Abstract
The concept of accessibility needs to take into account factors like individual needs, preferences, and abilities as well as transportation, land use, and finances. This article proposes a sensitive space–time accessibility indicator from a regional perspective. This indicator, representing cumulative available activity time with a regional context, is different from both traditional accessibility measures (e.g., gravity-based accessibility, cumulative accessibility, utility-based accessibility, and so on) and previous concepts of individual space–time accessibility. The major advantage of this indicator is its sensitivity to the location of the anchor point, the transportation network, the distribution of activity sites over the whole region, and certain individual needs such as minimum activity times and activity time budgets. Several examples are worked to demonstrate these sensitivities and an innovative accessibility pattern which was previously unknown. This indicator should be helpful for planning agencies to assess urban environmental patterns and for business owners to evaluate potential business locations.
1. Introduction
Accessibility measures are often used by planning agencies to reflect the characteristics of both the land-use system and the transportation system (Shaw Citation2009). Conventional accessibility measures are sensitive to land-use changes, transportation, the amount and distribution of the supply of and demand for opportunities, and temporal constraints. For example, traditional accessibility measures (e.g., gravity-based accessibility, cumulative accessibility, utility-based accessibility) have usually focused on reflecting the impact of the transportation network, the efficiency and spatial extent of transport choices, social and economic equality, and similar factors on travel demand. Moreover, traditional accessibility measures have often been represented in a regional context (Handy and Clifton Citation2001) which can be easily understood by planning agencies and the public. However, up till now, the requirements of reflecting the influence of individual needs (Dong et al. Citation2006), preferences, and abilities (Geurs and Wee Citation2004) on space–time accessibility have remained open issues.
Space–time accessibility, based on Hägerstrand's (Citation1970) time-geography framework, is an alternative way to measure accessibility by incorporating a time dimension. Time geography offers an elegant conceptual framework for representing and analyzing human activities under various constraints in the space–time context (Yu Citation2006, Citation2007, Neutens et al. Citation2008). Existing space–time accessibility research efforts can be classified into three categories. First, some of these efforts have concentrated on conceptualizing space–time accessibility within transportation networks. For example, some studies have improved the capability to determine the impact of static and dynamic transportation networks on space–time accessibility (Wu and Miller Citation2001, Ettema and Timmermans Citation2007). These efforts need to be extended to reflect space–time accessibility in a regional context and also to capture the influence of individual needs (e.g., minimum activity times, activity time budgets) (Weber and Kwan Citation2002, Kim and Kwan Citation2003). The second category investigates accessibility from an individual perspective. Several studies have been carried out to incorporate space and time concepts into accessibility in a theoretical manner (Miller and Wu Citation2000) so as to provide analytic indicators to reflect individual and household characteristics. For instance, Kwan (Citation1998) developed 12 space–time accessibility measures to capture interpersonal differences and unravel gender differences in accessibility. Other individual-based approaches were developed to capture certain activity-based contextual effects (e.g., minimum activity times, activity time budgets) in accessibility analysis (Miller Citation1999, Smyth Citation2001, Wu and Miller, Citation2001, Weber and Kwan Citation2002, Citation2003, Miller Citation2005a, Yukio Citation2005). These efforts need to be extended if they are to reflect location-specific accessibility. The third category involves generating space–time accessibility surfaces from a regional perspective. Several attempts have been made to use space–time accessibility measures to provide different portrayals of individual accessibility in a regional context. For example, space–time accessibility surfaces have been generated to isolate the effects of individual-level variations from their geographical context using certain specific indicators, for example, the number of miles of street (MILES), the number of opportunities (OPPORTUNITIES), the area covered by these opportunities (AREA), the attractiveness of opportunities (WEIGHTED AREA), and a WEIGHTED AREA variable incorporating temporal constraints (called TIMED AREA) (Kwan Citation1998). These attempts need to be extended if they are to reflect the influence of anchor-point location and certain individual needs, because these indices do not use a single origin point when they sum up accessibilities from the daily potential path area.
Current space–time accessibility measures fall short of representing location-specific accessibility in a regional context. Time-geography-based space–time accessibility is subject to the constraints of the specific origin–destination (OD) pairs selected. It is useful for assessing a person's available activities from an individual perspective. With the help of the time-geography framework, several attempts have already been made to provide various portrayals of individual accessibility in regional contexts. For example, to compare space–time accessibility with gravity-based and cumulative accessibility indices, Kwan (Citation1998) estimated three space–time accessibility indices (length of network arcs, number of opportunities, and the weighted area of opportunities contained in prisms) by generating similar regional surfaces. Similarly, Weber and Kwan have used several space–time accessibility indicators (MILES, OPPORTUNITIES, AREA, WEIGHTED AREA and TIMED AREA) (Kwan Citation1998; Weber and Kwan Citation2003) to isolate the effects of individual-level variations from those of the geographical context. These space–time accessibility indicators reflected individual and household characteristics. The same approach was also used to evaluate the importance of distance on an individual's access to employment, shopping, and other services in the Portland, OR, metropolitan area (Kwan et al. Citation2003). In addition, as an alternative to representing space–time accessibility, several studies have been carried out to improve the ability to find realistic opportunity sets within a region. For instance, Kim and Kwan (Citation2003) developed a GIS-based geocomputational algorithm to improve space–time accessibility measures. This algorithm was designed to capture ‘the space–time characteristics of urban opportunities (e.g., their geographical distribution and opening hours), human behavior (e.g., time delays, minimum activity participation times, maximum travel-time thresholds), and the effect of the transport-network topology (e.g., one-way streets, turn restrictions, and overpasses)’ (Kim and Kwan Citation2003, p. 89). As a result, this algorithm can assess the available opportunities in a space–time prism (STP). The above approaches can be extended to reflect location-specific accessibility by incorporating individual needs.
This article presents a sensitive space–time accessibility indicator which is based on the available activity time within a prism and is capable of representing an anchor-point-specific local urban environment (Holl Citation2007) from a regional perspective. In addition, the indicator can capture the influence of individual needs (e.g., minimum activity times, activity time budgets) and preferences (e.g., kinds of activity places). The contribution of this indicator is that it is able to reveal the sensitivity patterns of cumulative available activity times between different specific locations. One potential use of this indicator may be to reflect the degree of space–time balance between various activity opportunities (e.g., points of interest, POIs) for social services, planning agencies, and business owners.
2. A space–time accessibility indicator
This section first introduces the basic concept of available activity time in a time-geography framework and then proposes a space–time accessibility indicator based on available activity time.
2.1. Available activity time in a time-geography framework
A space–time accessibility approach, as developed by Hägerstrand's (Citation1970) classical time-geography framework, is subject to both an individual's spatial and temporal constraints (e.g., capability, coupling, and authority). Two important concepts are central to time geography: the space–time path and the STP (Miller Citation2005a, Miller and Bridwell Citation2009). The space–time path traces an individual's movements and activities in space as a function of time. For example, in , there is a single space–time path between an OD pair. The time window between t1 and t4 represents the total time budget for the OD pair. The window between t1 and t2 is the shortest-path travel time from the origin to an activity location A, and that between t3 and t4 is the shortest-path travel time from the activity location A to the destination. The time period between t2 and t3 therefore indicates the available activity time at location A, which is the difference between the total time budget and the total travel time. The limits on all space–time paths between an OD pair create an accessibility regime known as the space–time prism (STP) (Wu and Miller Citation2001). If the available activity time in an activity place A is not less than the minimum activity time for this person's activity, this activity place is called an available activity place for this person.
2.2. Space–time accessibility indicator
This section introduces a space–time accessibility indicator, based on the available activity time concept in time geography, which is able to reveal location-specific space–time patterns from a regional perspective. This indicator is derived from the cumulative available activity time of all available activity places in an STP. This cumulative available activity time reflects the impact of the spatial and temporal balance of a transportation network and its distributed activity places on the activity time at a specific location.
Let T
o and T
d be, respectively, the expected departure time from the origin and the latest arrival time at the destination. The period between T
o and T
d is the total time budget, which is denoted as T; therefore, T = T
d−T
o. PSM(o,d) denotes the STP of an origin location o and a destination location d. The potential path area (Miller Citation2005b) of PSM(o,d) is denoted as PPA(o,d). For a particular kind of activity place (e.g., a shopping mall), there is an activity place set K, , where ks
represents an activity place s. Each element of K can be viewed as a candidate activity place. This activity place can be either available or unavailable. In this set, an available activity set is denoted as K′(o,d), where
,
. If there is no available activity place in K′(o,d), then K′(o,d) = φ. In contrast, if all activity places in K are available activity places, then K′(o,d) = K. In addition, the available activity time of
is denoted as
.
For a destination i, the cumulative activity time of all available activity places in a PSM(o,i) is assigned to the value of a space–time accessibility indicator Ai at the destination location. This indicator can be defined by the following equation:
The cumulative available activity time represents the total available time between an origin and a destination. The influence of a transportation network and its distributed activity places on accessibility measures is incorporated into this proposed space–time accessibility indicator by way of calculating the shortest path in the transportation network and identifying available activity places from among all activity places in this network. In addition, this indicator reflects the influence of available activity time as constrained by individual needs, such as kinds of activity places, total time budgets, and maximum travel time. The use of this indicator includes representing the space–time balance of various activity opportunities (e.g., POIs). To evaluate space–time accessibility between different POIs, each POI is chosen as an anchor point for calculating the values of the proposed space–time accessibility indicator.
According to the above definition, this indicator can be differentiated from traditional accessibility measures in two respects. First, the pattern of this proposed space–time accessibility measure reflects only the influence of available activity places. In the calculation of traditional accessibility measures, both available and unavailable activity places are included. Second, the contexts of spatial separation are different. For example, travel time, travel distance, and generalized travel cost are commonly used indices in traditional accessibility measures. However, the proposed space–time accessibility indicator, which represents available activity time, shifts the focus from information about travel in traditional accessibility measures to time availability in urban environments. These two differences distinguish the proposed space–time accessibility measure from traditional accessibility measures.
The context of the proposed space–time accessibility indicator is also different from that of other individual or household space–time accessibility measures. For example, existing measures concentrate on reflecting spatial separation patterns by means of travel miles, number of opportunities, weighted areas, and so on (Weber and Kwan Citation2003). The motivations of these traditional accessibility measures are to capture individual or household characteristics and to reflect the impacts of accessibility on individuals or households. The proposed indicator depicts the patterns of available activity time under constraints of space, time, and activity places, which has the potential to identify important business locations with high values on this space–time accessibility measure.
The choice of study area is a practical question for calculating space–time accessibility. Traditional accessibility measures are highly sensitive to the choice of study area (e.g., traffic analysis zones or census tracts). Choices in traditional accessibility measures can still be used to measure this proposed space–time accessibility. However, traffic analysis zones or census tracts are not appropriate to capture variations in locations’ available activity time within each area. In this article, a grid-based framework is used to measure space–time accessibility. If planners want more precise values of space–time accessibility, they can resample their study area with a higher grid resolution.
3. Implementation and examples
3.1. Implementation
The Beijing area in China () was chosen as the study area, and several types of POI (e.g., shopping malls, bank branches, and hospitals) were selected to illustrate space–time accessibility patterns using the proposed indicator. The study area included 1573 shopping malls, 3119 bank outlets, and 405 hospitals. The transportation network in this study area consisted of 74,212 directed links and 55,334 nodes and included complete topology data and connectivity relationships. The travel speed for each link was set equal to the accepted speed limit for the corresponding road type. For example, the accepted speed limit on China National Highways is 80 km/h (http://en.wikipedia.org/wiki/China_National_Highways). According to the demarcation method discussed previously, the study area was divided into a grid with 40 rows and 40 columns. To demonstrate the capability of the proposed indicator to reveal different spatial patterns of cumulative available activity time under constraints of different locations and different kinds of activity places, two anchor points, WANGFUJING and ZHONGKEYUAN on the map in , were selected as the origins of OD pairs in the following examples.
In this article, a space–time accessibility prototype program was developed to provide certain functions such as computing an STP, analyzing available activity places in an STP, calculating the proposed space–time indicator, and so on. In this program, the STP was calculated using the network-based method proposed by Yu and Shaw (Citation2008). ArcScene (a 3D GIS software component of ArcGIS 9.2) was chosen as a visualization environment for the space–time accessibility measure. The ‘Interpolate to Raster’ function (reverse distance-weighted) in the three-dimensional analyst module of ArcGIS9.2 was used to interpolate space–time accessibility raster maps.
3.2. Examples
This section illustrates several space–time accessibility results based on various constraints such as minimum activity times, anchor-point locations, the transportation network, and different kinds of activity places.
A simple scenario was designed as one in which a person is in WANGFUJING or ZHONGKEYUAN and wants to buy certain goods (requiring at least 10 minutes for small gifts, 20 minutes for an iPod Touch (an Apple multimedia player), and 30 minutes for a cellular phone) in a shopping mall located in the Beijing area. The total activity time budget is 1 hour.
Based on this scenario, three questions are probably of concern to planning agencies. For instance, what are the space–time accessibility patterns under different minimum activity time constraints (e.g., 10, 20, and 30 minutes)? What are the differences in the space–time accessibility patterns derived from different types of POI? What are the differences in space–time accessibility between different anchor points (e.g., WANGFUJING and ZHONGKEYUAN)? The following paragraphs will illustrate some of these differences to demonstrate the capability of the proposed measure to answer such questions about space–time accessibility.
illustrates the space–time accessibility results for WANGFUJING based on shopping malls. –c is constrained by minimum activity times of 30, 20, and 10 minutes, respectively. It has been observed that the maximum space–time accessibility values increase with decreasing minimum activity time. This phenomenon reveals that this kind of space–time accessibility measure is subject to the constraint of minimum activity time. Essentially, the explanation for this phenomenon is that the accessible activity places become fewer as the minimum activity time increases. As a result, within a fixed time budget, the longer the activity time that a person wants, the smaller the value of space–time accessibility that this person has. Similarly, the space–time accessibility of WANGFUJING based on banks is shown in . Both figures illustrate that patterns of space–time accessibility vary with minimum activity time. The locations of local peak values are not persistent as the minimum activity time decreases. As the minimum activity time constraint decreases, some new peak values appear, whereas other existing peak values disappear. This phenomenon reveals that this kind of space–time accessibility measure represents the actual separation patterns in both space and time dimensions to depict the influence of this constraint. As a result, the proposed indicator is helpful in analyzing changes in space–time accessibility for different minimum activity time constraints.
Figure 3. Space–time accessibility of WANGFUJING based on shopping malls with different minimum activity time constraints: (a) 30 minutes, (b) 20 minutes, (c) 10 minutes.

Figure 4. Space–time accessibility of WANGFUJING based on banks with different minimum activity time constraints: (a) 30 minutes, (b) 20 minutes, (c) 10 minutes.
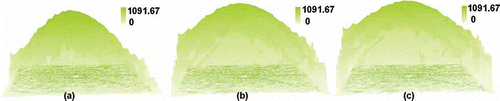
and show the space–time accessibilities of ZHONGKEYUAN based on shopping malls and banks. Both figures also illustrate the variation in space–time accessibilities as constrained by minimum activity time. The space–time accessibility results from to reveal that the proposed indicator can reflect the influences of minimum activity time and of different kinds of POI.
4. Analysis and discussion
4.1. Analysis
This section analyzes the space–time accessibility results from three perspectives: patterns of space–time accessibility results, space–time accessibility patterns for different kinds of POI, and space–time accessibility patterns for different anchor points. All three perspectives are helpful in answering the three questions posed in the previous section.
illustrates results from the first perspective. It shows a space–time accessibility pattern of the proposed indicator which is derived from ZHONGKEYUAN and based on the POI of banks. The minimum activity time in this space–time accessibility scenario is 30 minutes. The accessibility value decreases as one moves from the central area to the boundary area. This result can be explained by the fact that the central area contains more activity places than the boundary area, and therefore the cumulative activity time in the central area is higher than that in the boundary area. Moreover, several specific phenomena related to the spatial pattern of this accessibility measure are illustrated here. First, the spatial pattern in the area of ZHONGKEYUAN is more dependent on the distribution of activity locations than on the structure of the transportation network. For instance, area C, which has high space–time accessibility, is located in an area of high density of activity places. Second, the spatial pattern of space–time accessibility in areas far from the anchor point, ZHONGKEYUAN, is subject to two dominant factors: the structure of the transportation network and the distribution of activity places. For example, the radial roads near area A have higher space–time accessibility than the beltways in area A. This pattern is controlled by the structure of the transportation network rather than by the sparse distribution of activity places. In the case of area D, it contains more activity places than area A. In , the space–time accessibility in area D is higher than in its neighboring area. This pattern reveals that the influence of the density of activity places within area D on space–time accessibility is much greater than that of the transportation network. Third, the spatial pattern of space–time accessibility in inaccessible areas shows little variability. For example, the space–time accessibility in area B is close to zero because the POIs in area B are inaccessible when constrained by the minimum activity time of 30 minutes. This pattern can reveal the accessible area according to various constraints, including OD pairs, total time budgets, activity places, and minimum activity times. Such a representation of the proposed indicator should be helpful for planning agencies in capturing the sensitive influences of temporal constraints and transportation networks.
Figure 7. Space–time accessibility of ZHONGKEYUAN based on banks and the requirement of minimum activity time 30 minutes.
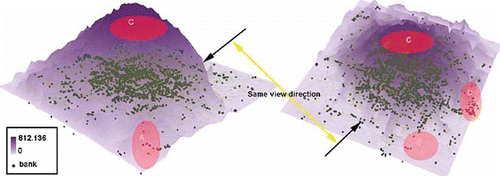
The second perspective involves the influence of different kinds of POI on space–time accessibility patterns. illustrates the space–time accessibilities of WANGFUJING based on banks, shopping malls, and hospitals. All of these are constrained by a minimum activity time of 20 minutes. The accessibility values of banks are indicated in green in and have the highest values. The accessibility values for hospitals are indicated in blue and are lower than those for banks and shopping malls. The accessibilities indicated in orange (shopping malls) are higher than those for hospitals and lower than those for banks. These patterns reveal that the proposed space–time accessibility indicator is capable of reflecting sensitive influences from different kinds of POI. The quantitative representation of this proposed indicator should be helpful for planning agencies in capturing the influencing characteristics of the supply of and demand for activity opportunities.
Figure 8. Space–time accessibility of WANGFUJING based on banks, shopping malls, and hospitals, all constrained by a minimum activity time of 20 minutes.
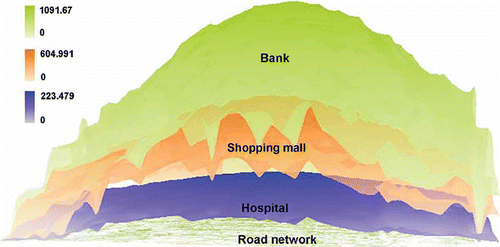
The third perspective involves the influence of different anchor points on space–time accessibility patterns. shows the space–time accessibilities of ZHONGKEYUAN and WANGFUJING based on banks under the constraint of minimum activity times of 10, 20, and 30 minutes. The spatial pattern of space–time accessibility originating from WANGFUJING is distinct from that originating from ZHONGKEYUAN, even under the same minimum activity time constraint. For example, and b illustrates that the space–time accessibilities derived for WANGFUJING (shown in green) are similar to but higher than those derived for ZHONGKEYUAN, whereas shows a different pattern, in that the space–time accessibility of other areas from ZHONGKEYUAN is higher than that from WANGFUJING, but the space–time accessibility of other areas from ZHONGKEYUAN is lower than that from WANGFUJING. The spatial demarcation of accessible areas is decided by anchor point and minimum activity time constraints. This phenomenon suggests that this proposed space–time accessibility measure is capable of reflecting the influence of different anchor points.
Figure 9. Space–time accessibility of ZHONGKEYUAN and WANGFUJING based on banks with minimum activity time constraints of 10 (a), 20 (b), and 30 (c) minutes.
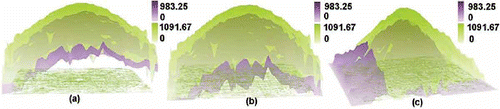
In summary, the above analysis of three perspectives suggests that the proposed regional space–time accessibility measure is sensitive to the distribution of different kinds of activity places, minimum activity times, and anchor points. These analyses also demonstrate that this indicator is capable of answering the three questions posed in the previous section.
4.2. Discussion
This section discusses some questions about the proposed space–time accessibility indicator, for example, what is the fundamental difference between it and gravity-based accessibility measures? To which category does this indicator belong? And what is its potential application?
shows gravity-based accessibility results for different kinds of activity places, such as banks and shopping malls. The patterns of gravity-based accessibility measures often reflect the influence of certain factors (e.g., different kinds of activity places) on the whole area, whereas the pattern of the proposed space–time accessibility measure is more responsive to the influences of different anchor points. For the same kind of activity place, the gravity-based accessibility patterns are unique because they are derived from all activity places of a given kind. However, the pattern of the proposed space–time accessibility measure represents more the influence of various factors on a specific anchor-point location. This space–time accessibility pattern varies with the location of the anchor point. This indicator thus provides an additional dimension for agencies to evaluate space–time accessibility. This fundamental difference brings new insights into the study of space–time balance in urban areas.
Figure 10. Gravity-based accessibility of Beijing City derived for different kinds of activity places: (a) banks, (b) shopping malls.
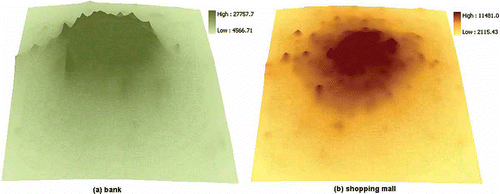
The proposed indicator addresses the field of urban accessibility while integrating individual-based space–time accessibility. This indicator not only represents individual requirements such as time budgets, minimum activity times, kind of activity places, and so on, but also represents the influence of the transportation network and the distribution of activity places on accessibility patterns in the urban environment. This indicator provides an easy approach to envisioning differences in individual needs and may even facilitate more practical ways for planning agencies to evaluate the needs of individuals in a whole planning area.
The implications of the patterns generated by this proposed indicator reveal a sensitive space–time balance of cumulative available activity time, which can be used to find the activity places with a high total available activity time in the urban environment. As a practical application, if one business owner wants to open a new place of business and needs more activity time from potential consumers, (s)he can discover the currently available time pattern of related kinds of activity places using this indicator and can also find the potential business areas which meet the activity time criteria. By integrating these results with traditional accessibility measures, (s)he can evaluate potential activity locations with a large service area. As for planning agencies, they can create traditional accessibility maps to find the places with high accessibility and then can use this proposed indicator to check specific locations with high space–time accessibility to evaluate the actual accessibility service level in their plans.
5. Conclusions
This article presents a sensitive space–time accessibility indicator for measuring space–time accessibility in a regional context. This indicator differs from traditional accessibility measures (e.g., gravity, cumulative, and utility-based accessibility measures) and from individual space–time accessibility measures. For example, traditional accessibility measures do not take individual needs (e.g., minimum activity time) into account, and individual space–time accessibility measures do not reflect regional accessibility patterns. However, this proposed space–time accessibility indicator reflects space–time accessibility from a regional perspective while incorporating individual needs including minimum activity times, different kinds of activity places, and different locations of anchor points.
The proposed indicator has several advantages. First, a major advantage is that this indicator is sensitive to the minimum activity time, the location of the anchor point, the transportation network, and different kinds of activity places. This advantage has already been demonstrated by the examples in the previous section. Second, this indicator provides an innovative approach to examining regional space–time accessibility. Traditional accessibility measures have often focused on travel time, travel distance, or generalized transport cost. They have seldom concentrated on available activity time. However, available activity time is an important factor for individuals to make an informed travel decision. The proposed indicator shifts the focus from information about travel in traditional accessibility measures to time availability in urban environments. Third, this indicator can be extended to assess the spatial balance of various activity opportunities and temporal constraints. Spatial balance is a key factor in the process of designing urban land-use and transportation plans. It also plays an important role in assessing social equity and incorporating socioeconomic status into the concept of space–time accessibility.
Inevitably, this proposed indicator also has several disadvantages. The first is its daunting and time-consuming computational intensity. The most time-consuming procedure is the calculation of a number of STPs equal to the number of grid cells. Therefore, the computation of a higher resolution grid will cost more time than that of a lower resolution grid in the same area. One alternative approach is to take full advantage of parallel computing technologies using high-performance computers. Second, this space–time accessibility indicator needs to take into account even more time constraints, for example, working hours, opening hours, dynamic travel-time measures, congestion, time delays, maximum and minimum activity time thresholds for different human activities, time budget for daily activities, individual time preferences and abilities, and even the time availabilities of e-stores in virtual space. Without a doubt, all these time constraints are very helpful in defining more realistic space–time accessibility measures than those proposed in previous research. One vital challenge is the difficulty of collecting time-based data. A feasible solution is using location-aware technologies (LAT) to collect detailed space–time trajectories at the individual level. For example, global positioning system (GPS) receivers or radiolocation methods, which piggyback on wireless communication networks (Smyth Citation2001, Miller Citation2004), have already collected a huge amount of individual data. It remains difficult to design an interactive interface for this data-collection task while meeting the needs of the majority of individuals.
Several research avenues remain to be pursued in future. One pressing challenge is to mitigate the problems of computation intensity and data availability for the proposed indicator. Although some alternatives are suggested in the previous paragraph, more innovative yet feasible methods are still needed to address these deficiencies. The second research challenge is to incorporate these proposed indicators into currently prevalent accessibility models (e.g., gravity models, activity travel/choice models, etc.). For example, in what ways can this indicator be incorporated into commonly used accessibility models? How can this indicator help the prevailing accessibility models to represent more useful patterns? The third research challenge is to measure space–time accessibility in a hybrid space which includes both physical and virtual environments (Kwan Citation2000, Miller Citation2007, Yu and Shaw Citation2008, Shaw and Yu Citation2009). Information and communication technologies (ICT) have changed people's lifestyle in society. One challenge of traditional accessibility measures is to reflect the influences of ICT on accessibility. For example, Muhammad has analyzed the influence of ICT on job accessibility (Muhammad et al., Citation2008). However, more efforts are needed to measure the space–time accessibility of hybrid space in both theory and practice. In short, these research avenues challenge current models and methods of accessibility.
Acknowledgments
This research was supported in part by the National Science Foundation of China (grants #40701153, #40971233, #60872132) and the Technical Innovation Project of the Ministry of Education of China (grant #108085).
References
- Dong , X.J. 2006 . Moving from trip-based to activity-based measures of accessibility . Transportation Research Part A , 40 : 163 – 180 .
- Ettema , D. and Timmermans , H. 2007 . Space–time accessibility under conditions of uncertain travel times: theory and numerical simulations . Geographical Analysis , 39 : 217 – 240 .
- Geurs , K.T. and Wee , B.V. 2004 . Accessibility evaluation of land-use and transport strategies: review and research directions . Journal of Transport Geography , 12 : 127 – 140 .
- Hägerstrand , T. 1970 . What about people in regional science? . Papers of the Regional Science Association , 24 : 7 – 21 .
- Handy , S.L. and Cliftton , K.J. 2001 . Evaluating neighborhood accessibility: possibilities and practicalities . Journal of Transportation and Statistics , 4 ( 2/3 ) : 67 – 78 .
- Holl , A. 2007 . Twenty years of accessibility improvements. The case of the Spanish motorway building programme . Journal of Transport Geography , 15 : 286 – 297 .
- Kim , H.M. and Kwan , M.P. 2003 . Space–time accessibility measures: a geocomputational algorithm with a focus on the feasible opportunity set and possible activity duration . Journal of geographical Systems , 5 : 71 – 91 .
- Kwan , M.P. 1998 . Space–time and integral measure of individual accessibility: a comparative analysis using a point-based framework . Geographical Analysis , 30 ( 3 ) : 191 – 216 .
- Kwan , M.P. 2000 . “ Extensibility and individual hybrid-accessibility in space–time: a multi-scale representation in GIS ” . In Information, place and cyberspace: issues in accessibility , Edited by: Janelle , D. and Hodge , D. 241 – 256 . Berlin : Springer-Verlag .
- Kwan , M.P. 2003 . Recent advances in accessibility research representation, methodology and applications . Journal of geographical Systems , 5 : 129 – 138 .
- Miller , H.J. 1999 . Measuring space–time accessibility benefits within transportation networks: basic theory and computational procedures . Geographical Analysis , 31 : 187 – 212 .
- Miller , H.J. 2004 . Tobler's first law and spatial analysis . Annals of the Association of American Geographers , 94 ( 2 ) : 284 – 289 .
- Miller , H.J. 2005a . “ Place-based versus people-based accessibility ” . In Access to destinations Edited by: Levinson , D. and Krizek , K. J. 63 – 89 . London: Elsevier
- Miller , H.J. 2005b . “ What about people in geographic information science? ” . In Re-presenting GIS , Edited by: Fisher , P. and Unwin , D. Chichester, , UK : Wiley .
- Miller , H.J. 2007 . Place-based and people-based geographic information science . Geography Compass , 1 : 503 – 535 .
- Miller , H.J. and Bridwell , S.A. 2009 . A field-based theory for time geography . Annals of the Association of American Geographers , 99 : 49 – 75 .
- Miller , H.J. and Wu , Y.H. 2000 . GIS software for measuring space–time accessibility in transportation planning and analysis . Geoinformatica , 4 ( 2 ) : 141 – 159 .
- Muhammad , S. , de Jong , T. and Ottens , H.F.L. 2008 . Job accessibility under the influence of information and communication technologies, in the Netherlands . Journal of Transport Geography , 16 : 203 – 216 .
- Neutens , T. , Van de Weghe , N. and Witlox , F. 2008 . A three-dimensional network-based space–time prism . Journal of Geographical Systems , 10 ( 1 ) : 89 – 107 .
- Shaw , S-L. 2009 . “ Transportation and land use ” . In International encyclopedia of human geography , Edited by: Kitchin , R. and Thrift , N. Vol. 11 , 470 – 475 . Oxford, , UK : Elsevier .
- Shaw , S-L. and Yu , H. 2009 . A GIS-based time-geographic approach of studying individual activities and interactions in a hybrid physical-virtual space . Journal of Transport Geography , 17 ( 2 ) : 141 – 149 .
- Smyth , C.S. 2001 . “ Mining mobile trajectories ” . In Geographic data mining and knowledge discovery , Edited by: Miller , H.J. and Han , J. 337 – 361 . London : Taylor and Francis .
- Weber , J. and Kwan , M.P. 2002 . Bring time back In: A study on the influence of travel time variations and facility opening hours on individual accessibility . The Professional Geographer , 54 ( 2 ) : 226 – 240 .
- Weber , J. and Kwan , M.P. 2003 . Evaluating the effects of geographic contexts on individual accessibility: a multilevel approach . Urban Geography , 24 ( 8 ) : 647 – 671 .
- Wu , Y.H. and Miller , H.J. 2001 . Computational tools for measuring space–time accessibility within dynamic flow transportation networks . Journal of Transportation and Statistics , 4 ( 2/3 ) : 1 – 14 .
- Yu , H. 2006 . Spatial-temporal GIS design for exploring interactions of human activities . Cartography and Geographic Information Science , 33 ( 1 ) : 3 – 19 .
- Yu , H. 2007 . Visualizing and analyzing activities in an integrated space–time environment: temporal GIS design and implementation . Transportation Research Record: Journal of the Transportation Research Board , 2024 : 54 – 62 .
- Yu , H.B. and Shaw , S.L. 2008 . Exploring potential human activities in physical and virtual spaces: a spatio-temporal GIS approach . International Journal of Geographical Information Science , 22 ( 4 ) : 409 – 430 .
- Yukio , S. 2005 . Spatio-temporal analysis of the distribution of urban facilities in terms of accessibility . Papers in Regional Science , 1 ( 03 ) : 61 – 84 .