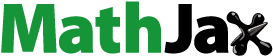
Prospectively designed studies that make use of real-world data (RWD) are increasingly being used for studies with regulatory implications, including both premarket and post-market studies, aided by the Food and Drug Administration’s Citation2017 guidance on using real-world evidence to support regulatory decisions for medical devices. The design that Lu, Xu, and Yue (Citation2020) considered in their article is a nonrandomized comparative study, where the treated group consists of volunteers prospectively enrolled into a single-arm clinical study of a medical device and the entire control group is made up of patients from an RWD source (e.g., a registry). The authors propose using propensity scores to balance baseline covariates between the treated and control groups to reduce confounding. To maintain objectivity, the authors suggest that investigators adhere to an outcome-free principle in the design phase. That is, the process of balancing the groups being compared, or more generally selecting a design (i.e., propensity score balancing approach), is completely independent of the outcome data. This represents the first phase of a two-phase process in executing a nonrandomized comparative study, with the second phase of outcome analysis only being undertaken after the first phase (i.e., balancing groups on covariates) has been finalized.
We wholeheartedly agree with the merits of separating the design from the outcome analysis in maintaining objectivity during study execution. However, the process of selecting a design as described by Lu, Xu, and Yue (Citation2020) and others (Yue, Lu, and Xu Citation2014; Li et al. Citation2016; Yue et al. Citation2016; Li et al. Citation2020), may be less than optimal with respect to minimizing measured confounding, the primary design consideration in a nonrandomized comparative study. In this commentary, we describe how to improve the design by reducing confounding by measured covariates in the context of the two-phase framework proposed by Lu, Xu, and Yue (Citation2020).
In order to reduce confounding by measured covariates, we argue that, in the first phase of the process, a large number of candidate designs should be considered, and selection should be based on the design that minimizes imbalance among the design choices evaluated (Harder, Stuart, and Anthony Citation2010). Candidate designs can be described along two dimensions, methods for propensity score estimation and propensity score application (Harder, Stuart, and Anthony Citation2010). Propensity score estimation refers to how the propensity score (i.e., the probability of receiving treatment) is calculated. Typically, propensity score estimation uses a logistic regression model with only main effects, but more flexible logistic models are possible with the inclusion of interaction and/or higher-order terms, or alternatively, nonparametric approaches (e.g., boosting, random forest) (Lee, Lessler, and Stuart Citation2010). Propensity score application refers to how the propensity scores are used. Commonly used methods are matching, weighting and stratification. One approach would cross-classify several propensity score estimation methods with several propensity score application methods to generate a comprehensive set of candidate designs (Harder, Stuart, and Anthony Citation2010). Notably, some propensity score applications may be more robust to propensity score model misspecification (i.e., nonlinear/nonadditive covariate effects on treatment assignment), such as trimming after weighting (Lee, Lessler, and Stuart Citation2011), weighting after propensity score stratification (Hong Citation2010; Desai and Franklin Citation2019) and stabilized balance weighting (Zubizarreta Citation2015; Chattopadhyay, Hase, and Zubizarreta Citation2020). A choice of one design is then made based on minimizing imbalance. The proposed approach is compatible with the outcome-free principle described by Lu, Xu, and Yue (Citation2020), in that the outcome is not used in any way in selection of a propensity score balancing approach. Notably, Lu, Xu, and Yue (Citation2020) propose considering more than one design, but only if a simple logistic regression propensity score model combined with propensity score stratification leads to inadequate balance. This is a small subset of possible options, at most only considering a total of two propensity score estimation methods (a model with main effects only, and a model with additional interaction and/or higher-order terms) and one propensity score application method (stratification). Therefore, there is limited opportunity to optimize the design of the study.
The approach described above relies on selection of a design based on reducing imbalance. There is a consensus that, for an individual covariate, an absolute standardized difference less than 0.10 (Austin Citation2009) is considered good or adequately balanced, however some authors consider values below 0.25 acceptable (Harder, Stuart, and Anthony Citation2010). Regardless of the threshold, it follows that if one covariate exceeds the threshold then the balance would be considered inadequate. This leads to one criterion for selecting an optimal design, to minimize the number of variables exceeding a prespecified threshold of imbalance. Another criterion that might be used instead, or in conjunction, is to minimize the average absolute standardized difference across all measured variables.
Factors other than imbalance may play a role in which methods are included as candidates in the selection process. One consideration is generalizability. For example, if a method leads to exclusion of treated patients, which can happen when a caliper is used in matching algorithms, generalizability can be an issue. The problem is that when treated patients are excluded, it may change the population to which inferences are being made, because unmatched treated patients may differ systematically from those who were matched. This can make labeling of the target population for use of a medical device more complex, and therefore is not recommended in regulatory applications (Lu, Xu, and Yue Citation2020). A second consideration is transparency of a balancing method. Matching is arguably the method that is most transparent because comparison patients are selected into the sample based on their similarity to treated patients on measured covariates. In contrast, weighting has been argued to be less transparent when each patient in the treatment group receives a different weight (e.g., inverse probability weighting) and the weighting applied to each patient is not apparent in the estimated treatment effect. However, weighting methods can be limited to those that have acceptable transparency and generalizability, for example, using unweighted data (i.e., weights of 1) for all patients in the treatment group. Specifically, using this method, all patients receiving the target treatment receive a weight of 1 and the nc patients receiving the comparator treatment are weighted up or down to resemble the target treatment group using weights based on the odds of receiving the target treatment, , where
estimated propensity score for patient
(where nc denotes the sample size in the comparator arm). When weights are constructed in this way, the estimand is the average treatment effect among the treated (ATT). A third set of considerations, in some circumstances, is related to outcome modeling but in the absence of viewing the actual outcome data. For example, with small sample sizes and rare outcomes, methods such as stratification can be challenging to apply because for many commonly used effect estimation methods, including the one used by Lu, Xu, and Yue (Citation2020), at least one event must be present for both treatment and comparison groups within each stratum to calculate a stratum-specific treatment effect, and in turn a treatment effect averaged across strata. Therefore, stratification may not be considered a good candidate if the anticipated event rate is low and/or the sample size is small. A final set of considerations are related to accuracy of estimating the precision of the treatment effect and power. While propensity-score weighted estimators of average treatment effects are known to be unbiased for many commonly used ratio and difference measures of effect (Austin Citation2010, Citation2013; Austin and Schuster Citation2016; Lunceford and Davidian Citation2004), concerns may remain regarding accurate methods to calculate the variance of those effects. Robust variance estimation and non-parametric bootstrapping are two common approaches, with the latter typically providing more accurate estimates than the former. Robust variance estimation is conservative because it typically over-estimates the variance, due to not accounting for uncertainty in the weights (e.g., when estimating the ATT hazard ratio (Austin Citation2016)). When comparing balancing methods, pair matching can lead to less precision of the treatment effect because the number of observations from the comparison group are limited to the number of observations in the treatment group, while with weighting, especially using the odds of treatment assignment without trimming, large weights can have the same effect. Therefore, upon identifying some candidate designs that achieve similar balance, a power analysis based on simulating the outcome data may reveal that one method is preferred over another.
A final consideration when identifying candidate designs is the type of inference desired. Lu, Xu, and Yue (Citation2020) rightfully highlighted the importance of this topic in referencing the International Council for Harmonisation E9(R1) guidelines, which have also been considered in the context of retrospective comparative observational studies (Stuart Citation2010; Austin Citation2011; Desai and Franklin Citation2019). Collectively, we would argue that only candidate propensity score methods that target the same estimand, such as the ATT, be considered. The ATT estimates the effect of treatment on the outcome if treatment were removed (i.e., if patients belonged to the comparison group) among those patients that ultimately received treatment. If the data do not support any design for a particular estimand, then designs for alternative estimands may be considered (Stuart Citation2010; Austin Citation2011; Desai and Franklin Citation2019). For example, if it is not feasible to estimate the ATT then the first stage might be revised to consider only propensity score estimation methods that target the Average Treatment Effect (ATE). Additionally, sensitivity analyses may lead to analyses that target estimands that are different from those proposed in the primary/secondary analyses, but such analyses would be strictly exploratory.
In this commentary, we suggest that in a nonrandomized comparative study, the approach to addressing confounding using the propensity score should be to select one method, among many candidates, that minimizes imbalances across measured covariates. Consistent with past recommendations, we advocate that the search for an optimal design take place by considering several methods of propensity score estimation and application, blinded to any outcome data in the first phase of a study. The approach fits within a two-phase framework, while also adhering to the outcome-free principle, as proposed by Lu, Xu, and Yue (Citation2020). While reducing imbalance should be the main objective, other considerations may be important in determining candidate designs and the selection criteria, including: generalizability, transparency, ability to calculate treatment effects with small sample sizes/infrequent outcomes, accuracy of estimating the precision of the treatment effect, statistical power and the desired estimand. For RWD to be useful for regulatory purposes, they must not only be reliable and relevant but also used in a design that ideally can provide an unbiased answer to the proposed research question. Future research incorporating the recommendations proposed in this commentary will lead to improved designs of nonrandomized comparative studies, thereby maximizing the contributions of RWD.
Declaration of Interest
At the time of article preparation all authors were employees of Johnson & Johnson.
Funding
The author(s) reported there is no funding associated with the work featured in this article.
References
- Austin, P. C. (2009), “Balance Diagnostics for Comparing the Distribution of Baseline Covariates Between Treatment Groups in Propensity-Score Matched Samples,” Statistics in Medicine, 28, 3083–3107. DOI: https://doi.org/10.1002/sim.3697.
- Austin, P. C. (2010), “The Performance of Different Propensity-Score Methods for Estimating Differences in Proportions (Risk Differences or Absolute Risk Reductions) in Observational Studies,” Statistics in Medicine, 29, 2137–2148.
- Austin, P. C. (2013), “The Performance of Different Propensity Score Methods for Estimating Marginal Hazard Ratios,” Statistics in Medicine, 32, 2837–2849.
- Austin, P. C. (2011), “An Introduction to Propensity Score Methods for Reducing the Effects of Confounding in Observational Studies,” Multivariate Behavioral Research, 46, 399–424.
- Austin, P. C. (2016), “Variance Estimation When Using Inverse Probability of Treatment Weighting (IPTW) with Survival Analysis,” Statistics in Medicine, 35, 5642–5655.
- Austin, P. C., and Schuster, T. (2016), “The Performance of Different Propensity Score Methods for Estimating Absolute Effects of Treatments on Survival Outcomes: A Simulation Study,” Statistical Methods in Medical Research, 25, 2214–2237. DOI: https://doi.org/10.1177/0962280213519716.
- Chattopadhyay, A., Hase, C.H., and Zubizarreta, J.R. (2020), “Balancing vs Modeling Approaches to Weighting in Practice,” Statistics in Medicine, 39, 3227–3254. DOI: https://doi.org/10.1002/sim.8659.
- Desai, R. J., and Franklin, J. M. (2019), “Alternative Approaches for Confounding Adjustment in Observational Studies Using Weighting Based on the Propensity Score: A Primer for Practitioners,” British Medical Journal, 23, l5657. DOI: https://doi.org/10.1136/bmj.l5657.
- Food and Drug Administration (2017), Use of Real-World Evidence to Support Regulatory Decision-Making for Medical Devices, Guidance for Industry and Food and Drug Administration Staff.
- Harder, V. S., Stuart, E. A., and Anthony, J. C. (2010), “Propensity Score Techniques and the Assessment of Measured Covariate Balance to Test Causal Associations in Psychological Research,” Psychological Methods, 2010, 15, 234–249. DOI: https://doi.org/10.1037/a0019623.
- Hong, G. (2010), “Marginal Mean Weighting Through Stratification: Adjustment for Selection Bias in Multilevel Data,” Journal of Educational and Behavioral Statistics, 35, 499–531. DOI: https://doi.org/10.3102/1076998609359785.
- ICH Expert Working Group (2019), “Addendum on Estimands and Sensitivity Analysis in Clinical Trials to the Guideline on Statistical Principles for Clinical Trials.”
- Lee, B. K., Lessler, J., and Stuart, E. A. (2010), “Improving Propensity Score Weighting Using Machine Learning,” Statistics in Medicine, 29, 337–346. DOI: https://doi.org/10.1002/sim.3782.
- Lee, B. K., Lessler, J., and Stuart, E. A. (2011), “Weight Trimming and Propensity Score Weighting,” PLoS One, 6, e18174.
- Li, H., Mukhi, V., Lu, N., Xu, Y., and Yue, L. (2016), “A Note on Good Practice of Objective Propensity Score Design for Premarket Nonrandomized Medical Device Studies with an Example,” Statistics in Biopharmaceutical Research, 8, 282–286. DOI: https://doi.org/10.1080/19466315.2016.1148071.
- Li, H., Chen, W-C., Lu, N., Wang, C., Tiwari, R., Xu, Y., and Yue, L.Q. (2020), “Novel Statistical Approaches and Applications in Leveraging Real-World Data in Regulatory Clinical Studies,” Health Services and Outcomes Research Methodology, 20, 237–246, DOI: https://doi.org/10.1007/s10742-020-00218-4.
- Lu, N., Xu, Y., and Yue, L.Q. (2020), “Some Considerations on Design and Analysis Plan on a Nonrandomized Comparative Study Utilizing Propensity Score Methodology for Medical Device Premarket Evaluation,” Statistics in Biopharmaceutical Research, 12, 155–163. DOI: https://doi.org/10.1080/19466315.2019.1647873.
- Lunceford, J. K., and Davidian, M. (2004), “Stratification and Weighting Via the Propensity Score in Estimation of Causal Treatment Effects: A Comparative Study,” Statistics in Medicine, 23, 2937–2960. DOI: https://doi.org/10.1002/sim.1903.
- Stuart, E.A. (2010), “Matching Methods for Causal Inference: A Review and a Look Forward,” Statistical Science, 25, 1–21. DOI: https://doi.org/10.1214/09-STS313.
- Yue, L., Lu, N., and Xu, Y. (2014), “Designing Pre-market Observational Comparative Studies Using Existing Data as Controls: Challenges and Opportunities,” Journal of Biopharmaceutical Statistics, 24, 994–1010. DOI: https://doi.org/10.1080/10543406.2014.926367.
- Yue, L. Q., Campbell, G., Lu, N., Xu, Y., and Zuckerman, B. (2016), “Utilizing National and International Registries to Enhance Pre-Market Medical Device Regulatory Evaluation,” Journal of Biopharmaceutical Statistics, 26, 1136–1145. DOI: https://doi.org/10.1080/10543406.2016.1226336.
- Zubizarreta, J. R. (2015), “Stable Weights that Balance Covariates for Estimation with Incomplete Outcome Data,” Journal of the American Statistical Association, 110, 910–922. DOI: https://doi.org/10.1080/01621459.2015.1023805.