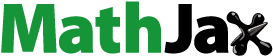
We thank and commend Dr. Levenson and colleagues for their insightful overview and are grateful for the opportunity to discuss their excellent article (Levenson et al. Citation2021). We would also like to congratulate its companion articles led by Dr. Chen and Dr. Ho (Chen et al. Citation2021; Ho et al. Citation2021). These articles provide clear and detailed explanations of the biostatistical considerations when using real world data (RWD) in regulatory submissions. Although randomized controlled trials (RCTs) are the gold standard for evaluating the efficacy and safety of drugs, conducting traditional large-scaled phase 3 RCTs is often not feasible especially in rare disease settings mainly due difficulty in recruitment. Therefore, there is a move toward investigating the efficient use of RWD for drug development in innovative ways, which is expected to play an important role in supporting regulatory decision making. In using RWD, it is critical to understand biostatistical considerations from a scientific point of view as well as regulatory, data reliability and ethical points. In Levenson et al.’s (Citation2021) and the companion articles (Chen et al. Citation2021; Ho et al. Citation2021), the authors introduced the study designs and analysis methods of clinical trials using RWD, summarized the typical biases to be considered when using these designs, and presented how to set the estimand(s) in an easy-to-understand manner. In addition, Ho et al. (Citation2021) provided a detailed explanation of the Causal Roadmap as a statistical inference framework for obtaining real-world evidence (RWE).
In Japan, as in other countries, the use of RWE in regulatory decision making has been attracting attention, and the Ministry of Health, Labour and Welfare (MHLW), a Japanese regulatory authority, has released two notifications and guidelines drafted by Pharmaceuticals and Medical Devices Agency (PMDA) (MHLW Citation2021a, Citation2021b). Also, to facilitate efficient drug development using disease and patient registries, which is one of the data sources of RWD, the Clinical Innovation Network (CIN) has been launched by MHLW in 2015. Using the registry mainly established by National Centers for Advanced and Specialized Medical Care (NCs), CIN is a network mainly composed of such organizations as the NCs, core clinical research hospitals, PMDA, Japan Agency for Medical Research and Development, forming a clinical trial consortium. As a part of its recommendations, this initiative has called for promoting new clinical trial/clinical research by using disease/patient registries (Takeda et al. Citation2017). Herein, as members of one of the working groups within CIN, we have been discussing the reliability of the data and the biostatistical considerations on using data from the registries for clinical trials or research, and published the recommendations (Shibata and Research team for AMED, 2019; Research team for AMED, 2019). In particular, as the working group addressing biostatistics aspects, we put special emphasis on (i) the transparency of conducting clinical trials using RWD and (ii) possible biases relative to conventional clinical trial settings. Though not mentioned in this comment, the working group also discusses the possible linkage of multiple data sources. As members of the working group, we would like to discuss some points to concern from our current report, focusing on the differences with the content of the article by Levenson et al. (Citation2021).
In a clinical trial using RWD, ensuring transparency is crucial for evaluating the quality of the trial. For traditional clinical trials, transparency of research practices has been improved and well-established; protocols and statistical analysis plans are time-stamped and documented before study start or unblinded as relevant (Levenson et al. Citation2021). On the other hand, clinical research using RWD is potentially more complicated in terms of prespecifying the study design before analyzing any data, and thus the lack of up-front transparency may result in data dredging and cherry picking of the results (Wang et al. 2017). Of course, transparency is not the only factor which ensures the high quality and validity of the research. However, transparency enables to examine the quality and validity of the research presented and allows for a better understanding of why and how the research was conducted, and whether the results reflect preplanned questions and methods. For example, a joint task force of the International Society for Pharmacoeconomics and Outcomes Research (ISPOR) and the International Society for Pharmacoepidemiology (ISPE) has reported on good practice to maintain transparency in conducting clinical research using RWD (Berger et al., Citation2017; ISPOR 2019; Wang et al. 2017). As a specific approach to use RWD for an external control, Levenson et al. (Citation2021) and its companion articles (Chen et al. Citation2021; Ho et al. Citation2021) also introduced the “two-step” approach proposed by Yue et al. (Citation2014), which implements propensity score analysis to adjust for covariate-difference between external control from RWD and a study sample. In a “design stage”, analysts who are not allowed to access outcome data select candidate covariates and choose propensity score models possibly referring to model-fit measures. In the following “analysis stage”, (possibly different) analysts compare outcomes between the groups. Separating the design and analysis stages as above commits to a predefined specification of analysis and reproducible results. As pointed out by Ho et al. (Citation2021), however, a propensity score-based method needs to select covariates to be adjusted for based on the outcome–covariate relationship as well as the treatment–covariate relationship. Given adjusted covariates need to be determined in outcome-blinding situations, innovative methods are expected to be applied in regulatory situations, and further development will be desired to protect data dredging. As another example in randomized trial settings, Tsiatis et al. (2018) recommended to differentiate an “outcome-modeling” stage and a “comparison” stage. In the outcome-modeling stage, two different analyst teams separately develop the outcome models by treatment groups. Then, treatments are compared using predicted counterfactual outcomes through a semiparametric efficient estimator, also known as doubly robust or targeted maximum likelihood estimators (van der Laan and Rose, Citation2011). The approach can be modified to nonrandomized settings including a RWD control group. Moreover, all these confounding adjustment methods, as well as static or dynamic data integrating methods are not guaranteed to strictly control the Type I error rate, which is the absolute and established requirement in a regulatory decision making. This may require further refinement in their applications to actual clinical datasets.
Dealing with epidemiologic biases is also essential when RWD is used as an external control because various types of biases are anticipated: the time-related bias (protopathic, latency time, immortal time, time-window, depletion of susceptibles, and immeasurable time biases) (Suissa and Dell’Aniello Citation2020), the bias resulting from the difference of the measurement intervals between RWD and a trial setting, and the bias due to the lack of randomization, etc. In our working group, we have focused on the biases inherent in the use of RWD as an external control in single arm trials and hybrid control approaches in RCTs; we summarized those biases in terms of information bias, selection bias, and confounding bias. Levenson etal. (2021) and Chen et al. (Citation2021) also provided a detailed description of those biases and corresponding analysis methods, including their pros and cons.
To organize and identify an appropriate design and analysis in the use of RWD, the “estimand” framework may be useful to clarify the study objective. The five “attributes” of estimands for clinical trials as outlined in ICH E9 (R1), that is, treatment, population, variable or endpoint of interest, the methods for accounting for intercurrent events, and population-level measure for comparison of treatments or interventions, would be common issues regardless of the study type and source of the data. Levenson et al. (Citation2021) detailed the points to consider for each element regarding the use of RWD. In addition, we would like to note that when using RWD to make inferences about efficacy and safety, emulating a hypothetical RCT (i.e., a target trial) which answers the clinical hypothesis of interest allows us to clarify design and analysis considerations in advance and avoid pitfalls that may lead to the abovementioned biases. In particular, the following process would identify and address the potential biases: (i) set the estimand by specifying the hypothetical target trial protocol (eligibility criteria, timing of inclusion and randomization, comparable treatment regimens, follow-up and outcome assessment, etc.); (ii) use a trial emulation framework and specify the variables that can mimic the “exchangeability” in a target trial (i.e., baseline/post-randomization covariates); and (iii) specify analysis methods (inverse probability weighting estimation, parametric g-formula, or doubly robust estimation such as targeted maximum likelihood estimation).
In relation to the above, we would like to emphasize Ho et al.’s (Citation2021) “six steps” of the Causal Roadmap (van der Laan and Rose, Citation2011; Petersen and van der Laan, Citation2014; Petersen, Citation2014) as a useful framework for considering the design and analysis of clinical trials using RWE in regulatory settings. The Causal Roadmap would be useful in these situations because it allows us to set up two-stage inference targets: for example, a causal estimand ] defined by a counterfactual distributions of a potential outcome Ya
that would be observed as Y under treatment A = a, and a estimand
defined by a functional of the distribution of the observed data, which would be identical under a potential outcome framework if covariates W are sufficient to control for confounding. For those who accept the potential outcome framework and counterfactual definitions of treatment effects, the difference between these two quantities would be trivial. However, we think that this two-stage framework is an especially useful platform in the context of clinical trials as a type of scientific technology assessment: in assessment and regulation of newly developed drugs, we should make consensus on data to collect and the selection of estimators on the basis of the “real-world” terminology, rather than “causal (counterfactual) world” one, even if these are connected to each other under appropriate assumptions (which may or may not be verified by study designs and procedures). Separating a causal estimand, which is always subject to philosophical controversy due to its ontological and epistemological subtlety, from the estimand defined in observed distribution would lead to more chances to reach consensus on practical issues from prespecified design and analysis plan to interpretation and evaluation of the study results among the parties involved in clinical trials.
In summary, Levenson (Citation2021) and its companion articles (Chen et al. Citation2021; Ho et al. Citation2021) provide a detailed summary of the biostatistical considerations in design and analysis of clinical trials when using RWD/RWE. These articles are particularly useful for biostatisticians who will use RWD/RWE in drug development. In this comment, we introduced the activities in Japan, focusing on the similarity and differences with Levenson et al. (Citation2021), and reiterated the points that we considered important. As the application of RWD/RWE in medicine and other fields is increasing in various countries around the world, we hope to share and harmonize the issues and examples from biostatistical viewpoints.
Acknowledgments
The authors thank to the research members of AMED Research on Regulatory Science of Pharmaceuticals and Medical Devices.
Additional information
Funding
References
- Berger, M. L., Sox, H., Willke, R. J., Brixner, D.L., Eichler, H.G., Goettsch, W., Madigan, D., Makady, A., Schneeweiss, S., Tarricone, R., Wang, S.V., Watkins, J., and Mullins, D. C. (2017), “Good Practices for Real-World Data Studies of Treatment and/or Comparative Effectiveness: Recommendations From the joint ISPOR-ISPE Special Task Force on Real-World Evidence in Health Care Decision Making,” Pharmacoepidemiology and Drug Safety, 26, 1033–1039. DOI: 10.1002/pds.4297.
- Chen, J., Ho, M., Lee, K., Song, Y., Fang, Y., Goldstein, B. A., He, W., Irony, T., Jiang, Q., van der Laan, M., Lee, H., Lin, X., Meng, Z., Mishra-Kalyani, P., Rockhold, F., Wang H., and White, R. (2021), “The Current Landscape in Biostatistics of Real-World Data and Evidence: Clinical Study Design and Analysis,” Statistics in Biopharmaceutical Research, DOI: 10.1080/19466315.2021.1883474.
- Hernán, M. A., and Robins, J. M. (2017), “Per-Protocol Analyses of Pragmatic Trials,” New England of Journal of Medicine, 377, 1391–1398. DOI: 10.1056/NEJMsm1605385.
- Hernán, M. A., and Robins, J. M. (2016), “Using Big Data to Emulate a Target Trial When a Randomized Trial is Not Available,” American Journal of Epidemiology, 183, 758–764.
- Ho, M., van der Laan, M., Lee, H., Chen, J., Lee, K., Fang, Y., He, W., Irony, T., Jiang, Q., Lin, X., Meng, Z., Mishra-Kalyani, P., Rockhold, F., Song, Y., Wang H., and White, R. (2021), “The Current Landscape in Biostatistics of Real-World Data and Evidence: Causal Inference Frameworks for Study Design and Analysis,” Statistics in Biopharmaceutical Research, DOI: 10.1080/19466315.2021.1883475.
- ICH E9 (R1). (2019), “ICH E9 (R1): Addendum to Statistical Principles for Clinical Trials on Choosing Appropriate Estimands and Defining Sensitivity Analyses in Clinical Trials.”
- Levenson, M., He,W., Chen, J., Fang, Y., Faries D., Goldstein, B. A., Ho, M., Lee, K., Mishra-Kalyani, P., Rockhold, F., Wang, H., and Zink, R. C. (2021), “Biostatistical Considerations When Using RWD and RWE in Clinical Studies for Regulatory Purposes: A Landscape Assessment, Statistics in Biopharmaceutical Research,” Statistics in Biopharmaceutical Research, DOI: 10.1080/19466315.2021.1883473.
- Ministry of Health, Labour and Welfare (MHLW) (2021a), “Basic principles on Utilization of Registry for Applications, PSEHB/PED Notification No.0323-1 and PSEHB/MDED Notification No.0323-1,” March 23, 2021, available at https://www.pmda.go.jp/files/000240806.pdf.
- Ministry of Health, Labour and Welfare (MHLW) (2021b), Points to Consider for Ensuring the Reliability in Utilization of Registry Data for Applications PSEHB/PED Notification No.0323-2 and PSEHB/MDED Notification No.0323-2,” March 23, 2021, available at https://www.pmda.go.jp/files/000240807.pdf.
- Petersen M. L. (2014), “Commentary: Applying a Causal Road Map in Settings With Time-Dependent Confounding.” Epidemiology (Cambridge, Mass.), 25, 898–901. DOI: 10.1097/EDE.0000000000000178.
- Petersen, M. L., and van der Laan, M. J. (2014), “Causal Models and Learning from Data: Integrating Causal Modeling and Statistical Estimation,” Epidemiology (Cambridge, Mass.), 25, 418. DOI: 10.1097/EDE.0000000000000078.
- Research Team for AMED Research on the Regulatory Science of Pharmaceuticals and Medical Devices: Utilization of Real World Evidence Using Patient Registry Data to Support Regulatory Decision-Making. (2019), “Proposal on Assuring the Reliability of Patient Registry Data for Use in Application Dossiers of Pharmaceuticals and Medical Devices.” Japan Society of Clinical Trials and Research, 47(s1), s23–35.
- Shibata, T., and Research Team for AMED Research on the Regulatory Science of Pharmaceuticals and Medical Devices: Utilization of Real World Evidence Using Patient Registry Data to Support Regulatory Decision-Making. (2019), “A Background of Proposal on Ensuring the Reliability of Patient Registry Data for Use in Application Dossiers of Pharmaceuticals and Medical Devices,” Japan Society of Clinical Trials and Research, 47, S9–S12.
- Suissa, S., and Dell’Aniello, S. (2020), “Time-Related Biases in Pharmacoepidemiology,” Pharmacoepidemiology and Drug Safety, 29, 1101–1110. DOI: 10.1002/pds.5083.
- Takeda, S., Shishido T., Yamamoto H., Haraguchi R., Sakurai T., Suzuki K., Hayashi K., Wada, K., Tachimori, H., Shibata, T., Ohmatsu, H., Tashiro, S., Nosaka, K., Sugiyama, Y., Yahagi, N., and Matsuyama A. (2017), “Research on the Strategy for Promoting Clinical Trials/Researches by Utilizing Disease Registration System (Patient Registry) Established by National Centers and Other Institutions,” Japan Journal of Medical Informatics, 37, 28–29.
- The Real-World Evidence Transparency Initiative Partnership, International Society for Pharmacoepidemiology (ISPOR), Duke-Margolis Center for Health Policy, and the National Pharmaceutical Council. (2019), “Draft White Paper: Improving Transparency In Non-Interventional Research for Hypothesis Testing—WHY, WHAT, and HOW: Considerations From the Real-World Evidence Transparency Initiative,” available at improving-transparency-in-non-interventional-research-for-hypothesis-testing_final.pdf (ispor.org).
- Tsiatis, A. A., Davidian, M., Zhang, M., and Lu, X. (2008), “Covariate Adjustment for Two-Sample Treatment Comparisons in Randomized Clinical Trials: A Principled Yet Flexible Approach.” Statistics in Medicine, 27, 4658–4677. DOI: 10.1002/sim.3113.
- van der Laan, M. J., and Rose, S. (2011), Targeted Learning: Causal Inference for Observational and Experimental Data, New York: Springer Science & Business Media.
- Wang, S. V., Schneeweiss, S., Berger, M. L., Brown, J., de Vries, F., Douglas, I., Gagne, J. J., Gini, R., Klungel, O., Mullins, C. D., Nguyen, M. D., Rassen, J. A., Smeeth, L., Sturkenboom, M.; joint ISPE-ISPOR Special Task Force on Real World Evidence in Health Care Decision Making. (2017), “Reporting to Improve Reproducibility and Facilitate Validity Assessment for Healthcare Database Studies V1.0,” Value Health, 20, 1009–1022.
- Yue, L. Q., Lu, N., and Xu, Y. (2014), “Designing Premarket Observational Comparative Studies Using Existing Data as Controls: Challenges and Opportunities,” Journal of Biopharmaceutical Statistics, 24, 994–1010. DOI: 10.1080/10543406.2014.926367.