Abstract
The ability to replicate, or reproduce, research is fundamental to the scientific process. Research combining a variety of georeferenced data is spreading rapidly across scientific domains and international borders. This suggests a growing potential for the use and integration of new and existing data sets to create new multi-disciplinary scientific collaborations. Yet, the unique characteristics of georeferenced data present special challenges to such collaborations. These data are highly identifiable when presented in maps and other visualizations or when combined with sensor data or other related geospatial data sets. The potential opportunities of collaboration may thus be constrained by the need to protect the locational privacy (geoprivacy) and confidentiality of subjects in research using georeferenced data. This paper reviews the obstacles to and potential methods for sharing georeferenced data in order to support a growing and dynamic geospatial research community and build capacity for data-intensive research across the social and environmental sciences. The development and implementation of a geospatial virtual data enclave methodology is proposed as an innovative and viable solution to share and archive georeferenced data among researchers while protecting the geoprivacy of research subjects and the confidentiality of these data. The ability to share confidential geospatial data among researchers is crucial to ensuring replicability of scientific research, and to enable researchers to verify and build upon the research of others.
1. Introduction
The ability to replicate and reproduce research is a cornerstone of the scientific method (McNutt Citation2014). Research combining a variety of intensive geographically referenced data streams is now spreading across many scientific domains, ranging from environmental science to transportation to epidemiology. Several contemporary trends are driving this research ranging from massive quantities of data streaming to data warehouses from global positioning systems (GPS)-enabled devices, sensors, and location-aware technologies, to advances in web services and cyberinfrastructure, to new geoprocessing tools for analysing, exploring, and visualizing large, multi-scale spatiotemporal data sets (Richardson Citation2013).
Figure 1. Point aggregation: assigning 100 points to pre-defined point locations in a unit square: (a) original pattern and (b) aggregated pattern.
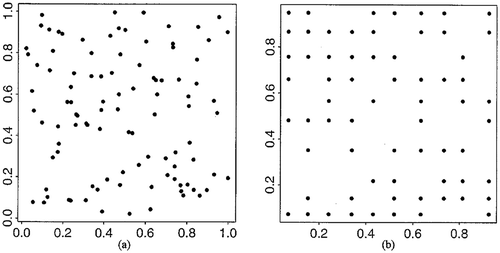
These trends suggest a growing and exciting potential for the use and integration of new and existing data sets to create multi-disciplinary and data-intensive scientific collaborations. Yet, the unique characteristics of georeferenced data present special data sharing challenges to such collaborations, and to replication of geospatial research. These data are highly identifiable when presented in maps and other visualizations or when combined with sensor data or other related geospatial data sets. The potential opportunities and benefits of collaboration are constrained by the need to protect the locational privacy (geoprivacy) and confidentiality of subjects in research using georeferenced data.
This paper reviews the obstacles to and potential methods for sharing georeferenced data among geospatial researchers in order to support a growing and dynamic international geospatial research community and build capacity for data-intensive research across the social and environmental sciences. First, the unique confidential characteristics of large georeferenced data sets (including geospatial cyberinfrastructure and ‘big’ data) are discussed together with viable ways to manipulate these data and their geovisualizations to protect confidentiality and privacy of research subjects. Second, current methods and procedures are examined that have been used to assess and reduce disclosure risks in maps, conduct statistical analyses, and produce other research products derived from locationally identifiable data. Third, the development and implementation a geospatial virtual data enclave (GVDE) methodology for sharing and archiving confidential georeferenced research data and protecting the privacy of research subjects is proposed. The GVDE methodology would provide a secure server and protocols that enable researchers to store and conduct sophisticated analyses of sensitive geospatial data, share that data with other researchers, and apply geographic masking technologies that, once evaluated for disclosure risk, allow for publication of data and maps without disclosing sensitive information about individuals or groups. Finally, the paper argues that this approach offers the potential for innovative and viable solutions to share and archive georeferenced data among researchers while protecting the geoprivacy of research subjects and the confidentiality of these data. It can contribute to the formation of research communities by facilitating access to data and data sharing that has heretofore been difficult or impossible to access and use without sacrificing confidentiality.
2. Confidentiality issues associated with georeferenced data
In recent years, advances in geographic information system (GIS) as tools for the storage, retrieval, manipulation, analysis, and display of spatial data have led to the collection and use of enormous amounts of more precise and accurate data that also contain information on geographic locations. Georeferenced data are increasingly rich in attributes and available, from street networks to remotely sensed images, from land parcel data to disease outbreaks, and more (Richardson et al. Citation2013). Location and spatial patterns, processes, and relationships can offer new insights in the search for answers to important questions, particularly as the capabilities to link social survey data with geographic data improve (VanWey et al. Citation2005; Gutmann et al. Citation2008). Spatial analysis and mapping of georeferenced individual-level data can help identify important geographic patterns or lead to significant knowledge for dealing with specific social issues (Kwan Citation2000, Citation2004; Thomas, Richardson, and Cheung Citation2008).
However, disclosure of the locations of subjects’ homes, workplaces, daily activities, or trips may lead to serious negative consequences (Dobson and Fisher Citation2003; Curtis, Mills, and Leitner Citation2006a). This is especially true in research on public health or social issues (see Boulos, Curtis, and AbdelMalik Citation2009 for an overview of locational privacy in biomedical and public health research literature). For example, there is considerable interest in the effect of neighbourhood on crime, delinquency, and immigrant assimilation (Simcha-Fagan and Schwartz Citation1986; Brooksgunn et al. Citation1993; Sampson, Morenoff, and Earls Citation1999; Sampson, Morenoff, and Gannon-Rowley Citation2002; Jackson, Pebley, and Goldman Citation2010; Sastry and Pebley Citation2010; Mennis et al. Citation2011; Mennis and Mason Citation2012). Complex data collections, such as the Project on Human Development in Chicago Neighborhoods and the Los Angeles Family and Neighborhood Survey (L.A. FANS) have been designed to provide the geospatial detail needed for this kind of research. These developments, however, often raise important issues about protecting the privacy of individuals’ characteristics, attitudes, and behaviours that have been associated with precise – ‘spatially explicit’ – locations (Onsrud, Johnson, and Lopez Citation1994; Kwan, Casas, and Schmitz Citation2004; VanWey et al. Citation2005; Gutmann et al. Citation2008). Georeferenced coordinates are highly identifiable, and individuals become even more identifiable when data about them and/or their itineraries (e.g., travel to work) are available.
With the capabilities of GIS to integrate and analyse a large amount of georeferenced data and the increasing use of web-based services and cyberGIS in compiling and delivering data (Richardson Citation2006), the potential of GIS to be far more invasive of personal privacy than many other information technologies has caused serious concern among privacy advocates, GIS researchers, and the public (Onsrud, Johnson, and Lopez Citation1994; Cutter, Richardson, and Wilbanks Citation2003; Armstrong and Ruggles Citation2005; Blumberg and Eckersly Citation2009). The need to protect individual privacy is particularly acute because of the ethical and legal implications of disclosure of sensitive data. When a statistical population can be sufficiently narrowed to the point where one individual can be identified, statistical disclosure has been achieved (Fellegi Citation1972). Disclosure has the potential to violate personal privacy and data confidentiality.
Different types of location data are often collected in social research. For example, collecting continuous space-time coordinates of people’s daily lives using GPS has become more common in recent years. Location data about people’s activities and trips are also collected in many social surveys (e.g., the L.A. FANS dataset has subjects’ activity locations). Access to high-resolution, remotely sensed imagery has also become commonplace. Imagery can be readily combined with population data, along with locational information related to land use, ownership and household or individual characteristics collected from social surveys (see Liverman et al. Citation1998). Such locational data can be sensitive and carry considerable disclosure risk. For example, how would maps showing the locations (and addresses) of HIV-infected persons affect people living in a small community?
Different types of location data and personal information are also collected by government agencies and private companies and compiled into large databases, increasingly using ‘location-aware’ (geolocation) technologies and location-based services facilitated by GPS technology (see, for example, Armstrong and Ruggles Citation2005; Cottrill Citation2011). Blumberg and Eckersly (Citation2009) illustrate the extent and potential of these activities with location-aware technologies such as monthly transit swipe cards, electronic tolling devices (and congestion pricing), cellphones, swipe cards to open doors, parking metres that can text you when time is running out, activity trackers which collect bio-markers such as heart rate, and services reporting what friends are near. Web technologies and social media have helped complement these data collection efforts by encouraging individuals to reveal personal information linked to specific geographic locations (geo-tagged tweets are one example).
In such a data-intensive environment, researchers using georeferenced data face several challenges. First, disclosure of detailed locational data is often illegal. Many countries have laws which govern data collection and its disclosure. On the one hand, they frequently give statistical agencies or researchers the right to collect information. On the other hand, they often require that the agency or the researcher not disclose such data in any way (Fellegi Citation1972). In the USA, the Privacy Act of 1974 attempted to ensure that only authorized and necessary data are collected by federal agencies and that this collection is done in a manner that would ‘preclude unwarranted intrusions upon individual privacy’ (Gordis and Gold Citation1980). In addition to the various legal obligations statistical agencies must abide by, many make additional representations or guarantees to respondents regarding the confidentiality of the data collected, often in an effort to obtain better response rates. For a summary of US privacy laws, including location privacy, see Pomfret (Citation2012).
Second, disclosure can be unethical, especially when a study involves sensitive issues or human subjects that are ‘hidden, secret or concealed’ (Brown Citation2000, 62), since disclosing their identities or locations through mapping may put them at unforeseeable risk. Disclosure is also unethical when respondents have been previously guaranteed confidentiality. Under current Institutional Review Board (IRB) requirements at universities, researchers cannot display analytical results or maps that may lead to re-identification of subjects. These include illustrations that show the locations of subjects’ homes or workplaces (e.g., precise plots of individual space-time paths constructed with GPS data).
Third, while researchers may apply protections to the data and maps they release in response to the legal and ethical requirements described above, it is still possible that maps and other geovisualizations may unintentionally include enough information to overcome those protections. Few studies have systematically evaluated the risk of disclosure or the effectiveness of protection methods. Tests using techniques to ‘reverse-geocode’ locations on maps provide examples of potential problems. Reverse geocoding is a reverse engineering process through which the actual address of a point location in the geographic database is identified, leading to the identification of the home, work, or activity address of the subject and possibly the identification of the subject (see, for example, Armstrong and Ruggles Citation2005; Brownstein, Cassa, and Mandl Citation2006; Curtis, Mills, and Leitner Citation2006a). Curtis, Mills, and Leitner (Citation2006a), using a newspaper map of body-recovery locations published in New Orleans after Hurricane Katrina, tested the potential for this ‘reverse engineering’. Even though the map was published without a road network, other features allowed them to georeference the maps, restore the road network, and locate specific houses. Ironically, geocoding has had some unintentional built-in protections due to errors (completeness and positional error) (Zandbergen Citation2009). However, as the accuracy of geocoding techniques improve, the chance of successfully re-engineering mapped locations has also improved (Boulos, Curtis, and AbdelMalik Citation2009). As a result, these researchers are calling for standards and guidelines for the display of maps derived from georeferenced data that require privacy protections (Curtis, Mills, and Leitner Citation2006b; Boulos, Curtis, and AbdelMalik Citation2009).
In addition, the potential unintended privacy consequences of matching data and maps published by researchers with large private or public sector databases described above have yet to be explored fully:
The danger of public release of information is made more complicated by the rapid increase in computing power available to all, which means that efforts at data matching and data mining that would have been unattainable … a few years ago are no longer outside the realm of possibility. (Gutmann et al. Citation2008, 647)
For example, address information can be potentially linked to these other databases, revealing personal information that was intended to be protected. Importantly, federal laws that deal with privacy have limited applicability to private firms (Cottrill Citation2011). Two recent reports issued by the US government provide an overview of current policy and technological issues relating to data privacy (Executive Office of the President Citation2014; President’s Council of Advisors on Science and Technology Citation2014).
3. Limiting disclosure risk and protecting geoprivacy: current status
There are several ways in which geoprivacy may be protected when georeferenced individual-level data are involved, ranging from regulations to specific methodologies. One approach is through more elaborate and stricter government regulation. Legislation that seeks to protect individual privacy, however, may hinder non-intrusive and socially desirable uses of georeferenced data. Onsrud, Johnson, and Lopez (Citation1994) proposed self-regulation as a possible solution to the problem and provided a set of privacy protection guidelines. Based on a similar approach, the Urban and Regional Information Systems Association (URISA) released a ‘GIS Code of Ethics’ that provides principles and guidelines for protecting individual privacy when using GIS (URISA Citation2003). The National Science Foundation (NSF) supported Oregon State University, Penn State University, the University of Minnesota and the Association of American Geographers (AAG) from 2007 to 2009 in a project to develop and institute graduate seminars that rigorously explored the ethical implications of geographic information science and technology (GIS&T). Open educational resources designed and produced by a team of professional ethicists and GIS&T educators from this research project are available at http://gisprofessionalethics.org.
Besides federal law that regulates the collection and release of data collected by government agencies (and self-regulation), there are human subject protection procedures implemented by IRBs of academic institutions. While IRBs review, approve and monitor the collection and use of data in academic research involving human subjects, it is not unusual within an IRB to encounter confusion about the risks of disclosure with respect to maps and other geospatial output (Boulos, Curtis, and AbdelMalik Citation2009).
Several methods are also available to modify or hide the original location information embedded in maps or geovisualizations generated using georeferenced data. Geographic masks add stochastic or deterministic noise to the original data matrix through modifying the geographic coordinates of the data points (Armstrong, Rushton, and Zimmerman Citation1999; Kwan, Casas, and Schmitz Citation2004; Armstrong and Ruggles Citation2005; Leitner and Curtis Citation2006; Gutmann et al. Citation2008; Cottrill Citation2011), or what computer scientists call ‘obfuscation’ (Krumm Citation2009). Masking techniques hide the original location associated with particular attributes or data (e.g., data of the household or individuals at that point). By geographically masking all locations in a data set, researchers can still use illustrations that include the locations of subjects’ homes or workplaces in their maps or geovisualizations when publishing their results, while protecting the geoprivacy of the individuals represented by those points.
Examples of geographic masking methods include: aggregation, affine transformations, random perturbation, and the donut method (Armstrong, Rushton, and Zimmerman Citation1999; Kwan, Casas, and Schmitz Citation2004; Chen, Rushton, and Smith Citation2008; Zimmerman, Armstrong, and Rushton Citation2008; Hampton et al. Citation2010). Aggregation masks the original location through organizing data by areal units, assigning multiple individual records to one point location, or generating aggregate pattern using individual data (see ). Areal aggregations can also be achieved by using regionalization methods that construct larger areas with population or attributes more similar than census units or zip code areas (Wang, Guo, and McLafferty Citation2012; Mu et al. Citation2015). In affine transformations, the scale of the point pattern may be changed, the point locations may be shifted a determined distance and direction, or the distribution may be rotated around a chosen point a certain number of degrees (see ). A random perturbation mask allows both the amount and direction of spatial displacement to vary between points (see ). The donut method is an extension of random perturbation in which each point is relocated in a random direction by at least a minimum distance but less than a maximum distance (Hampton et al. Citation2010).
Figure 2. Examples of affine transformations: (a) rescaling (top) and (b) rotation (bottom).
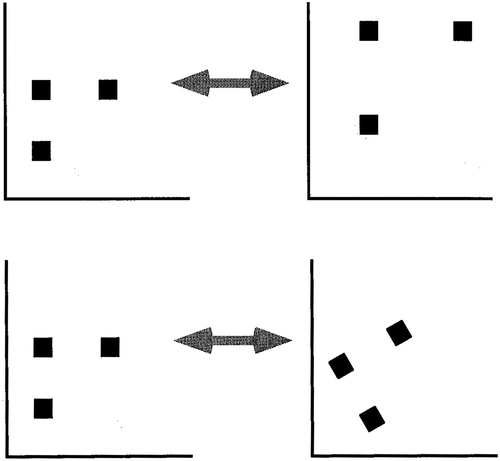
Figure 3. 3D density surfaces of masked data of the geographical distribution of lung cancer deaths in Franklin County, Ohio in 1999. Left: 3D surface obtained with circular mask. Right: 3D surface obtained with weighted mask.
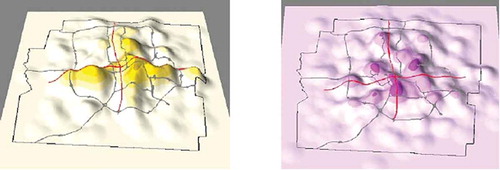
Geographic masking techniques may be applied either to the data before analysis or to the products of research (e.g. maps) after analysis. From a data sharing perspective, researchers strongly prefer post-analysis masking. Since all masking procedures change the data in some way, pre-analysis masking may affect the results of the data analysis when analysis of georeferenced individual-level data could help identify important geographical patterns. Restricted access to individual-level data may leave many needs for understanding critical social issues unfulfilled and constrain opportunities for replication of scientific studies, as well as comparative or longitudinal studies. Few studies have examined the extent to which analytical results are affected by different methods of geoprivacy protection (especially when precise geographic locations are modified with geographic masking methods).
In this paper, we propose an alternative method to provide data for analysis in a secure environment. We call this method the GVDE, which helps researchers develop and implement procedures for accessing and sharing georeferenced data in ways that offer adequate protection of geoprivacy and confidentiality, and provides guidance on and procedures for re-distributing, re-using, and publishing georeferenced data. It will also help researchers formulate robust plans for securely archiving data and survey samples while allowing interested researchers to access and use them. We suggest that this approach has the potential to support NSF researchers in meeting the data dissemination and sharing requirements of NSF projects, helping them to prepare and implement the data management plans of their NSF projects, and providing NSF with new options to evaluate and understand data management plans involving geospatial data. IRBs will also benefit when researchers are able to demonstrate clearly how human subjects will be protected through such a GVDE methodology.
4. The GVDE methodology
While previous studies on methods for protecting geoprivacy exist (e.g., Armstrong, Rushton, and Zimmerman Citation1999; Kwan, Casas, and Schmitz Citation2004; Armstrong and Ruggles Citation2005; Zimmerman, Armstrong, and Rushton Citation2008), few studies have systematically evaluated the risk of disclosure or the effectiveness of protection these methods offer. We propose the GVDE methodology as an alternative for addressing these issues more systematically and thoroughly.
Since aggregation by fairly large administrative units (e.g., county, census tracts) is a common and established practice for protecting geoprivacy, the method we conceive here also focuses on the use of data sets that include street addresses or geographic coordinate locations at high positional accuracy (e.g., GPS data). This proof of concept research specifically addresses the following questions within the context of the GVDE: (1) What kinds of geographic masking methods (e.g., random perturbation) are suitable for which kinds of data? (2) What are the trade-offs between geoprivacy protection and accuracy of analytical results? Specifically, what values of the masking parameters offer reasonable level of geoprivacy protection without significantly affecting the results? (3) What values of the masking parameters for different masking methods can most effectively reduce the possibility of reverse geocoding (e.g., the bandwidth to use in kernel density estimation)? (4) What kind of cartographic output or maps (e.g., the standard deviational ellipse (SDE) or the kernel density surface (KDS), and at what scale, can be released publicly to researchers or used in publications? (5) What are the computing and physical constraints and limitations for conducting advanced geospatial analyses within the geospatial digital enclave environment?
The GVDE is designed to allow researchers to access and analyse georeferenced data while protecting the confidentiality of subjects. In a virtual data enclave (VDE), all data and analysis take place on a server in a secure data centre. Users of the VDE open a connection to a ‘Virtual Machine’ (VM) running on a server in the data centre, and communicate with the VM via client software installed on their local computer. The VM is isolated from the user’s physical desktop computer, restricting the user from downloading files or parts of files to their physical computer. The VM is also restricted in its external access, preventing users from emailing, ftp’ing, copying, or otherwise moving files outside of the secure environment, either accidentally or intentionally. The secure environment has high-level access controls and firewalls in place to prevent unauthorized access to data from outside sources, and communication between the data centre and the user is encrypted. The GVDE approach also has significant advantages in terms of accessibility, cost, and ongoing usage over existing methods which require researchers to travel to secured physical sites in order to view or access confidential data.
The VDE presents the user with a familiar desktop operating system with all the functionality of a standard PC, but computing occurs on the server rather than the local machine. Users perform their analyses as they normally would, and they obtain output by depositing it in a ‘drop box’, where it can be checked for disclosure risks before being sent to them. Systems of this sort have been developed in the USA and a number of countries for access to data underlying government statistics. But this paper, to the best of our knowledge, is the first that conceives the application of this technology in light of the unique challenges of georeferenced identifiable data.
This GVDE methodology supports a wide range of statistical and spatial analysis techniques that are used by researchers in various disciplines: social statistics, spatial statistical techniques for area-based data, and geospatial methods for point-based data. Examples of the kinds of analysis that can be supported include the following.
4.1. Social statistics
Since summary statistics and statistical results normally do not reveal the identity of subjects or any locational information that risks violating geoprivacy, all commonly used social statistical techniques can be supported in the GVDE. These include multiple regression, principal component analysis, cluster analysis, factor analysis, multidimensional scaling, discriminant analysis, contingency table analysis, general linear models, survival analysis, log-linear models, multi-level models, and structural equation models.
4.2. Spatial statistical techniques for area-based data
Since area-based geographic data are already aggregated at different spatial scales (e.g., county, census tracts), all geospatial analytical techniques for area data can be supported in the GVDE together with procedures to ensure that the aggregation level is adequate for geoprivacy protection. These techniques include various area-based measures of spatial association and spatial cluster analysis methods (e.g., Anselin’s (Citation1995) local indicator of spatial association (LISA), Moran’s I, Geary’s C, and Getis’s Gi), and a suite of spatial regression models.
4.3. Geospatial methods for point-based and linear data
Researchers in various disciplines now collect detailed space-time data of subjects using GPS and other location-aware devices (e.g., smart phones) (e.g., Wiehe et al. Citation2008; Maddison et al. Citation2010; Rainham et al. Citation2010; Troped et al. Citation2010; Zenk et al. Citation2011). These data sets contain accurate and continuous locational information that can be used to construct the daily space-time trajectory of the subject, and thus can be used to identify the subject and the location of his/her home, workplace, and daily activities. An individual’s activity space, for example, is the area containing all georeferenced locations with which an individual has direct contact as a result of the sequence of his or her activities. Activity spaces can be generated and revealed using the SDE, the KDS, and the potential path area (PPA) methods. The SDE captures the geographic distribution or directional trend of a series of points (Yuill Citation1971; Arcury et al. Citation2005; Wong and Lee Citation2005; Rainham et al. Citation2010). The KDS is a density surface (e.g., population density) derived from the location of a set of points using a kernel function and a predetermined search radius (or bandwidth). The PPA is the area that a subject can reach given his or her daily space-time constraint (e.g., fixed locations like workplace) (Kwan Citation1998, Citation1999).
Since point-based and linear geographic data can reveal the location of subjects’ home, workplace, or activity locations, these data sets pose particularly high risks of disclosure and geoprivacy violations. Many spatial statistical methods that use point-based data are available that generate results that normally do not reveal the original point locations of the data set. These methods include geographically weighted regression, kriging, spatial point pattern analysis, spatial cluster analysis, the spatial scan statistic, kernel density estimation, and the K function. However, in desired data sharing situations, researchers may need to see the original point locations during the analytical process (e.g., to assess visually the spatial distribution of the points). The GVDE thus accommodates a set of procedures for visualizing these point locations while preventing disclosure of the identity of the subjects, masking the point locations and evaluating cartographic output to examine the effect of different parameters (e.g., bandwidth and impedance functions that model the effect of distance decay) on disclosure risks, and testing the robustness of these procedures and deciding the level of protection required to prevent the possibility of reverse geocoding.
5. Implementation
The Inter-university Consortium for Political and Social Research (ICPSR) at the University of Michigan has developed a VDE system for use with other forms of data. The AAG and the ICPSR have received an NSF-funded joint project to apply their complementary research expertise to develop and implement the GVDE methodology outlined in the previous section. The project is developing solutions to long-standing problems of sharing geospatial data and their visualizations, and building capacity for data-intensive geospatial research across the social and environmental sciences.
Specifically, research on the following areas has been conducted through this AAGICPSR joint project: (1) research on the unique confidential characteristics of large georeferenced data sets (including issues associated with geospatial cyberinfrastructure and ‘big’ data), and on viable ways to manipulate these data and their geovisualizations to protect confidentiality and privacy of research subjects; (2) research on methods and procedures to assess and reduce disclosure risks in maps, statistical analyses, and other research projects derived from locationally identifiable data; (3) research regarding the viability of sharing and archiving confidential georeferenced research data, including the exploration, development, and testing of cost-effective and potentially far-reaching procedures and technology using a VDE to enable sophisticated analyses of these data under conditions that protect the privacy of research subjects; and (4) engaging representatives from multiple research communities that utilize georeferenced data to (a) intensively test confidentiality methods and procedures within the GVDE to reduce disclosure risk and (b) assist in developing standards and specifications for disclosure review that can be further tested to address the unique characteristics of georeferenced data.
To evaluate the GVDE, researchers from multiple geospatial research communities which deal with georeferenced data and regularly encounter privacy and confidentiality issues uniquely related to geographic information and data have been recruited to test it. They are working within the GVDE to analyse different kinds of georeferenced data sets; apply and work with different masking techniques and potentially identify customized software extensions or applications that are needed; and identify, explore, test, and assess the range of different visualization outputs or extractions related to disclosure risk. Their usage patterns are monitored and their feedback, experiences, and needs regularly recorded. Input from this research community is helping to formulate training materials for future users of the GVDE system and methods.
Sample use cases for the GVDE include many geographic and geospatial technology tools and data collection practices which generate questions about locational privacy and confidentiality such as research involving personal travel itineraries, geographical information and data related to health (including m-health initiatives), or those involving minors or at risk populations, among many others.
6. Conclusion
Advances in GIS and related technologies have provided new and increasingly powerful tools for researchers to visualize and analyse a wide range of phenomena, and they are particularly useful for integrating data across domains of the natural and social sciences. New research designs are being developed to take advantage of the ubiquity of devices with integrated real-time interactive global positioning system and geographic information system capabilities and new geolocation technologies for both mobile and environmental sensors. These developments, however, raise important issues about protecting the privacy of subjects or other individuals who have provided confidential information. Georeferenced coordinates are highly identifiable, and individuals become even more identifiable when their individual locations (e.g., home or work) and itineraries (e.g., travel to work) are available. These issues are further complicated by the existence of large and growing corporate databases that capture and store real-time locational information about individuals and their surroundings that can be mined and matched with research data.
The inherent identifiability of georeferenced data makes it difficult for researchers to share their data, which reduces scientific replication opportunities, secondary analysis, and increases the costs of research. Restrictions on the re-use of data are particularly burdensome for students and early-career researchers, who may not have the resources to collect data themselves. The innovative GVDE methodology described in this paper (a) provides researchers with full and secure access to unmodified georeferenced data, while assuring the protection of human subjects, (b) enables publication of research results (e.g., maps) to which disclosure protections have been applied, and (c) allows researchers to satisfy both human subject protections guidelines and NSF data management and data sharing requirements. Unlike alternative approaches, which involve introducing noise or creating simulated data prior to release of sensitive data (and hinder collaboration), researchers using the GVDE may gain access to unmodified georeferenced data. The confidentiality of subjects can be protected while sharing data among researchers by developing and applying disclosure protection methods and standards to the products of research – maps and other geovisualizations – rather than to the inputs to the research process.
The GVDE methodology described in this paper and the ongoing AAG and ICPSR research program will contribute to the development and implementation of a new methodology for sharing and using confidential georeferenced data in a wide variety of research contexts. These contributions include: (1) advances in understanding the unique confidential features of large georeferenced data sets and how to analyse and manipulate these data and their geovisualizations to protect confidentiality and geoprivacy of research subjects; (2) progress in developing procedures and standards to assess and reduce disclosure risks in georeferenced data, maps, statistical analyses, and other research products derived from locationally identifiable data with input from research communities that utilize geospatial data; (3) development and testing of a GVDE system designed specifically for analysis of georeferenced data; (4) outlining of training materials needed to help educate researchers about ways that re-identification of subjects can occur and safe ways to present their results and protect georeferenced information; (5) review of the requirements of NSF data management plans in order to inform our current research regarding researchers’ needs in preparing these plans; and (6) development of a sustainable model for building scalable and fully operational VDE facilities for georeferenced data at ICPSR.
Further, specific application areas are poised to benefit from the development and use of the GVDE methodology in light of their current research trajectories. These areas include, for example, the increasing use of geographic technologies in health research and the growing awareness of the importance of geographic context to health behaviour and outcomes (Kwan Citation2012). As disclosure protection is extended to a wide array of analytical methods beyond static output, the potential to develop new geovisualization tools that are interactive or dynamic (screens/slices) will be greatly enhanced. The development of automatic disclosure protection for real-time spatiotemporal data will enable the use of these data in many real-time or near real-time applications, including crowdsourcing. New opportunities also exist for integrating research developments around the GVDE methodology with emerging cyberinfrastructure research agendas to meet the needs of researchers to share and archive these confidential data and to enable researchers around the world to replicate and build upon scientific research involving geospatial data.
Disclosure statement
No potential conflict of interest was reported by the authors. The content is solely the responsibility of the authors and does not necessarily represent the official views of the NIH or the NSF.
Acknowledgements
We would like to thank and acknowledge permission to reprint and from John Wiley & Sons, Ltd. (Copyright © 1999) and Figure 3 from University of Toronto Press (Copyright © 2004).
Additional information
Funding
References
- Anselin, L. 1995. “Local Indicators of Spatial Association – LISA.” Geographical Analysis 27 (2): 93–115. doi:10.1111/j.1538-4632.1995.tb00338.x.
- Arcury, T. A., W. M. Gesler, J. S. Preisser, J. Sherman, J. Spencer, and J. Perin. 2005. “The Effects of Geography and Spatial Behavior on Health Care Utilization among the Residents of a Rural Region.” Health Services Research 40 (1): 135–156. doi:10.1111/j.1475-6773.2005.00346.x.
- Armstrong, M. P., and A. J. Ruggles. 2005. “Geographic Information Technologies and Personal Privacy.” Cartographica: The International Journal for Geographic Information and Geovisualization 40 (4): 63–73. doi:10.3138/RU65-81R3-0W75-8V21.
- Armstrong, M. P., G. Rushton, and D. L. Zimmerman. 1999. “Geographically Masking Health Data to Preserve Confidentiality.” Statistics in Medicine 18: 497–525. doi:10.1002/(SICI)1097-0258(19990315)18:5<497::AID-SIM45>3.0.CO;2-#.
- Blumberg, A. J., and P. Eckersley. 2009. “On Locational Privacy, and How to Avoid Losing it Forever.” Accessed May 5, 2012. http://www.eff.org/wp/locational_privacy (Electronic Frontier Foundation).
- Boulos, M. N. K., A. J. Curtis, and P. AbdelMalik. 2009. “Musings on Privacy Issues in Health Research Involving Disaggregate Geographic Data about Individuals.” International Journal of Health Geographics 8: 46. doi:10.1186/1476-072X-8-46.
- Brooksgunn, J., G. J. Duncan, P. K. Klebanov, and N. Sealand. 1993. “Do Neighborhoods Influence Child and Adolescent Development?” American Journal of Sociology 99 (2): 353–395. doi:10.1086/230268.
- Brown, M. P. 2000. Closet Space: Geographies of Metaphor from the Body to the Globe. London: Routledge.
- Brownstein, J. S., C. A. Cassa, and K. D. Mandl. 2006. “No Place to Hide – Reverse Identification of Patients from Published Maps.” New England Journal of Medicine 355 (16): 1741–1742. doi:10.1056/NEJMc061891.
- Chen, Z., G. Rushton, and G. Smith. 2008. “Preserving Privacy: Deidentifying Data by Applying a Random Perturbation Spatial Mask.” In Geocoding Health Data: The Use of Geographic Codes in Cancer Prevention and Control, Research, and Practice, edited by G. Rushton, M. P. Armstrong, J. Gittler, B. R. Greene, C. E. Pavlik, M. M. West, and D. L. Zimmerman, 139–146. Boca Raton, FL: CRC Press.
- Cottrill, C. D. 2011. “Location Privacy: Who Protects?” URISA Journal 23 (2): 49–59.
- Curtis, A. J., J. W. Mills, and M. Leitner. 2006a. “Spatial Confidentiality and GIS: Re-Engineering Mortality Locations from Published Maps about Hurricane Katrina.” International Journal of Health Geographics 5: 44. doi:10.1186/1476-072X-5-44.
- Curtis, A. J., J. W. Mills, and M. Leitner. 2006b. “Keeping an Eye on Privacy Issues with Geospatial Data.” Nature 441: 150. doi:10.1038/441150d.
- Cutter, S., D. Richardson, and T. Wilbanks, eds. 2003. The Geographical Dimensions of Terrorism. New York: Routledge.
- Dobson, J. E., and P. F. Fisher. 2003. “Geoslavery.” IEEE Technology and Society Magazine Spring 22: 47–52. doi:10.1109/MTAS.2003.1188276.
- Executive Office of the President. 2014. “Big Data: Seizing Opportunities, Preserving Values.” http://www.whitehouse.gov/sites/default/files/docs/big_data_privacy_report_may_1_2014.pdf.
- Fellegi, I. P. 1972. “On the Question of Statistical Confidentiality.” Journal of the American Statistical Association 67 (337): 7–18. doi:10.1080/01621459.1972.10481199.
- Gordis, L., and E. Gold. 1980. “Privacy, Confidentiality, and the Use of Medical Records in Research.” Science 207 (4427): 153–156. doi:10.1126/science.7350648.
- Gutmann, M. P., K. Witkowski, C. Colyer, J. M. O’Rourke, and J. McNally. 2008. “Providing Spatial Data for Secondary Analysis: Issues and Current Practices Relating to Confidentiality.” Population Research and Policy Review 27: 639–665. doi:10.1007/s11113-008-9095-4.
- Hampton, K. H., M. K. Fitch, W. B. Allshouse, I. A. Doherty, D. C. Gesink, P. A. Leone, M. L. Serre, and W. C. Miller. 2010. “Mapping Health Data: Improved Privacy Protection with Donut Method Geomasking.” American Journal of Epidemiology 172 (9): 1062–1069. doi:10.1093/aje/kwq248.
- Jackson, M. I., A. R. Pebley, and N. Goldman. 2010. “Schooling Location and Economic, Occupational and Cognitive Success among Immigrants and Their Children: The Case of Los Angeles.” Social Science Research 39 (3): 432–443. doi:10.1016/j.ssresearch.2009.11.001.
- Krumm, J. 2009. “A Survey of Computational Location Privacy.” Personal and Ubiquitous Computing 13: 391–399. doi:10.1007/s00779-008-0212-5.
- Kwan, M.-P. 1998. “Space-Time and Integral Measures of Individual Accessibility: A Comparative Analysis Using a Point-Based Framework.” Geographical Analysis 30 (3): 191–216. doi:10.1111/j.1538-4632.1998.tb00396.x.
- Kwan, M.-P. 1999. “Gender and Individual Access to Urban Opportunities: A Study Using Space-Time Measures.” The Professional Geographer 51 (2): 211–227. doi:10.1111/0033-0124.00158.
- Kwan, M.-P. 2000. “Interactive Geovisualization of Activity-Travel Patterns Using Three-Dimensional Geographical Information Systems: A Methodological Exploration with a Large Data Set.” Transportation Research Part C: Emerging Technologies 8: 185–203. doi:10.1016/S0968-090X(00)00017-6.
- Kwan, M.-P. 2004. “GIS Methods in Time-Geographic Research: Geocomputation and Geovisualization of Human Activity Patterns.” Geografiska Annaler B 86 (4): 267–280. doi:10.1111/j.0435-3684.2004.00167.x.
- Kwan, M.-P. 2012. “The Uncertain Geographic Context Problem.” Annals of the Association of American Geographers 102 (5): 958–968. doi:10.1080/00045608.2012.687349.
- Kwan, M.-P., I. Casas, and B. C. Schmitz. 2004. “Protection of Geoprivacy and Accuracy of Spatial Information: How Effective are Geographical Masks?” Cartographica: The International Journal for Geographic Information and Geovisualization 39 (2): 15–28. doi:10.3138/X204-4223-57MK-8273.
- Leitner, M., and A. Curtis. 2006. “A First Step Towards A Framework for Presenting the Location of Confidential Point Data on Maps – Results of an Empirical Perceptual Study.” International Journal of Geographical Information Science 20 (7): 813–822. doi:10.1080/13658810600711261.
- Liverman, D., E. F. Moran, R. R. Rindfuss, and P. C. Stern, eds. 1998. People and Pixels: Linking Remote Sensing and Social Science. Washington, DC: National Academy Press.
- Maddison, R., Y. Jiang, S. Vander Hoorn, D. Exeter, C. N. Mhurchu, and E. Dorey. 2010. “Describing Patterns of Physical Activity in Adolescents Using Global Positioning Systems and Accelerometry.” Pediatric Exercise Science 22 (3): 392–407.
- McNutt, M. 2014. “Editorial: Reproducibility.” Science 343 (6168): 229. doi:10.1126/science.1250475.
- Mennis, J., P. W. Harris, Z. Obradovic, A. J. Izenman, H. E. Grunwald, and B. Lockwood. 2011. “The Effect of Neighborhood Characteristics and Spatial Spillover on Urban Juvenile Delinquency and Recidivism.” The Professional Geographer 63 (2): 174–192. doi:10.1080/00330124.2010.547149.
- Mennis, J., and M. J. Mason. 2012. “Social and Geographic Contexts of Adolescent Substance Use: The Moderating Effects of Age and Gender.” Social Networks 34 (1): 150–157.
- Mu, L., F. Wang, V. W. Chen, and X.-C. Wu. 2015. “A Place-Oriented, Mixed-Level Regionalization Method for Constructing Geographic Areas in Health Data Dissemination and Analysis.” Annals of the Association of American Geographers 105 (1): 48–66. doi:10.1080/00045608.2014.968910
- Onsrud, H. J., J. Johnson, and X. Lopez. 1994. “Protecting Personal Privacy in Using Geographic Information Systems.” Photogrammetric Engineering and Remote Sensing 60 (9): 1083–1085.
- Pomfret, K. 2012. “Summary of Location Privacy in the United States.” In Geographic Data and the Law: Defining New Challenges, edited by K. Janssen and J. Crompvoets, 77–90. Leuven: Leuven University Press.
- President’s Council of Advisors on Science and Technology. 2014. “Report to the President: Big Data and Privacy: A Technological Perspective.” http://www.whitehouse.gov/sites/default/files/microsites/ostp/PCAST/pcast_big_data_and_privacy_-_may_2014.pdf
- Rainham, D., I. McDowell, D. Krewski, and M. Sawada. 2010. “Conceptualizing the Healthscape: Contributions of Time Geography, Location Technologies and Spatial Ecology to Place and Health Research.” Social Science & Medicine 70 (5): 668–676. doi:10.1016/j.socscimed.2009.10.035.
- Richardson, D. B. 2006. “GIS&T: Transforming Science and Society.” In Geographic Information Science & Technology: Body of Knowledge, edited by D. DiBiase, M. DeMers, A. Johnson, K. Kemp, A. T. Luck, B. Plewe, and E. Wentz, vii–x. Washington, DC: Association of American Geographers.
- Richardson, D. B. 2013. “Real-Time Space-Time Integration in Giscience and Geography.” Annals of the Association of American Geographers 103 (5): 1062–1071. doi:10.1080/00045608.2013.792172.
- Richardson, D. B., N. D. Volkow, M.-P. Kwan, R. M. Kaplan, M. F. Goodchild, and R. T. Croyle. 2013. “Spatial Turn in Health Research.” Science 339: 1390–1392. doi:10.1126/science.1232257.
- Sampson, R. J., J. D. Morenoff, and F. Earls. 1999. “Beyond Social Capital: Spatial Dynamics of Collective Efficacy for Children.” American Sociological Review 64 (5): 633–660. doi:10.2307/2657367.
- Sampson, R. J., J. D. Morenoff, and T. Gannon-Rowley. 2002. “Assessing ‘Neighborhood Effects’: Social Processes and New Directions in Research.” Annual Review of Sociology 28: 443–478. doi:10.1146/annurev.soc.28.110601.141114.
- Sastry, N., and A. R. Pebley. 2010. “Family and Neighborhood Sources of Socioeconomic Inequality in Children’s Achievement.” Demography 47 (3): 777–800. doi:10.1353/dem.0.0114.
- Simcha-Fagan, O., and J. E. Schwartz. 1986. “Neighborhood and Delinquency: An Assessment of Contextual Effects.” Criminology 24 (4): 667–699. doi:10.1111/j.1745-9125.1986.tb01507.x.
- Thomas, Y., D. B. Richardson, and I. Cheung, eds. 2008. Geography and Drug Addiction. Dordrecht: Springer.
- Troped, P. J., J. S. Wilson, C. E. Matthews, E. K. Cromley, and S. J. Melly. 2010. “The Built Environment and Location-Based Physical Activity.” American Journal of Preventive Medicine 38 (4): 429–438. doi:10.1016/j.amepre.2009.12.032.
- URISA (Urban and Regional Information Systems Association). 2003. “A GIS Code of Ethics.” WWW Document. http://www.urisa.org/about/ethics
- VanWey, L. K., R. Rindfuss, M. P. Gutmann, B. Entwisle, and D. L. Balk. 2005. “Confidentiality and Spatially Explicit Data: Concerns and Challenges.” Proceedings of the National Academy of Sciences 102 (43): 15337–15342. doi:10.1073/pnas.0507804102.
- Wang, F., D. Guo, and S. McLafferty. 2012. “Constructing Geographic Areas for Cancer Data Analysis: A Case Study on Late-Stage Breast Cancer Risk in Illinois.” Applied Geography 35: 1–11. doi:10.1016/j.apgeog.2012.04.005.
- Wiehe, S. E., A. E. Carroll, G. C. Liu, K. L. Haberkorn, S. C. Hoch, J. S. Wilson, and J. D. Fortenberry. 2008. “Using GPS-Enabled Cell Phones to Track the Travel Patterns of Adolescents”. International Journal of Health Geographics 7:22.
- Wong, D., and J. Lee. 2005. Statistical Analysis of Geographic Information with ArcView GIS and ArcGIS. Hoboken, NJ: John Wiley & Sons.
- Yuill, R. S. 1971. “The Standard Deviational Ellipse; an Updated Tool for Spatial Description.” Geografiska Annaler: Series B, Human Geography 53 (1): 28–39. doi:10.2307/490885.
- Zandbergen, P. A. 2009. “Geocoding Quality and Implications for Spatial Analysis.” Geography Compass 3 (2): 647–680. doi:10.1111/j.1749-8198.2008.00205.x.
- Zenk, S. N., A. J. Schulz, S. A. Matthews, A. Odoms-Young, J. Wilbur, L. Wegrzyn, K. Gibbs, C. Braunschweig, and C. Stokes. 2011. “Activity Space Environment and Dietary and Physical Activity Behaviors: A Pilot Study.” Health & Place 17 (5): 1150–1161. doi:10.1016/j.healthplace.2011.05.001.
- Zimmerman, D. L., M. P. Armstrong, and G. Rushton. 2008. “Alternative Techniques for Masking Geographic Detail to Protect Privacy.” In Geocoding Health Data: The Use of Geographic Codes in Cancer Prevention and Control, Research, and Practice, edited by G. Rushton, M. P. Armstrong, J. Gittler, B. R. Greene, C. E. Pavlik, M. M. West, and D. L. Zimmerman, 127–138. Boca Raton, FL: CRC Press.