Abstract
Geographic information systems (GIS) are widely used for mapping wildlife movement patterns, and observed wildlife locations are surrogates for inferring on wildlife movement and habitat selection. We present a new approach to mapping areas where wildlife exhibit sustained use, which we term slow movement areas (SMAs). Nested within the habitat selection concepts of home range and core areas, SMAs are an additional approach to identifying areas important for wildlife. Our method for delineating SMAs is demonstrated on a grizzly bear (Ursus arctos) case study examining road density. Our results showed that subadult females had significantly higher road densities within SMAs than in their potential path area home ranges. The lowest road density was found in the SMAs of adult male grizzly bears. Given increased mortality risks associated with roads, female encampment near roads may have negative conservation implications. The methods presented in this manuscript compliment recent developments to identify movement suspension and intensively exploited areas defined from wildlife telemetry data. SMA delineation is sensitive to missing data and best applied to telemetry data collected with a consistent resolution.
1. Introduction
Due to improved GPS technology there has been an increase in availability of telemetry data that has led to growth in movement analysis methods development (e.g., Thériault, Claramunt, and Villeneuve Citation1999; Dodge, Weibel, and Lautenschütz Citation2008; Long and Nelson Citation2013). At the frontier of movement research are wildlife studies that use movement as a surrogate for understanding behaviour. Taking a classic spatial statistics perspective, the spatial pattern of observed wildlife locations is an expression of spatial processes that are difficult to measure directly (Nelson and Boots Citation2008). In this case, the spatial processes are biological and originate from dynamic wildlife behaviour. Given that we cannot observe behaviour continuously in space and time, patterns of movement are a surrogate measure for behavioural states (Morales et al. Citation2004). For instance, Hunter (Citation2007) determined that foraging behaviours occurred when grizzly bears (Ursus arctos) were moving at a velocity of less than 52 m/minute. Food searching was associated with movement velocities of 52 to 223 m/minute and active walking occurred at velocities of greater than 223 m/minute.
Related to wildlife movement research is the use of telemetry data for understanding spatial and temporal patterns of habitat selection (e.g., Berland et al. Citation2008). Telemetry data represent discrete locations of an individual animal in space and time and have been used extensively to study habitat selection by wildlife (Smulders et al. Citation2010). Most habitat selection research employs the concept of home range or core area (Smulders et al. Citation2012). A home range is typically defined as the area to which an animal confines its normal movements (Burt Citation1943) and the core area is an intensively utilized subset of the home range (Samuel and Green Citation1988). Recently, other concepts such as intensively selected areas are also being employed (Benhamou and Riotte-Lambert Citation2012). There are many ways to define a home range and core area, but arguably the most common is by applying kernel density estimation to telemetry data to generate an utilization distribution. The 95% contour of the utilization distribution is associated with the home range while the 50% contour is associated with the core (Worton Citation1987). Spatial units, like home range and core area, represented as discrete polygons, are integrated with spatially continuous data on the physical environment to characterize habitat conditions. The end goal is often to characterize the environmental elements (e.g., land cover types) that can be best managed for the purpose of wildlife conservation (Bourbonnais et al. Citation2013).
Even with the availability of detailed wildlife location data and more continuous and timely landscape data from remotely sensed imagery, it has proven difficult to quantify links between movement, behaviour and habitat. As typical with spatial pattern analysis, assumptions are required to make a linkage between spatial patterns of wildlife occurrence and processes of wildlife behaviour (Getis and Boots Citation1978). These assumptions pose difficulty due to subjective thresholds that are applied to patterns to categorize behaviour (Hunter Citation2007). Concepts of home range and core area have persisted in the literature, in part, because they are conceptually tidy and do not require inference on behaviour. Using home ranges and related concepts, habitats are associated with locations where wildlife is observed and the more often they are observed in a location the greater the resource utilization. Though home range and core areas are important for identifying habitats selected by wildlife, they cannot be employed to identify wildlife ‘use’ areas, as the activity of wildlife at any given location is not known.
In this paper we revisit the notion of spatial–temporal patterns of resource utilization, selection and use by defining slow movement areas (SMAs). SMAs are defined at the finest spatial and temporal scale afforded by given telemetry data-sets. We argue that when animals are moving the slowest there is a high likelihood that the selected or available habitat is being actively utilized for a specific biological function. We do not classify use behaviour in SMAs; however, likely behaviours are resting and feeding or stopovers, depending on the scale of data.
We propose a new method for delineating SMAs by modifying an existing technique for quantifying animal home ranges: the potential path area (PPA) home range (Long and Nelson Citation2012). This method builds upon an existing analytical framework, termed time geography (Hägerstraand Citation1970), useful for quantifying and examining the role of spatial–temporal constraints on movement (Baer and Butler Citation2000). The PPA method takes a pragmatic approach to movement analysis, focused on defining areas that are spatially and temporally accessible. Given the importance of relating movement to habitat conditions, the PPA polygons provide a simple approach for characterizing habitats associated with locations likely utilized. The benefit of using the existing PPA to define new SMAs is demonstrated through a case study on grizzly bears in Alberta, Canada. We show how within PPA home ranges, which represent habitat selection, and SMAs, which represent likely habitat use, the density of roads varies and trends for males and females are also opposite. Given that bears in Alberta are most likely to die near roads (Benn and Herrero Citation2002), intensive habitat use near roads may negatively impact survival (Bourbonnais et al. Citation2013).
2. Slow movement areas (SMAs)
While there are several methods for computing a home range, we based our approach for computing SMAs on Long and Nelson (Citation2012) and calculated a grizzly bear home range using the PPA home range method. The PPA home range delineates the area accessible to the animal given its sequence of telemetry fixes and a movement parameter (vmax – defined as an animal’s maximum travelling speed). The spatial area of accessibility between two fixes can be defined based on the single parameter – vmax, and the time difference between the two fixes, and is easily computed as a perfect ellipse shape ( – upper panel). The PPA ellipse encompasses the entire area the animal could have traversed based on its maximum travel speed and the location and time duration between consecutive fixes. By combining the n−1 ellipses, from a data-set of n telemetry fixes, the PPA home range is delineated (Long and Nelson Citation2012).
Figure 1. Delineating potential path area (PPA) and calculating the slow movement area (SMA).
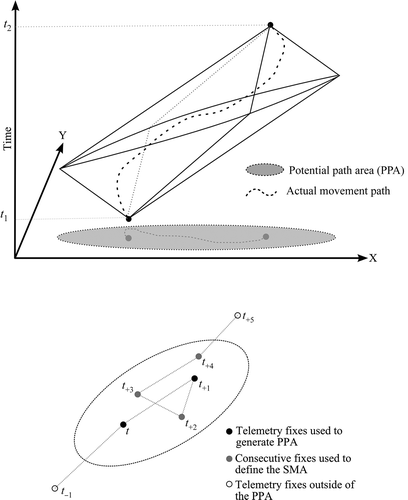
In order to compute SMAs we first define a statistic mi (i = 1…n−1) representing the number of consecutive telemetry fixes that fall within each PPA ellipse ( – lower panel). By definition, each ellipse will include, at a minimum, two fixes (mi ≥ 2; i.e., the current fix and the next telemetry fix). The mi with the highest scores can then be used to represent SMAs on a map, taking the highest score(s), or based on some threshold. Mapping of SMAs involves taking the union of the mj−1 PPA ellipses of the mj fixes beginning with index j, where an SMA can be defined as:
The calculation of the PPA home range and SMAs will be impacted by the selection of the vmax parameter. Parameterizing vmax is subject to similar issues as have been discussed for the bandwidth selection when using kernel density estimation to define home ranges (Seaman et al. Citation1999; Gitzen, Millspaugh, and Kernohan Citation2006, Nelson and Boots Citation2008). Higher values of vmax will lead to the delineation of larger PPA home range and SMAs. As vmax increases the animal is represented as being able to move more quickly and therefore has more accessible habitat. Like kernel density bandwidth selection, selecting vmax will always be prone to some subjectivity (see Nelson and Boots Citation2008 for discussion of bandwidth selection). We suggest that analysts use multiple confirmatory sources when determining the most appropriate vmax parameter. Biological information on maximum or typical speeds of travel can be compared to estimates generated from observed data to build confidence in the vmax value selected.
The spatial–temporal extent and resolution of telemetry data will also impact the interpretation, and indeed appropriateness, of home range and SMAs defined using PPA approaches (). Ideally, PPA approaches are applied when the spatial–temporal resolutions and extents of telemetry fixes are similar throughout a data-set (see Wiens Citation1989 for discussion of scale). For instance, if wildlife data are collected every 20 minutes in a 10×10 km area the data are relatively fine and SMAs are likely representation of sleeping or feeding. In contrast, landscape scale trends, such as migratory stopover locations, could be identified when the SMA is defined for data collected once a day over a broad area. Interpreting a SMA will be problematic if the resolution of the data is coarse and the study area fine, as the areas delineated as SMA will be overgeneralized. Similarly, if the study area is very large and the resolution of telemetry data fine, the SMAs defined will likely be too small. Partitioning the telemetry data into smaller subsets prior to analysis may lead to more meaningful results.
Figure 2. Data resolutions and extents most appropriate for use with PPA home range and SMA delineation do not mix scale.
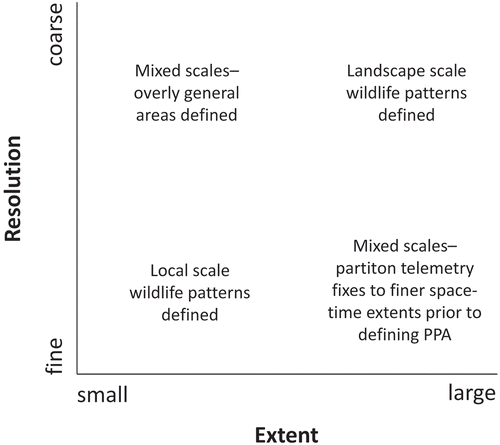
Users should also be cautioned against defining SMAs in data-sets that have variable spatial–temporal resolutions and missing fixes. If some fixes are taken at both 1- and 4-hour intervals, the longer intervals will have larger PPA ellipses and be biased towards higher counts of consecutive points within the ellipse. When data are sampled at varying resolutions the data can be partitioned by resolution, for separate analysis and SMA delineation, or all the data downgraded to the coarsest resolution.
Missing fixes are also problematic. If dropped fixes are not accounted for, PPA ellipses could be artificially large and/or counts of consecutive points within the ellipse low. If many fixes are dropped we recommend excluding that portion of the telemetry data from SMA calculations to preserve analysis integrity. However, if only a few fixes are missing it may be possible to clean the data by interpolating fixes. Given the sensitivity of many methods to missing data (Frair et al. Citation2010) corrections, such as linear interpolation based on curvilinear interpolation, have been demonstrated to improve data quality (Tremblay et al. Citation2006). Another technical issue will arise when fixes are dropped if an individual is denning or resting in an area that has poor signal coverage. No method can pick up habitat selection or use in locations that for reasons of terrain or vegetation do not record telemetry fixes and many movement metrics are sensitive to missing data (Laube and Purves Citation2011). However, since SMAs are intended to pick up slow movement, which may be associated with resting, missed resting location is an important omission to consider. There is no systematic way to identify omitted resting area due to missing data. Rather, when fix frequency becomes low or is missed for an extended period we recommend manual assessment.
3. Case study
Our methodology to define SMAs is demonstrated with a case study of grizzly bears in Alberta, Canada. In the Kakwa region of west central Alberta, grizzly bears share their habitat with many anthropogenic disturbances that are affecting the bears’ traditional use and selection of habitat. To illustrate the utility of our methodology, we examined the density of roads within the SMAs compared to the PPA home range. Road density has been found to correlate with mortality risk and reduced survival in grizzly bears (Benn and Herrero Citation2002; Nielsen et al. Citation2004), yet areas with high road densities are often selected as habitat (Roever, Boyce, and Stenhouse Citation2008; Graham et al. Citation2010; Stewart et al. Citation2013). It is not fully understood why bears appear to select habitat with roads, but it has been speculated that roadside areas offer bear food (Roever, Boyce, and Stenhouse Citation2008; Graham et al. Citation2010; Stewart et al. Citation2013).
Differences in habitat selection by male and female grizzly bears are becoming increasingly documented as the body of grizzly bear research grows. The much larger males are known to have larger home ranges (Proctor et al. Citation2004; Roever, Boyce, and Stenhouse Citation2008; Graham et al. Citation2010) and greater daily movement rates when compared to females (Boulanger et al. Citation2013). In contrast, females have been found to select habitat containing roads more than males (Roever, Boyce, and Stenhouse Citation2008; Graham et al. Citation2010; Stewart et al. Citation2013). Female selection of roads is of concern, especially for a threatened population, given that female survival is paramount for population viability (Eberhardt, Blanchard, and Knight Citation1994; Stewart et al. Citation2013). Understanding the behaviour of female grizzly bears associated with roads will provide important conservation information for those tasked with land-use decision-making.
3.1. Study area and data
The study area for this research is an 8308 km2 landscape in the Kakwa region of west central Alberta, Canada. The elevation ranges from 549 to 2446 m, and the area comprises a diverse and multi-use landscape. Resource extraction industries have been active in the area for a number of decades (White et al. Citation2011), with most disturbances in the area arising from the forest industry and oil and gas exploration (Schneider Citation2002).
A data-set of GPS locations collected over 2005 to 2010 from 25 grizzly bears in the study area were provided by the Foothills Research Institute Grizzly Bear Program (Hinton, AB). The FRI researchers followed the accepted protocols of the Canadian council of animal care for the safe handling of bears (animal use protocol number 20010016) (Stenhouse and Munro Citation2000). Bears were fitted with Televilt/Followit brand GPS collars (Lindesburg, Sweden). We obtained road data for the study area from Alberta Environment and Sustainable Resource Development and updated it through heads-up digitizing of medium- and high-resolution satellite imagery (SPOT and air photos).
3.2. Analysis
By definition, SMAs are delineated using both the spatial and the temporal structure of telemetry data. Thus, we began our analysis by correcting for missing fixes that are inevitable with GPS-based telemetry systems (Rempel, Rodgers, and Abraham Citation1995). An interpolation algorithm was used to accommodate for missing fixes in order to generate trajectories with consistent sampling intervals (regular trajectories – Calenge, Dray, and Royer-Carenzi Citation2009). When the GPS signal was disrupted for an extended period of time (i.e., >4 fixes) we analysed the bear trajectory separately on either side of the disruption.
For each individual bear we calculated the PPA home range and SMA (). We took a simple approach to SMA analysis here using only the longest encamped period (i.e., the mi with the highest score) to generate the SMA. Road density within individual bear home ranges and SMAs were then calculated and summarized by age and sex. We excluded the SMA from the home range when calculating road density to compare between the home range and SMA. Grizzly bears less than five years old are considered subadults and their selection of habitat has been shown to be different from adult bears (Mueller, Herrero, and Gibeau Citation2004). We partitioned bears by age (subadult or adult) and by sex. For each age-sex class (adult females, adult males, subadult females, subadult males) we assessed the statistical differences in the road density within home ranges and SMAs by comparing frequency distributions using a Mann–Whitney U statistical test.
3.3. Results
For adult females, the average PPA home range was 466.83 km2, whereas the average SMA size was 117.24 km2. The average PPA home range for sudadult females was 540.00 km2 and the average SMA was calculated to be 153.91 km2. When all female data were combined, the average PPA home range was 479.44 km2 and the average SMA was 131.72 km2.
Adult male grizzly bears were found to have an average PPA home range that was 674.58 km2 and an average SMA that was 149.21 km2. The average PPA home range for subadult males was 560.70 km2 whereas their average SMA was found to be 136.81 km2. When all males were considered together, the average PPA home range was 651.49 km2 and the SMA was calculated to be 144.53 km2.
Average road density was calculated for all groups in both the PPA home range and the SMAs (, and ). Road density in the SMAs for adult females was very similar to the road density in the PPA home range (0.60 and 0.59 km/km2, respectively) ( and ). However, for subadult females, the road density was significantly higher (p = 0.0209) in the SMA compared to the PPA home range (0.66 and 0.50 km/km2, respectively) ( and ).
Table 1. A comparison between the road density in the SMAs and the home range.
Figure 4. Box plots comparing the road density within all female (a) and all male (b) bear PPA home ranges to their SMAs.
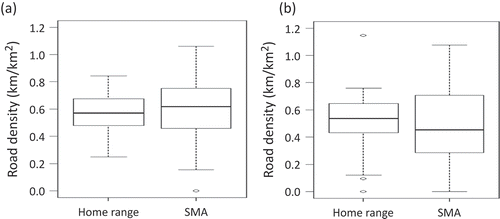
Figure 5. Box plots of road densities within PPA home ranges compared to SMAs for (a) adult females, (b) subadult females, (c) adult males and (d) subadult males.
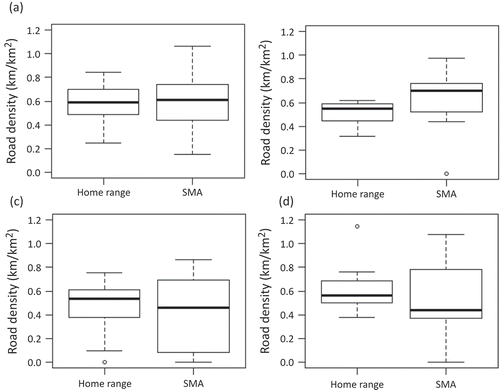
In general, male grizzly bears were found to have lower road densities in their SMAs compared to their PPA home ranges ( and ). The lowest road density was found in the SMAs of adult males (0.43 km/km2). Males also generally had lower road densities in both their SMAs and PPA home ranges (0.46 and 0.52 km/km2, respectively) compared to their female counterparts (0.63 and 0.57 km/km2, respectively).
4. Discussion
Grizzly bears often rest adjacent to sites recently used for feeding (Phillips Citation1987) and it is reasonable to assume that low mobility activities in SMAs consisted primarily of feeding/foraging and resting. Previous research has demonstrated the selection of roads by grizzly bears (Chruszcz et al. Citation2003; Graham et al. Citation2010; Stewart et al. Citation2013), yet were not able to provide movement details. Roads have been associated with increased mortality in grizzly bears (Benn and Herrero Citation2002) and it is important to fully recognize their attraction to bears when making land-use decisions that support conservation (see Stewart et al. Citation2013 for a more in-depth discussion) and aid in population recovery efforts. It is concerning that the subadult females in our study had a significantly greater concentration of roads in their SMAs compared to the remainder of their home ranges. The survival of vulnerable subadult females into the adult breeding stage is essential for population viability (Mueller, Herrero, and Gibeau Citation2004). While previous studies have observed the selection of roads by subadult females (Mueller, Herrero, and Gibeau Citation2004), the results of our case study provide insights into the behaviour associated with roads.
It is interesting that the male grizzly bears had fewer roads in their SMAs compared to the remainder of their home range and also to female bears. A previous study using the same database had found male grizzly bears to select natural edge habitats over anthropogenic edges (Stewart et al. Citation2013). Our case study has enabled us to determine that slow movement behaviours in males are associated with areas with fewer roads. A road density of 0.6 km/km2 has been previously postulated as the limit for naturally functioning landscapes containing sustained populations of large predators including grizzly bears (Forman and Alexander Citation1998). Our study suggests that areas with lower road densities appear to be most desirable for adult males’ encampment and it is possible that the female bears are being competitively excluded from these areas by more dominant conspecifics (Mattson, Knight, and Blanchard Citation1987; Edwards et al. Citation2011).
Methods for analysing spatial–temporal data have been touted as an opportunity area for spatial science development (Nelson Citation2012). Movement data are inherently spatial and temporal and there are many examples of recent developments in methods for quantifying movement in people (Jankowski et al. Citation2010), wildlife (Langrock et al. Citation2012) and traffic (Andrienko and Andrienko Citation2013). SMA delineation compliments recent progress in movement science, such as the development of methods to identify suspension in human movement (Orellana and Wachowicz Citation2011), stopover ecology (Sawyer and Kauffman Citation2011), and areas intensively exploited by wildlife (Benhamou and Riotte-Lambert Citation2012).
Wildlife researchers require a range of methods to characterize different types of movement patterns. The most simple movement pattern measure is velocity obtained by dividing the spatial distance by the time difference of two consecutive fixes (Brillinger et al. Citation2004; Chapman, Dytham, and Oxford Citation2007). Velocity (speed, time lag or step length) (Brillinger et al. Citation2004; Calenge, Dray, and Royer-Carenzi Citation2009) and other metrics (turning angle and bearing) (Turchin Citation1986; Calenge, Dray, and Royer-Carenzi Citation2009) are related to behaviour by defining arbitrary thresholds. A one-to-one relationship between spatial patterns of movement and behaviour is difficult to define making it problematic to relate behaviour and habitat. As well, it is often desirable to associate movement behaviour with an area, as is often the case in habitat selection studies, point-based representations are limited. Another potential limitation of basic velocity measures is that they are computed based on only two consecutive fixes, ignoring potentially useful information from larger consecutive intervals within the telemetry data. Turning angle is typically computed on three points but has similar limitations.
The theory of SMAs links conceptually with existing notions of home range and core area delineation (Worton Citation1987). Without requiring a classification of wildlife behaviour, SMAs allow us to define areas that have a high probability of resource use. The nature of that resources use will vary depending on species and scales of data. Like home range and core area delineation the strength of SMAs lies in the assumption that spatial patterns are expressions of spatial processes (Getis and Boots Citation1978). A single spatial pattern can be related to many different processes making behaviour difficult to infer. Therefore, methods that can identify utilization, without requiring one-to-one relationships with behaviour, are important for wildlife research and support assessment of utilized habitats and wildlife conservation. However, a unique component of both the PPA and SMA methods is the inclusion of the temporal component of the data. The selection of SMAs is consecutive in time. With increasingly available high-resolution telemetry data, the SMA approach to identifying habitats associated with subregions of the home range associated with encamped or slow movement behaviours. In the case of the grizzly bear, identifying SMAs may indicate critical foraging regions that are important in conservation management efforts since grizzly bears require almost continuous feeding to meet their nutritional needs (Rode, Robbins, and Shipley Citation2001).
As SMAs are an extension of the PPA home range approach there are also strong ties to other recently developed path-based measures of animal home range, namely the Brownian bridge home range (Bullard Citation1999; Horne et al. Citation2007), and time geographic kernel density estimation (Downs Citation2010; Downs, Horner, and Tucker Citation2011). The PPA home range represents a direct measure of spatial range (as a spatial polygon) while both the Brownian bridge and time geographic kernel density estimation methods first estimate a utilization distribution, followed by extracting a home range polygon. The definition of SMAs within a PPA home range is another mechanism for understanding utilization, though it is a measure of encampment rather than percentage time spent at a location. A benefit of the SMA delineation is that areas are defined using maximum speed as the only subjective parameter. Our approach specifically does not identify frequently revisited areas and alternative approaches (e.g., Benhamou and Riotte-Lambert Citation2012) are more appropriately designed specifically for discovering these revisitation areas, for example associated with important movement corridors.
To calculate SMAs, every telemetry location is assigned both an ellipse and a value (mi) identifying the number of consecutive points that fall within that ellipse. As such, it is possible to map how long an animal was in an area for all PPA ellipses or telemetry locations. Different lengths of utilization could be linked with different behaviours (i.e., foraging, resting and travelling); however, as with velocities, linkages to behaviour require that subjective thresholds be defined. In this analysis we defined the SMA using only the largest – max(mi) – value, identifying a SMA; however, in many applications it will be advantageous incorporate, for example, the 10 largest values of mi. This may be especially important with larger telemetry data-sets covering long temporal durations, where multiple SMAs could identify recurring behaviour associated with sustained use and low mobility rates.
Future research could develop techniques for more objective definitions of movement pattern thresholds. For instance, using theory from spatial statistics it may be possible to begin teasing apart when various movement patterns are most likely realizations of different processes (Getis and Boots Citation1978; Smulders et al. Citation2010). Similar to variability in the nature of home ranges, how the SMA is utilized is related to the spatial and temporal scales of the telemetry data. As in the grizzly bear example, when data are hourly or finer, the behaviours most likely associated with SMAs are feeding or resting. If telemetry data are collected at coarse temporal resolutions and extents, the SMAs will reflect broader scale processes such as migratory stopovers.
5. Conclusions
New methods for characterizing wildlife movement patterns will give researchers greater flexibility in the types of hypotheses investigated. We present a new approach to delineating areas where an animal exhibits sustained use. Similar to home ranges and core areas, SMAs are areas where spatial patterns indicate habitat selection and do not require explicit categorization of behaviour. However, SMAs are related to encampment and will represent a range of short-term behaviours such as foraging or resting when telemetry data are collected frequently, and migratory stopover locations for data-sets with a long temporal extent. Regardless of scale, the areas defined by the SMA have a high likelihood of wildlife resource use. SMA delineation methods require consistent spatial–temporal resolutions and minimal missing data. Future research should investigate how a time geographic framework, such as the PPA ellipses presented here, can be used to map a range of habitat utilization behaviours based on length of time spent in each area.
Acknowledgement
Data used for the case study are provided by the Foothills Research Institute (FRI) Grizzly Bear Program.
References
- Andrienko, N., and G. Andrienko. 2013. “Visual Analytics of Movement: An Overview of Methods, Tools and Procedures.” Information Visualization 12 (1): 3–24. doi:10.1177/1473871612457601.
- Baer, L. D., and D. R. Butler. 2000. “Space-time Modeling of Grizzly Bears.” Geographical Review 90 (2): 206–221.
- Benhamou, S., and L. Riotte-Lambert. 2012. “Beyond the Utilization Distribution: Identifying Home Range Areas that are Intensively Exploited or Repeatedly Visited.” Ecological Modelling 227: 112–116. doi:10.1016/j.ecolmodel.2011.12.015.
- Benn, B., and S. Herrero. 2002. “Grizzly Bear Mortality and Human Access in Banff and Yoho National Parks, 1971–98.” Ursus 13: 213–221.
- Berland, A., T. Nelson, G. Stenhouse, K. Graham, and J. Cranston. 2008. “The Impact of Landscape Disturbance on Grizzly Bear Habitat Use in the Foothills Model Forest, Alberta, Canada.” Forest Ecology and Management 256 (11): 1875–1883. doi:10.1016/j.foreco.2008.07.019.
- Boulanger, J., M. Cattet, S. E. Nielsen, G. Stenhouse, and J. Cranston. 2013. “Use of Multi-State Models to Explore Relationships between Changes in Body Condition, Habitat and Survival of Grizzly Bears Ursus Arctos Horribilis.” Wildlife Biology 19 (3): 274–288. doi:10.2981/12-088.
- Bourbonnais, M. L., T. A. Nelson, M. R. Cattet, C. T. Darimont, and G. B. Stenhouse. 2013. “Spatial Analysis of Factors Influencing Long-Term Stress in the Grizzly Bear (Ursus Arctos) Population of Alberta, Canada.” PLoS ONE 8 (12): e83768. doi:10.1371/journal.pone.0083768.
- Brillinger, D. R., H. K. Preisler, A. A. Ager, and J. G. Kie. 2004. “An Exploratory Data Analysis (EDA) of the Paths of Moving Animals.” Journal of Statistical Planning and Inference 122 (1–2): 43–63. doi:10.1016/j.jspi.2003.06.016.
- Bullard, F. 1999. “Estimating the Home Range of an Animal: A Brownian Bridge Approach.” Master of Science, University of North Carolina, Chapel Hill.
- Burt, W. H. 1943. “Territoriality and Home Range Concepts as Applied to Mammals.” Journal of Mammalogy 24: 346–352. doi:10.2307/1374834.
- Calenge, C., S. Dray, and M. Royer-Carenzi. 2009. “The Concept of Animals’ Trajectories from a Data Analysis Perspective.” Ecological Informatics 4: 34–41. doi:10.1016/j.ecoinf.2008.10.002.
- Chapman, D. S., C. Dytham, and G. S. Oxford. 2007. “Landscape and Fine-Scale Movements of a Leaf Beetle: The Importance of Boundary Behaviour.” Oecologia 154 (1): 55–64. doi:10.1007/s00442-007-0806-z.
- Chruszcz, B., A. P. Clevenger, K. E. Gunson, and M. L. Gibeau. 2003. “Relationships among Grizzly Bears, Highways, and Habitat in the Banff-Bow Valley, Alberta, Canada.” Canadian Journal of Zoology 81: 1378–1391. doi:10.1139/z03-123.
- Dodge, S., R. Weibel, and A.-K. Lautenschütz. 2008. “Towards a Taxonomy of Movement Patterns.” Information Visualization 7 (3–4): 240–252. doi:10.1057/palgrave.ivs.9500182.
- Downs, J. A. 2010. “Time-Geographic Density Estimation for Moving Point Objects.” Lecture Notes in Computer Science 6292: 16–26. doi:10.1007/978-3-642-15300-6_2.
- Downs, J. A., M. W. Horner, and A. D. Tucker. 2011. “Time-Geographic Density Estimation for Home Range Analysis.” Annals of GIS 17: 163–171. doi:10.1080/19475683.2011.602023.
- Eberhardt, L. L., B. M. Blanchard, and R. R. Knight. 1994. “Population Trend of the Yellowstone Grizzly Bear as Estimated from Reproductive and Survival Rates.” Canadian Journal of Zoology 72: 360–363. doi:10.1139/z94-049.
- Edwards, M. A., A. E. Derocher, K. A. Hobson, M. Branigan, and J. A. Nagy. 2011. “Fast Carnivores and Slow Herbivores: Differential Foraging Strategies among Grizzly Bears in the Canadian Arctic.” Oecologia 165: 877–889. doi:10.1007/s00442-010-1869-9.
- Forman, R. T. T., and L. E. Alexander. 1998. “Roads and Their Major Ecological Effects.” Annual Review of Ecology and Systematics 29: 207–231. doi:10.1146/annurev.ecolsys.29.1.207.
- Frair, J. L., J. Fieberg, M. Hebblewhite, F. Cagnacci, N. J. DeCesare, and L. Pedrotti. 2010. “Resolving Issues of Imprecise and Habitat-Biased Locations in Ecological Analyses Using GPS Telemetry Data.” Philosophical Transactions of the Royal Society B: Biological Sciences 365 (1550): 2187–2200. doi:10.1098/rstb.2010.0084.
- Getis, A., and B. Boots. 1978. Models of Spatial Processes. Cambridge: Cambridge University Press.
- Gitzen, R. A., J. J. Millspaugh, and B. J. Kernohan. 2006. “Bandwidth Selection for Fixed-Kernel Analysis of Animal Utilization Distributions.” Journal of Wildlife Management 70: 1334–1344. doi:10.2193/0022-541X(2006)70[1334:BSFFAO]2.0.CO;2.
- Graham, K., J. Boulanger, J. Duval, and G. Stenhouse. 2010. “Spatial and Temporal Use of Roads by Grizzly Bears in West-Central Alberta.” Ursus 21: 43–56. doi:10.2192/09GR010.1.
- Hägerstraand, T. 1970. “What about People in Regional Science?” Papers in Regional Science 24: 7–24. doi:10.1111/j.1435-5597.1970.tb01464.x.
- Horne, J. S., E. O. Garton, S. M. Krone, and J. S. Lewis. 2007. “Analyzing Animal Movements Using Brownian Bridges.” Ecology 88: 2354–2363. doi:10.1890/06-0957.1.
- Hunter, A. 2007. “Sensor-Based Animal Tracking.” PhD diss., Department of Geomatics Engineering, University of Calgary.
- Jankowski, P., N. Andrienko, G. Andrienko, and S. Kisilevich. 2010. “Discovering Landmark Preferences and Movement Patterns from Photo Postings.” Transactions in GIS 14 (6): 833–852. doi:10.1111/j.1467-9671.2010.01235.x.
- Langrock, R., R. King, J. Matthiopoulos, L. Thomas, D. Fortin, and J. M. Morales. 2012. “Flexible and Practical Modeling of Animal Telemetry Data: Hidden Markov Models and Extensions.” Ecology 93 (11): 2336–2342. doi:10.1890/11-2241.1.
- Laube, P., and R. Purves. 2011. “How Fast Is a Cow? Cross-Scale Analysis of Movement Data.” Transactions in GIS 15: 401–418. doi:10.1111/j.1467-9671.2011.01256.x.
- Long, J. A., and T. A. Nelson. 2012. “Time Geography and Wildlife Home Range Delineation.” The Journal of Wildlife Management 76 (2): 407–413. doi:10.1002/jwmg.259.
- Long, J. A., and T. A. Nelson. 2013. “A Review of Quantitative Methods for Movement Data.” International Journal of Geographical Information Science 27 (2): 292–318. doi:10.1080/13658816.2012.682578.
- Mattson, D. J., R. R. Knight, and B. M. Blanchard. 1987. “The Effects of Developments and Primary Roads on Grizzly Bear Habitat Use in Yellowstone National Park, Wyoming.” International Conference of Bear Research and Management 7: 259–273.
- Morales, J. M., D. T. Haydon, J. Frair, K. E. Holsinger, and J. M. Fryxell. 2004. “Extracting More Out of Relocation Data: Building Movement Models as Mixtures of Random Walks.” Ecology 85 (9): 2436–2445. doi:10.1890/03-0269.
- Mueller, C., S. Herrero, and M. L. Gibeau. 2004. “Distribution of Subadult Grizzly Bears in Relation to Human Development in the Bow River Watershed, Alberta.” Ursus 15 (1): 35–47. doi:10.2192/1537-6176(2004)015<0035:DOSGBI>2.0.CO;2.
- Nelson, T. A. 2012. “Trends in Spatial Statistics.” The Professional Geographer 64 (1): 83–94.
- Nelson, T. A., and B. Boots. 2008. “Detecting Spatial Hot Spots in Landscape Ecology.” Ecography 31 (5): 556–566.
- Nielsen, S. E., S. Herrero, M. S. Boyce, B. Benn, R. D. Mace, M. L. Gibeau, and S. Jevons. 2004. “Modelling the Spatial Distribution of Human-Caused Grizzly Bear Mortalities in the Central Rockies Ecosystem of Canada.” Biological Conservation 120: 101–113. doi:10.1016/j.biocon.2004.02.020.
- Orellana, D., and M. Wachowicz. 2011. “Exploring Patterns of Movement Suspension in Pedestrian Mobility.” Geographical Analysis 43: 241–260. doi:10.1111/j.1538-4632.2011.00818.x.
- Phillips, M. K. 1987. “Behavior and Habitat Use of Grizzly Bears in Northeastern Alaska.” International Conference on Bear Research and Management 7: 159–167.
- Proctor, M. F., B. N. McLellan, C. Strobeck, and R. M. R. Barclay. 2004. “Gender-Specific Dispersal Distances of Grizzly Bears Estimated by Genetic Analysis.” Canadian Journal of Zoology 82: 1108–1118. doi:10.1139/z04-077.
- Rempel, R. S., A. R. Rodgers, and K. F. Abraham. 1995. “Performance of a GPS Animal Location System Under Boreal Forest Canopy.” The Journal of Wildlife Management 59: 543–551. doi:10.2307/3802461.
- Rode, K. D., C. T. Robbins, and L. A. Shipley. 2001. “Constraints on Herbivory by Grizzly Bears.” Oecologia 128: 62–71. doi:10.1007/s004420100637.
- Roever, C. L., M. S. Boyce, and G. B. Stenhouse. 2008. “Grizzly Bears and Forestry II: Grizzly Bear Habitat Selection and Conflicts With Road Placement.” Forest Ecology and Management 256: 1262–1269. doi:10.1016/j.foreco.2008.06.006.
- Samuel, M. D., and R. E. Green. 1988. “A Revised Test Procedure for Identifying Core Areas within the Home Range.” The Journal of Animal Ecology 57 (3): 1067–1068. doi:10.2307/5112.
- Sawyer, H., and M. J. Kauffman. 2011. “Stopover Ecology of a Migratory Ungulate.” Journal of Animal Ecology 80: 1078–1087. doi:10.1111/j.1365-2656.2011.01845.x.
- Schneider, R. R. 2002. Alternative Futures: Alberta’s Boreal Forest at the Crossroads. Edmonton: The Federation of Alberta Naturalists.
- Seaman, D. E., J. J. Millspaugh, B. J. Kernohan, G. C. Brundige, K. J. Raedeke, and R. A. Gitzen. 1999. “Effects of Sample Size on Kernel Home Range Estimates.” The Journal of Wildlife Management 63 (2): 739–747. doi:10.2307/3802664.
- Smulders, M., T. A. Nelson, D. E. Jelinski, S. E. Nielsen, and G. B. Stenhouse. 2010. “A Spatially Explicit Method for Evaluating Accuracy of Species Distribution Models.” Diversity and Distributions 16 (6): 996–1008. doi:10.1111/j.1472-4642.2010.00707.x.
- Smulders, M., T. A. Nelson, D. E. Jelinski, S. E. Nielsen, G. B. Stenhouse, and K. Laberee. 2012. “Quantifying Spatial–Temporal Patterns in Wildlife Ranges Using STAMP: A Grizzly Bear Example.” Applied Geography 35 (1–2): 124–131. doi:10.1016/j.apgeog.2012.06.009.
- Stenhouse, G. B., and R. H. M. Munro. 2000. Foothills Model Forest Grizzly Bear Research Program – 1999 Annual Report. Hinton: Foothills Model Forest.
- Stewart, B. P., T. A. Nelson, K. Laberee, S. E. Nielsen, M. A. Wulder, and G. B. Stenhouse. 2013. “Quantifying Grizzly Bear Selection of Natural and Anthropogenic Edges.” The Journal of Wildlife Management. doi:10.1002/jwmg.535.
- Thériault, M., C. Claramunt, and P. Villeneuve. 1999. “A Spatio-Temporal Taxonomy for the Representation of Spatial Set Behaviours.” In Spatio-Temporal Database Management, LNCS, International Workshop STDBM’99, Edinburgh, September 10–11, edited by M. H. Böhlen, C. S. Jensen, and M. O. Scholl, Vol. 1678, 1–19. Berlin: Springer-Verlag.
- Tremblay, Y., S. A. Shaffer, S. L. Fowler, C. E. Kuhn, B. I. McDonald, M. J. Weise, C.-A. Bost, et al. 2006. “Interpolation of Animal Tracking Data in a Fluid Environment.” Journal of Experimental Biology 209 (1): 128–140. doi:10.1242/jeb.01970.
- Turchin, P. 1986. “Modelling the Effect of Host Patch Size on Mexican Bean Beetle Emigration.” Ecology 67 (1): 124–132. doi:10.2307/1938510.
- White, J. C., M. A. Wulder, C. Gómez, and G. B. Stenhouse. 2011. “A History of Habitat Dynamics: Characterizing 35 Years of Stand Replacing Disturbance.” Canadian Journal of Remote Sensing 37 (2): 234–225. doi:10.5589/m11-034.
- Wiens, J. A. 1989. “Spatial Scaling in Ecology.” Functional Ecology 3: 385–397. doi:10.2307/2389612.
- Worton, B. J. 1987. “A Review of Models of Home Range for Animal Movement.” Ecological Modelling 38 (3–4): 277–298. doi:10.1016/0304-3800(87)90101-3.