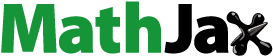
In Statistics in Biopharmaceutical Research, the roles of hypothesis testing and p-values was discussed in a special section featuring the article entitled “The Role of p-Values in Judging the Strength of Evidence and Realistic Replication Expectations,” (Gibson Citation2021). Accompanying discussions were invited and provided by distinguished researchers. As noted in the special section’s editorial (Hamasaki et al. Citation2021), hypothesis testing and p-values continue to have an essential role in assessing evidence from clinical studies and biopharmaceutical research. However, additional measures are to comprehensively interpret and understand study results and to characterize the effect of a medical product quantitatively. Statistics in Biopharmaceutical Research will continue to stimulate further discussions in this important topic.
In 2019, Professor Karen Kafadar, the President of the American Statistical Association (ASA), convened a Task Force on statistical significance and replicability consisting of esteemed scientists in multiple statistical disciplines, to write a succinct statement about the proper use of these methods in scientific studies. The Task Force carefully considered the issues and developed a unified Task Force statement. Multiple journals have published the statement to inform and educate the broad community of scientific inquiries. Therefore, it is reprinted here, in Statistics in Biopharmaceutical Research, with permission from the Institute of Mathematical Statistics.
ASA President’s Task Force Statement on Statistical Significance and Replicability
Authors:
Yoav Benjamini
Richard D. De Veaux
Bradley Efron
Scott Evans
Mark Glickman
Barry I. Graubard
Xuming He (co-chair)
Xiao-Li Meng
Nancy M. Reid, OC, FRS, FRSC
Stephen M. Stigler
Stephen B. Vardeman
Christopher K. Wikle
Tommy Wright
Linda J. Young (co-chair)
Karen Kafadar (ex-officio)
ASA President’s Task Force Statement on Statistical Significance and Replicability
Over the past decade, the sciences have experienced elevated concerns about replicability of study results. An important aspect of replicability is the use of statistical methods for framing conclusions. In 2019 the President of the American Statistical Association (ASA) established a task force to address concerns that a 2019 editorial in The American Statistician (an ASA journal) might be mistakenly interpreted as official ASA policy. (The 2019 editorial recommended eliminating the use of “” and “statistically significant” in statistical analysis.) This document is the statement of the task force, and the ASA invited us to publicize it. Its purpose is two-fold: to clarify that the use of P-values and significance testing, properly applied and interpreted, are important tools that should not be abandoned, and to briefly set out some principles of sound statistical inference that may be useful to the scientific community.
P-values are valid statistical measures that provide convenient conventions for communicating the uncertainty inherent in quantitative results. Indeed, P-values and significance tests are among the most studied and best understood statistical procedures in the statistics literature. They are important tools that have advanced science through their proper application.
Much of the controversy surrounding statistical significance can be dispelled through a better appreciation of uncertainty, variability, multiplicity, and replicability. The following general principles underlie the appropriate use of P-values and the reporting of statistical significance and apply more broadly to good statistical practice.
Capturing the uncertainty associated with statistical summaries is critical. Different measures of uncertainty can complement one another; no single measure serves all purposes. The sources of variation that the summaries address should be described in scientific articles and reports. Where possible, those sources of variation that have not been addressed should also be identified.
Dealing with replicability and uncertainty lies at the heart of statistical science. Study results are replicable if they can be verified in further studies with new data. Setting aside the possibility of fraud, important sources of replicability problems include poor study design and conduct, insufficient data, lack of attention to model choice without a full appreciation of the implications of that choice, inadequate description of the analytical and computational procedures, and selection of results to report. Selective reporting, even the highlighting of a few persuasive results among those reported, may lead to a distorted view of the evidence. In some settings, this problem may be mitigated by adjusting for multiplicity. Controlling and accounting for uncertainty begins with the design of the study and measurement process and continues through each phase of the analysis to the reporting of results. Even in well-designed, carefully executed studies, inherent uncertainty remains, and the statistical analysis should account properly for this uncertainty.
The theoretical basis of statistical science offers several general strategies for dealing with uncertainty. P-values, confidence intervals and prediction intervals are typically associated with the frequentist approach. Bayes factors, posterior probability distributions and credible intervals are commonly used in the Bayesian approach. These are some among many statistical methods useful for reflecting uncertainty.
Thresholds are helpful when actions are required. Comparing P-values to a significance level can be useful, though P-values themselves provide valuable information. P-values and statistical significance should be understood as assessments of observations or effects relative to sampling variation, and not necessarily as measures of practical significance. If thresholds are deemed necessary as a part of decision making, they should be explicitly defined based on study goals, considering the consequences of incorrect decisions. Conventions vary by discipline and purpose of analyses.
In summary, P-values and significance tests, when properly applied and interpreted, increase the rigor of the conclusions drawn from data. Analyzing data and summarizing results are often more complex than is sometimes popularly conveyed. Although all scientific methods have limitations, the proper application of statistical methods is essential for interpreting the results of data analyses and enhancing the replicability of scientific results.
The most reckless and treacherous of all theorists is he who professes to let facts and figures speak for themselves, who keeps in the background the part he has played, perhaps unconsciously, in selecting and grouping them. (Alfred Marshall, 1885)
Authors:
Yoav Benjamini, Professor Emeritus of Applied Statistics, Department of Statistics and Operations Research, and Member, Sagol School of Neuroscience and the Edmond Safra Bioinformatics Center, Tel Aviv University
Richard De Veaux, C. Carlisle and Margaret Tippit Professor and Chair, Department of Mathematics and Statistics, Williams College
Bradley Efron, Max H. Stein Professor of Humanities and Sciences, Professor of Statistics and Biostatistics, Department of Statistics and Department of Biostatistics, Stanford University
Scott Evans, Professor and Chair, Department of Biostatistics & Bioinformatics and Director of Biostatistics Center, George Washington University
Mark Glickman, Senior Lecturer, Department of Statistics, Harvard University; and Senior Statistician, Center for Healthcare Organization and Implementation Research, a Veterans Administration Center of innovation
Barry I. Graubard, Senior Investigator, Biostatistics Branch, National Cancer Institute
Xuming He (co-chair), H.C. Carver Collegiate Professor of Statistics, University of Michigan
Xiao-Li Meng, Whipple V.N. Jones Professor, Department of Statistics, Harvard University
Nancy Reid, OC, FRS, FRSC, University Professor of Statistics, University of Toronto
Stephen M. Stigler, Ernest DeWitt Burton Distinguished Service Professor, Department of Statistics, University of Chicago
Stephen B. Vardeman, University Professor, Department of Statistics and Department of Industrial & Manufacturing Systems Engineering, Iowa State University
Christopher K. Wikle, Curators Distinguished Professor and Chair, Department of Statistics, University of Missouri
Tommy Wright, Research Mathematical Statistician and Chief, Center for Statistical Research and Methodology, U.S. Bureau of the Census
Linda J. Young (co-chair), Chief Statistician and Director of Research & Development, National Agricultural Statistics Service
Karen Kafadar (ex-officio), Commonwealth Professor and Chair, Department of Statistics, University of Virginia
Additional information
Funding
References
- Gibson, E. W. (2021), “The Role of p-Values in Judging the Strength of Evidence and Realistic Replication Expectations,” Statistics in Biopharmaceutical Research, 13, 6–18. DOI: https://doi.org/10.1080/19466315.2020.1724560.
- Hamasaki, T., Bretz, F., LaVange, L. M., Müller, P., Pennello, G., & Pinheiro, J. C. (2021), “Roles of Hypothesis Testing, p-Values and Decision Making in Biopharmaceutical Research,” Statistics in Biopharmaceutical Research, 13, 1–5.