Abstract
This article addresses several issues related to historical GIS data using a project studying the social culture of Republican Beijing as an illustration. For large-scale historical GIS projects, certain data layers or themes are fundamental to and provide the context for various types of investigation. We suggested that these data may be regarded as framework data, similar to the concept of the core dataset identified in the US National Spatial Data Infrastructure (NSDI) framework, but in a GIS project context. Due to various reasons, most historical GIS data always invite concerns about their quality. We discussed how typical spatial data quality concepts are partially applicable to historical GIS data. We also highlighted the data quality aspects that are more significant to historical than contemporary GIS data. Compiling high-quality historical GIS data is challenging. We used the data layer of temple locations as an example to illustrate the process of using a set of principles to resolve the inconsistencies of data from multiple sources to deal with location accuracy and data completeness problems. Two common but related quality concerns of historical GIS data are their relatively low spatial resolution and imprecise locations. The original population dataset of Republican Beijing suffers from these two issues. Using ancillary data, more precise population locations and population distribution at a higher resolution were estimated. Compilation of historical GIS data requires fusing data of different sources in order to enhance the quality of the data.
1. Introduction
Most definitions of spatial data adopt a simple binary view that spatial information is either presence or absence in the data. If location information is in the data, then the data are spatial data and vice versa. However, due to the conceptual and theoretical advancements in Geographic Information Science (GIScience) and the development of new information technologies, the definition of spatial data becomes more fluid. One may argue that location information is captured everywhere, including historical documents describing various wars, such as the Battle of First Manassas during the US Civil War,Footnote 1 and various documents providing directions in text, such as the one for visiting the US National Endowment for Humanities.Footnote 2 The recent concept of volunteered geographic information (VGI) highlights the fact that spatial information exists in many forms other than maps and drawings (Goodchild Citation2007, Sui Citation2008). Individuals are creating a large amount of spatial data by volunteering the geographic information they have gathered or access to. Data gathered through these processes are informative usually at the local scale, but their quality is often of great concern. The question is whether such data are good enough to be regarded as ‘useful’ spatial data for specific uses.
Similarly, the quality of historical GIS data is always a concern as the compilation of historical GIS data can be very complicated. The straightforward mechanical scanning and digitizing processes of converting map documents into digital formats to be stored in GIS rarely happen in compiling historical GIS data. The process can be quite challenging when certain requirements have to be met in order to support historical studies. For instance, the consistency of boundaries over multiple periods has to be maintained for the US National Historical Geographic Information System (NHGIS) project (Fitch and Ruggles Citation2003, McMaster and Noble Citation2005). Historical map documents may be of greater concerns than most contemporary map documents in terms of their quality such as location precision, completeness, and attribute accuracy. From another perspective, some of these quality issues may be perceived as spatial and temporal resolution issues, not to mention the concerns of extracting geographic information from nonmap documents to assist the creation of historical GIS data.
In the context of decision support, Heuvelink and Burrough (Citation2002) point out that ‘(i)t is crucially important to know how accurate the data contained in spatial databases really are, because without that knowledge we cannot assess the true value of the derived information, nor the correctness of the decisions it supports’ (p. 111). Such concern is also applicable to data for historical GIS projects. However, determining the accuracy of historical GIS data may not be possible in many situations. While certain historical GIS data may not possess the desirable quality levels, they may be indispensable to the project because they provide the basic geographical context of the study region. Then, the only option is to improve the quality of the data so that they can support the intended analyses. One possible approach is to use ancillary data, some of which may not capture explicit spatial information. The concept is similar to the concept of data fusion in spatial database management.
The main objective of this article is to highlight the challenges of compiling historical GIS data with an emphasis on data quality issues. The particular historical GIS database of our concern is the urban culture GIS database for Beijing during the Republican era, that is, between 1912 and 1937. For more background information about the project, refer to the article by So et al. (Citation2012) in this special issue. We suggest borrowing the notion of framework data in the US National Spatial Data Infrastructure (NSDI) to label those indispensable data layers that provide the contextual backgrounds for the interpretations of data and analysis results in the historical GIS project context. In the next section, we will present our arguments, together with a brief overview of the Republican Beijing database and the issues of spatial data quality in the historical GIS context.
To illustrate the challenges in data quality when compiling historical GIS data, we use two data themes of the Republican Beijing project. First, the location accuracy of a point data theme for temple locations was enhanced using an enhanced addressing matching framework. This framework leverages the availability of multiple data sources, but the difficulty is to resolve inconsistent information. In the second demonstration, we integrated building information to derive a higher resolution population data layer partly to support statistical analyses. The overall goal is to show how the quality of historical GIS data may be enhanced through various methods integrating different data sources.
2. Historical GIS data and data quality issues
In this section, we will first provide an overview of the GIS database compiled for the Republican Beijing project. Then we will discuss several spatial data quality issues, with an emphasis on their relevancy to historical GIS data.
2.1. Framework GIS data for the Republican Beijing project
The Republican Beijing project is intended to investigate various dimensions of Republican Beijing, such as city structure, economic environment, education, public health, law enforcement, and religion (So et al. Citation2012). While a diverse set of topics can be examined, a large number of historical spatial data along many themes have been gathered and compiled. Each theme focuses on a specific cultural or societal aspect of Republican Beijing, but few data of a single theme can exist or be interpreted meaningfully alone. These data themes are largely interdependent. Some offer explanations to phenomenon manifested by another theme, but some data themes are fundamental across the entire range of investigated topics. In fact, these data themes are critical to the project as they provide the geographical context of the study area. Without these data layers, other GIS layers may not make any geographical sense. They serve as the backbone of the entire diverse spatial database and are referenced to frequently in specific investigations. Two of these data themes are the district boundaries and the layout of the hutongs (alleys and streets). Another one is the population data for different police districts and subdistricts in Beijing during the Republican era.
In developing the US NSDI, the Federal Geographic Data Committee (FGDC Citation2004) developed a framework. Through established guidelines, technology, and procedures in the framework, participants can contribute to the development of a set of spatial data themes that are most commonly used. This set of geospatial data layers is labeled as framework data, which include geodetic control, orthoimagery, elevation and bathymetry, transportation, hydrography, cadastral, and governmental units. Various government agencies and local governments at all levels in the United States contribute to the development of these core GIS data themes.
The notion of framework data may be expanded to large-scale GIS projects where multiple parties are involved, each investigating a specific aspect of the project, but all sharing some core or common data themes that serve as the geographical foundation of the extensive and sometimes diverse geospatial database. If the large-scale GIS project focuses on socioeconomic analysis or policy-oriented studies in the United States, some of the core data layers may include political and statistical enumeration unit boundaries and population and demographic information derived most likely from the censuses. While, in this specific example, these core data themes are not contributed by different project participants as in the development of the framework data for the US NSDI, these core data themes likely are used by all investigators of the project. Therefore, these data themes may be regarded as framework data in this project-specific context. On the other hand, these projects may leverage the concept of the framework, engaging project participants to contribute to the development and improvement of the core data themes.
In the historical GIS context, gathering historical spatial data is expensive, tedious, and technically challenging. If the research topic has substantial interest in the research community, the framework concept can be employed such that individual investigators can contribute to the development of the core data themes supporting the research. Apparently, such effort should be appealed to interested researchers rather than to the general public. This approach in building core data themes for historical GIS is a more restricted version of the VGI approach.
In the Republican Beijing project, two of the core data themes are the boundaries of districts and the layout of the hutongs (alleys and streets). These layers are similar to the transportation and governmental unit themes in the US NSDI framework data layers. The Republic Beijing project has emphases on the social, economic, and political environments. Therefore, the population layer is also regarded as a core layer. Population distribution provides the context of interpreting many socioeconomic and political issues, as Trewatha (Citation1953) argued that ‘population is the pivotal element around which all the others are oriented … it is only from man or population that these other elements derive geographical significance’ (p. 86).
The city of Republican Beijing included the North City and South City. For administrative purposes, Beijing was divided into 20 police districts in 1912, which were restructured into 11 police districts in 1928 (). The central districts were in the Imperial City, the inside districts were in the North City, and the outside districts were in the South City. The left districts were on the east, and the right districts were on the west (Gamble Citation1921). Three historical maps were used to compile the base map in They are the Map of inner and outer cities of capital produced by the Office of Surveying and Mapping, Zhifang Department, Ministry of the Interior, at a scale of 1:8000 in 1916, the Survey map of inner and outer cities of Beiping produced by the Public Works Bureau of the Beiping Municipal Government at a scale of 1:5000 in 1937, and the Beiping city map published by China Map Press at the scale of about 1:14,285 in 1940. All these maps show the layout of hutongs, which reflects the urban structure of the city.
The 1937 map was treated as the main source for the base map partly because of its relatively high spatial resolution. However, the 1937 map is too old and the names of quite a few hutongs were not decipherable. Some names could be verified and corrected by comparing with the maps of 1916 and 1940. Nevertheless, not all the names on the 1937 map could be verified because it has more hutongs than the other two maps. To further improve the quality of our data, we also verified the hutong names on the maps with the Hutong of Beiping published by the Economic News Press in 1936. This book contains detailed information about hutongs and streets during that time and was useful for verifying and correcting hutong names on the 1937 map. Eventually, more than 3100 hutongs (i.e., line features) were recorded.
2.2. Data quality issues
During the process of creating the hutong data for Republican Beijing, accuracy of data was clearly an issue. Spatial data quality, which includes data accuracy, has been a concern for a long time in GIScience (e.g., Beard and Buttenfield Citation1991, Buttenfield Citation1993). According to US FGDC metadata standard for geospatial data, data quality information includes (1) attribute accuracy, (2) logical consistency, (3) completeness, (4) positional accuracy, (5) lineage, and (6) cloud cover (FGDC Citation1998). Most of these items are self-explanatory, but a few need clarifications. The last item of data quality, cloud cover, is applicable to remote-sensing data only. Logical consistency refers to the fidelity or correctness of spatial relationships represented in the data structure. A typical example is whether lines crossing each other create an intersection or not. Lineage refers to the historical development or the sources of the data. In general, all these items, with the exception of cloud cover, are relevant to the creation of historical GIS data.
Thomson et al. (Citation2005) suggest a topology of data uncertainty, with some elements overlapping with the FGDC metadata standard of data quality. Among the items they added, several are particularly relevant to historical GIS data. They are precision (exactness of measurement), currency/timing (temporal gaps between occurrence, information collection, and use), and credibility (reliability of information source). While precision is not a data quality item under the FGDC metadata standard, they are related to the positional accuracy (horizontal and vertical accuracy) and entity and attribute information (attribute accuracy) items of the metadata standard. However, precision takes into account factors that control or determine the environments of data gathering, but can affect data quality. Examples include the size of areal units for which the data are gathered, the precision of the instruments used to capture the data, and the measurement scale (nominal, ordinal, and interval/ratio) chosen to report the data. Information about currency/timing of data suggested by Thomson et al. (Citation2005) is partly recorded under the identification information, not data quality of the metadata standard. Credibility may partially be documented through the lineage information, but is more qualitative in nature.
While the data quality items suggested by FGDC and Thomson et al. (Citation2005) focus on the sources of uncertainty or error, Zhang and Goodchild (Citation2002) addressed the data quality issues with respect to the nature of uncertainty. They suggested that the three types of data uncertainty are error, randomness, and vagueness (Zhang and Goodchild Citation2002, p. 7). Error is the discrepancy between the observed and truth values. Randomness is a characteristic of the nature, and vagueness is the limitation of our measurement. While these natures of uncertainty are related to data quality issues discussed above, they provide another perspective to address the data quality issues.
The additional issues suggested by Thomson et al. (Citation2005) and the perspective adopted by Zhang and Goodchild (Citation2002) are applicable to spatial data in general, but some of them are more relevant to the compilation of historical GIS data than to other data. Historical GIS data are generally less precise than contemporary GIS data in terms of positional accuracy and attribute accuracy. Determining and recording a position in the historical past could be quite challenging and boundaries were usually not well recorded. Larger political and enumeration units were used and they in turn can support spatial data of relatively lower resolution. Measurements of characteristics were also less precise as compared to today's standards, partly due to the use of nominal or ordinal scale measurements rather than the interval–ratio scale, and partly due to the precision of instruments. Historical data, by definition, are not current data, and the currency or timing issues of the data need to be addressed. In order to analyze a particular historical event or period and the corresponding geography, available data may not match the study period very well. Using data of a different time may be the only alternative, but surely will raise a data quality concern. The challenge is to select alternate data sources to minimize potential errors. A related issue is to determine the credibility of different data sources.
3. Enhancing the quality of historical GIS data
Quite often, the quality of spatial data gathered from historical documents is too poor for the data to be useful in this digital era. If they are nonessential data, abandoning them will not hamper the entire project significantly. But if they are part of the framework dataset in the historical GIS project context, then their quality needs to be enhanced to the level to provide relatively reliable information. In this article, two framework GIS data themes for the Republican Beijing project are used to demonstrate the relevancy of some of the data quality issues discussed above and to illustrate how their quality levels were enhanced through incorporating ancillary data. Using the temple data, we illustrate the quality issues of completeness, lineage–currency, positional accuracy–vagueness, and attribute accuracy–credibility. We improved the positional accuracy of temple locations through a modified address-matching process and by resolving discrepancies from different data sources. Using the population data, we illustrate the issues of precision/spatial resolution, attribute accuracy, and currency. The original population data are tabulated just for a few large units covering the entire Republican Beijing area, failing to show the population distribution at a resolution that supports realistic analyses. We estimated the distribution of population for smaller areal units (i.e., at a higher spatial resolution) by incorporating ancillary data through an areal weighting method.
3.1. Example 1: temple data
Republican Beijing project gathered data describing various types of establishments, landmarks, and facilities. These features may be establishments such as schools, temples, hospitals, and police stations or physical objects such as wells and guard posts. Data describing these features have been extracted from various historical documents published by governments and organizations in the private sector. Data describing the features are stored in a database as attributes of the features. These attribute data have to be linked to geographical features or objects in GIS. Most of these features can be represented by points appropriately. However, location information, such as hutong addresses, may or may not be available. Sometimes, only some imprecise descriptions of the locations (e.g., street names) are available. Even if hutong addresses are available, no hutong address databases are available for Republican Beijing for address matching. Some innovative and heuristic methods were used to match the feature attributes to locations on a map.
To support the study of religious environment in Republican Beijing, we gathered data of 638 temples for 1928 and 576 temples for 1936, with a significant proportion of duplication. The dataset contains a large amount of attribute information, including addresses, numbers of rooms, and other properties owned by the temples. More than 80% of the temple addresses have street number information. On the other hand, many historical maps have symbols showing temple locations. A total of 462 temple symbols on maps were digitized. The 1937 map is supposed to provide the most accurate location information among other maps because it is closest to the year of the temple data for 1936, a criterion for data currency. Occasionally, the 1916 map was used for reference. The task was to correctly locate temples along the streets, create point features or use existing point features to represent temples in the spatial database, and assign the correct attributes to the point features. Unfortunately, the process was not straightforward as we had to deal with issues of data imprecision–randomness–vagueness, currency–creditability, and completeness.
Several assumptions and principles were formulated to guide the geocoding process, based upon our knowledge of the general street layout and numbering systems in Beijing at that time and the assumptions of how information was recorded.
1. | Street numbers of each hutong are assumed to follow the direction of the hutong label shown on the map. If the hutong has a horizontal direction on a map with north pointing upward, the street label in Chinese characters usually follows an east–west orientation, and the hutong is assumed to start on the eastern tip and end on the west.Footnote 3 | ||||
2. | Houses with odd and even street numbers are located on different sides of a hutong.Footnote 4 | ||||
3. | Temples with lower street numbers should be closer to the beginning of the hutong than temples with higher street numbers. | ||||
4. | We assume that it was more likely to record larger temples than smaller temples on a map. Thus when a map indicates a certain number of temples on a hutong, but our database has more temples than those on the map, then larger temples (i.e., with more rooms) are assigned to the temple features on the map. | ||||
5. | For those temple records in the database that cannot be matched to symbols on a map, they are plotted according to their street numbers. The location of a particular street number is determined by referencing to other point features with street numbers on the same hutong. |
Below, we use a few examples of typical situations to illustrate the modified geocoding process and the data quality issues involved.
Case 1: The number of temple symbols on the map is the same as the number of temple records in the database. |
When only one temple was found in the database for a specific hutong and only one temple symbol on the map, the situation is simple – assign the temple to the location with the temple symbol. The situation could be difficult when two temple symbols were on the map for a hutong, and two temple records were found in the database. In the database, two temples are on the wuwanghou hutong
(). On the 1937 map, a temple symbol indicated by an arrow was labeled with the name of the temple, bao'chansi
. Then the other temple is assigned to the location of the remaining temple symbol
on the map. This is relatively straightforward.
Case 2: The number of temple symbols on the map was fewer than the number of temple records in the database. |
In this case, many situations were possible and only a few are presented here. A temple on damochang
was found in the database, but both the 1937 and the 1916 maps show no temple symbol. Then the temple was registered to the middle of the hutong. In another situation, three temples on shitou hutong
appeared in the database (). Referring to the maps of 1937 and 1916, a temple location was identified on each map ( and b indicated by the arrows). Because the locations of the two temple symbols are quite different, the two symbols were considered to represent two different temples. Based on the assumption that temples with more rooms were more likely to be recorded, the two larger of the three temples, zhuntian
and yanshouan
, were assigned to the symbol locations. The temple with the lower street number, zhuntian
, was assigned to the symbol location closer to the beginning of the hutong, and the other temple, yanshouan
, with higher street number was assigned to the symbol at the other end. The remaining temple was plotted relative to the locations of yanshouan
and zhuntian
.
Case 3: The number of temple symbols on the map is more than the number of temple records in the database. |
In the database, only one temple was on guloudong dajie
(). Both the 1937 and the 1916 maps show two temple symbols (indicated by arrows in the figures). In this case, caishenmiao
is assigned to the middle of guloudong dajie
. Many possible reasons may cause this inconsistency across data sources, but one likely reason is the currency issue. New temples might have been built, but data were not updated timely.
Figure 4. The temple record for caishenmiao

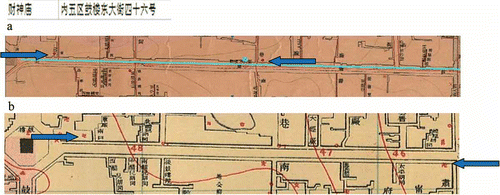
Case 4: Inconsistencies between the database records, the 1916 and the 1937 maps for the same hutong. |
Several examples are used to illustrate different types of inconsistencies. A temple was found on Beixincang
in the database, but the 1937 map shows no temple symbol on that hutong and the 1916 map shows one temple symbol as in the database. Then, the symbol location on the 1916 map was used as the location of that temple.
A more complicated example is that two temples were on zhongsitiao
according to the database (see ). The 1937 map has only one temple symbol (), while the 1916 map shows two symbols, with one located close to the temple symbol shown on the 1937 map (). In this situation, we took into consideration the temple street numbers. Because the street number of shoufosi
is 31 and guandimiao
is 43, shoufosi
should be located before guandimiao
on that hutong. Thus, shoufosi
was registered to the symbol location on the 1937 map, a location closer to the beginning of the street, while guandimia
is assigned to the next location on the hutong shown on the 1916 map.
In another example, three temples are found on xiaoshi dajie
according to the database (). However, only one temple label is found on the 1937 map, and three symbols are on the 1916 map ( and b). One of the symbols is labeled zhenwumiao
(right-hand side of ) and that symbol location was used for that temple. The other two temples were geo-registered to the remaining symbols according to their street numbers. Then caishenmiao
was placed after haichaoan
on the hutong, according to the symbol locations on the 1916 map.
In this final example, two temples are on the picai hutong
in the database (). The street numbers of both temples are very close together (34 and 38). But on the 1937 map (), the two temple symbols are quite far apart as indicated by the two arrows, while on the 1916 map (), the symbols are closer together. The symbol locations for one of the temples (likely shifosi
according to street number) are quite similar on the two maps. In this case, information on the 1916 map seems to be slightly more creditable, corroborated with the database. Thus, shifosi
was assigned to the symbol location on the 1937 map, and the other temple was assigned to the other location according to the 1916 map.
These are just a few examples of situations that we came across in compiling the temple data layer. Although the general principles discussed above were adopted most of the time, there are exceptional occasions that these principles were not followed completely. Nevertheless, these principles are instrumental in handling some of the data quality issues we discussed earlier. While these principles are not universal, similar principles can be developed based upon the particular geographical settings and societal conditions. For instance, identifying the general relationships between street numbers and orientations provides the foundation to all address matching processes. Knowing how the historical spatial data were gathered may help explain why some data are missing. Thus, procedures in handling missing data may be formulated. Developing such principles and guidelines are critical in addressing data quality issues.
3.2. Example 2: population counts of smaller synthetic districts
Population information is important in various types of inquiry in social science and humanities. From identifying the distribution of cultural trace (Sauer Citation1941) to understanding the spread of diseases in the past (Cliff et al. Citation1981), information about population distribution and its characteristics is crucial. In studying the social, cultural, and economic environments of Republican Beijing, population data are needed to support various types of analysis.
Many sources of population data have been gathered for the Republican Beijing project. The more reliable one is the Statistical graph of registered residence of Beiping police department published by Beiping Police Department in 1937. The dataset recorded the types of household and female and male population counts for the 11 police districts at that time. The primary source of boundary information for these districts is the Survey map of inner and outer cities of Beiping produced by the Public Works Bureau of the Beiping Municipal Government at a scale of 1:5000 in 1937. The map was digitized to create boundaries of the 11 districts.
Configurations of the police districts in Beijing had been restructured several times during the Republican era. The city was divided into 20 police districts in 1912, and the districts were divided and reaggregated into 11 police districts in 1928, same number as in 1937. With slightly more than 1 million in population at that time, 11 areal units seem to be too few to show any meaningful population distribution (too low in spatial resolution), and the locations of population are too imprecise. Knowing where people lived is important to various types of analysis. In terms of statistical analysis, 11 or even 20 areal units are too few for any robust statistical analysis. Therefore, we would like to locate the population more precisely to develop a higher resolution population distribution for the city.
After carefully examining the boundaries of the 20 police districts on the 1916 map, we found that the delineation of the 20 police districts seemed to follow primarily the physical layouts of streets and blocks (hutongs). Using this premise, we delineated 80 subdistricts for the city, following very much the street and block structures (map on the left in ). These smaller districts are not necessarily nested under the larger 11 districts in 1937. Some smaller districts cross the boundaries of larger districts. These subdistricts may be regarded as ‘synthetic’ districts (Gregory Citation2002, p. 295). Clearly, one may claim that multiple configurations of the 80 subdistricts could be derived, and data compiled according to different configurations will not be consistent. This well-known modifiable areal unit problem (MAUP) will be applicable to any partitioning system (Openshaw and Taylor Citation1979). Nevertheless, higher resolution population data are preferable from the perspectives of spatial pattern analysis and statistical analysis.
Figure 8. Boundaries of the 11 police districts and the 80 synthetic districts (a) and nonresidential polygons in the city (b).
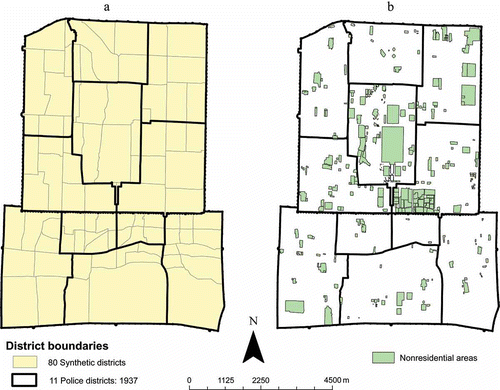
The next step was to derive the population counts for these subdistricts. In the United States, the Census Bureau considers several methods to estimate population for states and counties (Smith and Mandell Citation1984). While these methods are not directly usable to estimate the population sizes of the 80 synthetic districts based upon the population counts of the 11 districts (1937), the concept of housing unit method can be exploited to assist our estimation. Researchers have proposed other methods to estimate population sizes. Wu et al. (Citation2005) provide an overview of different types of methods, including the general areal interpolation approach. Many researchers have developed and applied areal interpolation techniques to estimate population sizes for situations in modern era (e.g., Langford et al. Citation1991, Martin Citation1996) and in the past (e.g., Gregory Citation2000, Citation2002). Some interpolation methods are relatively simple, weighted by areas. Some are quite complicated, such as the dasymetric mapping (e.g., Mennis Citation2003) and computational intensive cokriging method (e.g., Wu and Murray Citation2005). The applicability of different methods depends on various situations, including data availability, size of areal units, and nature of the spatial partitioning systems. The method proposed here may be labeled as a spatial interpolation method, but is combined with the housing unit method.
Our population estimation method leverages the availability of other spatial data themes gathered for the Republican Beijing project. Some layers that can be used to improve population estimation are government buildings, including offices, public facilities such as libraries, schools, and hospitals; religious establishments including temples and churches; and markets. As Beijing has been the capital of China for some time, many buildings in the city were government buildings, not for residential use. Estimating population distribution should take into account the nature of buildings. The general idea of our population estimation procedure is simply applying the population density levels in the police districts to all smaller synthetic districts within the corresponding police districts, but removing all nonresidential buildings in the process such that the estimated population counts are not distorted by nonresidential land use. This process put people into the appropriate buildings, improving the geographical precision of population distribution.
The process involves several steps. First, we derived a map of residential polygons by removing all polygons for nonresidential buildings and spaces not for housing people (map on the right in ). These polygons include government offices, libraries, schools, hospitals, markets, temples, churches as well as open public spaces such as parks, old palace, and the lakes. Thus, this map indicates where people lived in Republican Beijing and provides the residential area RA i for polygon i within police district j. Then, we derived the total residential area for each police district j by summing the areas of all residential polygons i within district j. That is
Then we assign this density level DP j to residential polygon i within the district j such that the population size of the residential polygon i in district j is
Using this method, the population sizes of the 80 synthetic districts of 1937 were estimated. The results are shown in . One may suggest that using this method, population estimates of any geographical partitioning system can be derived. Conceptually, such argument is correct, but caveats and limitations of the proposed approach should be acknowledged. The proposed method relies on the assumption that the population density of residential area within the police district was quite uniform. Thus we imposed the same population density levels across all residential polygons within the same police districts. This assumption surely can be challenged. However, we contend that this baseline population estimation method produces quite reasonable results. shows the population density maps for the 11 police districts and the 80 synthetic districts. Although the two maps have similar macro spatial patterns for population density, they do not correspond directly. Patterns of population density for the smaller districts are more refined than that for the larger districts. Locations of residential polygons, which vary quite significantly within police districts, mediated the effects of the population density constraints when they were imposed onto the synthetic districts ().
Figure 9. Population counts of 11 police districts (a) and 80 synthetic districts (b), 1937, using quantile classification method.
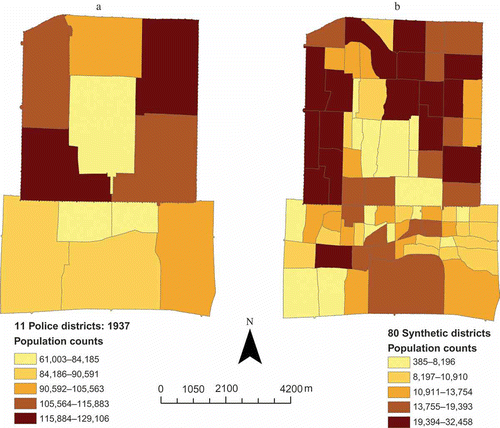
Figure 10. Population density levels of the 11 police districts (a) and the 80 synthetic districts (b).
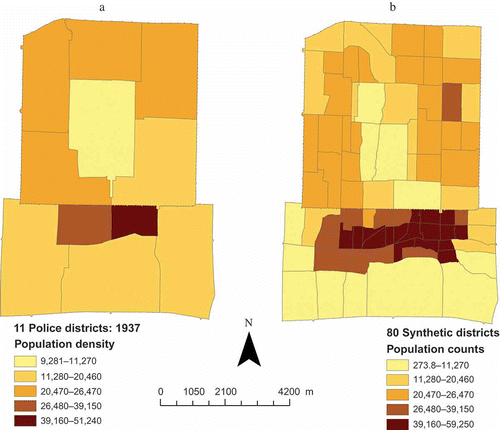
In many areal interpolation methods to estimate population, ancillary data including land use data are incorporated (Wu et al. Citation2005). Our method does not explicitly incorporate those ancillary data. However, during the process of removing nonresidential polygons in the estimation process, those ancillary data, such as different building types, were taken into account. Nevertheless, the proposed method can be improved if more detailed data, such as housing types, are available. Similar to the lesson learned in the temple address matching example above, quality of historical spatial data in different themes may be enhanced if we can leverage on ancillary data.
4. Conclusion and significance
For most GIS projects, data acquisition usually requires the most resources. During this process, data quality is occasionally a major concern. But for most historical GIS projects, these two related issues are of disproportional significance. Acquiring relevant historical geospatial data is challenging by itself. Quality of historical geospatial data complicates the data acquisition process significantly. Not only historical geospatial are scarce in general, but their quality may not be at the preferred level. Quite often, the issue comes down to the decision of using data of less than the desirable quality versus not using the data at all. While using data of suboptimal quality may be the more likely decision, this article demonstrates how the quality of historical GIS data may be enhanced. Using two data themes of temple locations and population counts, we show that usage of ancillary data can enhance the accuracy of geocoding temple locations and improve the precision of locating population.
To a large degree, the validity and power of analytical results from historical GIS are hinged upon the quality of data. Therefore, understanding the limits of data in terms of their quality is of great importance. In this article, we also discuss data quality concepts particularly relevant to historical GIS data. Standard geospatial data quality attributes may not be comprehensive enough to document certain quality aspects of historical geospatial data. Currency/timing is of great importance in the historical context. Older geospatial data in general tend to be less precise and are of lower resolution both for attribute measurement and for location accuracy.
So far, we have not been able to systematically evaluate the accuracy of our raw and enhanced data. Using ancillary data we may be able to spot check or indirectly verify the accuracy of our data, but a systematic evaluation of the entire dataset will be very unlikely. The reason is that whenever possible and appropriate, we have incorporated all ancillary data that we have gathered to improve our dataset. If data of Republican Beijing from independent sources are available, then a formal evaluation of data accuracy and quality will be possible. Currently, we should expect that our raw and enhanced data should have a certain level of inaccuracy, but hoping for the best that the quality is still reasonable. Not being able to obtain geospatial data at the preferred level of data quality is not uncommon, and using data of questionable quality is often an unavoidable situation. As Openshaw (Citation1989) points out, we have to live with uncertainty or error in spatial data. This is even more so for historical GIS projects. What level of data quality is acceptable is apparently dependent upon the specific applications and objectives of the study.
Acknowledgments
We are grateful for the financial support provided by Research Grant Council of Hong Kong through an Earmarked Grant (Project no. 450407). The constructive comments provided by the two anonymous referees are also greatly appreciated.
Notes
1. US National Park Service [online]. Available from: http://www.nps.gov/mana/historyculture/first-manassas.htm [Accessed 5 June 2011].
3. While we list this ordering of street numbers under assumption, we have examined historical documents, confirming that our understanding of the street number ordering system is correct.
4. Similar to the previous assumption, we have verified this assumption through examining historical documents.
References
- Beard , M.K. and Buttenfield , B.P. 1991 . NCGIA Research Initiative 7: visualization of spatial data quality. NCGIA, Technical Paper , 91 – 26 . Santa Barbara , CA : UC Santa Barbara .
- Buttenfield , B.P. 1993 . Representing data quality . Cartographica , 30 ( 2 ) : 1 – 7 .
- Cliff , A.D. 1981 . Spatial diffusion: an historical geography of epidemics in an Island community , Cambridge University Press .
- Federal Geographic Data Committee (FGDC), 1998. Content standard for digital geospatial Metadata (version 2.0). http://www.fgdc.gov/standards/projects/FGDC-standards-projects/metadata/base-metadata/v2_0698.pdf (http://www.fgdc.gov/standards/projects/FGDC-standards-projects/metadata/base-metadata/v2_0698.pdf) (Accessed: 8 June 2011 ).
- Federal Geographic Data Committee (FGDC), 2004. Framework introduction and guide. http://www.fgdc.gov/framework/handbook/index_html (http://www.fgdc.gov/framework/handbook/index_html) (Accessed: 8 June 2011 ).
- Fitch , C.A. and Ruggles , S. 2003 . Building the national historical geographic information system . Historical Methods , 36 : 41 – 51 .
- Gamble , S.D. 1921 . Peking: a social survey , New York : George H. Doran .
- Goodchild , M.F. 2007 . Citizens as sensors: the world of volunteered geography . GeoJournal , 69 ( 4 ) : 211 – 221 .
- Gregory , I.N. 2000 . Longitudinal analysis of age and gender specific migration patterns in England and Wales: a GIS-based approach . Social Science History , 24 : 471 – 503 .
- Gregory , I.N. 2002 . The accuracy of areal interpolation techniques: standardising 19th and 20th century census data to allow long-term comparisons . Computers, Environment and Urban Systems , 26 : 293 – 314 .
- Heuvelink , G.B.M. and Burrough , P.A. 2002 . Developments in statistical approaches to spatial uncertainty and its propagation . International Journal of Geographical Information Science , 16 ( 2 ) : 111 – 113 .
- Langford , M. , Maguire , D. and Unwin , D.J. 1991 . “ The areal interpolation problem: estimating population using remote sensing in a GIS framework ” . In Handling geographical information: methodology and potential applications , Edited by: Masser , I. and Blakemore , M. 55 – 77 . Harlow , , UK : Longman .
- Martin , D. 1996 . “ Depicting changing distributions through surface estimations ” . In Spatial analysis: modelling in a GIS environment , Edited by: Longley , P. and Batty , M. 105 – 122 . Cambridge , , UK : GeoInformation International .
- McMaster , R.B. and Noble , P. 2005 . The US national historical geographic information system . Historical Geography , 33 : 134 – 136 .
- Mennis , J. 2003 . Generating surface models of population using dasymetric mapping . The Professional Geographer , 55 ( 1 ) : 31 – 42 .
- Openshaw , S. 1989 . “ Learning to live with errors in spatial databases ” . In Accuracy of spatial databases , Edited by: Goodchild , M.F. and Gopal , S. 263 – 276 . London : Taylor & Francis .
- Openshaw , S. and Taylor , P.J. 1979 . “ A million or so correlation coefficients: three experiments on the modifiable areal unit problem ” . In Statistical applications on the spatial sciences , Edited by: Wrigley , N. 127 – 144 . London : Pion .
- Sauer , C.O. 1941 . Foreword to historical geography . Annals of the Association of American Geographers , 31 ( 1 ) : 1 – 24 .
- Smith , S.K. and Mandell , M. 1984 . Comparison of population estimation methods: housing unit versus component II, ratio-correlation and administrative records . Journal of American Statistical Association , 79 ( 386 ) : 282 – 289 .
- So , B.K.L. 2012 . GIS in urban cultural studies: reflections from the project on Republican Beijing . Annals of GIS, (in this issue) ,
- Sui , D.Z. 2008 . The wikification of GIS and its consequences: or Angelina Jolie's new tattoo and the future of GIS . Computers, Environment and Urban Systems , 32 ( 1 ) : 1 – 5 .
- Thomson , J. Typology for visualizing uncertainty . Proceedings of the IS&T/SPIE symposium on electronic imaging, conference on visualization and data analysis . January 16–20 , San Jose , CA .
- Trewartha , G.T. 1953 . A case for population geography . Annals of the Association of American Geographers , 43 ( 2 ) : 71 – 97 .
- Wu , C. and Murray , A.T. 2005 . A cokriging method for estimating population density in urban areas . Computers, Environment and Urban Systems , 29 ( 5 ) : 558 – 579 .
- Wu , S.-S. , Qiu , X. and Wang , L. 2005 . Population estimation methods in GIS and remote sensing: a review . GIScience and Remote Sensing , 42 ( 1 ) : 80 – 96 .
- Zhang , J. and Goodchild , M.F. 2002 . Uncertainty in geographical information , London : Taylor & Francis .