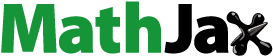
Abstract
Two pivotal, randomized, double-blind studies in a chronic dermatology disease were ongoing when the COVID-19 pandemic broke out. The trials had identical design, with four parallel treatment arms: two doses of active drug, an active comparator and placebo. When recruitment was temporarily halted due to the pandemic, both trials had already achieved over 80% of the targeted sample size. The unprecedented and unpredictable dynamic of the pandemic caused concerns about potential risks to data integrity and interpretability, which needed to be addressed. In this article, we describe some of these challenges and mitigation strategies. In particular, the complex confirmatory situation involving multiple active doses, endpoints and comparators led to a careful modification of the testing scheme to maintain the power of key endpoints and comparisons while still controlling the relevant Type I error rates. Specifically, the strategy was adjusted to perform some lower-level statistical tests in the hierarchy on the pool of both studies, taking advantage of the replicate study design. Primary endpoint comparisons against placebo and active control remained separately by study. In this article, we share our ideas and experiences in devising this risk mitigation proposal for the statistical methodology of these pivotal trials during the COVID-19 pandemic.
1 Introduction
The COVID-19 pandemic has disrupted normalcy of life, work and research on a scale that could not be anticipated. Clinical trials in particular have been facing a multitude of issues, such as logistical (e.g., missed study visits/doses due to a lockdown), medical (e.g., COVID-19 infections), statistical (e.g., analyses of COVID-19 impact on study conduct and conclusions)— or a combination of all. In practice, these issues have manifested in clinical studies to a varying degree, sometimes negligible, sometimes massive. Sponsors need to define mitigation strategies quickly, while studies are running, and not knowing whether any present issue will aggravate or vanish, or which new challenges might appear. At the same time, these strategies must be logistically feasible, scientifically sound, aligned across multiple stakeholders (including health authorities), and producing interpretable trial results. This can be a daunting task.
A number of interesting and innovative case studies, situations, or mitigation strategies have already been put forward. Dequin et al. (Citation2020), for example, used an ongoing pneumonia trial to their advantage, embedding a COVID-19 subtrial into it. Mütze and Friede (Citation2020) discuss strategies and experiences of setting up Data Safety Monitoring Boards (DSMBs) for COVID-19 trials. Geerts and van der Graaf (Citation2020) propose methods for salvaging clinical trials in central nervous system disorders which are halted due to COVID-19, using physiologically based pharmacokinetics (PBPK) and quantitative systems pharmacology (QSP). Akacha et al. (Citation2020) and Meyer et al. (Citation2020) provide comprehensive discussions of challenges and recommendations for clinical trials in light of the COVID-19 pandemic. Importantly, the U.S. Food and Drug Administration (FDA) as well as the Committee for Medicinal Products for Human Use (CHMP) of the European Medicines Agency (EMA) have issued several guidance documents (FDA 2020; EMA/CHMP 2020b, Citation2020a). In this article we describe a case study, consisting of two identical pivotal clinical trials in a chronic dermatology disease, in which we encountered the above-described challenges and found innovative mitigation strategies. At the time of writing this article, the trials are still ongoing. While the proposed solutions are motivated by, and tailored to, the situation of this particular case study, some may be applicable more broadly, even outside the COVID-19 pandemic.
The remainder of this article is structured as follows. In Section 2, we lay out the situation of this case study and the challenges caused by the COVID-19 pandemic. Section 3 describes the mitigation strategies proposed to address these challenges. Section 4 covers the related confirmatory testing schemes in detail, and Section 5 shows related power simulation results. The article closes with a discussion in Section 6.
2 Situation and Challenges
The current Phase 3 development program for a new compound in a chronic dermatology disease consists of two replicate, randomized, double-blind, active- and placebo-controlled studies of 1 year treatment duration. The primary endpoint of these studies is the change from baseline in a symptom score based on patient self-reported diary assessments. Several secondary endpoints are also part of the confirmatory testing scheme. The primary endpoint is based on the efficacy assessments at week 12. The comparisons versus placebo and active control are both included in the hierarchical testing strategy, for the primary and four secondary endpoints. The phase 3 program plans to enroll approximately 2100 participants (1050 per study) in more than 400 clinical study sites across five continents. The purpose of these studies is to establish the efficacy and safety of two doses of the new compound in the targeted indication.
Multi-center recruitment for the two pivotal studies started in October 2018 and was well-advanced when the COVID-19 pandemic was declared in March, 2020 by the World Health Organization. In light of the pandemic control measures which included restrictions on movement, prioritization of healthcare infrastructure to cope with COVID-19 related medical needs, and assurance of trial participant safety, Novartis placed the two studies as well as most other ongoing clinical studies on temporary recruitment halt in April 2020. At the time of recruitment halt, more than 80% of the total planned participants were enrolled in the two pivotal studies. Participants who were in screening when recruitment had been temporarily paused were allowed to continue in the study, where feasible. Recruitment was cautiously resumed in May 2020 in selected countries and sites after thorough evaluation of the patients’ benefits and risks based on the local COVID-19 situation. However, it was clear that the ongoing nature of the COVID-19 pandemic could cause further unforeseen challenges to the recruitment of trial participants, and an increase in COVID-19 related protocol deviations (e.g., missed visits, missed doses, etc.) appeared likely. Already, difficulties were observed, such as missed or delayed visits by trial participants due to travel restrictions, nonavailability of site staff, countries in quarantine, etc. It could also not be excluded that another trial halt would become necessary.
In the normal course of a clinical trial, the composition of the patient population at participating regions/centers is expected to be homogeneous with respect to demographics and comorbidities throughout the duration of the study, with no major perturbations. Analysis plans are therefore set under the assumption of no major disruptive events occurring that may affect the entire trial population and introduce nonrandom heterogeneity. However, the COVID-19 pandemic is an example of such an unanticipated disruptive event. Its potential impact on the homogeneity of the data collected is hard to anticipate. The demographics of the pre-, during- and post-COVID-19 study population could be potentially inconsistent causing additional challenges for the analyses.
In this context, EMA provided guidance in the “Points to consider on implications of Coronavirus disease (COVID-19) on methodological aspects of ongoing clinical trials” (EMA 2020b). The document recognizes that:
“ measures taken in relation to the COVID-19 pandemic may interfere with study treatments, study assessment schedule and individual participants’ observation time. It can be expected that study participants within a certain trial will be unequally affected by such general (i.e. external to the trial) COVID-19 pandemic measures.”
It suggests to consider, among other measures, “proposals to deal with any identified potential sources of bias comprising identification of newly emerging intercurrent events or missing values, or other unforeseeable required changes to trial elements,” as well as, potentially, “the need to adjust the trial sample size.” FDA guidance (FDA 2020) stipulates that “sponsors should also proactively plan to address the impact of COVID-19 on the ability to meet the trial objectives.” And in particular: “For sponsors considering stopping a trial and conducting a final analysis, a major consideration is the loss of statistical power from a smaller sample size or less follow-up time than was anticipated.”
In this case study, the sponsor considered three such “changes to trial elements” (EMA 2020b), including the definition of a new intercurrent event and a change (reduction) in study population for the primary analysis. In this article, we focus mainly on the third, that is, a change in the confirmatory testing scheme.
3 Mitigation Strategies
With more than 80% of the targeted sample size already recruited in both trials, and given the unpredictable nature of the emerging pandemic, the sponsor initially considered restricting the primary analysis to those patients enrolled before the recruitment halt due to COVID-19. This would also mitigate any risk of heterogeneity in the pre- vs. during/post-pandemic population. The analysis of the primary endpoint against placebo would still retain sufficient power since the original sample size had been chosen large enough even for the comparisons against the active comparator. These latter comparisons, however, would no longer be sufficiently powered when restricted to the pre-pandemic population. These comparisons against the active comparator were therefore initially planned to be carried out on the pool of both trials, as part of the pre-specified hierarchical testing strategy. This meant the introduction of an overarching confirmatory testing scheme across both trials. In essence, the idea was to perform all hypothesis tests against placebo in each individual trial (similar to the original trial protocols), and to perform the hypothesis tests against the active comparator based on pooled data from both trials. (An additional minor variation was to propagate half of the available Type I error in the sequence of placebo comparisons to the respective other dose before reaching the last two endpoints in the hierarchy. We include this in the testing schemes below without further commenting on it.) The confirmatory testing scheme was devised such that the family-wise Type I error was controlled at 2.5% (one-sided) at the study level for the tests against placebo and (simultaneously) at the submission level for the tests against the active comparator. In this context, it should be emphasized that the two trials were identical in design and recruiting from practically the same countries, using identical eligibility criteria. In addition, any analysis performed on the pool of both studies would also be done in each study separately, to check consistency and establish a notion of replication.
When recruitment resumed after the temporary halt, it gradually improved such that the full trial population could be recruited without further major disruptions. This allowed the sponsor to settle on a different mitigation strategy than the one originally envisioned. Concretely, all safety and efficacy assessments would still be done on 100% of the trial population. The confirmatory testing strategy would perform not only the comparisons against placebo separately by study, but also the primary endpoint comparison against the active comparator. However, given the ongoing pandemic and its unpredictable impact on this complex network of hypothesis tests with multiple doses, treatment arms and endpoints, the idea of using the pool of both studies for low-power comparisons was retained for subordinate secondary endpoints tested against the active comparator, controlling the Type I error on the submission level for these. This will de-risk the potentially larger variation caused by the pandemic for the overall conclusion of the treatment effect.
In the following, we describe in detail these three confirmatory testing strategies: the one defined in the original trial protocols; the overarching scheme first considered to mitigate the impact of the COVID-19 pandemic; and the one finally proposed, which constitutes a middle ground between the two earlier ones. The central idea is to create a robust and rigorous overarching pivotal analysis of these trials which restores or even improves upon the originally intended statistical power despite the disruptions of the COVID-19 pandemic, leveraging the opportunity of having two Phase III studies with identical design conducted in the same countries.
4 Confirmatory Testing Schemes
The confirmatory testing strategy includes five endpoints (1: primary, 2-5: secondary), two doses of the experimental drug (l: low, h: high), and two comparators (p: placebo, c: active control). The null hypotheses pertaining to any combination of these elements are designated accordingly, starting with the letter “H.” For example, H2lp is the null hypothesis that there is no difference in treatment effect between the low dose and placebo with respect to the first secondary endpoint.
4.1 Original Option: Hierarchical Testing Strategy Based on the Individual Studies
displays the confirmatory testing scheme for these hypotheses as set out in the original trial protocols, separately and identically for each trial, using the graphical approach by Bretz et al. (Citation2009). The family-wise error was set to (one-sided). The hypotheses are mapped into two branches such that hypotheses within a branch correspond to the same dose of the experimental drug (low or high), and the Type I error is propagated along the arrows when null hypotheses are rejected. In essence, the Type I error probability was equally split for both branches, and within each branch the hypotheses are tested sequentially, with the primary endpoint first tested against placebo and the active comparator, followed by two sub-branches, testing the secondary endpoints against placebo and the active comparator. After successful rejection of all null hypotheses for one dose, the Type I error level can be passed to the other dose.
4.2 Modified Option 1—Pooling Strategy: All Comparisons Against the Active Control Group are Based on the Pool of Both Trials
The modified strategy initially considered focused on the trial population recruited before halting recruitment due to the pandemic. The statistical comparisons against placebo would still be sufficiently powered. However, it would be challenging to have sufficient power for the comparisons against the active control group due to the smaller sample size. This was the reason for proposing to pool the data from both replicate studies for these comparisons. As an additional pre-requisite, it was required that the corresponding endpoint comparisons against placebo demonstrate superiority based on both individual studies, before testing the same endpoint against the active control in the pool.
For testing the hypotheses based on the pooled dataset, the initial Type I error level for the comparison of active drug versus active control for the primary endpoint is set to (one-sided) for each dose (H
, H
). The subtraction of
is to account for the maximum possible Type I error of wrongly claiming any success against placebo (in both studies). We subtract this portion from the significance level
for H
and H
in order to control the Type I error rate at level α for the submission. Under the global null hypothesis, the testing procedure outlined above controls the Type I error rate at the level of the submission of
for the primary endpoint comparison versus placebo. Considering all possible configurations of true and false null hypotheses, the Type I error rate (one-sided) is controlled at the study-level at <0.025 for all comparisons of the experimental drug against placebo, and at the level of the submission of <0.025 for all hypotheses (Bretz, Maurer, and Xi Citation2019).
shows this modified strategy. The Type I error is controlled on the study level for all placebo comparisons, and on the submission level for all comparisons against the active comparator. Again, the null hypotheses are tested in the order indicated by the arrows for Type I error propagation, for each individual study as well as for their pool. For better legibility, the graph does not show the additional requirement that an endpoint can only be tested against the active control on the pool if the corresponding test of the same endpoint on the same dose is significant against placebo in both individual studies.
4.3 Modified Option 2—Modified Pooling Strategy: The Secondary Endpoint Comparisons Versus Active Control are Based on the Pool of Both Trials, the Primary Endpoints are Analyzed in the Individual Trials
Meanwhile, the two trials had restarted recruitment, and their recruitment speed was faster than expected. With 100% recruitment within reach, the proposed hierarchical testing strategy was reconsidered to perform not only all placebo comparisons in the individual studies, but also the primary endpoint comparison against the active control.
displays this testing strategy as proposed at the time of writing the article. Again, the hypotheses can only be tested in the order indicated by the arrows. If the primary endpoint has demonstrated the superiority versus placebo and versus active control, the Type I error can be passed to the tests for all of the secondary endpoints. For the secondary endpoints, if an endpoint demonstrates superiority versus placebo on the same dose in each of the individual studies, the same endpoint can be tested on that dose against the active comparator based on the pooled dataset as pre-specified. This procedure constitutes a middle ground between the original trial protocol and the mitigation strategy initially considered. It controls the Type I error on the trial level for all tests against placebo and for the test of the primary endpoint against the active comparator, and on the submission level for all secondary endpoint tests against the active comparator (Bretz, Maurer, and Xi Citation2019).
5 Power Simulations
Simulations were performed to compare the statistical power for the three options described above, based on the same range of assumptions of the treatment effect for the primary and secondary endpoints as used at the time of study design. Here we display the results using conservative assumptions; less conservative assumptions show essentially the same trends. displays the simulated power values for the original study design and the mitigation strategy first considered, based on 85% of the target sample size. This reflects approximately the population recruited before the temporary recruitment halt, as initially considered. It can be seen that the proposed strategy provides much better statistical power for the comparison against the active control group, retaining sufficient power even in this situation of a reduced sample size.
Fig. 4 The statistical power* for the primary/secondary endpoints based on the pre-COVID recruited population—original individual study strategy versus the first pooling strategy. *For a fair comparison, the statistical power is provided on the submission level throughout. That is, even for the individual study strategy, the given power is the probability to reach statistical significance in both trials (for the respective comparison of the respective endpoint).
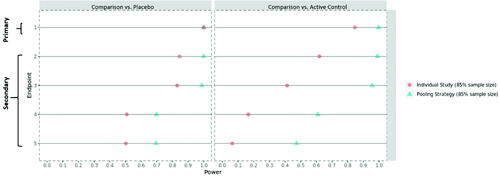
shows the simulated power values for the original study design and the final proposed mitigation strategy, based on 100% of the target sample size. With the ongoing COVID-19 pandemic, it cannot be excluded to see some extra variability in the trial, the exact nature of which may be difficult to discern (e.g., pre- vs. during/post-pandemic heterogeneity in trial population). To reflect this potential impact on the trial data, an extra 5% variability has been added to the simulation setup. It can be seen that the (modified) pooling strategy is well suited to absorb this extra level of variability and still retains sufficient power for all relevant endpoints and comparisons. In addition, this testing strategy will be further supported by additional sensitivity analysis to assess the robustness of any pooled analyses with respect to inter-trial variability. Clearly, the modified pooling strategy provides much higher statistical power for the secondary endpoint comparisons against the active control, which fulfills the purpose of de-risking the COVID-19 impact.
Fig. 5 The statistical power* for the primary/secondary endpoints based on the entire trial population—original individual study strategy versus the modified pooling strategy. *For a fair comparison, the statistical power is provided on the submission level throughout. That is, even for the individual study strategy, the given power is the probability to reach statistical significance in both trials (for the respective comparison of the respective endpoint).
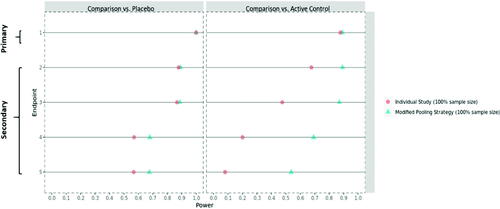
6 Discussion
The COVID-19 pandemic, which is still ongoing at the time of writing this article, has put significant challenges in the way of clinical research. A new viewpoint is added to patient safety, trial logistics have been disrupted, and statistical methodology needs to address unforeseen challenges such as new types of intercurrent events, a potentially curtailed or heterogeneous study population, and more. However, these predicaments also open up new opportunities to rethink traditional study designs. In this article, we describe one such case.
In the situation of two identical Phase III studies, the major idea put forward in this development program in light of the COVID-19 pandemic is to consider inference based on the pool of both studies. Unlike traditional overarching inference strategies, such as meta-analyses or integrated summaries of efficacy or safety, the proposed method formally embeds the pooled inference in the primary testing scheme of the two pivotal studies. Importantly, the most relevant statistical tests are still performed separately per study. Statistical tests based on pooled data are only performed for lower-level endpoints and comparisons (here against the active comparator) for which sufficient power in an individual study cannot be ensured, and only if higher-level tests (here placebo comparisons per study) have been passed successfully. These lower-level tests can additionally be performed per study, outside the confirmatory testing strategy, to check for consistency across studies.
One caveat for the implementation of pooled analyses in a pivotal setting is that they should still provide “substantial evidence of effectiveness” (FDA Citation1998), a term which has often been interpreted as calling for replication in two independent adequate and well-controlled trials. Nuanced discussions of this interpretation have been given previously, for example, by Maca et al. (Citation2002) and Shun et al. (Citation2005). In the present case study, any statistical test based on pooled data from both trials is part of an overarching testing scheme in which most, and the most important, tests (i.e., all tests of the primary endpoint against both comparators and all placebo comparisons of the secondary endpoints) serve as “gatekeepers” and are still performed separately by trial. By design, this provides a strong notion of consistency to the overall evidence package. Independent replication is given by the fact that even the pooled tests are not based on a single large trial, but on the pool of two identical and independently conducted trials, which allows checking for between-trial consistency in additional sensitivity analyses. The fundamental assumption for this case study is that the two trials are similar. In other situations, the strategy will need to be adapted for, for example, different study designs, endpoints, types of COVID-19 impact, etc., to the extent possible.
Overall, we believe this strategy allows the creation of a more robust inference package which retains the originally intended rigor of a pivotal program even in light of major disruptions such as caused by the COVID-19 pandemic. It should be emphasized that the use of the mitigation strategies discussed in this article as primary analyses for pivotal clinical trials requires pre-specifying them before database lock and after a clear and transparent communication with Health Authorities.
usbr_a_1905056_sm6313.pdf
Download PDF (356.1 KB)Acknowledgments
We thank Mouna Akacha, Frank Bretz, Paul Gallo, Charis Papavassilis, Vineeth Varanasi, Dong Xi, and two external expert consultants for helpful discussions. We are equally grateful to two anonymous peer reviewers who helped improve this article.
References
- Akacha, M., Branson, J., Bretz, F., Dharan, B., Gallo, P., Gathmann, I., Hemmings, R., Jones, J., Xi, D., and Zuber, E. (2020), “Challenges in Assessing the Impact of the COVID-19 Pandemic on the Integrity and Interpretability of Clinical Trials,” Statistics in Biopharmaceutical Research, 12, 419–426. DOI: https://doi.org/10.1080/19466315.2020.1788984.
- Bretz F., Maurer W., Brannath W., and Posch M. (2009), “A Graphical Approach to Sequentially Rejective Multiple Test Procedures,” Statistics in Medicine, 28, 586–604. DOI: https://doi.org/10.1002/sim.3495.
- Bretz, F., Maurer W., and Xi, D. (2019), “Replicability, Reproducibility, and Multiplicity in Drug Development,” Chance, 32, 4–11. DOI: https://doi.org/10.1080/09332480.2019.1695432.
- Dequin, P.-F., Le Gouge, A., Tavernier, E., Giraudeau, B., and Zohar, S. (2020), “Embedding a COVID-19 Group Sequential Clinical Trial Within an Ongoing Trial: Lessons From an Unusual Experience,” Statistics in Biopharmaceutical Research, 12. DOI: https://doi.org/10.1080/19466315.2020.1800509.
- European Medicines Agency Committee for Medicinal Products for Human Use. (EMA/CHMP). (2020), “Points to Consider on Implications of Coronavirus Disease. (COVID-19) on Methodological Aspects of Ongoing Clinical Trials,” EMA/158330/2020 Rev. 1. Available at https://www.ema.europa.eu/en/documents/scientific-guideline/points-consider-implications-coronavirus-disease-covid-19-methodological-aspects-ongoing-clinical_en-0.pdf.
- European Medicines Agency Committee for Medicinal Products for Human Use. (EMA/CHMP). (2021), “Guidance on the Management of Clinical Trials During the COVID-19 (Coronavirus) Pandemic.” Available at https://ec.europa.eu/health/sites/health/files/files/eudralex/vol-10/guidanceclinicaltrials_covid19_en.pdf.
- Food and Drug Administration. (FDA) (1998), Guidance for Industry, “Providing Clinical Evidence of Effectiveness for Human Drug and Biological Products.” Available at https://www.fda.gov/media/71655/download.
- Food and Drug Administration. (FDA) (2020), “Statistical Considerations for Clinical Trials During the COVID-19 Public Health Emergency.” Available on https://www.fda.gov/media/139145/download.
- Geerts, H., and van der Graaf, P. H. (2020), “Salvaging CNS Clinical Trials Halted Due to COVID-19,” CPT Pharmacometrics & Systems Pharmacology, 9, 367–370.
- Maca, J., Gallo, P., Branson, M., and Maurer, W. (2002), “Reconsidering Some Aspects of the Two-Trials Paradigm,” Journal of Biopharmaceutical Statistics, 12, 107–119. DOI: https://doi.org/10.1081/bip-120006450.
- Meyer, R. D., Ratitch, B., Wolbers, M., Marchenko, O., Quan, H., Li, D., Fletcher, C., Li, X., Wright, D., Shentu, Y., Englert, S., Shen, W., Dey, J., Liu, T., Zhou, M., Bohidar, N., Zhao, P. L., and Hale, M. (2020), “Statistical Issues and Recommendations for Clinical Trials Conducted During the COVID-19 Pandemic,” Statistics in Biopharmaceutical Research, 12, 399–411. DOI: https://doi.org/10.1080/19466315.2020.1779122.
- Mütze, T., and Friede, T. (2020), “Data Monitoring Committees for Clinical Trials Evaluating Treatments of COVID-19,” Contemporary Clinical Trials, 98, 106154. DOI: https://doi.org/10.1016/j.cct.2020.106154.
- Shun, Z., Chi, E., Durrleman, S., Fisher, L. (2005), “Statistical Consideration of the Strategy for Demonstrating Clinical Evidence of Effectiveness—One Larger vs Two Smaller Pivotal Studies”, Statistics in Medicine, 24, 1619–1637. DOI: https://doi.org/10.1002/sim.2015.