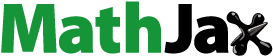
Abstract
The role of hypothesis testing, and especially of p-values, in evaluating the results of scientific experiments has been under debate for a long time. At least since the influential article by Ioannidis (Citation2005) awareness is growing in the scientific community that the results of many research experiments are difficult or impossible to replicate. Often, the (mis-)use of hypothesis testing is blamed for the lack of replicability. In 2016, the American Statistical Association (ASA) published a “Statement on Statistical Significance and p-Values” (Wasserstein and Lazar Citation2016), which led to continued scientific engagement and discussions. In this editorial, we summarize recent discussions on hypothesis testing, p-values and decision making, particularly in biopharmaceutical research, and share our views on these issues.
1 Context of ASA Statement
A series of articles appearing between 2010 and 2014 (see Wasserstein and Lazar Citation2016 and the references therein), including the widely cited Nature article by Nuzzo (Citation2014), led to a renewed discussion among members of the ASA Board of Directors about p-values and their role in inferential statistics, and scientific research, more broadly. The lack of replicability of published results had long been a concern for statisticians and just added fuel to the p-value fire. But when a psychology journal decided to take things a step further and ban p-values from all published articles (Trafimow and Marks Citation2015), the Board felt some action was needed. A task force was convened that reflected the broad expertise and variety of viewpoints held by ASA members. Their charge was to develop an official statement about statistical significance and p-values that would be issued on behalf of the ASA. After much discussion, several drafts, and one very long meeting, the committee agreed upon a formal statement, “clarifying several widely agreed upon principles underlying the proper use and interpretation of the p-value.” The statement was published in The American Statistician (TAS), along with an online supplement containing over 20 commentaries in response (Wasserstein and Lazar Citation2016).
The fact that the committee was able to reach common ground on the p-value statement, while still holding strong differences of opinion about the impact of that statement and what should follow, motivated the ASA to organize a conference to discuss next steps. The ASA Symposium on Statistical Inference was held in October 2017 (https://ww2.amstat.org/meetings/ssi/2017/). Speakers from academia, government, and industry were invited to speak on ways that p-values could be used to avoid misinterpretation, as well as alternatives to p-values in making inferences and decisions. The ASA statement emphasized how not to use p-values, so it was time to turn attention to what could be done instead. Subsequently, TAS published a special issue on “Statistical Inference in the 21st Century: A World Beyond p < 0.05” as a supplemental issue in 2019, with 43 articles targeting all users of p-values, regardless of profession, and providing a widely varying range of approaches. The editorial accompanying the special issue included a provocative call to action: “We conclude, based on our review of the articles in this special issue and the broader literature, that it is time to stop using the term ‘statistically significant’ entirely” (Wasserstein, Schirm, and Lazar Citation2019). The editorial goes on to give four principles for action in a post-statistical-significance world: “Accept uncertainty. Be thoughtful, open, and modest (ATOM).”
The aftermath of this call to action has included statements from journal editors describing how they plan to address the use of p-values in journal submissions going forward; see, for example, the guidelines issued from The New England Medical Journal (Harrington et al. Citation2019) and the editorial published by Clinical Trials (Cook et al. 2018). Research organizations, particularly those that rely on the concept of significant findings to make policy decisions, such as regulatory agencies, have undertaken discussions about the ASA p-value statement and the ideas for alternative approaches espoused in the special issue of TAS. Additionally, reports of some confusion surfaced about whether the special issue in general, and its editorial in particular, constituted an official statement of the ASA. It is clear from the editorial itself that the call to action represents the views of the three editors. It is also clear that a very important dialogue has been initiated about how we base decisions on inferential statistics. This issue of Statistics in Biopharmaceutical Research is a testament to how rich that dialogue is.
2 Roles of Hypothesis Testing and p-Values in Biopharmaceutical Research
In biopharmaceutical research, the chance that a clinical study will lead to erroneous conclusions is important to assess. For example, in confirmatory studies, controlling the chance of erroneous conclusions of safety or effectiveness (and incorrect conclusions of lack of safety or effectiveness) is critical for appropriate regulatory decision making. In exploratory studies, it may be of interest to control the chance of other types of erroneous conclusions, such as the chance of selecting an inappropriate or suboptimal dose. While the predominant approaches to the design and analysis of clinical studies have been based on frequentist statistical methods, this should not be taken to imply that other approaches are not suitable (ICH Citation1998).
2.1 Significantosis
Unfortunately, overreliance on p-values can lead to pathologies in researchers. In the 1990s, Akira Sakuma (Emeritus Professor at Tokyo Medical and Dental University) ironically referred to this overreliance as “significantosis” (“Yuisho” in Japanese). Symptoms of this “disease” are the beliefs that an effect is clinically significant if p < 0.05 and clinically insignificant if p > 0.05 (Sakuma Citation1999). Disease severity ranges from mild cases, where p is near 0.05, to severe cases, where the fate of the company or the research institute seems to be at risk if People suffering from “significantosis” often tend to “torture” or “manipulate” data by repeatedly performing data analyses (e.g., subgroup analyses) until a desired result is obtained, without a proper understanding of the population analyzed, the magnitude of the effects, and the statistical methods used for the analysis. Put another way, hypothesis tests and p-values can be useful, but cannot be over-relied upon to adequately interpret the results of clinical studies. Sakuma points out that researchers with “significantosis” can become immune to it after learning about how to contextualize and interpret clinical study results. It is very important to keep in mind that statistical significance is only one aspect of a medical product evaluation, be it a drug, biological product, medical device or diagnostic agent.
2.2 Regulatory Decision Making
Reasonable assurance that a medical product is safe and effective is based on valid and substantial scientific evidence. Valid scientific evidence can come in many forms, but is typically developed from adequate and well-controlled clinical studies. Strength of evidence is evaluated with appropriate statistical methods for quantifying uncertainty. Statistical significance, as determined by the p-value, is not the only accepted measure of the strength of evidence. For example, when appropriate, a high posterior probability of effectiveness (say) could also be considered as sufficient evidence (FDA Citation2010, Citation2019).
When deciding whether a study on a medical product can be used to support approval, a regulatory agency almost never relies solely on the p-value for the primary objective. On the contrary, the agency considers the totality of evidence for safety and effectiveness. Multiple safety and efficacy endpoints may be analyzed. Evaluation of the product in subgroups is often necessary to demonstrate generalizability of benefit and safety. A statistically significant result found in one study may need to be replicated in another study to show that it is generalizable. Sources of bias in study design, conduct, or analysis may override the p-value or other evidence summaries in the final determination of the regulatory decision. In short, multiple evaluations are commonly necessary to answer three basic questions: Is the product safe? Is it effective? Do its probable benefits outweigh its probable risks?
Nonetheless, the p-value has occupied a special place in influencing regulatory decision making on whether or not to approve a medical product for marketing. Typically, hypothesis tests are used to support objectives of clinical studies. A null hypothesis is rejected if the p-value is less than the significance level of the test. For clinical studies used to support approval, the U.S. Food and Drug Administration (FDA), as well as other regulatory bodies such as the European Medical Agency (EMA) or the Pharmaceuticals and Medical Devices Agency (PMDA), frequently recommend that the maximum probability of rejecting the null hypothesis when it is true (i.e., making a Type I error) be controlled at a specified significance level, say 0.025 (one-sided), for the primary objective(s) of a clinical study (ICH Citation1998). The sponsor of the medical product may also be asked to control the familywise Type I error rate among hypothesis tests of any secondary objectives, if the outcomes of the hypothesis tests will be used to support claims in labeling if and when the product is approved (FDA Citation2017b). By emphasizing Type I error rate control, regulatory agencies such as the FDA, EMA, and PMDA limit their long run probability of erroneously approving an ineffective or unsafe product.
Of course, controlling the proportion of true nulls that get rejected is just one operating characteristic that emphasizes protecting the public from ineffective or unsafe medical products. The proportion of false nulls that are rejected (i.e., power) should also be controlled to promote access to safe and effective products. Moreover, the posterior probability that a null is true given the data would be desirable to compute and would be available via Bayes’ theorem if the prior probability of the null can be quantified.
In the United States, considerable flexibility may be exercised in the types of data and evidence that may considered to support limited or full marketing of a medical product. For example, a drug that meets an unmet medical need may be granted accelerated approval based on a surrogate endpoint (e.g., an intermediate endpoint) that is reasonably likely to predict clinical benefit, with the understanding that the applicant will provide additional evidence to support eventual full approval, usually with other study(ies) on clinical endpoint(s). Other program areas in which flexibility may be exercised include expedited access of a medical device to address an unmet medical need, breakthrough designation of a medical device technology that offers advantages over approved or cleared alternatives, rare or neglected disease population, pediatric population, laboratory developed test, and emergency use authorization (FDA Citation2017a). For medical devices, the requisite level of evidence (i.e., regulatory control) depends on the risk category (I, II, or III) of the device class (Code of Federal Regulations Title 21, Section 360c, and Section 812 Parts 862–892).
2.3 Decision Making Within Development Programs and Clinical Significance
In the wider context of medical product development, the use of p-values is also pervasive in guiding internal decision making by pharmaceutical companies, such as establishing proof-of-concept, selecting a dose regimen, or determining if a biomarker can be useful for predicting clinical response. In contrast to confirmatory studies, when regulatory guidance/precedents drive the reliance on p-values to establish the strength of evidence in the results, there is considerably more latitude on what approach sponsors may use to decide what action to take based on results observed in an exploratory study. Perhaps because statisticians involved in the design and analysis of such studies are often also involved in confirmatory studies, it is common practice to apply a hypothesis testing framework to decision problems that can be more efficiently (and informatively) tackled via alternative approaches, such as modeling and utility functions.
Overreliance on p-values to drive decision making in early development studies, which often use small sample sizes and not (yet) well-studied endpoints, often leads to designs and decision approaches with poor statistical and clinical properties. For example, to stay within the limited budget available for such studies, teams often use (one-sided) significance levels in the range of 0.1–0.2 and power the study for unrealistically high effects. The inferential value of such studies is debatable at best and their pervasive utilization may partly explain the high failure rate observed in late development studies (the replicability crisis mentioned earlier). Alternative strategies to move internal decision making studies away from the rigid hypothesis testing/p-value framework have been proposed in the literature (e.g., Bornkamp et al. Citation2007; Chuang-Stein et al. Citation2011) and are gaining increasing traction in medical product development.
Finally, statistical significance does not necessarily imply clinical significance. In many studies, the p-value is used to test the null hypothesis that a drug (or other medical product) has no effect on an outcome. The p-value is the smallest significance level at which the null hypothesis of no effect would be rejected, that is, a real effect would be concluded. However, if a study is sufficiently large, a statistically significant observed effect may be too small to be considered clinically meaningful. In short, statistical significance is necessary but not sufficient for demonstrating that a medical product provides clinically significant benefit. Moreover, p-values are often difficult for clinicians to interpret. A p-value is a scaled measure between 0 and 1 of the evidence against a null hypothesis. Scaled measures of evidence are easily compared across studies. However, clinicians are often best served by providing evidence summaries in the units of the clinical endpoint. Confidence intervals are the standard-bearer for quantifying the uncertainty of the size of an effect on an outcome. They provide information in the units of the outcome that can be much more valuable for interpreting study results than merely the p-value. For Bayesian analyses, the analogue of a confidence interval is a credible interval of the likely values of the true effect. Bayesian credible intervals are commonly constructed on the basis of either central posterior probability, or highest posterior density.
3 Alternatives to Hypothesis Testing and p-Values
The previous section highlights the limitations of p-values, especially for smaller studies. However, despite the broad and by now long-standing agreement about the limitations of p-values and the use of significance bounds, p-values still remain the most commonly used standard to judge research findings. One of the reasons for this apparent paradox is the difficulty of establishing a convincing alternative that allows comparable ease of implementation (or at least seemingly easy implementation). There is, however, no lack of proposals for such alternatives. Perhaps the easiest fix to address unacceptably high false positive rates (i.e., false null hypothesis rejections) is the reduction of customary significance thresholds. For example, Benjamin et al. (2018) advocated to reduce a significance threshold of p < 0.05 to p < 0.005, arguing that this corresponds to more convincing Bayes’ factors in favor of the alternative hypothesis, H1, and that it would significantly reduce the false positive rate. The main attraction of such a move is that it would require procedurally almost no change and might therefore have the best chance for quick and wide acceptance. Of course, the limitation is that conceptual problems of using p-values would remain unaddressed (e.g., the difference between 0.0049 and 0.0051 is no easier to explain than the difference between 0.049 and 0.051).
An alternative and, from a Bayesian perspective, coherent way of implementing tests is based on Bayes’ factors, that is, the odds of observing the data under the null hypothesis, H0, versus observing it under H1 (or vice versa). Let B01 denote the Bayes’ factor. For composite hypotheses, like , the evaluation of the Bayes’ factor requires a prior distribution to define the appropriate averaging of the probability of observing the data. This dependence on a prior distribution gives rise to a minor complication in the interpretation, but can easily be overcome by establishing bounds, say
, where BFB is short for “Bayesian factor bound.” Figure 3 in Bayarri et al. (Citation2016) reproduces Figures 1–3 from Ioannidis (Citation2008), adding the simple bound
. The plots are based on more than 500 articles published across different fields. The bound is amazingly sharp for these 500 studies, providing empirical evidence for the relevance of this BFP. For example, for a p-value of 0.05, the Bayes’ factor is at most 2.46 in favor of the alternative (i.e.,
). This observation is one of the arguments for moving a significance threshold to p < 0.005, which moves the same bound to 13.9. Alternatives to the
bound are reviewed, for example, in Held and Ott (Citation2018).
The contrast between the suggested conclusions using p-value thresholds versus Bayes factors has long been observed in the literature, including, for example, Lindley (Citation1957), and leading Johnstone and Lindley (Citation1995) to argue that a small p-value under a large sample size could even be interpreted as evidence in favor of H0. For example, under a particular setup they argue that p = 0.05 could even imply a Bayes factor substantially below the mentioned BFB of 2.46, and even be in favor of H0.
One of the reasons underlying the limitations of p-values is the way in which p-values implicitly trade off the probability of a significant effect and the effect size into a single-number summary. Perhaps a more natural and more principled approach to mapping multiple criteria into a single number summary is an explicit characterization of the report of a test as a decision problem. A decision problem is characterized by an action set, a utility (or loss) function that reflects the decision maker’s relative preferences over actions, hypothetical truth and outcomes, and a probability model over all unknown quantities. In the case of a testing problem the action set is , that is, reporting H0 or H1. The unknown truth is represented by parameters θ, and the relevant probability model is the posterior or posterior predictive distribution—the latter in case the utility function involves future outcomes. Murray, Thall, and Yuan (Citation2016), for example, described how to set up inference for randomized clinical studies as a decision problem. The utility function balances between an efficacy outcome and a toxicity outcome. Lee, Thall, and Rezvani (Citation2019) developed a dose-finding design with five co-primary outcomes for six prognostic subgroups. In the context of medical product development in a Phase 2 study, the utility function could naturally involve a consideration of future success in a following confirmatory study. This is discussed, for example in Graf, Posch, and König (Citation2015) who introduced alternative utility functions characterized as “sponsor view” versus “public health view.” In general, a natural choice for a utility function for a stop-or-go type of decision could incorporate the size of the effect, a future significant effect, or even details like the availability of alternative treatment options for specific patient populations or the feasibility of conducting the next trial with sufficient power to be interpretable.
4 Concluding Remarks
In summary, echoing other editorials (Cook et al. 2018; Harrington et al. Citation2019), hypothesis testing and p-values are still essential in assessing evidence from clinical studies. They are not, however, sufficient to interpret and understand study results in a comprehensive way, in particular when characterizing the effect of a medical product quantitatively. As seen in the previous section, alternatives to hypothesis testing and p-values have been proposed in the literature. There are, however, no perfect statistical methods, and understanding their advantages and limitations is critical. Just as the results of hypothesis tests and p-values are often misunderstood and misused if nonstatisticians are not properly trained and educated, there is a risk that any alternative method will ultimately be equally misunderstood or misused. To promote good statistical practices in our biopharmaceutical research community, Statistics in Biopharmaceutical Research will continue its efforts to help statisticians better communicate the advantages and limitations of the methods they use as well as nonstatisticians better understand statistics and statistical methods used in medical product development, including the responsible use of hypothesis testing, p-values, confidence intervals, posterior probabilities and other inferential tools.
We hope that the article by Gibson (Citation2021) in this issue, with the accompanying discussions by distinguished researchers, will stimulate further discussions in this regard. The Editorial Board of Statistics in Biopharmaceutical Research encourages article submissions on alternative solutions and perspectives on this important problem.
Acknowledgments
We thank Professor T. Shun Sato of Kyoto University for sharing the story of “Significantosis” with us. We also thank Drs. Thomas Gwise, John Scott, and Berkman Sahiner of the FDA for reviewing a previous version of this editorial and providing comments that led to improvement of its content.
References
- Bayarri, M., Benjamin, D. J., Berger, J. O., and Sellke, T. M. (2016), “Rejection Odds and Rejection Ratios: A Proposal for Statistical Practice in Testing Hypotheses,” Journal of Mathematical Psychology, 72, 90–103. DOI: 10.1016/j.jmp.2015.12.007.
- Benjamin, D. J., Berger, J. O., Johannesson, M., Nosek, B. A., Wagenmakers, E., Berk, R., Bollen, K. A., Brembs, B., Brown, L., Camerer, C., Cesarini, D., Chambers, C. D., Clyde, M., Cook, R. D., De Boeck, P., Dienes, Z., Dreber, A., Easwaran, K., Efferson, C., Fehr, E., Fidler, F., Field, A. P., Forster, M., George, E. I., Gonzalez, R., Goodman, S., Green, E., Green, D. P., Greenwald, A. G., Hadfield, J. D., Hedges, L. V., Held, L., Ho, T. H., Hoijtink, H., Hruschka, D. J., Imai, K., Imbens, G., Ioannidis, J. P. A., Jeon, M., Jones, J. H., Kirchler, M., Laibson, D., List, J., Little, R., Lupia, A., Machery, E., Maxwell, S. E., McCarthy, M., Moore, D. A., Morgan, S. L., Munafó, M., Nakagawa, S., Nyhan, B., Parker, T. H., Pericchi, L., Perugini, M., Rouder, J., Rousseau, J., Savalei, V., Schönbrodt, F. D., Sellke, T., Sinclair, B., Tingley, D., Van Zandt, T., Vazire, S., Watts, D. J., Winship, C., Wolpert, R. L., Xie, Y., Young, C., Zinman, J., and Johnson, V. E. (2018), “Redefine Statistical Significance,” Nature Human Behaviour, 2, 6–10. DOI: 10.1038/s41562-017-0189-z.
- Bornkamp, B., Bretz, F., Dmitrienko, A., Enas, G., Gaydos, B., Hsu, C. H., König, F., Krams, M., Liu, Q., Neuenschwander, B., Parke, T., Pinheiro, J., Roy, A., Sax, R., and Shen, F. (2007), “Innovative Approaches for Designing and Analyzing Adaptive Dose-Ranging Trials,” Journal of Biopharmaceutical Statistics, 17, 965–995. DOI: 10.1080/10543400701643848.
- Chuang-Stein, C., Kirby, S., French, F., Kowalski, K., Marshall, S., Smith, M. K., Bycott, P., and Beltangady, M. (2011), “A Quantitative Approach for Making Go/No-Go Decisions in Drug Development,” Drug Information Journal, 5, 187–202. DOI: 10.1177/009286151104500213.
- Cook, J. A., Fergusson, D. A., Ford, I., Gönen, M., Kimmelman, J., Korn, K. L., and Beg, C. B. (2019), “There Is Still a Place for Significance Testing in Clinical Trials,” Clinical Trials, 16, 223–224. DOI: 10.1177/1740774519846504.
- FDA (2010), “Food and Drug Administration, Guidance for the Use of Bayesian Statistics in Medical Device Clinical Trials,” available at https://www.fda.gov/regulatory-information/search-fda-guidance-documents/guidance-use-bayesian-statistics-medical-device-clinical-trials-pdf-version.
- FDA (2017a), “Food and Drug Administration, Emergency Use Authorization of Medical Products and Related Authorities: Guidance for Industry and Other Stakeholders, January 2017,” available at https://www.fda.gov/regulatory-information/search-fda-guidance-documents/emergency-use-authorization-medical-products-and-related-authorities.
- FDA (2017b), “Food and Drug Administration, Multiple Endpoints in Clinical Trials Guidance for Industry, January 2017,” available at https://www.fda.gov/regulatory-information/search-fda-guidance-documents/multiple-endpoints-clinical-trials-guidance-industry.
- FDA (2019), “Food and Drug Administration, Demonstrating Substantial Evidence of Effectiveness for Human Drug and Biological Products: Guidance for Industry. Draft Guidance December 2019,” available at https://www.fda.gov/regulatory-information/search-fda-guidance-documents/demonstrating-substantial-evidence-effectiveness-human-drug-and-biological-products.
- Gibson, E. W. (2021), “The Role of p-Values in Judging the Strength of Evidence and Realistic Replication Expectations,” Statistics in Biopharmaceutical Research, 13, DOI: 10.1080/19466315.2020.1724560.(this issue).
- Graf, A. C., Posch, M., and König, F. (2015), “Adaptive Designs for Subpopulation Analysis Optimizing Utility Functions,” Biometrical Journal, 57, 76–89. DOI: 10.1002/bimj.201300257.
- Harrington, D., D’Agostino, R. B., Sr., Gatsonis, C., Hogan, J. W., Hunter, D. J., Normand, S. L. T., Drazen, J. M., and Hamel, M. B. (2019), “New Guidelines for Statistical Reporting in the Journal,” The New England Journal of Medicine, 381, 285–286. DOI: 10.1056/NEJMe1906559.
- Held, L., and Ott, M. (2018), “On p-Values and Bayes Factors,” Annual Review of Statistics and Its Application, 5, 393–419. DOI: 10.1146/annurev-statistics-031017-100307.
- ICH (1998), “Topic E9 on Statistical Principles for Clinical Trials,” available at www.ich.org.
- Ioannidis, J. P. A. (2005), “Why Most Published Research Findings Are False,” PLoS Medicine, 2, e124. DOI: 10.1371/journal.pmed.0020124.
- Ioannidis, J. P. A. (2008), “Effect of Formal Statistical Significance on the Credibility of Observational Associations,” American Journal of Epidemiology, 168, 374–383.
- Johnstone, D. J., and Lindley, D. V. (1995), “Bayesian Inference Given Data ‘Significant at α’: Tests of Point Hypotheses,” Theory and Decision, 38, 51–60. DOI: 10.1007/BF01083168.
- Lee, J., Thall, P., and Rezvani, K. (2019), “Optimizing Natural Killer Cell Doses for Heterogeneous Cancer Patients on the Basis of Multiple Event Times,” Journal of the Royal Statistical Society, Series C, 68, 461–474. DOI: 10.1111/rssc.12271.
- Lindley, D. V. (1957), “A Statistical Paradox,” Biometrika, 44, 187– 192. DOI: 10.1093/biomet/44.1-2.187.
- Murray, T., Thall, P., and Yuan, Y. (2016), “Utility-Based Designs for Randomized Comparative Trials With Categorical Outcomes,” Statistics in Medicine, 35, 4285–4305. DOI: 10.1002/sim.6989.
- Nuzzo, R. (2014), “Scientific Method: Statistical Errors,” Nature, 506, 150–152. DOI: 10.1038/506150a.
- Sakuma, A. (1999), “Statistics Anecdotes,” in Clinical Trials in the 21st Century, eds. H. Tsubaki, T. Fujita, and T. Sato, Tokyo: Asakura Publishing (in Japanese).
- Trafimow, D., and Marks, M. (2015), “Editorial,” Basic and Applied Social Psychology, 37, 1–2, DOI: 10.1080/01973533.2015.1012991.
- U.S. Code of Federal Regulations, available at https://uscode.house.gov/.
- Wasserstein, R. L., and Lazar, N. A. (2016), “The ASA Statement on p-Values: Context, Process, and Purpose,” The American Statistician, 70, 129–33. DOI: 10.1080/00031305.2016.1154108.
- Wasserstein, R. L., Schirm, A. L., and Lazar, N. A. (2019), “Moving to a World Beyond ‘p<0.05 ’,” The American Statistician, 73, 1–19.