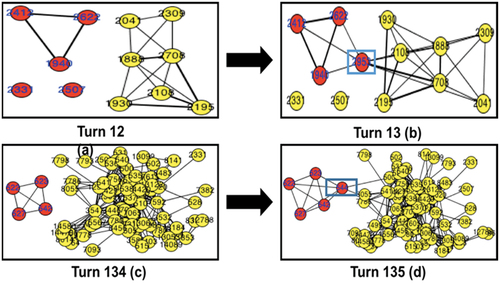
4.4.4. Interactional ethnography
One final, powerfully illuminating, albeit complex, method addressing all three challenges discussed is interactional ethnography (Green and Bridges Citation2018; Jordan and Henderson Citation1995): holistically analysing the ‘intertwined chains of action, dialogue and multimodal texts that are locally and collaboratively constituted and reconstituted as they are guided and scaffolded by an expert facilitator’ (S. M. Bridges et al. Citation2020, 308). It traces knowledge development and actors’ agency through multiple levels of iterative, recursive and abductive microanalysis, exploring connections among events, artefacts, key texts and actor configuration. Bridges et al. (Citation2019) constructed a detailed event map of a problem-based learning (PBL) cycle of inquiry by medical students in Hong Kong studying a hole-in-the-heart clinical case (). Students used various technologies to generate digital texts and diagrams, building collective understanding in this rich dialogic space. Interactional Ethnography is very time consuming but could complement Tech-SEDA to provide deep understanding through tracing in-the-moment knowledge artefact construction.
Figure 19. Artefact evolution across one PBL cycle.
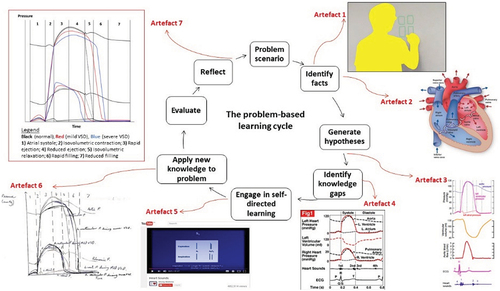
5. Implications and future developments
This work has added to understanding in the field concerning how technology use can extend and mediate dialogic practice, and how researchers can analyse the ensuing multimodal interaction. It has produced and illustrated a pioneering coding scheme. Tech-SEDA provides a practical research tool that is informed by dialogism and sociocultural learning theory in which participants orient themselves to others’ perspectives and construct new knowledge through creating and manipulating (digital) knowledge artefacts, as illustrated through the examples and references in Appendix A. Complementary methods have been discussed that can help mitigate impacts of the methodological challenges outlined above (especially pertaining to entanglement). Questions that can be addressed include what is the developmental trajectory of specific knowledge artefacts and who participates in their development? How does the digital medium augment and transform oral interaction?
Tech-SEDA was developed with input from multiple colleagues; a limitation is that the current incarnation suits the contexts we tested it against to date. However, the scheme, like its predecessors, is eminently adaptable for use by other researchers in diverse contexts and for new purposes; it will continually evolve as new technologies and research agendas emerge. The scheme and related methods have implications for developing (a) technology environments themselves (e.g. discussion forums and similar technologies often lack facilities for synthesis or creating shared outputs) and (b) pedagogy (e.g. encouraging metacognitive reflection) to support higher quality dialogue. A related limitation is that researchers may be interested in additional aspects of interaction and learning besides the scheme’s 13 theoretically and empirically derived core codes. Some have already adapted our various schemes to incorporate specific elements of disciplinary and cultural dialogues (Hennessy, Bouton, and Asterhan Citationin press); this is a particular area for further development.
A key next step is to integrate the analytic scheme into our existing set of flexible, multilingual resources for practitioner inquiry that have been developed and used by over 360 practitioners (from pre-school to higher education) in 15 countries: T-SEDA (T-SEDA Collective Citation2023). The T-SEDA systematic observation tools make classroom dialogue more visible, developing critical awareness that supports pedagogical change and enhances student participation (Calcagni et al. Citation2023). Recent practitioner inquiries into technology-mediated dialogic practices, already feeding into populating Tech-SEDA, indicate that a Tech-T-SEDA version could be similarly supportive here. For example, teachers might want to explore which configurations of face-to-face synchronous and online asynchronous dialogues support subject learning in their contexts, or whether/how normally reticent students’ participation can be increased through technology mediation.Footnote41 The T-SEDA materials illustrate adaptation of coding scheme descriptors for young children’s dialogue – perhaps a further area for Tech-SEDA development.
The field is nascent and there is considerable scope to explore how new forms of multimodal, dialogic interaction might be supported and analysed across different technology, cultural and learner contexts. While the methods outlined collectively offer pointers for integrating the outcomes to offer insights that go beyond their parts, more systematic empirical research is needed on interactions where bodily actions and talk outside the technology are entangled with those in other (verbal or nonverbal) modes via the technology environment, including detecting emotional states. How to capture what teachers/educators are doing to orchestrate multimodal interaction requires more attention too; in particular, whether and how tech affordances are being differentially exploited in (or constraining) the multiple interaction modes. Methodological developments allow us to consider future substantive research questions such as whether and how technology use can support the specific dialogue forms and supportive culture shown to be associated with attainment gains through in-person learning (e.g. Alexander Citation2018; Howe et al. Citation2019). Finally, we are currently programming a generative AI system to code using Tech-SEDA, raising new issues again concerning multimodal dialogue analysis.
Supplemental Material
Download Zip (19.7 MB)Acknowledgments
Tech-SEDA was originally conceived during a UK-Mexico collaboration (‘A Tool for Analysing Dialogic Interactions in Classrooms’: http://tinyurl.com/BAdialogue) funded through the British Academy International Partnership and Mobility Scheme in 2013–2015. The work continued via a UK-Hong Kong collaboration funded via a British Academy Visiting Fellowship project (‘Dialogic teaching and knowledge building supported by technology’) co-led by Carol Chan in 2018. A large number of people have contributed to this work over the years. The conceptual and practical contributions of Omar Torreblanca in the initial stage of the work were especially valuable. I am also exceedingly grateful for the significant inputs of Qian Liu, Yuyao Tong, Carol Chan, Louis Major and Aitor Kelvin Gomez. Further contributors included Mariana Alarcón, María José Barrera, Annabel Amodia-Bidakowska, Gary Beauchamp, Patricia Brooks, Meaghan Brugha, Lydia Cao, Vincent English, Lindsay Hetherington, Andrew Joyce-Gibbons, Nermin Karademir, Ruth Kershner, Michelle Kroll, Manoli Pifarré, Ingvill Rasmussen, Shengpeng Shi, Toshi Tsuruhara, Alison Twiner, Paul Warwick, Rupert Wegerif, and several others who participated in workshops, contributed examples from their research to the scheme, or provided feedback on an earlier draft of the article. I also appreciated the anonymous peer reviewer’s constructive comments. Finally, where not explicitly mentioned, images taken from dissertations and conference papers have all been reproduced with the authors’ kind permission.
Disclosure statement
No potential conflict of interest was reported by the author(s).
Supplemental data
Supplemental data for this article can be accessed online at https://doi.org/10.1080/19463014.2024.2339346.
Data available statement
The following supporting files are available at https://osf.io/4gthf/:
Appendix A: Tech-SEDA with worked examples (two per code plus multiple-code examples), including link to Glossary of technologies.
Appendix B: Full Tech-SEDA scheme (with short examples)
Appendix C: Tech-SEDA coding rules
Correction Statement
This article has been corrected with minor changes. These changes do not impact the academic content of the article.
Additional information
Funding
Notes
1. For example, learners can critique and improve others’ texts using online reciprocal peer review systems (Cho and Schunn Citation2007) or Google Classroom (Igglesden Citation2021).
2. Unlike other forms of school writing, there is hence a lower threshold for contributions.
3. Digital knowledge artefacts are construed by Hennessy (Citation2011) as secondary artefacts rather than mental entities. They are actual physical or perceptual embodiments of forms of action – symbolic externalisations or ‘reflections’ of modes of action (Wartofsky Citation1979, 201–202).
4. Visual representations of developing knowledge have long been known to support collaborative learning; in one study, knowledge maps of a problem were provided either as a supplement or replacement for threaded discussion so that the discourse could be focused on the knowledge being constructed; students using the map alone were more likely to converge on the same conclusion and also scored higher on the post-test (Suthers et al. Citation2008).
5. More details of this example in Tech-SEDA, Multiple codes tab, example M3.
6. The need to externalise ideas for the community may prompt students to offer deeper explanations in collaborative inquiry (Hakkarainen Citation2004; Lipponen Citation2002) and thereby extend individual knowledge (Cress and Kimmerle Citation2008); equally it may highlight incongruities between an individual’s and others’ ideas that stimulate an attempt at resolution.
7. Bodily-material resources may be used alongside technology resources and can act as epistemic tools with their own added cognitive value as a tool for a group’s collaborative thinking. In Bernhard et al. (Citation2020) study of engineering students in collaborative design projects, the use of bodily resources (e.g. gestures, utterances, bodily orientations), concrete materials (e.g. 3D-foam models, paper models), ‘low-tech’ inscriptions (e.g. sketches, drawings on paper, sticky notes) and ‘high-tech’ inscription devices (e.g. computer-assisted drawings) were heavily interwoven with rapid, seamless shifts between them.
8. This is not accidental but systematically achieved through close attention to the emerging structure of the activity in progress, as participants continually process and assess others’ contributions. When they overlap, this may indicate congruent understanding or be querying, perpetuating an extended overlap (Goodwin Citation2018).
9. Jamboard is an open source virtual board allowing sticky ‘post-it’ notes to be written and (re)positioned by users.
10. Such multimodal interactions and other examples in this article that construct meanings spanning across the spatial locations outlined in also blur the boundary between material and immaterial dimensions of educational space (Burnett Citation2011; Wohlwend et al. Citation2017).
11. These are not deterministic, however; conceptions of a technology develop during both the evolution of its design and its appropriation as a functional tool, to become aligned with user concerns (Ruthven, Hennessy, and Deaney Citation2008).
12. Hennessy (Citation2020) elaborates and illustrates some of these methods. See further illustrations in this video.
13. Some of these methods are common in computer-mediated discourse analysis and other work on computer-supported collaborative learning (CSCL). Detailed atomic events captured in a log stream (as with TalkWall) can be hard to interpret though. Moreover, ethical issues arise when researchers have access to the learners’ complete record of technology usage and advance consent will be needed.
14. This may be particularly helpful for interpreting contributions of young children, whose language may be less developed.
15. Agreement and Other invitations were dropped as they are not intrinsically dialogic and did not prove productive for learning in the study by Howe et al. (Citation2019).
16. See examples 17 and 18 in Tech-SEDA. In turn, the collaboration led to an explicit focus on dialogue and adoption of Tech-SEDA codes in some KF discourse analysis, with a few additions, including Idea development and Invite idea development (Tong and Chan Citation2020).
17. More details of this example in Tech-SEDA, Multiple codes tab, example M2.
18. More details of this example in Tech-SEDA, Multiple codes tab, example M4.
19. Templates are included in the Toolkit for Systematic Educational Dialogue Analysis (T-SEDA Collective Citation2023).
20. See the range in Appendices A and B.
21. For example: ‘Precisely! I have been wondering about the same thing. Since if the definition of intelligence changes by culture, situation, and the individual who is doing the measuring, … , then how on earth is it possible to create something reliable and temporally relevant?!’ (Muukkonen, Lakkala, and Hakkarainen Citation2005, 552)
22. More details of this example in Tech-SEDA, Multiple codes tab, example M1.
24. Indeed, Muukkonen et al. (Citation2005) found that posts of technology groups were shorter and less precise than written learning logs technology, although technology mediation supported significantly more collaborative development of ideas through dialogue than students without access to technology.
25. Video clip available at https://sms.cam.ac.uk/media/1388048.
26. In the FLE (Future Learning Environment) used by Muukkonen et al. (Citation2005), metacognitive reflection on learning or dialogue processes, including explaining changes in one’s thinking to others, is likewise built in.
27. A detailed example (no. 16) appears in Tech-SEDA. Tong and Chan (Citation2023) argue that such meta-discourse helps students understand the epistemic and dialogic nature of discourse as well as enriching the progressive inquiry.
28. Social dimensions of dialogue, including interpersonal relationships and the degree of power sharing (Lefstein and Barak Citation2020), are influential but often glossed over by researchers. ‘Communicative anxiety’ can arise in an online medium where the acknowledgements, body language cues and relationships that underpin face-to-face interactions are less evident and trust may need to be more consciously developed (Wegerif Citation2007). Using symbolic representations like the challenge emojis in Hay’s study and other similar devices may play an important role. We need to recognise that emoji literacy and cultural meaning may not be universal though.
29. This idea is adapted from Hay’s (Citation2022) study where this design was used in Padlet to get learners to show whether they were in support of a hypothesis, arguing against it or neutral/undecided: see .
30. Davidsen (Citation2019) offers a simple example, describing how two children at a touch screen computer learning about scale position a vertical line and make a gesture in the air (away from the screen) to indicate how big a metre is.
31. The extremely helpful Timemachine tool in KF, for instance, replays all such actions.
32. Note that nonverbal interaction with/within tech can even be used to allocate or signify the taking of turns, that is, it is pertinent to managing the group communication dynamic rather than to the learning activity per se. In one study, an electronic roulette application was used on an IWB to randomly assign turns and phrases to students using a story machine (Fernández-Cárdenas and Silveyra-De La Garza Citation2010). The researchers considered clicking the roulette icon, and pulling the lever to generate a new prompt, as turns in the dialogue.
33. These units can be tightly linked through devices such as (1) tight spatiotemporal co-occurrence in place and time (both produced by the same source), (2) use of explicit deictic expressions (e.g. ‘like this)’; (3) use of eye gaze for directing attention towards a developing artefact.
34. Simpson (Citation2022) points out that Barad’s (Citation2007) theory does not highlight the importance of the timing or sequencing of events and activity – the chain of actions and reactions – within a specific entanglement, and nor does it highlight the nature of the dependencies of an entanglement. While a series of actions by one participant may count as one turn (like a string of sentences), are overlapping nonverbal and/or verbal interactions integrated parts of a multimodal turn or separate turns (with varied lengths) in different modes?
35. Researchers usually identify emotional connection manually, analysing both verbal and non-verbal interaction (e.g. surprise, admiration, happiness: Pifarré Citation2019), but the whole area is under-researched in the dialogue field. In Ilic’s study, analysis of e-journals concerning collaborative mobile learning, students expressed positive sentiment consisting of anticipation, trust, joy, and surprise, plus fear.
36. Specialised tools are available to anonymise videos and photographs and blur faces, e.g. YouTube Studio, Gom Mix or OpenCV and Python.
37. Various softwares are available that facilitate coding and annotation of video and multimodal transcription, including the open source Elan used by our team and Visual Studio Code used by Falloon (Citation2013) and Wohlwend (Citation2017).
38. ENA can be applied to referential gaze coordination during collaborative task sequences (Andrist et al. Citation2015) or emotional engagement (joy, anxiety, etc.) and metacognition in tech-mediated inquiry (Yang et al. Citation2022), offering many exciting possibilities for multimodal dialogue analysis. ENA has also shown how higher performing Japanese students use systematic, regulatory epistemic actions upon their conceptual to improve ideas, generate explanations and create new knowledge during collaborative science learning (Oshima, Oshima, and Saruwatari Citation2020).
39. More details of this example in Tech-SEDA, example 8.
40. Other prevalent nonverbal codes included: rotates object, adjusts dimensions, hand gestures to express idea.
41. Ongoing graduate student work in our group focuses (post-pandemic) on online teaching, exploring affordances for dialogue and the impact on participation through synchronous teaching using video conferencing (audio-visual interactions) and polling/response systems, and chatbot facilitation of online discussion.
42. There is also often dialogic interaction and co-construction of artefacts away from the technology that is related to the tech-mediated activity, acting as a ‘priming’ mechanism to allow students to rehearse ideas before sharing them (Ravenscroft Citation2007), or constituting an interim period of exploration, or following up the tech-mediated activity; researchers may want to capture these episodes and artefacts too (Hennessy Citation2020).
43. More details about this example from the author’s observation of a secondary school English lesson are available in this video resource.
References
- Alexander, R. 2020. A Dialogic Teaching Companion. London: Routledge.
- Alexander, R. J. 2018. “Developing Dialogic Teaching: Genesis, Process, Trial.” Research Papers in Education 33 (5): 561–598. https://doi.org/10.1080/02671522.2018.1481140.
- Amodia-Bidakowska, A. 2020. Disciplinary Dialogues: Examining the Influence of Subject on Classroom Dialogue, Activity Type and Learning Outcomes in English Primary Schools. PhD, University of Cambridge. https://doi.org/10.17863/CAM.69281.
- Andrist, S., W. Collier, M. Gleicher, B. Mutlu, and D. Shaffer. 2015. “Look Together: Analyzing Gaze Coordination with Epistemic Network Analysis.” Frontiers in Psychology 6. https://doi.org/10.3389/fpsyg.2015.01016.
- Bakhtin, M. M. 1981. The Dialogic Imagination. Austin, TX: University of Texas Press.
- Barad, K. 2007. Meeting the Universe Halfway: Quantum Physics and the Entanglement of Matter and Meaning. Durham, NC: Duke university press.
- Beers, P. J., H. P. A. Boshuizen, P. A. Kirschner, and W. H. Gijselaers. 2005. “Computer Support for Knowledge Construction in Collaborative Learning Environments.” Computers in Human Behavior 21 (4): 623–643. https://doi.org/10.1016/j.chb.2004.10.036.
- Bereiter, C. 2002. Education and Mind in the Knowledge Age. Mahwah, NJ: Lawrence Erlbaum.
- Bernhard, J., J. Davidsen, and T. Ryberg. 2020. “By Hand and by Computer: –A Video-Ethnographic Study of Engineering Students’ Representational Practices in a Design Project.” Educate for the Future: PBL, Sustainability and Digitalisation: 561–570.
- Blake, C., E. Scanlon, A. Twiner, T. Collins, A. Jones, and L. Kerawalla. 2013. “Analysis of learners’ Fieldtrip Talk during a Collaborative Inquiry Task.” Scaling up Learning for Sustained Impact. Paper presented at the Proceedings of the 8th European Conference on Technology Enhanced Learning, Paphos, Cyprus, September (32-42). edited by D. Hernández-Leo, T. Ley, R. Klamma, and A. Harrer. Heidelberg: Springer.
- Boyd, M., and D. Rubin. 2006. “How Contingent Questioning Promotes Extended Student Talk: A Function of Display Questions.” Journal of Literacy Research 38 (2): 141–169. https://doi.org/10.1207/s15548430jlr3802_2.
- Bridges, S. M. 2020. Keynote Address. “Dialogic Intervisualizing: Re-Conceptualising the Role of Digital Texts in Collaborative Multimodal Inquiry.” 9th International Conference on Information Technology in Education (CITE 2020), Guilin, China.
- Bridges, S., L. K. Chan, J. Green, A. Saleh, and C. Hmelo-Silver. 2019. “Dialogic Intervisualizing: Rethinking Text-Discourse-Learning Relations in Multimodal Problem-Based Learning.” A Wide Lens: Combining Embodied, Enactive, Extended, and Embedded Learning in Collaborative Settings, 13th International Conference on Computer Supported Collaborative Learning (CSCL) 2019, edited by K. Lund, G. P. Niccolai, E. Lavoué, C. Hmelo-Silver, G. Gweon, and M. Baker, 915–916, Volume 2). Lyon, France. International Society of the Learning Sciences. https://repository.isls.org//handle/1/1728.
- Bridges, S. M., C. E. Hmelo-Silver, L. K. Chan, J. L. Green, and A. Saleh. 2020. “Dialogic Intervisualizing in Multimodal Inquiry.” International Journal of Computer-Supported Collaborative Learning 15 (3): 283–318. https://doi.org/10.1007/s11412-020-09328-0.
- Burnett, C. 2011. “The (Im)materiality of Educational Space: Interactions Between Material, Connected and Textual Dimensions of Networked Technology Use in Schools.” E-Learning & Digital Media 8 (3): 214–227. https://doi.org/10.2304/elea.2011.8.3.214.
- Calcagni, E., F. Ahmed, A. L. Trigo Clapés, R. Kershner, and S. Hennessy. 2023. “Developing Dialogic Classroom Practices through Supporting Professional Agency: Teachers’ Experiences of Using the T-SEDA Practitioner-Led Inquiry Approach.” Teaching and Teacher Education. https://www.sciencedirect.com/science/article/pii/S0742051X23000550.
- Cao, Y. 2022. Developing Teachers’ Contingent Responsiveness in Dialogic Science Teaching via Mixed-Reality Simulations: A Design-Based Study. Doctoral thesis, University of Cambridge. https://doi.org/10.17863/CAM.90530.
- Chan, C., S. Hennessy, Y. Tong, J. van Aalst, and R. Wegerif. 2019. “Temporal Analysis of Interaction Between Spoken and Digitally Mediated Dialogue in Knowledge Building.” Annual Conference of the American Educational Research Association, Toronto, April.
- Chan, C. K. K., Y. Y. Tong, Y. Zhang, A. K. L. Cheng, X. Hu, and X. Feng. 2023. “Developing Collaborative Dialogic Inquiry and Theory-Practice Integration Using Online Discussion for Preservice Teachers.” Paper to be presented at the Annual Meeting of the American Educational Research Association, Chicago, April.
- Cho, K., and C. D. Schunn. 2007. “Scaffolded Writing and Rewriting in the Discipline: A Web-Based Reciprocal Peer Review System.” Computers & Education 48 (3): 409–426. https://doi.org/10.1016/j.compedu.2005.02.004.
- Cook, V., P. Warwick, M. Vrikki, L. Major, and R. Wegerif. 2019. “Developing Material-Dialogic Space in Geography Learning and Teaching: Combining a Dialogic Pedagogy with the Use of a Microblogging Tool.” Thinking Skills and Creativity 31:217–231. https://doi.org/10.1016/j.tsc.2018.12.005.
- Cowan, K. 2014. “Multimodal Transcription of Video: Examining Interaction in Early Years Classrooms.” Classroom Discourse 5 (1): 6–21. https://doi.org/10.1080/19463014.2013.859846.
- Cress, U., and J. Kimmerle. 2008. “A Systemic and Cognitive View on Collaborative Knowledge Building with Wikis.” International Journal of Computer-Supported Collaborative Learning 3 (2). https://doi.org/10.1007/s11412-007-9035-z.
- Cukurova, M., M. Giannakos, and R. Martinez‐Maldonado. 2020. “The Promise and Challenges of Multimodal Learning Analytics.” British Journal of Educational Technology 51 (5): 1441–1449. https://doi.org/10.1111/bjet.13015.
- Davidsen, J. 2019. Bodily Material Resources in Collaborative Learning. Guest lecture, University of Oslo. https://www.uv.uio.no/english/research/groups/lida/open-events/guest-lecture-with-jacob-davidsen.html.
- Du Bois, J. W. 2014. “Towards a Dialogic Syntax.” Cognitive Linguistics 25 (3): 359–410. https://doi.org/10.1515/cog-2014-0024.
- Emerson, A., E. B. Cloude, R. Azevedo, and J. Lester. 2020. “Multimodal Learning Analytics for Game‐Based Learning.” British Journal of Educational Technology 51 (5): 1505–1526. https://doi.org/10.1111/bjet.12992.
- Enfield, N. J. 2009. The Anatomy of Meaning: Speech, Gesture and Composite Utterances. Cambridge: Cambridge University Press.
- Falloon, G. 2013. “Young Students Using iPads: App Design and Content Influences on their Learning Pathways.” Computers & Education 68:505–521. https://doi.org/10.1016/j.compedu.2013.06.006.
- Fawns, T. 2022. “An Entangled Pedagogy: Looking Beyond the Pedagogy—Technology Dichotomy.” Postdigital Science & Education 4 (3): 711–728. https://doi.org/10.1007/s42438-022-00302-7.
- Fernández-Cárdenas, J. M., and M. L. Silveyra-De La Garza. 2010. “Disciplinary Knowledge and Gesturing in Communicative Events: A Comparative Study between Lessons Using Interactive Whiteboards and Traditional Whiteboards in Mexican Schools.” Technology, Pedagogy & Education 19 (2): 173–193. https://doi.org/10.1080/1475939X.2010.491219.
- Floriani, A. 1993. “Negotiating What Counts: Roles and Relationships, Texts and Contexts, Content and Meaning.” Linguistics & Education 5 (3/4): 241–274. https://doi.org/10.1016/0898-5898(93)90002-R.
- Frøytlog, J. I. J., and I. Rasmussen. 2020. “The Distribution and Productivity of Whole-Class Dialogues: Exploring the Potential of Microblogging.” International Journal of Educational Research 99:101501. https://doi.org/10.1016/j.ijer.2019.101501.
- Gibson, J. J. 1979. The Ecological Approach to Visual Perception. Boston, MA: Houghton Mifflin.
- Goldin‐Meadow, S. 2009. “How Gesture Promotes Learning throughout Childhood.” Child Development Perspectives 3 (2): 106–111. https://doi.org/10.1111/j.1750-8606.2009.00088.x.
- Gomez, A. 2021. The Design, Development and Evaluation of an Augmented Reality Application to Support Collaborative Creative Thinking at Lower Secondary School. PhD, University of Cambridge. https://doi.org/10.17863/CAM.81976.
- Goodwin, C. 2003. “Pointing as Situated Practice.” In Pointing: Where Language, Culture, and Cognition Meet, edited by S. Kita, 217–241. Mahwah, New Jersey: Lawrence Erlbaum.
- Goodwin, C. 2018. Co-Operative Action. Cambridge: Cambridge University Press.
- Green, J. L., and S. M. Bridges. 2018. “Interactional Ethnography.” In International Handbook of the Learning Sciences, edited by F. Fischer, C. E. Hmelo-Silver, S. R. Goldman, and P. Reimann, 475–488. NY: Routledge.
- Hakkarainen, K. 2004. “Pursuit of Explanation within a Computer-Supported Classroom.” International Journal of Science Education 26 (8): 979–996. https://doi.org/10.1080/1468181032000354.
- Hay, L. 2022. Enacting Challenge in Online Dialogue. Practitioner Professional Development ‘Educational Dialogue’ Module Inquiry Report. University of Cambridge.
- Hennessy, S. 2011. “The Role of Digital Artefacts on the Interactive Whiteboard in Mediating Dialogic Teaching and Learning.” Journal of Computer Assisted Learning 27 (6): 463–586. https://doi.org/10.1111/j.1365-2729.2011.00416.x.
- Hennessy, S. 2020. “Methods for Researching Technology-Mediated Dialogue.” In Research Methods for Educational Dialogue, edited by R. Kershner, S. Hennessy, R. Wegerif, and A. Ahmed, 135–172. London: Bloomsbury Academic.
- Hennessy, S., E. Bouton, and C. Asterhan. in press. “Mixing Methods for Analysing Classroom Dialogue: Coding and Beyond.” In Research Handbook on Classroom Observation, edited by S. Kelly. NY: Routledge.
- Hennessy, S., C. Howe, N. Mercer, and M. Vrikki. 2020. “Coding Classroom Dialogue: Methodological Considerations for Researchers.” Learning, Culture & Social Interaction 25. https://doi.org/10.1016/j.lcsi.2020.100404.
- Hennessy, S., S. Rojas-Drummond, R. Higham, A. M. Marquez, F. Maine, R. M. Rios, R. García-Carrión, O. Torreblanca, and M. J. Barrera. 2016. “Developing a Coding Scheme for Analysing Classroom Dialogue Across Educational Contexts.” Learning, Culture & Social Interaction 9:16–44. https://doi.org/10.1016/j.lcsi.2015.12.001.
- Hetherington, L., and R. Wegerif. 2018. “(Embargo). Developing a Material-Dialogic Approach to Pedagogy to Guide Science Teacher Education.” Journal of Education for Teaching 44 (1): 27–43. http://hdl.handle.net/10871/30118.
- Hodder, I. 2012. Entangled: An Archaeology of the Relationships between Humans and Things. Chichester, West Sussex, UK: John Wiley & Sons.
- Hofmann, R., and K. Ruthven. 2018. “Operational, Interpersonal, Discussional and Ideational Dimensions of Classroom Norms for Dialogic Practice in School Mathematics.” British Educational Research Journal 44 (3): 496–514. https://doi.org/10.1002/berj.3444.
- Howe, C., S. Hennessy, N. Mercer, M. Vrikki, and L. Wheatley. 2019. “Teacher-Student Dialogue during Classroom Teaching: Does it Really Impact Upon Student Outcomes?” Journal for the Learning Sciences 28 (4–5): 462–512. https://doi.org/10.1080/10508406.2019.1573730.
- Hymes, D. 1972. “Models of Interaction in Language and Social Life.” In Directions in Sociolinguistics: The Ethnography of Communication, edited by J. J. Gumperz and D. Hymes, 35–71. London: Basil Blackwell.
- Igglesden, T. 2021. Designs and Affordances for Dialogue in Google Classrooms: A Design-Based Research Study. Doctoral Dissertation, University of Cambridge. https://www.repository.cam.ac.uk/handle/1810/334854?show=full.
- Ilic, P. 2020. “Sentiment Analysis of students’ Attitudes Toward Mobile Learning Activities.” In CALL for Widening Participation: Short Papers from EUROCALL 2020, edited by K.-M. Frederiksen, S. Larsen, L. Bradley, and S. Thouësny, 114–119. Research-publishing.net. https://doi.org/10.14705/rpnet.2020.48.1174.
- Janin, L. 2023. “A Multimodal Perspective on Adult learners’ Vocabulary Explanations in the Beginner-Level L2 Classroom.” Classroom Discourse 15 (1): 94–115. https://doi.org/10.1080/19463014.2023.2222857.
- Jeong, H., and C. E. Hmelo-Silver. 2016. “Seven Affordances of Computer-Supported Collaborative Learning: How to Support Collaborative Learning? How Can Technologies Help?” Educational Psychologist 51 (2): 247–265. https://doi.org/10.1080/00461520.2016.1158654.
- Jordan, B., and A. Henderson. 1995. “Interaction Analysis: Foundations and Practice.” Journal of the Learning Sciences 4 (1): 39–103. https://doi.org/10.1207/s15327809jls0401_2.
- Kershner, R. 2020. “Dialogue, Participation and Social Relationships.” In Research Methods for Educational Dialogue, edited by S. H. R. Kershner, R. Wegerif, and A. Ahmed, 197–222. London: Bloomsbury Academic.
- Kim, M.-Y., and I. A. G. Wilkinson. 2019. “What is Dialogic Teaching? Constructing, Deconstructing, and Reconstructing a Pedagogy of Classroom Talk.” Learning, Culture & Social Interaction 21:70–86. https://doi.org/10.1016/j.lcsi.2019.02.003.
- Kirschner, P. A., P. J. Beers, H. P. A. Boshuizen, and W. H. Gijselaers. 2008. “Coercing Shared Knowledge in Collaborative Learning Environments.” Computers in Human Behavior 24 (2): 403–420. https://doi.org/10.1016/j.chb.2007.01.028.
- Kress, G. R. 2010. Multimodality: A social semiotic approach to contemporary communication. London: Routledge.
- Kumpulainen, K., and L. Lipponen. 2010. “Productive Interaction as Agentic Participation in Dialogic Enquiry. Chapter 3.” In Educational Dialogues: Understanding and Promoting Productive Interaction, edited by K. Littleton and C. Howe, 48–63. Abingdon, Oxon: Routledge.
- Kumpulainen, K., and A. Rajala. 2017. “Dialogic Teaching and students’ Discursive Identity Negotiation in the Learning of Science.” Learning & Instruction 48:23–31. https://doi.org/10.1016/j.learninstruc.2016.05.002.
- Lee, E. Y. C., C. K. K. Chan, and J. van Aalst. 2006. “Students Assessing their Own Collaborative Knowledge Building.” International Journal of Computer-Supported Collaborative Learning 1 (1): 57–87. https://doi.org/10.1007/s11412-006-6844-4.
- Lefstein, A., and M. Barak. 2020. “Tradeoffs and Dilemmas in Coding Classroom Dialogue – Commentary.” In Research Methods in Educational Dialogue, edited by R. Kershner, S. Hennessy, R. Wegerif and A. Ahmed, 95–134. London and NY: Bloomsbury.
- Lefstein, A., J. Snell, and M. Israeli. 2015. “From Moves to Sequences: Expanding the Unit of Analysis in the Study of Classroom Discourse.” British Educational Research Journal 41 (5): 866–885. https://doi.org/10.1002/berj.3164.
- Lemke, J. 1999. “Typological and Topological Meaning in Diagnostic Discourse.” Discourse processes 27 (2): 173–185. https://doi.org/10.1080/01638539909545057.
- Ley, T. 2019. “Knowledge Structures for Integrating Working and Learning: A Reflection on a Decade of Learning Technology Research for Workplace Learning.” British Journal of Educational Technology 51 (2): 331–346. https://doi.org/10.1111/bjet.12835.
- Lipponen, L. 2002. “Exploring Foundations for Computer-Supported Collaborative Learning.” Proceedings of CSCL 2002 edited by G. Stahl, 72–81. Boulder. ISLS.
- Liu, Q. 2023. Developing Dialogic Mathematics Teaching with Tablet Technology in Chinese Primary School Classrooms: A Design-Based Approach to Teacher Professional Development. PhD Thesis, University of Cambridge. https://doi.org/10.17863/CAM.92982.
- Major, L., P. Warwick, I. Rasmussen, S. Ludvigsen, and V. Cook. 2018. “Classroom Dialogue and Digital Technologies: A Scoping Review.” Education and Information Technologies: 1–34. https://doi.org/10.1007/s10639-018-9701-y.
- McGann, M., H. De Jaegher, and E. Di Paolo. 2013. “Enaction and Psychology.” Review of General Psychology 17 (2): 203–209. https://doi.org/10.1037/a0032935.
- Mercer, N., and K. Littleton. 2007. Dialogue and the Development of Children’s Thinking. London: Routledge.
- Michaels, S., C. O’Connor, and L. B. Resnick. 2008. “Deliberative Discourse Idealized and Realized: Accountable Talk in the Classroom and in Civic Life.” Studies in Philosophy and Education 27 (4): 283–297. https://doi.org/10.1007/s11217-007-9071-1.
- Mochizuki, T., H. Sasaki, T. Wakimoto, Y. Kubota, B. Eagan, R. Hirayama, and H. Kato, N. Yuki, H. Funaoi, H. Suzuki, H. Kato. 2022. “Mediating and Perspective-Taking Manipulatives: Fostering Dynamic Perspective-Taking by Mediating Dialogic Thinking and Bolstering Empathy in Role-Play and Reflection for Microteaching.” International Journal of Computer-Supported Collaborative Learning 17 (4): 1–30. https://doi.org/10.1007/s11412-022-09382-w.
- Muhonen, H., E. Pakarinen, H. Rasku-Puttonen, and M. K. Lerkkanen. 2020. “Dialogue through the Eyes: Exploring Teachers’ Focus of Attention during Educational Dialogue.” International Journal of Educational Research 102:101607. https://doi.org/10.1016/j.ijer.2020.101607.
- Muukkonen, H., M. Lakkala, and K. Hakkarainen. 2005. “Technology-Mediation and Tutoring: How do they Shape Progressive Inquiry Discourse?” Journal of the Learning Sciences 14 (4): 527–565. https://doi.org/10.1207/s15327809jls1404_3.
- Norris, S. 2004. Analyzing Multimodal Interaction. A Methodological Framework. New York and London: Routledge.
- Nystrand, M., L. L. Wu, A. Gamoran, S. Zeiser, and D. A. Long. 2003. “Questions in Time: Investigating the Structure and Dynamics of Unfolding Classroom Discourse.” Discourse Processes 35 (2): 135–198. https://doi.org/10.1207/S15326950DP3502_3.
- Olsen, J. K., K. Sharma, N. Rummel, and V. Aleven. 2020. “Temporal Analysis of Multimodal Data to Predict Collaborative Learning Outcomes.” British Journal of Educational Technology 51 (2): 1527–1547. https://doi.org/10.1111/bjet.12982.
- Omland, M., and K. A. Rødnes. 2020. “Building Agency through Technology-Aided Dialogic Teaching.” Learning, Culture & Social Interaction 26:1e14. https://doi.org/10.1016/j.lcsi.2020.100406.
- Oshima, J., R. Oshima, and S. Saruwatari. 2020. “Analysis of Students’ Ideas and Conceptual Artifacts in Knowledge-Building Discourse.” British Journal of Educational Technology 51 (4): 1308–1321. https://doi.org/10.1111/bjet.12961.
- Paavola, S., and K. Hakkarainen. 2014. “Trialogical Approach for Knowledge Creation.” In Knowledge Creation in Education, 53–73. Singapore: Springer.
- Paulus, T. M. 2005. “Collaboration or Cooperation? Analyzing Small Group Interactions in Educational Environments.” In Computer-Supported Collaborative Learning in Higher Education, edited by T. S. Roberts, 100–125. Hershey PA: Idea Group Inc.
- Perrone, C. 2016. Analysing the Quality of Peer-Peer Talk in a Secondary School Mathematics Class. Cambridge: Master of Education, University of Cambridge.
- Pifarré, M. 2019. “Using Interactive Technologies to Promote a Dialogic Space for Creating Collaboratively: A Study in Secondary Education.” Thinking Skills and Creativity 32:1–16. https://doi.org/10.1016/j.tsc.2019.01.004.
- Pifarré, M., and L. Li. 2018. “Characterizing and Unpacking Learning to Learn Together Skills in a Wiki Project in Primary Education.” Thinking Skills and Creativity 29:45–58. https://doi.org/10.1016/j.tsc.2018.06.004.
- Ravenscroft, A. 2007. “Promoting Thinking and Conceptual Change with Digital Dialogue Games: Thinking and Dialogue Games.” Journal of Computer Assisted Learning 23 (6): 453–465. https://doi.org/10.1111/j.1365-2729.2007.00232.x.
- Rødnes, K. A., I. Rasmussen, M. Omland, and V. Cook. 2021. “Who has Power? An Investigation of How One Teacher Led her Class Towards Understanding an Academic Concept through Talking and Microblogging.” Teaching & Teacher Education 98:103229. https://doi.org/10.1016/j.tate.2020.103229.
- Rovai, A. P. 2007. “Facilitating Online Discussions Effectively.” The Internet and Higher Education 10 (1): 77–88. https://doi.org/10.1016/j.iheduc.2006.10.001.
- Ruthven, K., S. Hennessy, and R. Deaney. 2008. “Constructions of Dynamic Geometry: A Study of the Interpretative Flexibility of Educational Software in Classroom Practice.” Computers & Education 51 (1): 297–317. https://doi.org/10.1016/j.compedu.2007.05.013.
- Säljö, R. 1995. “Mental and Physical Artefacts in Cognitive Practices.” In Learning in Humans and Machines: Towards an Interdisciplinary Learning Science, edited by P. Reimann and H. Spada, 83–96. Oxford: Pergamon.
- Samuelsson, R., S. Price, and C. Jewitt. 2022. “How Pedagogical Relations in Early Years Settings are Reconfigured by Interactive Touchscreens.” British Journal of Educational Technology 53 (1): 58–76. https://doi.org/10.1111/bjet.13152.
- Saville-Troike, M. 2003. The Ethnography of Communication: An Introduction. Third ed. Oxford: Blackwell.
- Scardamalia, M., and C. Bereiter. 2014. “Knowledge Building and Knowledge Creation: Theory, Pedagogy, and Technology.” In The Cambridge Handbook of the Learning Sciences, edited by R. K. Sawyer, 397–417. 2nd ed. New York, NY: Cambridge University Press.
- Schindler, A. K., T. Seidel, R. Böheim, M. Knogler, M. Weil, M. Alles, and A. Gröschner. 2021. “Acknowledging Teachers’ Individual Starting Conditions and Zones of Development in the Course of Professional Development.” Teaching & Teacher Education 100:103281. https://doi.org/10.1016/j.tate.2021.103281.
- Sedlacek, M., and K. Sedova. 2017. “How many are Talking? The Role of Collectivity in Dialogic Teaching.” International Journal of Educational Research 85:99–108. https://doi.org/10.1016/j.ijer.2017.07.001.
- Sedova, K., M. Sedlacek, and R. Svaricek. 2016. “Teacher Professional Development as a Means of Transforming Student Classroom Talk.” Teaching & Teacher Education 57:14–25. https://doi.org/10.1016/j.tate.2016.03.005.
- Shaffer, D. W. 2017. Quantitative Ethnography. Madison, WI: Cathcart.
- Simpson, A. M. 2022. Dialogic Activity: A Study of Learning Dialogues and Entanglements in a Vocational Tertiary Setting. PhD thesis, New Zealand: Massey University.
- Soter, A. O., I. A. Wilkinson, K. P. Murphy, L. Rudge, K. Reninger, and M. Edwards. 2008. “What the Discourse Tells Us: Talk and Indicators of High-Level Comprehension.” International Journal of Educational Research 47 (6): 372–391. https://doi.org/10.1016/j.ijer.2009.01.001.
- Stahl, G. 2002. “Contributions to a Theoretical Framework for CSCL.” Proceedings of CSCL 2002 edited by G. Stahl, 62–71. Boulder. USA.
- Stahl, G. 2005. “Group Cognition in Computer‐Assisted Collaborative Learning.” Journal of Computer Assisted Learning 21 (2): 79–90. https://doi.org/10.1111/j.1365-2729.2005.00115.x.
- Stahl, G., T. Koschmann, and D. D. Suthers. 2014. “Computer-Supported Collaborative Learning.” In Cambridge Handbook of the Learning Sciences, edited by R. K. Sawyer, 479–500. New York, NY: Cambridge University Press.
- Suthers, D. D., R. Vatrapu, R. Medina, S. Joseph, and N. Dwyer. 2008. “Beyond Threaded Discussion: Representational Guidance in Asynchronous Collaborative Learning Environments.” Computers & Education 50 (4): 1103–1127. https://doi.org/10.1016/j.compedu.2006.10.007.
- Thorne, S. L., and J. Hellermann. 2022. “Coda: The Interactional Affordances and Constraints of Technology-Rich Teaching and Learning Environments.” Classroom Discourse 13 (2): 231–239. https://doi.org/10.1080/19463014.2022.2071959.
- Tomasello, M. 2003. Constructing a Language: A Usage-Based Theory of Language Acquisition. Cambridge, Mass: Harvard Univ. Press.
- Tong, Y., and C. K. K. Chan. 2020. “Characterizing Metacognitive and Progressive Dialogue in Knowledge-Building Classrooms.” Paper presented at the 14th International Conference of the Learning Sciences (ICLS), Nashville, Tennessee. https://doi.org/10.2231/8icls2020.1453.
- Tong, Y., and C. K. K. Chan. 2023. “Promoting Knowledge Building through Meta-Talk and Epistemic Discourse Understanding.” International Journal of Computer-Supported Collaborative Learning 18 (3): 353–391.
- T-SEDA Collective. 2023. Toolkit for Systematic Educational Dialogue Analysis (T-SEDA): A Resource for Inquiry into Practice. V.9. University of Cambridge. http://bit.ly/T-SEDA.
- Vygotsky, L. S. 1978. Mind in Society: The Development of Higher Psychological Processes. Cambridge, MA: Harvard University Press.
- Wartofsky, M. 1979. Models: Representation and the Scientific Understanding. Vol. XLVIII. London: D. Reidel.
- Warwick, P., V. Cook, M. Vrikki, L. Major, and I. Rasmussen. 2020. “Realising ‘Dialogic intentions’ When Working with a Microblogging Tool in Secondary School Classrooms.” Learning, Culture & Social Interaction 24, [100376]. https://doi.org/10.1016/j.lcsi.2019.100376.
- Warwick, P., N. Mercer, R. Kershner, and J. Kleine Staarman. 2010. “In the Mind and in the Technology: The Vicarious Presence of the Teacher in pupils’ Learning of Science in Collaborative Group Activity at the Interactive Whiteboard.” Computers & Education 55 (1): 350–362. https://doi.org/10.1016/j.compedu.2010.02.001.
- Wegerif, R. 2007. Dialogic Education and Technology: Expanding the Space of Learning. London: Springer.
- Wells, G. 1999. Dialogic inquiry: Toward a Sociocultural Practice and Theory of Education. Cambridge: Cambridge University Press.
- Wells, G., and R. M. Arauz. 2006. “Dialogue in the Classroom.” Journal of the Learning Sciences 15 (3): 379–428. https://doi.org/10.1207/s15327809jls1503_3.
- Wilmes, S. E. D., and C. Siry. 2021. “Multimodal Interaction Analysis: A Powerful Tool for Examining Plurilingual students’ Engagement in Science Practices.” Research in Science Education 51 (1): 71–91. https://doi.org/10.1007/s11165-020-09977-z.
- Wise, A. F., S. N. Hausknecht, and Y. Zhao. 2014. “Attending to others’ Posts in Asynchronous Discussions: Learners’ Online ‘Listening’ and Its Relationship to Speaking.” International Journal of Computer-Supported Collaborative Learning 9 (2): 185–209. https://doi.org/10.1007/s11412-014-9192-9.
- Wise, A. F., Y. T. Hsiao, F. Marbouti, J. Speer, and N. Perera. In J. van Aalst. 2012. “Initial Validation of ‘Listening’ Behavior Typologies for Online Discussions Using Microanalytic Case Studies.” Proceedings of the 10th International Conference of the Learning Sciences 2012 edited by K. Thompson, M. Jacobson, and P. Reimann, 56–63. Sydney, Australia. ISLS.
- Wise, A. F., Y. Zhao, and S. N. Hausknecht. 2014. “Learning Analytics for Online Discussions: A Pedagogical Model for Intervention with Embedded and Extracted Analytics.” LAK’ 13: Proceedings of the Third International Conference on Learning Analytics and Knowledge, Leuven, 48–56.
- Wohlwend, K. 2017. “Chasing Literacies Across Action Texts and Augmented Realities: E-Books, Animated Apps, and Pokémon Go.” In The Case of the iPad: Mobile Literacies in Education, 49–66. Singapore: Springer.
- Wood, T. 1994. “Patterns of Interaction and the Culture of Mathematical Classrooms.” In Cultural Perspectives on the Mathematics Classroom, edited by S. Lerman, 149–168. Kluwer, Dordrecht, Netherlands: Kluwer Academic Publishers.
- Yang, Y., J. van Aalst, and C. K. K. Chan. 2019. “Dynamics of Reflective Assessment and Knowledge Building for Academically Low-Achieving Students.” American Educational Research Journal 57 (3): 1241–1289. https://doi.org/10.3102/0002831219872444.
- Yang, Y., J. van Aalst, C. K. K. Chan, and W. Tian. 2016. “Reflective Assessment in Knowledge Building by Students with Low Academic Achievement.” International Journal of Computer-Supported Collaborative Learning 11 (3): 281–311. https://doi.org/10.1007/s11412-016-9239-1.
- Yang, Y., K. Yuan, X. Feng, X. Li, and J. van Aalst. 2022. “Fostering Low‐Achieving Students’ Productive Disciplinary Engagement through Knowledge‐Building Inquiry and Reflective Assessment.” British Journal of Educational Technology 53 (6): 1511–1529. https://doi.org/10.1111/bjet.13267.
- Ye, D., and S. Pennisi. 2022. “Analysing Interactions in Online Discussions through Social Network Analysis.” Journal of Computer Assisted Learning 38 (3): 784–796. https://doi.org/10.1111/jcal.12648.