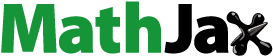
Abstract
The COVID-19 outbreak is impacting clinical trials in many ways, such as patient recruitment, data collection and data analysis. To proceed in this difficult time, the adoption of new technologies and new approaches for conducting clinical trials needs to be accelerated. Simultaneously, regulatory agencies such as the US FDA and EMA have issued guidance to help the pharmaceutical industry conduct clinical trials of medical products during the COVID-19 pandemic. In this article, we will address some statistical issues and operational experiences in the conduction of clinical trials during the COVID-19 pandemic. Specifically, we will share experiences in the applications of remote clinical trials in China. Statistical issues related to protocol modifications caused by COVID-19 will be raised.
1. Introduction
The World Health Organization has issued a declaration of a global public health emergency for the ongoing outbreak of coronavirus disease 2019 (COVID-19) (Zhu et al., 2020; Huang et al., 2020). The pandemic directly or indirectly affects each of us as members of the global community. Many government authorities have issued movement control orders or stay-at-home orders to restrict the movements of residents to suppress the spread of COVID-19. While these orders have successfully mitigated this global health threat, they are extensively creating obstacles to the conduct of ongoing clinical trials. Specifically, such actions restrict visits to clinical sites for trial participants so that patients cannot travel to receive medication or undergo regular monitoring, while site staff and monitoring resources are hindered from demonstrating strict oversight of the studies to ensure proper trial conduct, the safety of study subjects and the accuracy and completeness of the clinical data.
As the pandemic continues to evolve, the curtailed patient visits to clinical sites have also resulted in an interruption or slowing down of recruitment of new trial participants. A study conducted by Medidata (Melhem, 2020) on the repeated analyses of enrollment data from nearly 4,600 current clinical trials and more than 182,000 study sites worldwide has shown significant declines in the number of new patients entering clinical trials for actively recruiting studies. A 65% worldwide average decrease in new patient enrollment year-over-year during March has been reported. Among key world markets, there was a 43% decrease in Japan and an 84% decline in India. Simultaneously, the U.S. was found to have an average of 67% reduction. In China, there was a 68% decrease in new patients entering trials year-over-year in February; however, March saw 240% more patients added than in February. This may indicate that as residents begin to return to normality, a remarkable recovery in the recruitment of new trial participants can be expected. However, care should be taken to see whether the phenomenon is a reflection of COVID-19 recovery or a reflection of Chinese New Year without comparisons with 2019 data.
To navigate during this difficult time, we need to accelerate the adoption of new technologies and new approaches in conducting clinical trials. Most importantly, digital infrastructure can definitely provide great help in proceeding in times like these. To help trial sponsors, the US FDA released guidelines in March 2020 on the conduct of clinical trials of medical products during the COVID-19 pandemic (FDA, 2020). In addition to ensuring the safety of patients and the accuracy and validity of data, the US FDA has also encouraged the clinical research industry to explore more effective alternatives for adjusting and evolving trial operations, such as central, remote processes and procedures including remote consent, remote randomization, and remote data capture trial operations. The US FDA added seven new questions and answers to the guidelines on April 16, 2020.
Simultaneously, the European Medicines Agency (EMA) also issued guidelines on the management of clinical trials during the COVID-19 pandemic to mitigate the disruption of clinical research in Europe and therefore to negative effects of the pandemic without compromising quality and safety (EMA, 2020). EMA later updated their guidelines to provide new recommendations on the distribution of investigational medical products and data verification under social distancing measures and resource limitations. Both guidelines have provided timely recommendations and the technical assistance needed to help the pharmaceutical industry through the COVID-19 pandemic.
In this article, we will introduce some statistical issues and operational experience on the conduction of clinical trials during the COVID-19 pandemic. This article is organized as follows. In section 2, we will share experiences in the applications of remote clinical trials in China. In section 3, statistical issues related to protocol modifications during COVID-19 will be raised. Concluding remarks are given in section 4.
2. Applications of remote clinical trials in China
Since 23 January 2020, Wuhan and other cities in Hubei, China have gone through more than 70 days of lockdown due to the outbreak of COVID-19. During this period, most cities in China have also been impacted by COVID-19. Hospitals and clinics have mainly focused on fighting the disease, and most ongoing clinical trials have been suspended.
According to the survey results conducted by the DIA China Digital Health Community (DIA China, 2020) in February of 2020, among 176 responders, 131 (74.4%) responders stated that trial progress had been suspended, 126 (71.6%) were impacted by subjects failing to return for follow-up visits, suspension of subject recruitment occurred to 118 (67.1%), 88 (50%) were unable to make timely data entries, 70 (40%) were unable to deliver research drugs in a timely fashion. A total of 79 (44.9%) and 78 (44.3%) responders indicated that remote visits and video conferences, respectively, had been adopted as the business continuity plan. Additionally, some responders stated that they had used direct-to-patient (49 (27.8%)), remote/decentralized data monitoring (36 (20.5%)), electronic patient-reported outcomes (32 (18.2%)) and electronic informed consent forms (13 (7.4%)). Here, “direct-to-patient” was applied to deliver study medication directly to patient homes. Approximately 70% of the responders showed interest in learning more about remote clinical trial technology and application tools. Based on the responses and growing demand on remote clinical trials, the DIA China Digital Health Community held a series of online seminars on “Remote Digital Clinical Trials During the COVID-19 Pandemic” on 29 February 2020, 14 March 2020 and 28 March 2020. In these seminars, the veterans shared real cases of remote clinical trials in China and digital clinical trial technology and service and introduced solution plans for remote clinical trials.
Min Jiang, Director of the Beijing Institute for Cancer Research at the Beijing Cancer Hospital, was one of the online seminar speakers, to introduce the remote data monitoring system in the Beijing Cancer Hospital. On 10 February 2020, the Beijing Cancer Hospital launched the Peking University Cancer Hospital Clinical Trial Remote Monitoring System (Nature Research, 2020), which is an artificial intelligence clinical trial platform based on an information system (e.g., informatization of hospital information system in clinical trials and clinical trials management) and data management system (e.g., data process & application platform, digitalization of clinical trial data with data masking). Subject panoramic data, including certified copies of all the data in the electronic health record system and paper records via manual scanning (upcoming) with data masking, can be accessed by the clinical research associate (CRA) in the system. For data safety and security, CRAs from sponsors or contract research organizations need to submit an access application to the institute with a completed application form and a signed network and information security commitment, respectively. Until 27 February, users from 63 sponsors were granted access to the system, and clinical data from a total of 2,885 subjects involved in 154 clinical projects across 16 departments were verified. Issues found in 52 remote monitoring projects included failure of follow-up visit according to the protocol schedule (117 times), failure of drug administration on the protocol schedule (105 times), missing data in the electronic case report form (eCRF) (79 times), failure of drug delivery on the protocol schedule (74 times) and inconsistency between the CRF and source data (69 times). All these pandemic-related events need to be documented. Modification on planned statistical analyses may be needed due to the impact of the pandemic on clinical trials. A general summary of analysis considerations is provided in Meyer at al. (2020). For example, additional analyses for sensitivity to pandemic-related missingness may be needed to properly understand and characterize the treatment effect.
3. Changes in patient population due to protocol modification caused by COVID-19
Challenges in patient recruitment may arise from quarantines, site closures, travel limitations, or other considerations if site personnel or trial subjects become infected with COVID-19. However, the outbreak of COVID-19 did not begin at the same time in all countries and regions. Subsequently, it is likely to shift the sites of recruitment to lower-impacted countries and regions. These situations may result in a similar but slightly different actual patient population among these three periods: “before COVID-19”, “during COVID-19”, and “post COVID-19” (EMA 2020). In some cases, even if the patient population remains unchanged, it is necessary to take into account of unexpected confounding factors in clinical studies during the COVID-19 pandemic. For example, during the pandemic, the patients may experience stress and anxiety, and thus the symptoms of any mental health disorders can worsen (Pfefferbaum and North, 2020). Therefore, the consistency of the outcome measures before and during the COVID-19 pandemic for patients needs to be assessed. For each of the participated site, “during COVID-19” can be the period when the site launched traffic control bundling/triage prior to entering hospitals due to the outbreak till it relaxed such bans and restrictions. However, the real situation may be more complicated. For example, a patient may be enrolled on the last day of the “before COVID-19” period and most of observations for that patient were collected in the “during COVID-19” period. To define the period, in addition to only considering the date of the patients enter the trial, how much data collection for the patients was influenced by the pandemic should be taken into account.
Any changes in patient populations across the three periods that cause a dramatic shift in the mean response can be addressed with the approach developed by Hartung (1999) and Chen, Hung and Hsiao (2012). Suppose we focus on a clinical trial in which a test product is compared with a placebo control on a continuous efficacy endpoint. Let Xij and Yik denote the efficacy responses for the jth subject and the kth subject in the ith patient population receiving the test product and the placebo control, respectively, i = 1, 2, 3, j = 1,…, niT, and k = 1,…, niC, where niT and niC denote the numbers of patients recruited for the test product and the placebo control respectively in the ith population. Here, i = 1, 2 and 3 indexes the patient population recruited “before COVID-19”, “during COVID-19”, and “post COVID-19”, respectively. Assume that and
, where
represents a normal distribution with mean μ and variance ζ2. Let θi denote the treatment difference in the ith patient population. That is, θi = μiT-μiC. To address the heterogeneous treatment effect across patient populations, we assume that
, i = 1, 2, 3. We wish to test the following hypotheses for the overall treatment effect:
H0: θ≦0 vs. HA: θ > 0. (1)
The method proposed below can be easily extended to the two-sided null hypothesis.
Let define the estimator of θi. It leads to
, where
and
. The general random effect model can be expressed as
where
,
, and νi and εi are assumed to be independent. The distributional assumption leads to
Thus, . Let
,
, and
be the estimators of
,
, and
, respectively. We can then see that
and
,
where and
.
Set .and let
be the estimator of
. The method of moments estimator
for
by DerSimonian and Laird (1986) is given by
where
. If a negative estimate for
is derived, the estimate
is set to 0. Again, the distributional assumptions lead to
Set and let
be the estimate of
. That is,
When we treat the term as if it were the true variance of
, by Whitehead (2002), the maximum likelihood estimate of
is given by
, where
(2)
(2)
Clearly, is asymptotically unbiased for θ, with variance approximately equal to
(Whitehead, 2002). Let
. Here S can be thought of as the between-population variance. By Hartung (1999),
can be approximated by a (central)
-distribution with 2 degrees of freedom. Again, by Hartung (1999),
and S are stochastically independent. Consequently, the test statistic for (1) under H0 can be derived as follows:
(3)
(3) where tn represents the t distribution with degrees of freedom n. Note that comparability of treatment subjects across three time periods in terms of baseline demographics and severity of illness need to be examined first. If not comparable, the proposed method may not be suitable.
Let denote the usual estimator of the overall treatment effect. That is,
A simulation study was conducted to compare the proposed estimation with . First, we assume
and
For simplicity, we also assume that
i = 1, 2, 3, and k = 1,…, niC. Three scenarios were considered. The first scenario presented the situation where homogeneous treatment effects in the primary endpoint were observed across three time periods. In this case, we assume that
, i = 1, 2, 3, and j = 1,…, niT. The second situation is that the treatment effects were the same in both “before COVID-19” and “post COVID-19” periods but no difference exists between the test drug and control for the “during COVId-19” period. Here, we assume that
, i = 1, 3, j = 1,…, niT and
, j = 1,…, 20. The third scenario is the situation where the treatment effects were the same in both “before COVID-19” and “post COVID-19” periods but negative treatment effect was found in “during COVId-19” period. We assume that
, i = 1, 3, j = 1,…, niT and
, j = 1,…, 20. Table 1 displays the averages of
and
with standard deviations (SD) from 10,000 replicates. For scenario 1, when no treatment difference in the primary endpoint exists across three time periods (i.e.,
is close to 0), it can be seen that
is very close to
. In both scenarios 2 and 3, our proposed estimates were more conservative than the usual estimates if there exists a difference in treatment effect across time periods. Intuitively, when random effect model is used,
is dominated by the
in both scenarios 2 and 3 for all i such that
’s are all very close. That is,
, while
However, this phenomenon may be changed if
’s are large.
Table 1. Simulation studies for comparisons between the proposed and the usual estimations
For simplicity, we only consider the case that the treatment effect across populations are random and
are fixed. If modifications made due to COVID-19 cause inflation or reduction in the variability of the primary response, this will turn to the Behrens-Fisher problem. In this case, an approach developed by Chiang and Hsiao (2019) can be used. Approaches need to be extended when shifts in the mean response and inflation/reduction in the variability of the primary response occur simultaneously.
To illustrate practical application for the use of our method, a hypothetical example in which a randomized, double-blind, and active-controlled clinical trial was conducted in patients with hypercholesterolemia to compare a new lowering low-density lipoprotein cholesterol (LDL-C) drug (test drug) with a placebo control is given. In this trial, the primary efficacy endpoint is the percent change from baseline in LDL-C. Unfortunately, this trial has gone through the COVID-19 pandemic. After the clinical trial is completed, the number of patient per group, and the mean percent change from baseline and standard deviation in LDL-C for each period are provided in Table 2. Note that the test statistic in (3) can only be used for testing the hypotheses (1) when heterogeneous treatment effect across the three time periods is found (or the denominator in the test statistic is close to 0). To assess the heterogeneity across time periods, the Q test statisticis used. Under the null hypothesis that those three populations are homogeneous, Q is distributed as chi-square with 2 degrees of freedom. The resulting p-value of 0.043 gives statistical evidence of heterogeneous treatment effects across the three time periods. From Table 1, we have
Table 2. Descriptive statistics of percent change from baseline in LDL-C for each population
and
Consequently, we can derive that the overall treatment effect and the observed test statistic in (3) is 9.52. Statistical significance on the overall treatment effect can be concluded since the p-value is 0.0054.
In this example, the treatment effect is in the same direction but of different magnitude across the three time periods. Such quantitative treatment-by-period interactions may not cause difficulties in the interpretation of the overall results, and a conclusion about the overall effectiveness of the test drug can thus be drawn. When the result from the “During COVID-19” time period differs in the opposite direction compared with the other two periods, we need to explore possible reasons which account for the heterogeneity of the treatment effects. In this case, it would be important to check if there are differences in baseline demographic, disease characteristics, and disease history across the time periods. The difference may be attributed to regional difference if shifting the sites of recruitment to lower-impacted countries and regions occurred.
4. Concluding remarks
The scale of the impact of COVID-19 is unprecedented, and thus, its influence is difficult to measure. Nonetheless, it will definitely affect patient recruitment and immediate patient care as well as data collection and analysis. Regardless of the resulting impact, the safety of trial participants is of utmost importance. To proceed in times like these, new technologies for conducting clinical trials are encouraged, but their feasibility and safety should be assured.
In this article, we have shared our experience in the use of remote data monitoring. Remote monitoring will allow us to effectively reach patients and continue to collect as much data as we can. The US FDA has recommended remote monitoring if site visits are no longer feasible, and thus this development is definitively on the right track for the pharmaceutical industry. Before these learnings can be generalized to a global setting for a global pandemic, the risks that could affect the quality or safety of a trial by recognizing critical data and processes, performing a risk assessment, and revising monitoring plans needs to be identified. Most importantly, technology which can allow the access of EHR for remote source document verification and virtual conferencing should be developed. However, in this day and age, a lot of great technologies are already in place. Many companies are already performing remote and decentralized monitoring (Miseta, 2020).
Some key points based on our experiences may need to be considered to implement remote monitoring. First, the remote monitoring system needs to be verified before it can be used. Secondly, pandemic-related risks such as failure of drug taken on protocol schedule, or missing data in eCRF should be identified so that sponsors can develop standardized metrics of trial operation for risk monitoring. At last, performing remote monitoring will involve much work, high costs, and additional training. Sponsors should recognize that additional costs for sites will be inevitable.
It is of interest to measure the impact of any modifications made due to COVID-19 on the trial and/or statistical procedures. However, the information on how the patient population has been changed due to COVID-19 is difficult to derive because it is possible that only a few data are available during the pandemic. On the other hand, the statistical approach we proposed is only appropriate when the impact of COVID-19 on statistical inference is substantial. That is, changes in patient populations have caused a dramatic shift in the mean response. Even if this is not the case, assessing the consistency of the treatment effect across the three subgroups or adjusting for the time trend may have to be done. Another point we wish to make is that any modifications made due to COVID-19 may also imply that the original planned sample size cannot achieve the desired power at the end of the study, see also the discussion in Akacha et al. (2020).
Patient dropout can occur at any visit during a clinical trial, resulting in a challenge in the analysis of incomplete data. Due to the COVID-19 pandemic, patients may drop out from ongoing clinical trials. However, dropouts during the outbreak attributed to the patients being put on lockdown or under quarantine (either designated-site or home quarantine) based on an official pandemic prevention policy or a state of emergency would fit the definition of missing completely at random (MAR). Currently, some studies have been adjusted to ship patients’ drugs by mail or rely on telemedicine instead of in-person consultations (Webster 2020). Telemedicine and telehealth, the practice of remote care and distribution of information, may be able to reduce the problem of missing data. However, dropouts due to patients being infected with severe syndrome or mortality may not exactly fit the classical definition of MAR (missing at random) or MNAR (missing not at random). In this case, care should be taken when handling missing data so that an unbiased assessment and interpretation of the treatment effect can be provided. Meanwhile, current studies have focused on the risk factors for severe disease and mortality in COVID-19. These risk factors include chronic disease, diabetes, cardiovascular disease and cancer (Jordan, Adab, and Cheng 2020). If ongoing trials share similar risk factors as those associated with severe COVID-19 or its associated mortality, a considerable number of dropouts may be inevitable. In this regard, adjusting the sample size to compensate for the dilution effect (reduced power) may be needed.
ACKNOWLEDGMENTS
Thanks are due to the two referees and the associate editor for their detailed, constructive and thoughtful comments and suggestions which led to a significant improvement to this paper.
References
- Chen, C.T., Hung, H.M.J., Hsiao, C.F. (2012). Design and evaluation of multi-regional trials with heterogeneous treatment effect across regions. Journal of Biopharmaceutical Statistic, 22(5):1037-1050.
- Chiang, C., Hsiao, C.F. (2019). Use of interval estimations in design and evaluation of multi-regional clinical trials with continuous outcomes. Statistical Methods in Medical Research, 28(7):2179-2195.
- DerSimonia, R., Laird, N. (1986). Meta-analysis in clinical trials. Controlled Clinical Trials 7, 177-188.
- DIA China Digital Health Community. (2020). The impact of the pandemic on clinical trials and the results of a survey of digital technology needs. China: DIA subscription.
- EMA (2020). Guidance on the management of clinical trials during the COVID-19 (Coronavirus) pandemic. Available at: https://ec.europa.eu/health/sites/health/files/files/eudralex/vol-10/guidanceclinicaltrials_covid19_en.pdf. Accessed on June 12, 2020.
- Hartung, J. (1999). An alternative method for meta-analysis. Biometrical Journal 41(8), 901-916.
- Huang, C., Wang, Y., Li, X., et al. (2020). Clinical features of patients infected with 2019 novel coronavirus in Wuhan, China. Lancet 395(10223), 497-506.
- Jordan, R. E., Adab, P., and Cheng, K. K. (2020), Covid-19: risk factors for severe disease and death. BMJ, 368:m1198, DOI: 10.1136/bmj.m1198.
- Melhem, F. (2020). The global impact of COVID-19 on clinical trials and the way forward. Industry Insight. Available at: https://www.technologynetworks.com/drug-discovery/blog/the-global-impact-of-covid-19-on-clinical-trials-and-the-way-forward-333652. Accessed on June 12, 2020.
- Meyer, R.D., et al. (2020). Statistical issues and recommendations for clinical trials conducted during the COVID-19 pandemic. Statistics in Biopharmaceutical Research https://doi.org/10.1080/19466315.2020.1779122.
- Miseta, E. (2020). COVID-19 and its impact on clinical trials. Clinical Leader. Available at: https://www.clinicalleader.com/doc/covid-and-its-impact-on-clinical-trials-0001. Accessed on June 12, 2020.
- Nature Research Custom Media. (2020). AI lends speed and precision in the urgent search for new drugs with the help of big data and artificial intelligence, clinical trials are managed with greater efficiency and precision. Available at: https://www.nature.com/articles/d42473-020-00093-0. Accessed on June 12, 2020.
- Pfefferbaum, B., and North, C. S. (2020). Mental health and the Covid-19 pandemic. New England Journal of Medicine, DOI: 10.1056/NEJMp2008017.
- US FDA. (2020). Guidance on conduct of clinical trial of medical products during COVID-19 pandemic. Available at: https://www.fda.gov/media/136238/download. Accessed on June 12, 2020.
- Webster, P. (2020). Virtual health care in the era of COVID-19. The Lancet 395(10231), 1180-1181. DOI: 10.1016/S0140-6736(20)30818-7.
- Whitehead A. (2002). Meta-analysis of Controlled Clinical Trials. New York: Wiley.
- Zhu, N., Zhang, D., Wang, W., et al. (2020). A novel coronavirus from patients with pneumonia in China, 2019. N Engl J Med 382,727-733.