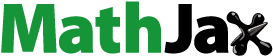
Eric Gibson is to be congratulated for a thoughtful review of the role of p-values in the assessment of the strength of evidence of research findings in pharmaceutical drug development. This perspective highlights important issues in a highly regulated environment, where study planning, protocol writing, and preregistration have been the standard for many years. It gives important insights to other disciplines, where similar standards are currently being implemented (Chambers Citation2019a, Citation2019b).
Gibson (Citation2020) mentions the “reproducibility probability” as a way to quantify the strength of evidence measured by p-values, his results are reproduced in . What is the probability that an identically designed second study (with the same sample size) will be significant, given the result from the first study? This is perhaps better referred to as the replication probability, following the distinction between reproducibility and replicability as suggested by National Academies of Sciences, Engineering, and Medicine (Citation2019), see also Goodman, Fanelli, and Ioannidis (Citation2016). We would like to comment on how this quantity can be further adjusted to give a more realistic estimate of how likely it is that a replication will again be significant.
Table 1 Comparison of Bayes factor bound, , and replication probability calibration of p-values.
In a seminal contribution, Goodman (Citation1992) showed that the replication probability solely depends on the original p-value and that it is only 50% for borderline significant studies (). In the best-case scenario, the observed effect estimate is the true effect, which is also assumed for the computation of the probabilities shown in Gibson (Citation2020). In practice, however, there is still uncertainty about the effect, and we may want to adjust the replication probability by averaging it over the distribution of the effect estimate, as also considered in Goodman (Citation1992). Incorporation of the uncertainty about the effect leads also to larger uncertainty about whether the replication will be significant. Specifically, the replication probability further decreases for significant p-values, while it increases for nonsignificant ones, see .
Although taking into account the uncertainty of the estimate may improve the calibration of the replication probability, taking a study result at face value might still not be good idea since effect estimates are often exaggerated due to publication bias and regression to the mean (as Gibson also mentions in Section 2.4). This problem is particularly severe for low powered studies, where significant findings are likely to be false positive. Copas (Citation1997) suggested a method to address this issue, shrinking the effect estimate toward zero. In short, the amount of shrinkage is where z is the standard z-statistic associated with p. The corresponding replication probabilities then decrease further, as shown in . For example, for p = 0.05, the amount of shrinkage is
and the replication probability decreases from 0.50 (without shrinkage) to 0.35, so only one in three borderline significant studies will achieve significance in a replication study.
Finally, the assumption that the true effect is exactly the same in original and replication is often inappropriate. While in theory we can think about an identically designed replication, in practice there will always be deviations from the original study, for example, the study population may differ in some characteristics. It is more reasonable to assume between-study heterogeneity of effects, as is also often done in drug development (see, e.g., Neuenschwander, Roychoudhury, and Branson Citation2018). also shows replication probabilities that were adjusted for between-study heterogeneity on top of the other adjustments. The heterogeneity parameter was chosen based on the upper limit of “negligible” heterogeneity () according to the Cochrane guidelines for systematic reviews (Deeks, Higgins, and Altman Citation2019). We can see that the replication probabilities decrease further. For example, for p = 0.0001 it decreases from unadjusted 0.97 to adjusted 0.80, the convention for a reasonable power in many fields.
Gibson (Citation2020) argues that for p-values below 0.001 replication probabilities do not calibrate as well as or Bayes factor bounds. However, this is not the case anymore after adjusting for uncertainty, regression to the mean, and heterogeneity. In an empirical investigation, we attempted to predict replication effect estimates using data from four different replication projects (Pawel and Held Citation2020). With the adjustments mentioned above, we were able to substantially improve predictive performance upon previous attempts. In fact, taking into account both regression to the mean and heterogeneity led to well calibrated predictions in two of the four datasets.
The case studies described in Gibson (Citation2020, sec. 3) are clear failures with replication effect estimates even in the wrong direction. However, quite often the effect estimates go in the same direction, but it is not clear whether the observed result can be regarded as replication success. The “two-trials rule” (Senn Citation2007) requires both studies to be significant, but can produce anomalies which do not reflect the available evidence. For example, two trials both with (two-sided) p = 0.049 (example 1 in ) will then lead to drug approval but carry less evidence for a treatment effect than one trial with p = 0.051, say, and the other one with p = 0.001. The latter, however, would not pass the two-trials rule, although its Bayes factor bound is much larger than for example 1.
Table 2 Three examples with different original and replication studies.
An alternative to the two-trials rule with better properties, the harmonic mean -test, was recently proposed (Held Citation2020b). This method produces a meta-analytic p-value pH and can be extended to more than two studies, but differs substantially from more standard meta-analytic approaches, as it requires all individual studies to be convincing to a certain degree. Using the p-value threshold
suggested by (Gibson (Citation2020, sec. 2.5), the first example would not lead to approval (
), whereas the second would (
).
Low powered original studies (with small sample size no) are not the only problem. Replication studies with relatively large sample sizes nr can also be misleading, as they may lead to significance even if the replication effect estimate is much smaller than the original one
. Let
and
denote the relative sample size and the relative effect size of replication to original study, respectively. Assume the two studies have the same primary endpoint. Under the usual normality assumption for the effect estimate combined with the standard
law for the standard error we obtain the relative effect size
(1)
(1) here zo and zr are the z-statistics of the original and replication study, respectively.
Consider now the third example with and
and assume the sample size of the replication study has been eight times as large compared to the original study, so c = 8. This sounds exaggerated, but is roughly the sample size needed to achieve 80% power to detect the effect observed in the first study accounting for the necessary shrinkage implied by regression to the mean (Pawel and Held Citation2020, Appendix S2). Then d = 0.59, so there is substantial shrinkage of the replication effect estimate. Common sense suggests that this result should be treated with more suspicion than example 2, say, where the effect estimate even increases (d = 1.69), but the p-values are virtually the same. These considerations suggest that the two-trials rule is a poor indicator of replication success (Simonsohn Citation2015).
A reverse-Bayes approach for the assessment of replication success was proposed in Held (Citation2020a), which penalizes shrinkage of the replication estimate compared to the original estimate, while ensuring that both effect estimates are statistically significant to some extent. The method takes into account not only the p-values from the two studies, but also the relative sample size c and therefore the relative effect size d via (1). A quantitative measure of the degree of replication success is proposed, the skeptical p-value pS. It quantifies the degree of conflict between the replication experiment and a skeptical prior that would make the original experiment no longer significant. gives the one-sided version of the skeptical p-value. While the interpretation of the actual value of pS requires a recalibration (Held, Micheloud, and Pawel Citation2020), it can be easily used to compare the degree of replication success of different study pairs (the smaller, the better). Interestingly, the first example with and c = 1 (and hence d = 1) is then more trustworthy (with
) than the seemingly more convincing third example with
, and c = 8 (with
). This shows how pS takes into account sample and effect sizes when assessing replication success.
We want to add a few final comments on the interpretation of the 5% level for statistical significance. It is now well accepted that p < 0.05 is a too lax criterion for a scientific discovery. Indeed, even in the absence of multiplicity issues, selective reporting, etc., gives only weak evidence against the null as quantified by the corresponding Bayes factor bound. This is why Benjamin et al. (Citation2018) have suggested the more stringent 0.005 significance threshold for claims of new discoveries. Studies with
are called “suggestive,” calling for confirmation through replication. It is worth noting that it was Fisher who said that a significant observation (at the 0.05 threshold) indicates that it is merely worth to repeat the experiment (Goodman Citation2016). This view underlines the central role of replication and has to be contrasted to the misleading, but still prevailing view that a single significant result gives “statistical proof” of a scientific claim.
Funding
Support by the Swiss National Science Foundation (Project # 189295) is gratefully acknowledged.
References
- Benjamin, D. J., Berger, J. O., Johannesson, M., Nosek, B. A., Wagenmakers, E.-J., Berk, R., Bollen, K. A., Brembs, B., Brown, L., Camerer, C., and Cesarini, D., et al. (2018), “Redefine Statistical Significance,” Nature Human Behaviour, 2, 6–10. DOI: 10.1038/s41562-017-0189-z.
- Chambers, C. (2019a), “The Registered Reports Revolution—Lessons in Cultural Reform,” Significance, 16, 23–27.
- Chambers, C. (2019b), “What’s Next for Registered Reports,” Nature, 573, 187–189.
- Copas, J. B. (1997), “Using Regression Models for Prediction: Shrinkage and Regression to the Mean,” Statistical Methods in Medical Research, 6, 167–183. DOI: 10.1177/096228029700600206.
- Deeks, J. J., Higgins, J. P., and Altman, D. G. (2019), “Analysing Data and Undertaking Meta-Analyses” (Chapter 10), in Cochrane Handbook for Systematic Reviews of Interventions, Chichester: Wiley, pp. 241–284.
- Gibson, E. W. (2020), “The Role of p-Values in Judging the Strength of Evidence and Realistic Replication Expectations,” Statistics in Biopharmaceutical Research, 1–13.
- Goodman, S. N. (1992), “A Comment on Replication, p-Values and Evidence,” Statistics in Medicine, 11, 875–879. DOI: 10.1002/sim.4780110705.
- Goodman, S. N. (2016), “Aligning Statistical and Scientific Reasoning,” Science, 352, 1180–1181.
- Goodman, S. N., Fanelli, D., and Ioannidis, J. P. A. (2016), “What Does Research Reproducibility Mean?,” Science Translational Medicine, 8, 341ps12. DOI: 10.1126/scitranslmed.aaf5027.
- Held, L. (2020a), “A New Standard for the Analysis and Design of Replication Studies” (with discussion), Journal of the Royal Statistical Society, Series A, 183, 431–469.
- Held, L. (2020b), “The Harmonic Mean χ2 Test to Substantiate Scientific Findings,” Journal of the Royal Statistical Society, Series C, 69, 697–708.
- Held, L., Micheloud, C., and Pawel, S. (2020), “The Assessment of Replication Success Based on Relative Effect Size,” Technical Report, arXiv no. 2009.07782.
- National Academies of Sciences, Engineering, and Medicine (2019), Reproducibility and Replicability in Science, Washington, DC: The National Academies Press.
- Neuenschwander, B., Roychoudhury, S., and Branson, M. (2018), “Predictive Evidence Threshold Scaling: Does the Evidence Meet a Confirmatory Standard?,” Statistics in Biopharmaceutical Research, 10, 76–84.
- Pawel, S. and Held, L. (2020), “Probabilistic Forecasting of Replication Studies,” PLOS ONE, 15, e0231416. DOI: 10.1371/journal.pone.0231416.
- Senn, S. (2007), Statistical Issues in Drug Development (2nd ed.), Chichester: Wiley.
- Simonsohn, U. (2015), “Small Telescopes: Detectability and the Evaluation of Replication Results,” Psychological Science, 26, 559–569. DOI: 10.1177/0956797614567341.