Abstract
Slums are examples of localized communities within third-world urban systems representing a range of vulnerabilities and adaptive capacities. This study examines vulnerability in relation to flooding, environmental degradation, social status, demographics, and health in the slums of Accra, Ghana, by utilizing a place-based approach informed by fieldwork, remote sensing, census data, and geographically weighted regression (GWR). The study objectives are threefold: (1) to move slums from a dichotomous into a continuous classification and examine the spatial patterns of the gradient, (2) to develop measures of vulnerability for a developing world city and model the relationship between slums and vulnerability, and (3) to assess if the most vulnerable individuals live in the worst slums. A previously developed slum index is utilized, and four new measures of vulnerability are developed through principal components analysis (PCA), including a novel component of health vulnerability based on child mortality. Visualizations of the vulnerability measures assess spatial patterns of vulnerability in Accra. Ordinary least squares (OLS), spatial, and GWR model the ability of the slum index to predict the four vulnerability measures. The slum index performs well for three of the four vulnerability measures, but is least able to predict health vulnerability, underscoring the complex relationship between slums and child mortality in Accra. Finally, quintile analysis demonstrates the elevated prevalence of high vulnerability in places with high slum index scores.
1. Introduction
Between 2010 and 2050, the world's estimated population increase of 2.3 billion people is expected to be absorbed by urban areas in less developed regions, with Africa's urban population projected to increase by 0.8 billion (United Nations Population Division Citation2010). Governments are falling behind in the struggle to provide infrastructure to these rapidly expanding urban areas. Even now, one in three urban dwellers live in slums globally, with that number increasing to three out of four in sub-Saharan Africa (UN-Habitat Citation2006, UN-Habitat Citation2008). Literature concerning the enumeration and categorization of slums is scarce, while the definition of what constitutes a slum is often vague (Milbert Citation2006). However, there is a ‘common knowledge’ understanding of slums as places characterized by overcrowding, marginalization, harmful environmental exposure, poverty, social disadvantage, insecurity, lack of access to amenities, and poor health. These features are associated with increased risk of and vulnerability to natural and man-made hazards, particularly decreased health and well-being (Pelling Citation2003, Davis Citation2006). In other words, slums are universally considered to be vulnerable places and are prime targets for mitigation efforts.
We argue, however, that slums exhibit considerable variability and represent examples of localized communities within third-world urban systems. They are microcosms of complex historical, social, and biophysical processes resulting in a range of vulnerabilities and adaptive capacities. Adger (Citation2006) points to mounting evidence that localized communities are able to adapt latent local knowledge and experience to help cope with hazards. If mitigation efforts are to be effective, city planning for hazard events or slum improvement must consider the variability of the physical (both location and physical traits), socioeconomic, demographic, and health-based characteristics that make up slums. In other words, it is not enough to dichotomize cities into slums and non-slums. Assessing slums must come from a place-based approach that recognizes place as a shaping force of unique local vulnerabilities and adaptability. Spatial technologies and techniques are particularly useful in developing a place-based approach to analyzing slum vulnerability. The National Research Council (Citation2007) recently emphasized the need for integrating demographic data with geospatial data, satellite imagery, and geographic information systems (GIS) overlays of infrastructure in order to better assess how many individuals are at risk and their particular demographic and social needs in times of crisis.
In this article, we explore the premise that slums vary in their nature and the vulnerability of their residents and that there is a strong spatial component to these patterns of vulnerability. We do this by integrating physical, socioeconomic, demographic, and health aspects of vulnerability for slums in Accra, Ghana, to explore the following research questions: (1) Can slums predict vulnerability? (2) Do the most vulnerable people live in the worst slums? Drawing on fieldwork, remotely sensed imagery, spatially referenced census data, and geographically weighted regression (GWR), we assess the complex spatial relationship of slums and vulnerability in Accra.
2. Defining slums and population vulnerability
According to UN-Habitat (Citation2003, 2006), a household is a slum if it lacks one or more of the following characteristics: structural quality and durability of dwellings, access to safe water, sufficient living area, access to sanitation facilities, and security of tenure. This definition of slum provides a starting point for identifying slums, and the focus on the physical characteristics of slums, namely poor housing and inadequate infrastructure (‘unsafe housing’ as UN-Habitat calls it), has been emphasized in recent literature concerning slums (Neekhra Citation2008, Beall and Fox Citation2009, Gulyani and Bassett Citation2010, Arimah Citation2011). There are, however, two important caveats: (1) the definition permits considerable variability in how unsafe a household may be, as UN-Habitat (2006) itself acknowledges; and (2) it is a household-based definition, not a place-based measure. It is this latter issue with which we are particularly concerned in this article.
Despite the recognition that households may vary considerably in their slum characteristics, there is a nearly universal tendency in the literature to uncritically assume that spatial autocorrelation exists with respect to housing unit quality or safety (i.e., all houses in a given area will be similar). The assumption is reflected in methodologies that categorize places by creating a dichotomous division of ‘slum’ versus ‘not slum’. This is a problematic dichotomy that hides the physical and social diversity of slums and makes it extremely difficult to understand why slums form, what their particular vulnerabilities are, and how best to introduce mitigation strategies. For example, Kundu (Citation2007) examined the differences between periphery and urban center slums in Delhi and found large disparities of local mobilization and political action, which were high in the center compared with the periphery. The historical processes that allowed city center slum dwellers to establish a foothold in local politics made their mitigation outlooks very different from their peripheral neighbors, a factor that would not be considered in a simple slum/not-slum dichotomy, but one that will make a difference when planning and/or implementing upgrades or infrastructural projects.
We find only two approaches in the literature for enumerating and measuring slums as continua rather than dichotomies: (1) that developed by Weeks et al. (Citation2007); and (2) that of Gulyani and Bassett (Citation2010). Weeks et al. (Citation2007) recognize that there may be some relatively arbitrary threshold beyond which no neighborhoods would be considered a slum, but among those identified as slums we can expect considerable variability, especially in cities of developing nations where a high proportion of people are living in neighborhoods nominally identified as slums (UN-Habitat Citation2010). The Weeks et al. (Citation2007) methodology revolves around the UN-Habitat's definition of slum, as described above. Yet rather than defining households as slum/not-slum, they first sum the number of slum conditions for each housing unit in a defined area and then calculate the average score for each area neighborhood, thus locating each place along a slum continuum. Gulyani and Bassett (Citation2010), on the other hand, introduce a ‘Living Conditions Diamond’, in which slum characteristics are distilled to four dimensions: tenure, infrastructure, unit quality, and neighborhood location. Slums can be placed within the diamond depending on their particular problems within the four domains, thus emphasizing differences in slums within a city or area. This framework provides a way of visualizing key differences among slums, although it does not provide an overall index of variability that can be used in a statistical analysis. Of particular importance, however, is that one of the domains is location. Thus, Gulyani and Bassett explicitly (but only qualitatively) bring a spatial element into the discussion of slums in a way that the quantitative method of Weeks and associates does not. People living in slums may differ in their vulnerability precisely because of where the slum is located, even if other conditions are similar. An important objective in this article is thus to quantify the spatiality of the Weeks and associates' method in order to test the idea that the variability in slumness and population vulnerability has a strong spatial component.
Turner et al. (Citation2003) define vulnerability as the degree to which a system (a person, household, neighborhood, or region) is likely to experience damage from exposure to a hazard or stressor, where a hazard is a single event and a stressor continues over time. Poverty and vulnerability are intimately tied together, yet there has been a recent shift in development discourse to focus on vulnerability as opposed to poverty, with the understanding that poverty is a component of vulnerability (Wratten Citation1995, Moser Citation1998, Beall and Fox Citation2009). The vulnerability literature reflects this shift, with a push to incorporate multiple aspects of vulnerability rather than examining only one tenet (such as physical or social) so that the complexity of vulnerability can be represented and understood (O'Brien et al. Citation2004b, Adger Citation2006).
From the literature, we can acknowledge that physical and social characteristics, demographic dynamics, and health all work together to make a place more or less vulnerable. Yet if the social and the physical framework of slums can vary drastically throughout a city, the vulnerability traditionally so closely tied to slums may also vary. In the Indian city of Chandigarh, for example, an estimated 231,415 people (20% of the population) live in slums. Rao and Thakur (Citation2007) surveyed these residents and found 15.5% of the dwellers to be living in high vulnerable areas, 44% living in medium vulnerable areas, and 40.5% living in low vulnerable areas. While this study did not look into the spatial distributions of the slums, nor did it examine hazards in the area, it does provide a glimpse at the potential variability of vulnerability within slums. Furthermore, slums might have unique mitigation and adaptation mechanisms to deal with vulnerability, thus producing variability in the resilience with which a neighborhood can recover from a disaster. In other words, slums are likely to be different from place to place even within a city, necessitating an analysis of vulnerability that takes this variation into account. A place-based approach can offer spatially specific insights while maintaining ties to the larger body of vulnerability knowledge. Rashed et al. (Citation2007) boil urban vulnerability down to one word, ‘particularity’, emphasizing the complex social, ecological, physical, and spatial dynamics that require vulnerability to be assessed in relation to a specific spatiotemporal context.
Cutter's (Citation1996) Hazards-of-Place Model of Vulnerability emphasizes place as an important avenue of research for vulnerability (Cutter et al. Citation2003). It integrates biological, physical, temporal, social, and spatial facets of vulnerability into one model and offers a framework for understanding the complex dynamics of urban areas in developing countries. Place-based vulnerability theory recognizes that while global measures and a unifying theory of vulnerability are important to keep the field cohesive, vulnerability drastically varies depending on scale and location (Turner et al. Citation2003). emphasizes three key aspects of the Cutter model: (1) physical hazards and stressors; (2) socioeconomic factors; and (3) demographics. A fourth aspect of vulnerability that is typically not considered, but is imperative to understanding vulnerability in the urban developing world, is health.
Table 1. Vulnerability variables, means, and standard deviations for n = 1717 enumeration areas (EAs)
Health may be thought of both as an outcome of vulnerability and as a cause of it. Those who are most vulnerable to hazards and stressors are likely to be people who have poorer access to health resources and facilities, which may then lead to lower-than-average health outcomes. At the same time, poor health will inevitably diminish a person's resilience. In the aggregate, we can expect that poor health in a neighborhood will impede recovery and redevelopment efforts in the face of a variety of hazards and stressors (López-Casasnovas et al. Citation2005). In the urban developing world, not being healthy can translate into an immediate loss of income, reduced job security, a burden on family members, and often-unaffordable health costs. This can quickly turn into a vicious cycle leading to even poorer health and lower economic outlooks, as well as higher vulnerability.
To our knowledge, an analysis of multiple aspects of vulnerability for slums in a developing world city has not been completed. Mapping vulnerability is primarily limited to the global, national, or regional scale (Trujillo et al. Citation2000, O'Brien et al. Citation2004a, Brooks et al. Citation2005), and we seek to fill a gap in the literature by providing a local and nuanced spatial exploration of vulnerability in Accra. We utilize GWR (Fotheringham et al. Citation2002) for spatial modeling of slum vulnerability. Based on the understanding that slums vary within a city, and some of this variation is accounted for by the location within the city, GWR can better uncover the particularities of slums as vulnerable places within. Global averages of spatial data are not always helpful, and GWR is a regression method that accounts for spatial non-stationarity by allowing coefficients to vary geographically (Comber et al. Citation2011). The method provides a novel examination of slums by examining the spatial variability of hazard, environment, social, demographic, and health vulnerability with slums throughout Accra.
3. Study site
The study site is Accra, the capital city of Ghana. It is a coastal city facing the Gulf of Guinea in western Africa. We draw from fieldwork, census data, and remotely sensed satellite imagery to create a comprehensive spatial understanding of vulnerability in Accra. This study focuses on the Accra Metropolitan Area (), which according to the 2000 census comprised 1.6 million people and 365,550 households dispersed over 1722 census enumeration areas (EAs), the unit of data analysis in this study. EAs are used as the finest spatial unit (household data do not include spatial coordinates) so that future analysis and policy makers may aggregate up to various neighborhood definitions. We provide one neighborhood boundary set throughout this article for ease of visualization, which we call the field-modified vernacular (FMV) neighborhoods, based on boundaries originally defined by Ghana Statistical Service, but adjusted through field observation. We utilize a 10% anonymized random sample of the individual- and household-level 2000 census for EAs in Accra, provided by Ghana Statistical Service, although one EA, the Korle Bu Teaching Hospital, was removed from analysis as an outlier.
Figure 1. Administrative and field-modified vernacular (FMV) neighborhood boundaries colored with slum index measures by enumeration area (EA).
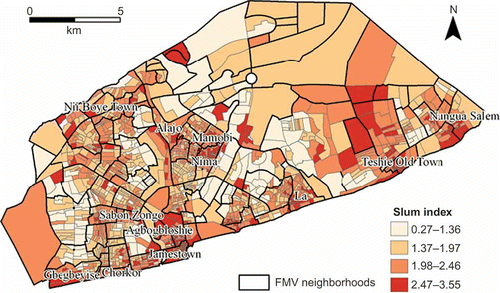
Accra is urbanizing at a quick rate and is projected to reach 3.5 million people by 2025 (United Nations Population Division Citation2010). The rapid population growth is mirrored by substantial changes in land use, as demonstrated by a study of remotely sensed imagery of Accra from 1985 to 2003 (Otoo et al. Citation2006), which revealed a 35% conversion of non-urban to urban areas in the region. The intense urban expansion coupled with a lack of infrastructural services from the government (Larbi Citation1996, Briggs and Yeboah Citation2001) has resulted in a large expansion of slum areas throughout Accra. We will analyze a more recent set of satellite imagery for this article, as noted below.
4. Data and methods
4.1. Defining the slum continuum
The slum index for each EA in Accra is calculated from data collected in the 2000 Census of Population and Housing, which asked questions that relate specifically to at least the first four of the UN-Habitat's five slum criteria. The fifth, which refers to the legal security of tenure, is more difficult to infer from census data. Each housing unit in our 10% anonymized sample was scored as follows:
-
If the housing unit does not have piped water, then slum1 = 1 (else 0).
-
If there is no toilet and no sewage connection, slum2 = 1 (else 0).
-
If the number of persons per room is greater than 2, slum3 = 1 (else 0).
-
If the building material is less durable, slum4 = 1 (else 0).
-
If the resident is not the owner, slum5 = 1 (else 0).
-
Slum index for each housing unit (Sh ) = Σ (slum1 … slum5).
For all housing units in Accra, the mean slum index was 2.43, with a standard deviation of 1.1. The minimum was 0 – no slum characteristics – and only 6.5% of homes in Accra had that score. The maximum score of 5 was shared by 0.7%. The median was 3 slum characteristics per housing unit, with those three most often being lack of piped water, no toilet connected to the sewer, and three or more people per room. Overall, the majority of homes in Accra do not have piped water, they do not have a toilet that is connected to the sewer, and the majority of units are not owned by a resident. Nearly half have three or more persons per room, but only a handful of the housing units have non-durable roofing and outer walls.
Once the slum index had been calculated for each housing unit, it was then indexed for each EA as the mean value for all housing units in that EA:
From these calculations, we can see in that slums are nearly ubiquitous in Accra, as in most sub-Saharan African cities, but there is considerable variability and a strong spatial pattern, evidenced by a global Moran's I of 0.33, which is statistically significant well beyond the 0.001 level.
4.2. Defining vulnerability
Variables to be used as measures of vulnerability were drawn from the Hazards-of-Place Model of Vulnerability (Cutter Citation1996), as well as fieldwork and literature concerning Accra (). Physical hazards and stressors focus on elevation and environmental degradation. Accra's open drain system combined with the naturally occurring waterways in the city (the largest of which are Odaw River and Korle Lagoon), consistent blocking of drainage with trash and debris, and overall low elevation puts the city at high risk for yearly flooding (Ludlow Citation2009, Rain et al. Citation2011). Residents living in the lowest elevations of the city are at high risk and are vulnerable not only to flooding, but to vector-borne diseases and the life-disrupting consequences that come with them. Flooding is arguably the most pressing environmental hazard for the inhabitants of Accra. Elevation was derived from 140 tiles of vector data provided by the Ghana Department of Lands and Surveys. Tiles were extracted from ArcInfo to coverage format, merged, and projected into one large vector dataset with elevation contours in ArcGIS 9.2. Topographic data were then converted to a digital elevation model (DEM) using the ‘Topo to Raster’ tool, which utilizes a discretized thin plate spline interpolation technique (ArcGIS Citation2007), clipped to the Accra Metropolitan Area shapefile, and aggregated to an EA average.
In order to assess vulnerability to environmental stressors, three variables were created: disposal of solid waste in a manner other than being collected by waste disposal services; cooking in the open air over charcoal, wood, or coconut husks; and a remotely sensed estimate of vegetation. Air pollution levels measured over time in Nima and Jamestown were found to be higher than developed countries as well as countries in Latin America, due especially to the burning of biomass for cooking and other household tasks (Arku Citation2008, Dionisio et al. Citation2010). Strong correlations have been consistently found between environmental problems in Accra such as low air quality, unsanitary waste disposal, polluted water, diarrhea and respiratory diseases among children, and poor health of women (Songsore and McGranahan Citation1993, Boadi and Kuitunen Citation2005, Songsore Citation2005). Lack of vegetation may be a proxy for multiple vulnerabilities including overcrowding (removal of any vegetation in order to increase building density), flooding (less ability for soils to take up water), and lack of sustainability (removal of sustainable fuel, shade, and a way of cleaning the air) (National Research Council Citation2007).
Solid waste not collected by sanitary crews and cooking outdoors over charcoal, wood, or coconut were aggregated as a percentage of sample households in the EA. The percent of vegetation for each EA was calculated based on Ridd's vegetation-impervious surface-soil (VIS) model (Ridd Citation1995) from a combination of QuickBird and Advanced Spaceborne Thermal Emission and Reflection Radiometer (ASTER) imagery of Accra in 2002 and 2001, respectively. The high spatial resolution QuickBird image (2.4 meters in multispectral, 0.6 meters in panchromatic) was the primary data source used, as it covered most of the study area. Some EAs in the eastern part of the city not included in the QuickBird image were filled in with ASTER imagery (Stoler et al. forthcoming). While ASTER imagery has a lower spatial resolution at 15 meters, it still allows for fairly detailed VIS model estimates.
Often cited as the missing tenet in vulnerability research, the social aspect is an imperative tool in understanding how and why systems are vulnerable (Cutter Citation1996). Cutter et al. (Citation2003) provide a list of potential social and demographic vulnerability variables taken from the literature. Demographic and health characteristics of a population fall under this social vulnerability umbrella but are rarely incorporated into vulnerability studies. Our study drew extensively from this list, as well as from fieldwork and knowledge of Accra. Poverty and wealth are the most commonly utilized measures of social vulnerability (Rashed et al. Citation2007). Literacy/education is another important aspect of social vulnerability, albeit closely related to poverty/wealth. Dichotomous variables of literacy, individuals working in high-status occupation jobs, and individuals working in the informal sector were aggregated as a percent of the EA and used as proxies to model poverty and education.
Belonging to a minority group may increase vulnerability, particularly when the group is marginalized or spatially segregated. Accra has a relatively high degree of ethnic mixing within its various neighborhoods (Agyei-Mensah and Owusu Citation2009) with the notable exception of the Ga ethnic group, which is highly concentrated in Accra's coastal neighborhoods. Unequal distribution of public services in these neighborhoods may increase vulnerability, although Weeks et al. (Citation2006) demonstrate that increased vulnerability due to being an ethnic minority goes beyond spatial segregation and impacts outcomes like child mortality regardless of where an individual of Ga ethnicity resides. Therefore, percent Ga ethnicity was calculated for each EA.
Vulnerability tied specifically to demographic characteristics or health is rarely considered in the literature, even though the number of young children in a neighborhood, fertility levels, dependency ratio, and migration can all contribute to a household and neighborhood's ability to reduce vulnerability. Furthermore, health operates in a circular pattern where it can be a direct result and cause of vulnerability. The factors that cause child mortality, for example, are likely operating on a number of household and neighborhood levels to increase vulnerability and reduce resilience of infants and young children. We include demographic and health variables in our analysis to attempt to account for these factors of vulnerability. Percent children under 14 and percent of recent migrants (defined as having migrated into Accra in the 5 years preceding the census) were calculated at the EA level.
Fertility was calculated as an EA z-score. For each woman in the census we calculated the number of children ever born in standard deviation units relative to all women in Accra in her 5-year age group. Thus, a woman aged 25–29 who had given birth to more children than the average for her age would have a positive z-score, whereas those with fewer than the average would have a negative z-score. These z-scores were then averaged for each EA to produce a relative measure of fertility in that EA compared with other EAs. Because children in Ghana begin working and contributing to the household at a very young age, the dependency ratio was calculated to include children under 5 rather than children under 14. While children under 14 are more vulnerable to a number of factors than adults, they are often not entirely dependent on adults. Additionally, rather than include only adults over the age of 65, the threshold was lowered to 55, to account for lower life expectancy and poorer health in Ghana. Finally, we utilized a measure of child mortality derived as EA z-scores, representing the proportion of a woman's children who have died relative to what we would expect for a woman her age with her number of live births in Accra. Thus, each woman's score represents her standard distance from the average for all women in Accra of her age and parity. This produces a variable at the individual level, which we aggregated to an average for each EA.
Principal components analysis (PCA) was run using varimax rotation selecting eigenvalues over 1.0 for all variables at the EA level in Although groups variables according to differing aspects of vulnerability, we included all variables in the PCA at the same time in order to control for multicollinearity among variables, regardless of conceptual grouping. The PCA of vulnerability variables produced four principal components of eigenvalues greater than 1, explaining 60.94% of the total variance (). These components replace the conceptual groupings shown in and reveal that the variables do not line themselves up in exactly the way predicted by those conceptual groupings. The first component, ‘SES–Env’, is illustrative of the intermingling effects of social processes and the resulting physical stressors. People of lower socioeconomic standing in Accra often cannot afford or do not live in areas with infrastructure for clean cooking fuels, indoor kitchens, and payment for trash collection. At the neighborhood level, places with lower socioeconomic standing are characterized by crowding, where vegetation must be removed to make room for housing and commercial interests. Fertility loads highly on this component indicating that higher rates of fertility are more closely tied to environmental behaviors and socioeconomic status, rather than other demographic characteristics like migration and household size. This component (SES–Env) is an illustration of the complicated relationship between humans and environment that persists in developing world cities.
Table 2. PCA components, eigenvalues, percent explained variance, and high loading variables
The second component, ‘elevation/Ga’, includes Ga ethnicity and elevation, which is an indicator of flooding hazards. As the main livelihood of the Ga is fishing, most EAs with a high prevalence of Ga are found in areas of low elevation on the coastline. The loading on this component underscores the multiple vulnerabilities that individuals of Ga ethnicity face in Accra, not only from social segregation, but also from the persistent physical hazard of flooding. Component three, ‘age structure’, loaded highly on percent of children under 14 and the <5 and 55 + dependency ratio. Child mortality and migration variables loaded onto the fourth component that we call ‘health’. Previous research has indicated that significant factors for child health in Accra revolve around poor sanitation, crowding, smoky kitchens, and pests (Songsore and McGranahan Citation1993), and therefore it is surprising that child mortality was not incorporated into the first component. The loading of migration and childhood mortality variables in the ‘health’ component illustrates that child mortality is a complicated part of vulnerability that may be tied to recent migration into Accra.
4.3. Overview of analytical procedures
We first gain an overview of spatial patterns by calculating Moran's I at the EA level for the slum index and for each vulnerability component. Then, in order to test how the classification of a place on the slum index can predict the level of vulnerability, the slum index at the EA level was used as the independent variable for a series of four ordinary least squares (OLS) regression models where the dependent variables were each of the vulnerability measures resulting from the PCA analysis, as described above. Each of these models was then tested for the size and nature of spatial dependence in the residuals using the OpenGeoDa software (Anselin Citation2003) focusing on the robust Lagrange multiplier (LM) lag and error measures. A spatial regression model was chosen for each vulnerability measure based on LM values. Diagnostics were run for the presence of spatial heterogeneity, which led us to model the data using GWR.
We utilize the ArcMap 10.0 GWR tool, which outputs global and local R 2 values, local parameter estimates, standard errors, and standardized residual values (documentation, parameters options, and code sample can be found at http://help.arcgis.com/en/arcgisdesktop/10.0/help/index.html). However, the tool within this software does not give certain calculations such as bandwidth range. Additionally, the tool does not provide global parameter estimates or statistical significance measures (Charlton et al. Citation2005). However, we are interested in local variation in parameter estimates rather than global estimates. GWR is able to calculate local measures based on weighted neighborhood values. Using different weighting functions can impact the model; however, this impact is often small (Brunsdon et al. Citation1998). For our purposes, the tool was allowed to create its own weights matrix for Accra's EAs. Due to the varying size and density of EAs in Accra, the adaptive kernel option, which utilizes Gaussian kernels, was selected in the GWR tool. An Akaike Information Criterion (AIC) bandwidth was applied for both models, which allows the tool to find the optimal distance for the varying kernel throughout the calculation. In the final stage of the analysis, quintile analysis was used to determine population percentages of the most vulnerable people living in the worst slums.
5. Results
5.1. Spatial variability of vulnerability in Accra
The spatial variability of the factor scores of the four vulnerability components are mapped in , with an indication of the global Moran's I for each map. All measures were statistically significantly clustered (P < 0.0001), indicating the existence of a spatial pattern to the different vulnerabilities in the city. SES–Env vulnerability is highlighted in Nima, Mamobi, Agbogbloshie, Teshie Old Town, Chorkor, and Jamestown – the well-known slums in the city, as assessed through the literature and our fieldwork. Elevation/Ga follows a pattern of higher vulnerability near the coastline, lagoon, and communities with a high prevalence of Ga. Demographics has a less distinguishable spatial pattern, but it is noteworthy that many of the established slums do not show high values of the component. Health vulnerability is the least spatially clustered component according to Moran's I value. Yet, shows multiple FMV neighborhoods that contain several EAs with a health component score that is one standard deviation or more above the mean, indicating higher vulnerability.
Figure 2. The factor scores of the four components of vulnerability with corresponding Moran's I values: (a) I = 0.172; (b) I = 0.513; (c) I = 0.128; (d) I = 0.029. One or more standard deviations above the mean are mapped in red, and one or more standard deviations below the mean are mapped in blue. Available in colour online.
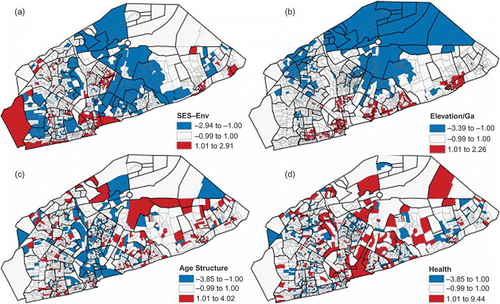
emphasizes that different places in the city are vulnerable in diverse ways. It is also useful to visualize the interplay of different vulnerabilities in Accra. illustrates the compounding effects of vulnerabilities, as well as the presence of dominant vulnerabilities in various neighborhoods. highlights the most vulnerable places in Accra by layering EAs that belong to the third and fourth quartile of each vulnerability measure, or high vulnerability. A score of 4 indicates an EA where each vulnerability factor score was high, while a score of 0 shows low scores for all vulnerability components. This map demonstrates the precarious situation of many of the coastal communities in Accra, particularly Teshie Old Town, La, Jamestown, and Chorkor, which are dealing with multiple aspects of vulnerability at the same time. However, the map also shows that much of the city, including most EAs in the well-known slums of Nima and Mamobi, are vulnerable to one or two measures at most, indicating the need for targeted mitigation strategies that will address those particular vulnerabilities.
Figure 3. (a) Total number of high factor score vulnerability components for each EA. (b) Dominant vulnerability of each EA.
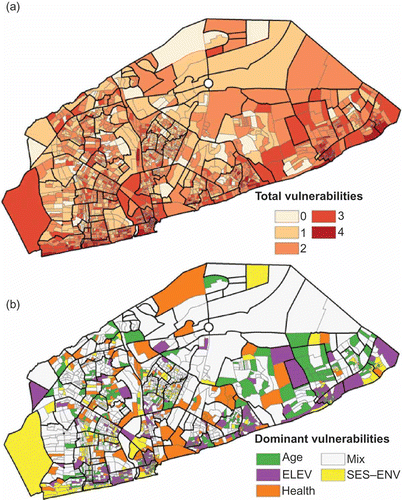
is a dominant classification visualization that highlights spatial codistribution of attribute values compared with each other (Andrienko and Andrienko Citation2001). The attribute values in this map are derived from a parallel coordinates plot, which normalizes the measures against each other and for each EA delineates if a particular measure has a ‘dominant’ value when compared with the other measures. The visualization allows for the exploration of the most pressing vulnerabilities in particular neighborhoods. Many dominant clusters appear along the coastline, although ‘elevation/Ga’ is not always the dominant vulnerability. For example, La has EAs with SES–Env, age, and health vulnerabilities mixed in with the dominant elevation/Ga component. Jamestown demonstrates an even more eclectic mix of vulnerabilities within the EAs that compose it. However, it is important to note that the EAs do not come up as ‘mixed’ in the classification, indicating that EAs within the neighborhood are exhibiting clearly dominant vulnerabilities compared with other EAs. This would indicate that Jamestown – often considered one large slum – is a highly complex place with specific vulnerabilities varying within the neighborhood itself. Nima and Alajo exhibit SES–Env, age, and health vulnerabilities, indicating complexities of health-based vulnerability that go beyond socioeconomic status and environmental health hazards. Based on these visualizations, we begin to see that places in Accra are exhibiting varying levels and types of vulnerabilities. Does the slum index predict these patterns of vulnerability? To explore this further, we turn to regression analysis of slums and vulnerability in Accra.
5.2. Does the slum index predict vulnerability?
Overall, we found that in an OLS regression the slum index significantly predicted all but the health vulnerability measure (). However, the standardized residuals for all models were spatially clustered, based on the highly significant Moran's I values of residuals (). This suggests a violation of the OLS model assumptions and indicates that the relationship between vulnerability and slums might be better modeled by accounting for the spatial component of vulnerability in Accra. Robust LM error measures were larger than lag measures for all models with the exception of the health model where both measures were insignificant.
Table 3. Ordinary least squares (OLS) regression, residual Moran's I, Lagrange multiplier, spatial error model, and geographically weighted regression (GWR) results for four models with vulnerability measures as dependent variables
A spatial error model was run for the SES–Env, elevation, and age structure variables. In the baseline OLS model, the slum index was best able to predict the SES–Env measure, where each unit increase of the slum index results in a magnitude 1.308 increase in the SES vulnerability component measure. When accounting for spatial error, R 2 is slightly improved from 0.500 to 0.586, while the predictive power of the slum index decreased to 1.220, but remained highly significant and larger than the spatial weight (lambda). The results suggest that the slum index is capturing most of the spatial autocorrelation that exists among EAs with respect to SES–Env vulnerability, or in other words slums are clustered similarly to SES–Env vulnerability. However, diagnostics for spatial heterogeneity/non-stationarity suggested that this was an additional spatial aspect to the relationships that needed to be taken into account. This refers to the phenomenon of spatially varying coefficients, a situation that we accounted for with the GWR model. The jump in the R 2 value for the GWR model to 0.74 for the slum index predicting the SES–Env vulnerability component signals the presence of spatial non-stationarity. The size of this relationship varies from one part of the city to another, as shown in
The association between slums and vulnerability driven by SES–environment interactions is to be expected. These results indicate that the slum index of an area may be a good proxy for predicting vulnerability tied to poverty, low social standing, and environmental degradation. However, it is important to note that mapped standardized residuals of the OLS and GWR (not shown) demonstrated high underestimation of SES–Env vulnerability in some slums including Nima, Mamobi, Jamestown, and Agbogbloshie. This result is reflected in the local R 2 values from GWR (). Agbogbloshie, La, Nii Boye Town, Alajo, and part of Jamestown show R 2 values close to 0, as do parts of Nima and Mamobi. Yet parts of Jamestown and Nima also demonstrate high R 2 values indicating considerable variability within slum neighborhoods, especially the more established slums near the center of the city. In contrast, Nungua Salam and Gbegbeyise, on the outskirts of the city, have high R 2 values.
As a predominantly low-rise city with poor infrastructure swells with population, older slums may be much closer to jobs than newer areas that are emerging at the urban fringe – a pattern typical of other developing cities (Ford Citation1993). This may be encouraging residents to stay put, even if they could otherwise afford to move to a better, but spatially less convenient neighborhood – a pattern demonstrated in Accra (Agyei-Mensah and Owusu Citation2009). In sub-Saharan Africa, ties of ethnicity and tribalism further propel individuals and families to stay in slum areas when their social or economic status would predict a different location for housing.
Nima, for example, is one of the most notoriously famous slums of Accra, yet a visitor to this neighborhood is greeted not only by slum-like dwellings, but also by a number of large, elaborately built and finely maintained households belonging to tribal leaders, businessmen, doctors, lawyers, and other financially successful individuals. In their in-depth study of Nima, Owusu et al. (Citation2008) showed the economic and livelihood diversity of individuals living there; however, stigmatization from the rest of the city depicting Nima as a community of criminals and poor people leads to marginalization. In their conclusions, Owusu et al. (Citation2008) argue that the pervasive perception of slums as homogenously poor places has actively prevented researchers from comparing the livelihood, socioeconomic, and cultural differences within slum communities. This criticism can be extended to different slums within a city. Such spatially varying differences may be key to not only better understanding vulnerabilities, but also using resources already present in slums to develop better coping strategies.
Elevation/Ga is a significantly spatially autocorrelated measure, and the regression models perform significantly better when accounting for spatial error and spatial non-stationarity. The spatial error model demonstrates that the worst slums are not necessarily found in areas with high elevation/Ga vulnerability. The global GWR R 2 is very high – the slum index is highly predictive of this component of vulnerability when spatial patterns are accounted for. The GWR local R 2 values show a stronger relationship between the slum index and elevation through a corridor of neighborhoods in the east of the city and in the central downtown area. Surprisingly, the slum index has very low local R 2 values along the western coastal areas, including Jamestown and Chorkor. The map illustrates that while the slum index may perform well as a general indicator of the elevation/Ga component, it is not able to consistently predict this vulnerability.
The slum index was not able to predict age structure vulnerability well in the baseline OLS model, but prediction improved when accounting for spatial error and spatial non-stationarity. The lambda coefficient in the spatial error model () is larger than the slum index coefficient, indicating that spatial autocorrelation of the slum index does not directly track the pattern of age structure clustering. This is apparent in the GWR local R 2 values, where areas with higher slum indices show low R 2 values for the age structure vulnerability outcome.
The slum index performed least well for the health measure and was not able to significantly predict health-driven vulnerability in the OLS model. LM lag and error tests were insignificant, thus the spatial models were not implemented. GWR results, however, do show some improvement in the R 2 value when accounting for spatial non-stationarity. Like all of the other vulnerability components, this one is spatially varying in its relationship to the slum index. Health vulnerability is clearly an issue throughout the city, and an area's status as a slum is only weakly able to predict this vulnerability's presence in the neighborhood. One possible hypothesis to explain the poor ability of the slum index to predict the health vulnerability component is the increasing number of NGO and government-led health clinics in slum communities assisting in preventing child mortality. While keeping in mind that the GWR health model is still a relatively poor fit, there are nonetheless pockets in the city where the slum index shows higher R 2 values for predicting health vulnerability, including downtown Accra, part of Nima, and Agbogbloshie.
The slum index is shown to have considerable variability in predicting the four vulnerability measures both between measures and within measures. provides an aggregated view of the spatial heterogeneity of the slum index's predictive ability. The 25th and 75th percentiles of the GWR slum index coefficient values for the four models were calculated to determine where the slum index had the smallest and largest positive association with each of the vulnerability measures. Co-occurrence was calculated for each EA by adding up the number of small and large predictive events, respectively, where 0 is the minimum (the EA falls outside of the 25th or 75th percentile for all four models) and 4 is the maximum (the EA belongs to the 25th or 75th percentile for all four models) for both calculations. The co-occurrence values indicate where the slum index is consistently poorly () and strongly () associated with vulnerability.
Figure 5. Co-occurrence of (a) the 25th and (b) the 75th percentiles of GWR slum index coefficient values for the four vulnerability models.
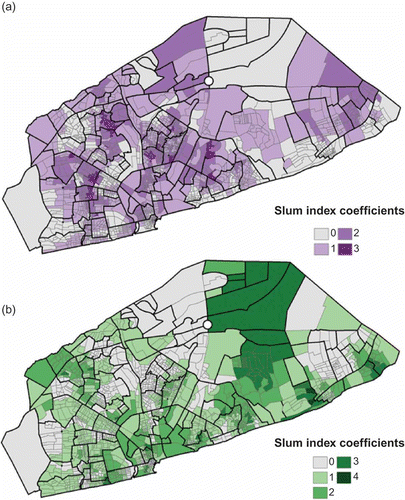
Notably, the slum index for Accra's most well-known slums do not show consistent strong positive association with the vulnerability scores. The only areas of the city where the slum index is consistently strongly associated with vulnerability are in the north, an industrial area, and some small pockets in the coastal east that are relatively recent slum neighborhoods. Most slum neighborhoods show 75th percentile co-occurrences of one or two. These neighborhoods have similar 25th percentile values, indicating that the slum index has high positive associations in slum neighborhoods with some vulnerabilities, but low associations with others. Nima and Mamobi have more co-occurrence in the 25th percentile than the 75th percentile, indicating that the slum index is not predicting vulnerability for these neighborhoods in the ‘expected’ way. However, based on Nima and Mamobi's history as some of the oldest slums in the city, these results lend support to the considerable variability of people and livelihoods within the slums making it very difficult to predict vulnerabilities in such places.
5.3. Do the most vulnerable people live in the worst slums?
Rather than draw an arbitrary line between slum and non-slum, we have emphasized the variability in neighborhoods ranging from those with the lowest slum score to those with the highest slum index. It is useful for analytical purposes, however, to create some demarcations and we have done so by creating percentile values (see above and ). Somewhat arbitrarily, we define the fifth quintile as representing the worst slums (with an average slum index of 2.74), encompassing 304,316 people – 18% of Accra's population. At the same time, there are 338,927 people living in the fifth (most vulnerable) quintile with respect to SES vulnerability. Of those people, 163,996 (48%) are also living in EAs that are in the fifth quintile with respect to slums. Thus, nearly half of those who are most vulnerable in terms of their socioeconomic status are living in the worst slums, which is well above the 20% that we would expect by chance alone (based on approximately 20% of the population living in each quintile of EAs).
Turning to vulnerability according to elevation/Ga ethnicity, there are 234,595 people living in the EAs in the fifth quintile of this component. Of these, 83,973 (36%) are living in EAs in the fifth quintile of the slum index. This too is well above the level expected by chance. The third component related to age/migration includes 304,118 people in the fifth quintile of vulnerability. Of these, 85,458 (28%) also live in the fifth quintile with respect to the slum index, which is still greater than expected by chance. The fourth component related to health (especially child mortality) includes 306,640 people in the fifth quintile. Of these, 71,540 (23%) live in EAs that are in the fifth quintile in terms of the slum index. This is just slightly more than would be expected by chance.
There are 15 EAs which rank in the fifth quintile on all four of the components. They are scattered around the city, and there are 10,842 people living in these EAs. Twelve of these EAs are in the fifth quintile with respect to the slum index, encompassing 8854 people or 82% of the most vulnerable. So, by that criterion, almost all of the clearly most vulnerable people in Accra do indeed live in the worst slums. Backing up a step, there are 49,566 people living in EAs that are in the fifth quintile for three of the four components of vulnerability, and 33,407 of them (67%) live in the worst slums. Thus, by this more liberal criterion, we can still conclude that the most vulnerable people in Accra are likely to be living in the worst slums. One step further back, there are 249,044 (15% of Accra's population) living in EAs in the fifth quintile of at least two components of vulnerability. Of these, 112,991 (45%) live in the worst slums. So it is reasonable to conclude that even with a generous definition of who is vulnerable, the probability is very high that such people will be living in the worst slums.
6. Conclusion
Our broadest conclusion from this research is clearly that one size does not fit all when it comes to the relationship between slums and vulnerability. The assumption of uniform vulnerability that is so often made about slums is a complex and multifaceted narrative. In Accra, even a simple statement – ‘slum neighborhoods are more vulnerable than non-slum neighborhoods’ – quickly falls apart when we consider what exactly constitutes a slum. If we were to use the dichotomous definition of the UN, almost all of Accra could be considered a slum. Is most of Accra equally vulnerable? To this question we can answer a resounding ‘no’ based on our analysis. No two slums are necessarily the same, and this variability should be accounted for.
An important objective of this article was to quantify the spatiality of the Weeks et al (Citation2007) slum index in order to test the idea that the variability in slumness and population vulnerability has a strong spatial component. Of singular importance from our results is the fact that location does indeed matter. In Accra, as almost certainly in all developing country cities, slums are scattered around the city rather than being concentrated in one specific area. This turns out to be an important factor in the relationship between slums and vulnerability because that relationship varies considerably across space, as demonstrated by the results from the GWR. The slumness of an EA is a better predictor of vulnerability in some parts of the city than in others. Furthermore, the slum index is better able to predict some kinds of vulnerability than other kinds, and the spatial patterns of the relationship between slumness and vulnerability are not the same for the four vulnerability components that we measured in this analysis.
Although complexity is a key theme in our findings, our overall goal was to evaluate whether the most vulnerable people in Accra live in the worst slums. Yet, even here the answer is complex because it depends upon which aspect of vulnerability is under discussion. People living in EAs in which vulnerability relates to the presence of low socioeconomic status are most likely to be living in the worst slums. People in EAs in which the vulnerability relates to low elevation and/or high levels of Ga ethnicity are next most likely to be living in the worst slums, followed by people in EAs vulnerable to age structure distortions. However, people living in EAs in which vulnerability relates to above average child mortality are only slightly more likely than average to be living in the worst slums. When we put vulnerabilities together and ask the question about who is most vulnerable in terms of multiple components of vulnerability, it becomes clear that people living in EAs with two or more high levels of vulnerability are indeed likely to be in Accra's worst slums.
Vulnerability with respect to health did not emerge as exhibiting a strong spatial pattern, nor as having as strong a relationship to the slum index as did the other components of vulnerability. That does not mean, however, that there is not a coherent spatial pattern to child mortality. We know from another research (Weeks et al. forthcoming) that such a pattern does exist in Accra, but at a different spatial scale than employed in this article. Here we focused on EAs in order to evaluate the spatial variability within slum neighborhoods. Our results show that child mortality tends not to vary predictably at this finer spatial scale, even though it does exhibit spatial patterns at the scale of more broadly defined neighborhoods – a classic case of the modifiable areal unit problem.
Acknowledgements
This research was funded by grant number R01 HD054906 from the Eunice Kennedy Shriver National Institute of Child Health and Human Development (‘Health, Poverty and Place in Accra, Ghana’, John R. Weeks, Project Director/Principal Investigator). The content is solely the responsibility of the authors and does not necessarily represent the official views of the National Institute of Child Health and Human Development or the National Institutes of Health. An earlier version of this article was a finalist in the ‘Places We Live’ research competition sponsored by USAID, the International Housing Coalition (IHC), The World Bank, Cities Alliance, and the Woodrow Wilson Comparative Urban Studies Program (CUSP) in 2010.
Notes
References
- Adger , W.N . 2006 . Vulnerability . Global Environmental Change , 16 : 268 – 281 .
- Agyei-Mensah , S. and Owusu , G. 2009 . Segregated by neighborhoods? A portrait of ethnic diversity in the neighborhoods of the Accra Metropolitan Area, Ghana . Population, Space and Place , 16 ( 6 ) : 499 – 516 .
- Andrienko , G. and Andrienko , N. 2001 . Exploring spatial data with dominant attribute map and parallel coordinates . Computers, Environment and Urban Systems , 25 ( 1 ) : 5 – 15 .
- Anselin, L., 2003. GeoDa (Tools for exploratory spatial data analysis) [online]. http://geodacenter.asu.edu/software (http://geodacenter.asu.edu/software) (Accessed: 24 May 2011 ).
- ArcGIS., 2007. Hydrologically correct surfaces: Topo to Raster. ArcGIS 9.2 desktop help [online]. http://webhelp.esri.com/arcgisdesktop/9.2/index.cfm?TopicName=Hydrologically_correct_surfaces%3A_Topo_to_Raster (http://webhelp.esri.com/arcgisdesktop/9.2/index.cfm?TopicName=Hydrologically_correct_surfaces%3A_Topo_to_Raster) (Accessed: 24 August 2010 ).
- Arimah , B.C. 2011 . One of the many faces of urban poverty: explaining the prevalence of slums in African countries . Paper presented at Center for the Study of African Economics 25th Anniversary Conference 2011: Economic Development in Africa . March 20–22 2011 , Oxford, UK. CSAE .
- Arku , R.E. 2008 . Characterizing air pollution in two low-income neighborhoods in Accra, Ghana . Science of the Total Environment , 402 ( 2–3 ) : 217 – 231 .
- Beall , J. and Fox , S. 2009 . Cities and development , New York : Routledge .
- Boadi , K. and Kuitunen , M. 2005 . Environmental and health impacts of household solid waste handling and disposal practices in third world cities: the case of the Accra Metropolitan Area, Ghana . Journal of Environmental Health , 68 ( 4 ) : 32 – 36 .
- Briggs , J. and Yeboah , I.E.A. 2001 . Structural adjustment and the contemporary sub-Saharan African city . Area , 33 : 18 – 26 .
- Brooks , N. , Adger , N.W. and Kelly , P.M. 2005 . The determinants of vulnerability and adaptive capacity at the national level and the implications for adaptation . Global Environmental Change , 15 : 151 – 163 .
- Brunsdon , C. , Fotheringham , S. and Charlton , M. 1998 . Geographically weighted regression modelling spatial non-stationarity . The Statistician , 47 : 431 – 443 .
- Charlton, M., Fotheringham, S., and Brundson, C., 2005. Geographically weighted regression [online]. NCRM methods review papers. http://ncg.nuim.ie/ncg/GWR/index.htm (http://ncg.nuim.ie/ncg/GWR/index.htm)
- Comber, A.J., Brundson, C., and Radburn, R., 2011. A spatial analysis of variations in health access: linking geography, socio-economic status and access perceptions [online]. International Journal of Health Geographics, 10. http://www.ij-healthgeographics.com/content/10/1/44 (http://www.ij-healthgeographics.com/content/10/1/44) (Accessed: 24 June 2011 ).
- Cutter , S.L. 1996 . Vulnerability to environmental hazards . Progress in Human Geography , 20 : 529 – 539 .
- Cutter , S.L. , Boruff , B.J. and Shirley , W.L. 2003 . Social vulnerability to environmental hazards . Social Science Quarterly , 84 : 242 – 261 .
- Davis , M. 2006 . Planet of slums , New York : Verso .
- Dionisio , K.L. 2010 . Air pollution in Accra neighborhoods: spatial, socioeconomic, and temporal patterns . Environmental Science and Technology , 44 ( 7 ) : 2270 – 2276 .
- Ford , L.R. 1993 . A model of Indonesian city structure . Geographical Review , 83 ( 4 ) : 374 – 396 .
- Fotheringham , A.S. , Charlton , M. and Brundson , C. 2002 . Geographically weighted regression: the analysis of spatially varying relationships , Chichester : John Wiley & Sons .
- Gulyani , S. and Bassett , E.M. 2010 . The living conditions diamond: an analytical and theoretical framework for understanding slums . Environment and Planning A , 42 ( 9 ) : 2201 – 2219 .
- Kundu , A. 2007 . “ Dynamics of growth and process of degenerated peripheralization in Delhi: an analysis of socio-economic segmentation and differentiation in micro-environment ” . In Scaling urban environmental challenges: from local to global and back , Edited by: Marcotullio , P.J. and McGranaham , G. 156 – 178 . London : Earthscan .
- Larbi , W.O. 1996 . Spatial planning and urban fragmentation in Accra . Third World Planning Review , 18 ( 2 ) : 193 – 215 .
- López-Casasnovas , G. , Rivera , B. and Currais , L. , eds. 2005 . Health and economic growth: findings and implications , Cambridge, MA : MIT Press .
- Ludlow , C.D. 2009 . “ Flood modeling in a data-poor region: a satellite data-supported flood model for Accra, Ghana ” . In Thesis (MS) , The George Washington University .
- Milbert , I. 2006 . Slums, slum dwellers and multilevel governance . The European Journal of Development Research , 18 ( 2 ) : 299 – 318 .
- Moser , C.O.N. 1998 . The asset vulnerability framework: reassessing urban poverty reduction strategies . World Development , 26 ( 1 ) : 1 – 19 .
- National Research Council . 2007 . Tools and methods for estimating populations at risk , Washington, , DC : National Academies Press .
- Neekhra , V. 2008 . “ Growing vulnerability crisis ‘Will slums ever reduce or improve?’: a case from India ” . In Vulnerable cities: realities, innovations and strategies , Edited by: Kidokoro , T. , Okata , J. , Matsumara , S. and Shima , N. 77 – 103 . Hicom : Springer .
- O'Brien , K. 2004a . Mapping vulnerability to multiple stressors: climate change and globalization in India . Global Environmental Change , 14 : 303 – 313 .
- O'Brien , K. 2004b . What's in a word? Conflicting interpretations of vulnerability in climate change research , Vol. 4 , Oslo : Center for International Climate and Environmental Research, Working Paper 2004 .
- Otoo , E.A. , Whyatt , D.J. and Ite , U.E. 2006 . Quantifying urban growth in Accra Metropolitan Area (AMA), Ghana and exploring causal mechanisms . Paper presented at 5th FIG Regional Conference . March 8–11 2006 , Accra, Ghana.
- Owusu , G. , Agyei-Mensah , S. and Lund , R. 2008 . Slums of hope and slums of despair: mobility and livelihoods in Nima, Accra . Norsk Geografisk Tidsskrift-Norwegian Journal of Geography , 62 ( 3 ) : 180 – 190 .
- Pelling , M. 2003 . The vulnerability of cities: natural disasters and social resilience , London : Earthscan Publications Ltd .
- Rain , D. 2011 . “ Accra, Ghana: a city vulnerable to flooding and drought-induced migration ” . In Background paper for chapter 4 of cities and climate change 2011: global report on human settlements , Nairobi, , Kenya : UN-Habitat .
- Rao, B.T. and Thakur, J., 2007. Vulnerability assessment in slums of union territory, Chandigarh [online]. Indian Journal of Community Medicine, 32. http://www.indmedica.com/journals.php?journalid=7&issueid=101&articleid=1373&action=article (http://www.indmedica.com/journals.php?journalid=7&issueid=101&articleid=1373&action=article) (Accessed: 24 December 2010 ).
- Rashed , R . 2007 . “ An integrative GIS and remote sensing model for place-based urban vulnerability analysis ” . In The integration of RS and GIS , Edited by: Mesev , V. 199 – 231 . New York : John Wiley and Sons .
- Ridd , M.K. 1995 . Exploring a V-I-S (vegetation-impervious surface-soil) model for urban ecosystem analysis through remote sensing: comparative anatomy for cities . International Journal of Remote Sensing , 16 ( 12 ) : 2165 – 2185 .
- Songsore , J. 2005 . State of environmental health report of the Greater Accra Metropolitan Area (GAMA), Ghana , Stockholm : Stockholm Environment Institute .
- Songsore , J. and McGranahan , G. 1993 . Environment, wealth and health: towards an analysis of intra-urban differentials within the Greater Accra Metropolitan Area, Ghana . Environment and Urbanization , 5 : 10 – 34 .
- Stoler , J. forthcoming . Assessing the utility of satellite imagery with differing spatial resolutions for deriving proxy measures of slum presence in Accra, Ghana . GIScience & Remote Sensing ,
- Trujillo , M. , Ordonez , A. and Hernandez , C. 2000 . Risk mapping and local capacities: lessons from Mexico and Central America , London : Oxfam GB .
- Turner , B.L. 2003 . A framework for vulnerability analysis in sustainability science . Proceedings of the National Academy of Sciences , 100 : 8074 – 8079 .
- United Nations Human Settlements Program (UN-Habitat) . 2003 . The challenge of slums: global report on human settlements 2003 , Sterling, , VA : Earthscan Publications Ltd .
- United Nations Human Settlements Program (UN-Habitat) . 2006 . State of the world's cities: 2006/7 , New York : United Nations .
- United Nations Human Settlements Program (UN-Habitat) . 2008 . State of the world's cities: 2008/2009: harmonious cities , London : Earthscan .
- United Nations Human Settlements Program (UN-Habitat) . 2010 . State of the world's cities 2010/2011 – cities for all: bridging the urban divide , New York : United Nations .
- United Nations Population Division, 2010. World Urbanization Prospects: the 2009 Revision [online]. United Nations. http://esa.un.org/unpd/wup/unup/ (http://esa.un.org/unpd/wup/unup/) (Accessed: 24 April 2011 ).
- Weeks , J.R. 2006 . Ethnic residential patterns as predictors of intra-urban child mortality inequality in Accra, Ghana . Urban Geography , 27 ( 6 ) : 526 – 548 .
- Weeks , J.R. 2007 . Can we spot a neighborhood from the air? Defining neighborhood structure in Accra, Ghana . Geojournal , 69 : 9 – 22 .
- Weeks , J.R. forthcoming . Connecting the dots between health, poverty and place in Accra, Ghana . Annals of the Association of American Geographers ,
- Wratten , E. 1995 . Conceptualizing urban poverty . Environment and Urbanization , 7 ( 1 ) : 11