Abstract
To understand the variation of coastal wetlands, Park initiated the sea level affecting marshes model (SLAMM) in 1986. One can use the model to simulate changes of land-use and land-cover types in coastal zones with ground measurement-based model inputs. Parameterization of the model is not easy because of the requirement of extensive field measurements that can be prohibitive in labor and cost for the study of a large spatial extent. Thus, an alternative approach to provide the model's inputs must be developed. Remotely sensed and geo-spatial datasets were explored to deliver the inputs. Using the datasets, we derived as many categories of the land-use and land-cover types determined and needed by the SLAMM as possible. Then, for categories required by the SLAMM and existing in the study area, but not derivable from the remote sensing and geo-spatial datasets, we simply recoded the categories into categories obtained from the remote sensing and geo-spatial datasets spatially. We compared the SLAMM outputs before and after the recoding. At the test site of the state of Washington, USA, promising results were obtained.
1. Introduction
Wetlands cover about 6% of the Earth's surface, but provide living environment for about 20% of the plant and animal species on Earth. Thus, they play irreplaceable and vital ecological and environmental roles. However, worldwide coastal wetlands are negatively affected by combinations of population growth, continuous increase in human migration toward coastal areas, nature- and human-induced coastal disturbances, and a rising sea level. Due to global climate changes, the Intergovernmental Panel on Climate Change indicated that the global average sea level would rise 30–100 cm by year 2100 (Solomon et al. Citation2007). This will inevitably cause the relocation and migration of human settlements and animal habitats, as well as coastal ecological and environmental changes.
To understand the processes and, eventually, the consequences, Park et al. (Citation1986) initiated the sea level affecting marshes model (SLAMM) that simulates changes of land-use and land-cover (LULC) types through time in coastal zones. After over 20 years of development, the SLAMM has become an important simulation and forecast model in coastal research. It has been used to provide information in decision-making processes for environmental protection and economic development (Park Citation1991, Craft et al. Citation2009). Unfortunately, parameterization of the model can be difficult because extensive field measurements are required, which can be labor intensive and cost prohibitive for the study of an area with a large spatial extent. In addition, some coastal areas are difficult to access, much less to carry out fieldwork. All will certainly limit the model's applicability. Therefore, an alternative to provide the model's inputs must be developed. Using remotely sensed and geo-spatial datasets, we will derive as many LULC types needed by the SLAMM as possible. For categories required by the SLAMM and existing in the study area, but not derivable from the remote sensing and geo-spatial datasets, we simply recode these categories into categories derived from the remote sensing and geo-spatial datasets spatially, and run the model. Finally, we compare the SLAMM outputs before and after the recoding. Details are given below.
2. A brief overview of the SLAMM
The SLAMM1 was developed in the 1980s (Park et al. Citation1986), followed by the SLAMM2 that was used to simulate about 20% of the US coast. In 1991, results from the SLAMM2 were used in a congressional hearing on the potential effects of the global climate change by the EPA of the United State government (Park Citation1991). Then, the SLAMM3 was applied to the St. Mary's Estuary of Florida and Georgia, Puget Sound of Washington, and coastal area of South Florida (Lee et al. Citation1992). More recent studies were undertaken with the SLAMM4, including simulations for the San Francisco Bay, Humboldt Bay, and most areas of the Delaware Bay and Galveston Bay (TX) (Clough and Park Citation2009). The SLAMM5 was used in coasts of South Carolina and Georgia (Clough et al. Citation2009, Citation2010). In 2007, research was also done with the SLAMM5 in the Puget Sound and Columbia River Basin, northwest of United States (Glick et al. Citation2007, Clough et al. (XL) Citation2010). The SLAMM6 was released in December 2009 (Clough et al. Citation2010). It includes numerous upgrades. Accretion feedback component, salinity model, integrated elevation analysis, and flexible elevation ranges, to name a few, are the most important improvements (Clough et al. Citation2009, Citation2010). The SLAMM6.01 is the most current version.
In general, the SLAMM is a grid-based model, which contains a flexible but complex decision tree to show changes among coastal LULC classes geographically (Clough et al. Citation2009). To simulate the changes in each grid, one needs to provide initial LULC-type conditions, which could include data from the National Wetlands Inventory, Digital Elevation Model (DEM) slope, dyke, percent imperious raster, and other coastal parameters. Of all the input data, elevation data with a fine vertical resolution can be critical because a high-quality DEM can better and accurately demarcate the penetration extent of salt water versus freshwater and frequency of the inundation of swamps and marshes than a DEM of coarse vertical resolution. In addition, the elevation value is one of the key factors in determining future geomorphic features of landforms in low elevation lands, such as beaches, tidal flats, marshes, and swamps (Clough et al. Citation2009). A set of scenarios in sea-level rise report by the Intergovernmental Panel on Climate Change for the next 100 years (IPCC Citation2001) is incorporated in the SLAMM. Furthermore, six primary processes – inundation, erosion, overwash, saturation, accretion, and salinity (Clough et al. Citation2009) – are included in model simulation. Finally, a model for wetland conversion has been built, which is mainly used to interpret and quantify the loss/gain of wetlands to other LULC types.
3. Analytical approach
3.1. Derivation of LULC types for SLAMM using remotely sensed and geo-spatial datasets
Within the SLAMM, 24 LULC types are included: developed dry land, undeveloped dry land, swamp, cypress swamp, tidal swamp, mangrove, inland freshwater marsh, tidal freshwater marsh, transitional salt marsh, regularly flooded marsh, irregularly flooded marsh, estuarine beach, tidal flat, ocean beach, ocean flat, rocky intertidal flat, vegetated tidal flat, inland shore, backshore, inland open water, riverine tidal water, estuarine open water, tidal creek, and ocean (Clough et al. Citation2009). It is possible that there are less or more than 24 types for a particular study area. These types can be derivable from fieldwork. Some or all of them can be obtained from remotely sensed data and geo-spatial datasets.
To facilitate the derivations of the model inputs from the remote sensing and geo-spatial datasets, we can regroup the 24 LULC types into 6 new categories on the basis of the following 6 variables: geographic location, spatial extent, level of moisture content, salinity, tidal water effect, and human activity (e.g., developed vs. undeveloped). The categories are
1. | dry lands including the original undeveloped dry land and developed dry land; | ||||
2. | swamps (the original swamp, cypress swamp, tidal swamp, and mangrove); | ||||
3. | marshes (the inland freshwater marsh, tidal freshwater marsh, transitional salt marsh, regularly flooded marsh, and irregularly flooded marsh); | ||||
4. | intertidal mudflats (the estuarine beach, tidal flat, ocean beach, ocean flat, rocky intertidal flat, and vegetated tidal flat); | ||||
5. | shores (the inland shore and backshore); and | ||||
6. | water bodies (the inland open water, riverine tidal water, estuarine open water, tidal creek, and ocean). |
The reason for this regrouping is that we can use the identified six variables to guide the classification or delineation processes of the LULC types using the remotely sensed and geo-spatial datasets. For instance, the dry land is characterized by its high ground, but land features can be a road or building (developed) or an undeveloped and vegetated surface at high ground. The swamp and marsh are constantly flooded whereas main land covers differ. There are trees such as mangroves and cypresses as well as aquatic plants in swamps, but aquatic plants dominate marsh lands. Intertidal mudflat is located at intertidal location and with a proximity to a water body. Therefore, our approach to derive the LULC types needed by SLAMM is to use the remotely sensed and geo-spatial datasets individually and/or in combination to obtain as many needed types as possible. For the underivable or undistinguishable types, we will recode them with a known category. For instance, if we can only identify shore and cannot determine whether it is an inland shore or a backshore, then we will recode both shores as the inland shore and then run the model. We also run the model after the recode of both shores as the backshore. We compare the model outputs with two different recordings and assess the discrepancy of the outputs. Given a particular threshold, we finally determine whether the recoding is acceptable. If acceptable, we reduce the model's requirement on the number of LULC types or minimize the data dependence of the SLAMM. Consequently, one moves away from the labor-intensive and cost-prohibitive fieldwork in order to get all the needed LULC types and expand the applicability of the model in a large spatial extent.
3.2. Study area and dataset
The study area, Bellingham Bay, is located from 48°41′ to 48°51′N and between 122°28′W and 122°48′W in northern Washington, USA. There are two major reasons for choosing this site. All the needed LULC types of the SLAMM are available here as this is one of the intensive study sites of the researchers who developed the SLAMM. Also, we have remotely sensed data from the Advanced Land Observation Satellite (ALOS) of Japan and geo-spatial datasets (e.g., DEM). The ALOS imagery includes optical images on 22 August 2009 and 17 February 2008, from the Advanced Visible and Near Infrared Radiometer type 2 (AVNIR-2) sensor and L-band HH radar image on 10 July 2006 from the Phased Array type L-band Synthetic Aperture Radar (PALSAR) sensor. Thus, we can have two sets of outputs and perform a cross-validation.
4. Results and discussions
4.1. Derivation of the LULC data using remote sensing and geo-spatial datasets
At the site, there are 18 LULC types (out of a total of 24), and the missing ones are cypress swamp, mangrove, ocean beach, vegetated tidal flat, backshore, and tidal creek. Then, image classification was done with optical images acquired in February 2008 and August 2009. Ten categories were obtained including river, lake, forest, road, intertidal mudflat, dry land, farmland, wetland, building, and ocean. is a portion of the classified image. Compared with the categories used in the SLAMM, the road and building, as well as farmland, are related to the developed dry land. Forest is the undeveloped dry land. The dry land in the classification could be the developed or undeveloped dry land category in the SLAMM. The lake and river correspond to inland open water. Thus, we have derived the developed dry land, undeveloped dry land, wetland, inland open water, intertidal mudflat, and ocean, the six categories of the SLAMM datasets. There are still 12 categories missing and they are mistakenly classified as one or more of the 6 categories. Thus, further delineation of the categories from the optical data with the incorporation of radar and geo-spatial datasets is needed. In particular,
1. | swamp and forest can be distinguished by using optical images and DEM data; | ||||
2. | inland freshwater marsh and dry land can be differentiated by using multi-temporal optical images; | ||||
3. | inland open water, riverine tidal water, and estuarine open water, all having similar spectral reflectance characteristics, can be separated using their geographic locations. For instance, for the given characteristics, if the feature is located inland, then it will be inland open water; and | ||||
4. | wetland and farmland can be recognized by using radar images (Kwoun and Lu Citation2009) together with optical images. |
Of the 18 classes of the SLAMM available in the study area, so far 10 of them have been derived using the combination of remote sensing and geo-spatial datasets. They are developed dry land, undeveloped dry land, swamp, tidal swamp, inland freshwater marsh, inland open water, ocean, estuarine open water, ocean beach, and riverine tidal water. However, there are still eight categories missing. Recoding them into the categories delineated by remote sensing and geo-spatial datasets spatially and the SLAMM's sensitivities on the recoding are studied next.
4.2. SLAMM's sensitivity on category recoding
At the study site, 10 categories have been derived using the remote sensing and geo-spatial datasets. To study the discrepancy in the model output, we run the model without and with the category recoding of the inputs. The null hypothesis is that there is no difference for recoding of some or all categories. The alternative hypothesis is that difference exists. It should be pointed out that even if the null hypothesis is only acceptable in the recoding of one category, then a (small) progress on reducing the SLAMM's dependency on LULC categories is made. Thus, the SLAMM's applicability increases. After overlaying the available LULC categories and derived categories from remote sensing and geo-spatial datasets, we observed the following:
1. | Original irregularly flooded marsh, transition salt marsh, tidal freshwater marsh, and regularly flooded marsh were classified as marsh lands because they have similar spectrum characteristics and radar backscatter, as well as similar geographical features (e.g., all are near water and with presence of vegetation). | ||||
2. | Original tidal flat and estuarine beach were classified or grouped as ocean beach. | ||||
3. | Original inland shore was identified as swamp. | ||||
4. | Original rocky intertidal category was developed as dry land. |
Therefore, a general question is: Can the categories required by the SLAMM be regrouped? If so, can we use the category derived from remote sensing and geo-spatial datasets as the replacement spatially, for example, the original tidal flat, estuarine beach, or ocean beach as ocean beach? What are the impacts on the land-ward or sea-ward migration of the estuarine open water with time and on the land-ward and/or sea-ward movements of ocean through time because the categories to be recoded are near water? We will answer these questions next.
Using the inputs including National Wetlands Inventory, DEM, slope, dyke, impervious layer datasets, and other coastal parameters (), we ran a series of SLAMM simulations without and with the recoding through time between 1980 and 2100. A subset of results is presented below, which highlights what is possible and what is not. When the irregularly flooded marsh, transition salt marsh, tidal freshwater marsh, and regularly flooded marsh were combined into any one of the categories, modeled sizes of the inland freshwater marsh were almost identical ( ). As sea level rises, the inland freshwater marsh will gradually disappear and be converted into one of the four marsh categories. Thus, one can not only combine these categories into a generic marsh class but also assess the conversion of freshwater marsh into nonfreshwater marsh.
Table 1. Seventeen input parameters at the study site, Bellingham, WA, USA (Clough et al. Citation2009)
Figure 2. The size of inland freshwater marsh through time as irregularly flooded marsh, transition salt marsh, tidal freshwater marsh, and regularly flooded marsh were recoded as any one of four categories.
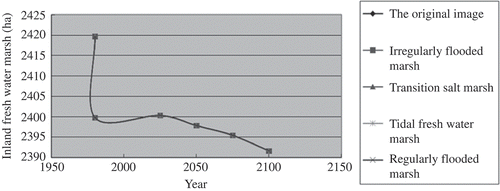
As the sea level continuously rises, the marsh lands eventually become open water in estuary or sea. Thus, the influence of the combining scheme on the size of estuarine open water was studied to assess the model's sensitivity and ultimately understand the transition process between marsh land and open water. Simulated sizes of the open water through time are given in . For irregularly flooded marsh, transitional salt marsh, tidal freshwater marsh, and regularly flooded marsh, regardless of how one recoded the other three types into any one of the types, all outputs or lines were identical. Thus, one can combine four marsh categories into one.
Figure 3. The size of estuarine open water through time as irregularly flooded marsh, transition salt marsh, tidal freshwater marsh, and regularly flooded marsh were recoded as any one of four categories.
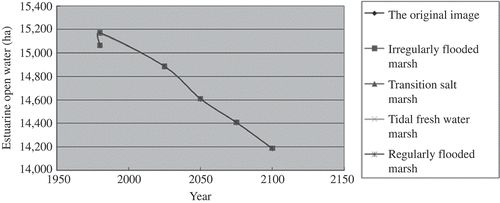
As we recoded the tidal flat, ocean beach, and estuarine beach as tidal flat (the line with a solid square symbol), ocean beach (the line with a solid triangle symbol), or estuarine beach (the line with a cross sign) and compared the modeled outputs with those without the category recoding (i.e., the original image or the line with a solid diamond symbol) (), we noticed that there was almost no difference in the size of estuarine open water through time when the categories were combined as the tidal flat or estuarine beach. However, there was some discrepancy when the categories were recoded as the ocean beach which is the category derived from the remote sensing and geo-spatial datasets. Thus, we could recode the categories as the tidal flat or estuarine beach, but would not group them as ocean beach. We have two options: renaming the derived ocean beach as the tidal flat or estuarine beach, or separating the ocean beach from the tidal flat and estuarine beach. No matter which option was chosen, we did combine the tidal flat and estuarine beach as one category and treated the tidal flat, ocean beach, and estuarine beach as the tidal flat or estuarine beach. The combinations have a minimum influence on the size of estuarine open water through time.
Figure 4. The size of estuarine open water through time as tidal flat, ocean beach, and estuarine beach were combined as tidal beach, ocean beach, or estuarine beach.
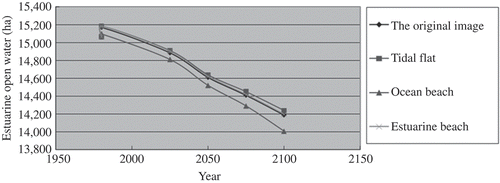
Tidal flat, ocean beach, and estuarine beach can be spatially near ocean. What was the impact of the above combination on the variation of ocean size through time? Again, there was almost none when the tidal flat, ocean beach, and estuarine beach were treated as tidal flat or estuarine beach. There was some difference when the categories were recoded as the ocean beach (). Therefore, options discussed above could still be applied.
5. Concluding remarks
In this study, the applicability of remotely sensed and geo-spatial datasets to the SLAMM was investigated. A two-step method was investigated. First, we used the remote sensing and geo-spatial datasets to delineate as many categories of the LULC types required by the SLAMM as possible. Second, categories that have very similar characteristics in remote sensing and geo-spatial datasets and therefore are undistinguishable are recoded into a category that is derived from the remote sensing and geo-spatial datasets. Doing so, one could reduce the number of input parameters to the SLAMM, and thus increase the SLAMM's applicability. This method was evaluated near Bellingham, WA, USA. This is one of the test sites included in the SLAMM release files. All input parameters the SLAMM needed were available. Also, remotely sensed optical and radar data of ALOS of Japan and geo-spatial datasets were available. Promising results were obtained when cross-comparing the model outputs with and without the recoding.
Acknowledgement
This work was supported by a grant from National Natural Science Foundation of China (No. 41021061). The ALOS PALSAR and AVNIR-2 data used in this article were provided by the Japan Aerospace Exploration Agency (JAXA) through a contract to the East Carolina University, NC, USA.
References
- Clough , J.S. , Park , R.A. and Fuller , R. 2009 . The nature conservancy: SLAMM 6 technical documentation ,
- Clough , J.S. , Park , R.A. and Fuller , R. 2010 . The nature conservancy: SLAMM6 release notes ,
- Craft , C. 2009 . “ Effects of accelerated sea level rise on delivery of ecosystem services provided by tidal marshes: a simulation of the Georgia (USA) Coast ” . In Frontiers in Ecology and the Environment Vol. 7 , doi: 10.1890/070219
- Glick , P. , Clough , J. and Nunley , B. 2007 . Sea-level rise and coastal habitats in the Pacific northwest National Wild life Federation, USA
- IPCC Third Assessment Report-Climate Change, 2001. http://www.grida.no/publications/other/ipcctar/ (http://www.grida.no/publications/other/ipcctar/) (Accessed: 25 March 2010 ).
- Kwoun , O.-I. and Lu , Z. 2009 . Multi-temporal RADARSAT-1 and ERS backscattering signatures of coastal wetlands in southeastern Louisiana . Photogrammetric Engineering & Remote Sensing , 75 : 607 – 617 .
- Lee , J.K. , Park , R.A. and Mausel , P.W. 1992 . Application of geoprocessing and simulation modeling to estimate impacts of sea level rise on the northeast coast of Florida . Photogrammetric Engineering and Remote Sensing , 58 : 1579 – 1586 .
- Park , R.A. 1991 . Global climate change and greenhouse emissions , 171 – 182 . Washington, DC : Subcommittee on Health and Environment, US House of Representatives .
- Park , R. A. , Armentano , T.V. and Cloonan , C.L. 1986 . “ Predicting the effects of sea level rise on coastal wetlands ” . In Effects of changes in stratospheric ozone and global climate , Edited by: Titus , J.G. Vol. 4 , 129 – 152 . Washington, DC : U.S. Environmental Protection Agency . sea level rise
- Solomon , S. 2007 . Climate change2007: the physical science basis: contribution of Working Group I to the Fourth Assessment Report of the Intergovernmental Panel on Climate Change , Cambridge : Cambridge University Press .