ABSTRACT
Different types of spatial analyses and visualizations can be used in the police practice for investigation, crime prediction, and planning of police forces. The public availability of crime data is one of the often discussed issues for the police, general public and academia. The efforts to open police data are rooted in the philosophy of the so-called ‘community policing’. In this article, we demonstrate the possibilities of spatial analysis and cartographic visualization of open crime data. We provide two use cases based on the data gathered by the municipal police in Pardubice, Czech Republic. We investigate the impact of gambling sites on crime offence intensity and found that gambling sites considerably influence their surroundings within 100 m. The other use case is focused on traffic offences caused by cyclists. We extracted hot spots of these offences and tried to identify their causation, since the police should not only carry out repressive measures, but also strive to eliminate the causes (e.g. add cycle lanes, bike paths, underpasses or overpasses). Different types of cartographic visualization have been designed and discussed for both use cases. The advantages, limitations and future development of the described concepts are commented on in the conclusion.
1. Introduction
Data on criminal offences collected by the police have considerable potential for spatial analysis. The analysis helps to improve the performance of the police, raise public awareness about local issues, and is used as an input for decision-making processes within the public administration. The advantage of using cartographic visualizations becomes apparent when communicating the information to the public. Text or audio visual messages are still preferred in the Czech Republic for transferring information on crime, both in the websites of Czech police and individual municipalities. In some cases, the involvement of public within these user interfaces is possible in the form of inserting anonymous information (e.g. in Kolín through the project Bezpečný Kolín www.bezpecnykolin.cz). However, this communication channel fails to offer an explicit expression of spatial components of given criminal acts. Therefore, the participation of public can be lower than in the case of direct cartographic visualization of these phenomena, which represents an interface integrating both components of the information (spatial and attributed).
Despite an ongoing development of open data access within the public administration in the Czech Republic (Fond Otakara Motejla Citation2015), the official crime statistics are not publicly available due to legislative constraints. The delivery of full statistics is not settled even between the state and municipal police forces and only simple statistical data without the spatial component and the victim and perpetrator details are exchanged. On the other hand, local police forces have a legal option to share selected information with the general public. Local police forces are also responsible for investigating minor crimes and for maintenance of an offence database. The Czech government adopted new legislation for the development of an offence register in 2015. This register will be centralized and shared among municipalities in order to help reduce minor crimes. The offences have only been registered on the local level without a centralized database. Habitual offenders were able to move without taking risks of accumulating their offences committed in different spatial locations and their potential punishments. The planned register will be a rich source of information for minor crime statistics and an active support tool for the decision-making process at courts. Several cities and towns (Pardubice, Kolín) already have criminal offence data available in the form of open data. For these reasons, we believe that police data should be, with certain limitations, distributed as open data, freely available to everyone to be used and republished, without restrictions from copyright, patents or other control mechanisms.
2. Related work
The police forces used different types of analyses and visualizations (including cartographic visualizations) in the past, when technological tools allowing their effective creation (geographic information systems, etc.) were unavailable. Those analyses were performed particularly for studying social processes and spatial contexts within some segments of population (e.g. impact of poverty, Boba Citation2001). According to Weisburd and McEwen (Citation1998) and Harries (Citation1999), the early days of mapping criminality can already be found at the beginning of the nineteenth century, even though until the twentieth century these only concerned simple visualizations of crime occurrence. At that time, the so-called pin method was frequently used. This method captured an occurrence of certain types of criminal acts mostly in a large-scale map. Only as late as in the 1960s and 1970s, the technological progress allowed the first computer-generated maps, which also dealt with criminal issues (Boba Citation2001). Similarly to other fields, the first more complex solutions started to appear in crime investigation only with the further development of geographic information systems in the 1990s. At that time most of the cases included simple static visualizations in the computer environment without any interactivity or interoperability by the police forces or public.
Chainey and Ratcliffe (Citation2013) discussed several criminological theories when describing how spatial factors influence offenders and victims. The crime pattern theory is mentioned as the one of potential relevance for geographic information system (GIS) researchers. Crime pattern theory suggests that offenders are influenced by daily activities and routines of their lives, and even if they search for a criminal opportunity, they tend to act in areas that are known to them (Brantingham and Brantingham Citation1984). This theory also introduces the terms, such as crime generator and attractors, the latter as a site attracting criminal opportunities. Following this theory, a group of studies focused on the role of facilities as crime generators and examined the crime in the vicinity (Groff Citation2011; Ratcliffe Citation2012). Thanks to the increasing availability of geolocated criminal statistics and the ability to perform very detailed studies, several investigations also explored whether the influence of a facility (bar, shopping centre, casino) diminishes with the distance or remains unchanged (Kumar and Waylor Citation2003; Ratcliffe Citation2011, Citation2012; Rengert, Ratcliffe, and Chakravorty Citation2005).
The mapping and analysis of crime investigation is based on an assumption that given types of criminal acts most frequently occur in typologically similar parts of towns. The mapping of these locations and further modelling can be used for a relatively reliable detection of potential locations with increased probability of crime and for potential remedial measures (Tošovský et al. Citation2010; Weisburd Citation2008). Furthermore, Weisburd (Citation2008) adds that, based on external reasons, a given locality contains sites with higher number of criminal act, i.e. the number of sites suitable for crime is lower than the number of crime offenders. These sites form so-called hotspots, i.e. sites with high crime occurrence. Up to a certain extent, this fact has an impact on the suitability of cartographic methods to visualize crime.
An overview of major applications of geoinformation technology in crime analysis and prediction was provided by several authors (Chainey and Ratcliffe Citation2013; Eck et al. Citation2008; Inspektor, Ivan, and Horák Citation2014; Wang Citation2012), mainly focusing on the United States (US) and the United Kingdom (UK) ground. Lately, Wang (Citation2012) discussed six major areas of GIS applications in law enforcements including crime investigation and prevention, policy implementation, and communication as those relevant for our article. In conclusions, Wang stressed the role of a close collaboration between academia and law enforcement professionals as one of the ‘areas with much work to do’.
The public availability of crime statistics and their possible use for analytical tasks is one of the often discussed issues both for the general public and academia. The police are aware of the fact that crime mapping is an effective tool for cooperation with the public, but there are still substantial obstacles, both of legislative and technological nature, to an open access to all crime statistics data.
The call for opening police data is rooted in the philosophy of ‘community policing’. Community policing focuses on the police working closely with community members. Police officers are seen as members of the public and should not only be considered as a tool of repression. Analyses of data collected by the police can positively affect safety as well as the quality of life in municipalities. The police should not only cooperate directly with citizens, but also with other government agencies (Office of Community Oriented Policing Services Citation2014). On the other hand, we have to admit that community policing using spatial data has some limits and risks. The limits include organizational complexity, need for communication and building mutual trust between the stakeholders, lack of informative value of the used data, need for expansion of data and information sources and unreadiness of the police and the public for community policing. Potential risks include abuse of input statistics or created maps, insufficient implementation tools, which reduces efficiency and high economic demands of the full implementation of the concept of community policing.
Crime analysis and prediction is one of the fields benefiting from the concept of Open Data. The Open Data initiative was encouraged by government projects, such as the 2009 US government project Data.gov. This open approach is traditionally used in the US as well as in the UK and some others countries (Fond Otakara Motejla Citation2015). However, some privacy issues that should be taken into consideration, such as exact location of the crime or full identification of the victim and perpetrator, are treated differently. Tomson et al. (Citation2015) analysed the spatial accuracy of the UK police data and their suitability for analysis. These data were intentionally distorted using the geomasking concept in order to protect the location privacy of victims. The authors recommended the street segment level as the geographical resolution best suited for the open access database and also regarded the provision of open data as beneficial to the academic and other communities. Although the concept of open data is considerably newer than the philosophy of community policing, it is clear that open data can be also used within the community policing. The publication of data on crime mainly depends on the national legislation. The personal data (including address, name and photography) about offenders are for example published in the United States (CriminalCheck.com, Citation2016, see http://criminalcheck.com). However, we will focus on creating map layouts that utilize the principles of aggregation and anonymization of the input data. These outputs can also be published under an existence of legal or even ethical constraints.
Within the EU countries, Temelkovski et al. (Citation2012) exemplified the use of open data for crime analysis in the Republic of Macedonia using the daily bulletin of the Ministry of Internal Affairs as a data source. Using the natural language processing method, they managed to get a structured form of the data and stored them in a database containing the full description (the actual text from the bulletin) and localization of the event. Piloting the open data approach in Macedonia, this study also recommends to summarize and relate the events to a point of public interest (cinema, park, etc.) to avoid privacy issues.
Focusing on the territory of the Czech Republic, Hruška et al. (Citation2015) produced a comprehensive study analysing the possibilities of modern approaches and implementation tools in the field of crime mapping, crime analysis, crime prediction and law enforcement planning. The study provided a description and evaluation of costs associated with the application of these approaches and tools, along with their benefits. In addition, the authors compared several ongoing implementations of geoinformation technologies within the local police agencies (towns of Kolín, Uherské Hradiště and Pardubice) and demonstrated the current shift from simple data visualization to analytical tools development. Organizational and methodological recommendations given in the study stressed the necessity to control cooperation between the police and citizens on the municipality level, including regular public opinion polls on safety situation and Web-based information campaigns on preventive measures.
In this article, we demonstrate the possibilities of spatial analysis and cartographic visualization of open crime data. We provide two use cases based on the data collected by the municipal police in Pardubice, the tenth largest city in the Czech Republic. The location of Pardubice in the Czech Republic is shown in . The article is organized as follows. The pilot study overview and justification of use cases is briefly described in Section 3, including the basic statistics of minor crimes in the examined city (Pardubice). The next sections present two case studies dealing with the influence of gambling sites on minor crime and the spatial distribution and frequency of cyclists’ offences. Both Sections 4 and 5 are further structured into review, data, methods and results subsections. Section 6 discusses the case studies results and exemplifies the use of open data for community policing using different level of anonymization. The final section concludes the article and gives directions for future work.
3. Pilot study data overview
Minor crime statistics were collected and processed by the Municipal police of Pardubice. The dataset contains all reported incidents in the city in 2014 and was provided as open data in Excel database file format. Since the most severe crimes occurred outside of the terms of reference of the Municipal police (and are investigated by the Police of the Czech Republic), the dataset includes rather minor offences like thefts, property damage or driving offences. Each entry in the dataset is classified by the type of reported criminal offence with a more detailed description attached. The police also register how each event was resolved by intervening officers. The other columns store on-the-spot fines, address (street), date, time, and (most importantly for GIS analysis) spatial coordinates.
Although the validity of spatial data is manually confirmed on a subset of the offences, the accuracy of recorded coordinates could be further discussed. As Schenewerk (Citation2003) mentioned considering the public GPS devices, the standard accuracy deviation can vary from several centimetres up to several metres. On the other hand, several metre deviations are acceptable precision considering our use cases.
Based on the input dataset containing over 38,000 municipal police activities, interventions and recorded offences in Pardubice in 2014, we selected subsets for further analysis in two use cases (see Section 4). To assure a sufficient level of victim privacy, we only worked with a single spatial component of the events and the additional information was anonymized and classified into more general groups. Police activities and interventions represent, e.g. delivery of subpoenas, cooperation with segments of the integrated rescue system, control facilities for civil protection and other similar tasks.
More than half of the records in the input dataset were offences (approximately 24,500). These may be further classified according to type, as shown in . The most common type is traffic offences. Parking can also be considered as a special category of traffic violation; more than three quarters of all recorded offences are related to traffic. However, the remaining category of offences – against public safety – is usually more serious and therefore has a bigger social impact than more numerous traffic offences.
The first use case deals with the impact of gambling sites on minor crime occurrence. The negative impact of gambling is widely discussed, and the results of spatial analysis can provide a factual basis for policy-making aimed at mitigating this impact. The second use case relates to offences and accidents of cyclists. Pardubice has the highest number of cyclists per capita among Czech cities. The spatial analysis can improve public safety by allowing to predict the behaviour of cyclists, and also by revealing issues in the public infrastructure.
Most of the data processing was performed in ArcGIS 10.3 software. The software was used for data analysis and visualization. Analyses were performed using the tools in the Model Builder component of ArcGIS. We also used QGIS 2.6 software for the transformation from the input XLS file to a shapefile. Every selected record from the XLS was transformed into a point data set. The transformation was based on GPS coordinates from the XLS file (WGS84 coordinate system in decimal form). QGIS with MMQGIS plug-in was also used for georeferencing of gambling site addresses in the first use case.
4. Use case 1 – the impact of gambling sites on crime occurrence
Gambling is one of common and persistent social issues. The impacts of gambling addiction are often fatal and destructive for the gamblers. The influence of gambling sites on population was described many times from different points of view and various scientific researches and studies have been conducted. The studies focused on social capital (Barmaki Citation2010; Griswold and Nichols Citation2006), gambling prevalence amongst adolescents (Fischer Citation2001), effects of regulation (Hansen and Rossow Citation2010), accessibility (Robitaillle and Herjean Citation2008) or availability (Jacques, Ladouceur, and Ferland Citation2000) of gambling and video lottery terminals. The basic theory assumes that pathological gamblers with their inclination to debts, frustration, hopelessness and naivety tend to attract crime.
Following the crime pattern theory introduced above, the gambling sites represent the crime generators in the sense of Brantingham and Brantingham (Citation1995, p. 7). Since substantial number of gambling sites sell alcohol, we can see a parallel between the situation in the Czech Republic and several studies analysed by Groff and Lockwood (Citation2014), who identified a positive correlation between alcohol outlets (bars, pubs, hotels) and crime rate in their surroundings. While some of the analysed studies examined just the effects of facilities on adjacent areas (Bernasco and Block Citation2011; Roncek and Faggiani, Citation1985; Roncek and Maier Citation1991), researchers have only recently begun to question how the relationship between crime generators (criminogenic facilities) and crime might deviate as the distance from facilities increases. Studies of criminal patterns near bars determined that the number of criminal offences cluster within different distances starting from 325 ft, as defined by Ratcliffe (Citation2011, Citation2012), and reaching as far as 1200 ft measured on street segments by Groff and Lockwood (Citation2014).
Grinols, Mustartd, and Hunt (Citation2000) analysed the relationship between casinos and crime. The conclusion of the study was that casino increased occurrence of all crimes except murder, which was the crime with the least obvious connection to casinos. In addition, the impact of casinos on crime increased over time and began approximately 3 years after its opening.
The Czech Republic has seen a sharp growth of gambling in the past 20 years, which has not been met with an effective preventive response and regulation (Szczyrba et al. Citation2015). The Czech Republic legislation did not allow the presence of a gambling site within a 100-m distance from specified buildings, such as schools, hospitals or places of worship (Act No. 202/1990) before 2011. This distance was defined because of the well-known negative effects of gambling sites. However, the regulation neither defined whether this distance is measured from the entrance or perimeter walls of the building, nor whether the distance is the direct distance or the distance on the street network (Spolek JURISTIC Citation2001). This competence was transferred directly to the local government in 2012. A permit and licence for a casino, slot machine or roulette is granted by the Ministry of Finance of the Czech Republic and the list with locations of permitted gambling sites is legally provided by the Ministry of Finance. (Ministry of Finance, Citation2014).
Based on the statistics provided by the Ministry of Finance, there were over 57,000 gambling sites in the Czech Republic at the end of 2014 and 66 of them were located in the city of Pardubice (Ministry of Finance Citation2014). Establishments, such as sports bars or restaurants with slot machines, gaming machines or roulette, are often opened nonstop, which attracts a specific clientele prone to commit illegal activities. Such mix of socially excluded people and people concentrated in a single place can have a potentially dangerous effect on its surroundings. In this study, we focus on the impact of gambling sites on spatial distribution of crime incidents in the city of Pardubice, the Czech Republic. We attempt to provide a methodology for establishing a radius of a provable effect of a gambling site on minor crime rate (offences) in the neighbourhood. The research questions are as follows:
What types of minor crime are the most frequent near gambling sites?
How far do gambling sites affect their surroundings?
4.1 Data and methods
We combined open data of minor offences from the Municipal police in Pardubice with open data of gambling sites provided by the Ministry of Finance. This dataset, which is regularly updated by the Ministry of Finance, includes addresses, type of gaming and operator. We studied whether the offences registered in the dataset by the Municipal police of Pardubice are influenced by the vicinity of a gambling site.
Before we start to analyse the spatial impact of gambling sites, we need to define what we understand as their surroundings. As we noticed, this is the crucial parameter for further analysis. The selected criminal offences were analysed in the distance from 5 to 200 m from gambling sites with the use of concentric buffer zones (dissolved areas). The buffer step was, therefore, 5 m, so 49 buffers were created with the ArcGIS Model Builder (see ).
Figure 3. Model Builder scheme used for creating buffer layers and database files with frequency of intersected crimes.

The exported databases were then merged into a single .csv file with the use of a Python script (see the Appendix). In every zone, we examined the proportion of incidents of a selected crime type out of the total number of incidents in that particular buffer. The cumulative nature of our method of proximity analysis means that the larger buffers always contain all incidents from smaller buffers. This approach was chosen since assessing each 5-m ring separately would increase the probability of empty rings, which would in turn deteriorate visual evaluation of results. We determined the relative proportion of crime types against the distance from gambling sites, and visually examined the line graph to detect possible trends. We identified three offence types that appear to be influenced by proximity of a gambling site: theft, violation of noise ordinance and property damage (see ), whose results are quite similar to those described by Grinols, Mustard, and Hunt (Citation2000).
Figure 4. Spatial distribution of gambling sites and selected offences (property damage, violation of noise ordinance and thefts) in the city of Pardubice in 2014.
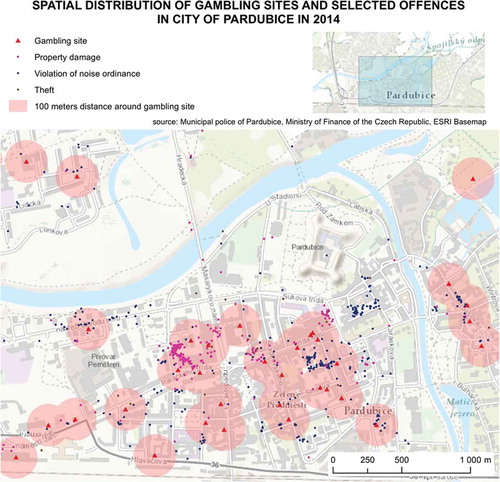
Figure 5. Correlation between value of distance for buffer and relative number of crimes for selected offence types.
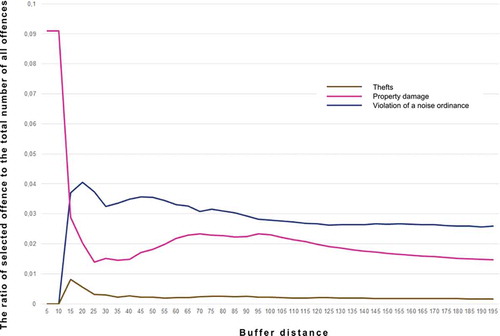
The number of these three types of offences, we analysed from the dataset from the city of Pardubice, are as follows: 68 thefts, 646 property damage offences and 688 violations of noise ordinance. The spatial distribution of gambling sites and the resulted offences in the city centre of Pardubice is shown in .
4.2 Results – gambling sites influence on their surrounding
As mentioned above, using concentric buffer zones we analysed how the proportion of selected offences (property damage, violation of noise ordinance and thefts) decreases with growing distance from gambling sites. In each buffer zone, we examined the proportion of incidents of selected offences to the total number of all offences in that buffer zone. Using relative numbers instead of the absolute number of offences compensates for differences between the social and environmental characteristics of gambling site neighbourhoods, such as varied population density. It also allows us to compare buffers of different radius without the need for area-correction used in an analogous method by Ratcliffe (Citation2012).
As we can see in , the value of the distance radius has a crucial effect on the structural distribution of crime types. The changes between radii with small distance values are quite notable. This could be caused by the relatively small number of recorded incidents in those buffers. The ratio in is naturally quite low because of the total amount of all offences in the city of Pardubice. The ratio of property damage starts along the y-axis at the highest value, which could be interpreted as coincidence of property damage inside gambling sites. If we dismiss the data from the shortest distances for insufficient reliability, the maximum violation of noise ordinance and thefts lies in distances around 15 m from gambling sites. Another threshold lies between 85 and 120 m where the number of incidents becomes independent on the distance from gambling sites.
Our findings confirm the validity of the 100-m restricted area around gambling sites regarding their negative impact. The threshold roughly corresponds with the 85–120-m influence radius we found in the input dataset. For the purpose of our analysis, we devised a method of concentric buffer zones to observe if the change in the relative number of crime incidences of different types depends on distance from gambling site. With this method, we identified three crime types that appear to be significantly influenced by the proximity of a gambling site: theft, violation of noise ordinance and property damage.
5. Use case 2 – traffic offences caused by cyclists
The city of Pardubice has the most cyclists in comparison to other Czech towns and cities with over 50,000 inhabitants, but the bicycle infrastructure is still poor comparing to other cities in Western Europe (Central MeetBike Citation2015). The idea of accident prevention is neither new nor unknown; for example Kjellén (Citation2000) studied the possibility of accident prevention by repeated accidents. The spatial analysis of traffic accidents is also described by Gundogdu (Citation2011). We have moved one step further so we try to find the main causes of traffic offences and use their analyses to propose solutions that would improve road traffic safety. The potential of evidence and spatial analysis of traffic law enforcement is briefly mentioned by Levine (Citation2008). However, the offences caused by cyclists have not yet been in the centre of spatial analysis by any extensive study. The research questions are as follows:
Where are the sites of offences caused by cyclists frequently localized?
What are the most common reasons for discontinuation of regulations for cyclists?
5.1 Data and methods
The input data were filtered since we only focus on offences caused by cyclists. We concentrate on spatial distribution of these offences, so we tried to produce a heat map and the number of offences in the street network to see the hot spots of offences and to propose possible solutions that can prevent the cause of offences. We used QGIS for the ‘XLS’ (specified in Section Data overview) to create a Shapefile and ArcGIS for final classification, application of filters, heat map analysis and creation of results. The newly created data were then imported into ArcGIS. The data were filtered by cyclist offences into a separate layer. Then we applied the ‘kernel density’ analysis on the separate layer. Kernel density estimation is a spatial method that calculates density of point or polyline features around area unit of output raster cell. We used point kernel density. It is one of the most popular methods for analysing a point event distribution since it is easy to understand and implement (Xie and Yan Citation2008). The density output is influenced by function parameters of cell size and search radius. The value of search radius determines the smoothness of the estimated density. The larger is the radius, the smoother the generalized density raster (Xie and Yan Citation2008). A neighbourhood is defined around each raster cell centre, and the number of points that fall within the neighbourhood is totalled and divided by the area of the neighbourhood. Parameterization is a significant advantage of kernel density estimation. In our study, we worked with a search radius of 400 m and output cell size of 10 m. Calculated kernel density for our problem area is shown in .
Figure 7. Heat map created using kernel density estimation depicting clusters of offences caused by cyclists.
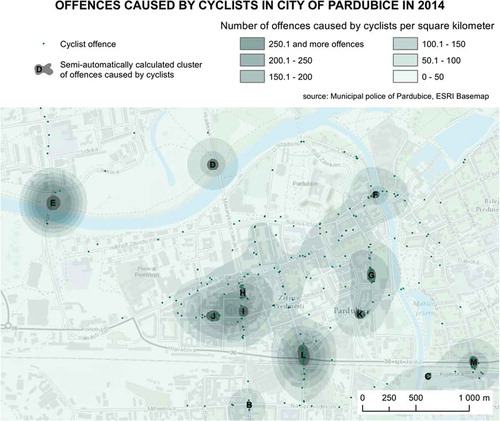
Clusters have been extracted semi-automatically from the heat map (). Workflow for the definition of clusters and extraction of particular points is shown in . This workflow was developed with ArcGIS Model Builder. Besides the kernel density parameters, the proper value of the threshold for reclassification needed to be chosen. The main purpose of this step was to distinguish sites related to cyclist offences. The threshold value was set to 0.002. This value is given by the range of the uppermost class, when the data is split into ten quantiles. The approach that uses quantiles is inspired by Chainey, Tompson, and Uhlig (Citation2008). In addition, classifications with fewer than 10 quantiles were tested, but they created large and inhomogeneous clusters. The output of the whole workflow (point layer) was exported to MS Excel and contingency tables were calculated. A list of extracted clusters is displayed in . The ratio of offence types was analysed across the clusters, and possible solutions were proposed. The distribution of clusters in the city centre is demonstrated in . Notice that the cluster A is located outside the city centre.
Table 1. List of identified clusters with description of most frequent offence types and proposal of possible solutions.
An alternative method to visualize point data is to use a regular grid (binning, hexbinning, fishnet). This type of segregation, regardless whether using hexagonal, rectangular or triangular grid, gives a more readable result comparing to the well-known point map method. As Scott (Citation1988) proposed, hexagons are the optimum shape for regular grids as they have the best effectiveness (defined as integrated mean squared error) and graphical perception. Triangles have lower effectiveness but are still useable for small samples. On the other hand, rectangles are easier to calculate and have only a slightly lower effectiveness than hexagons. Hexagons are more visually appealing and are much closer in effectiveness to circles for building a continuous and regular grid. The neighbouring edge is identical on each side and the distance between centroids is also identical in all six directions (comparing to rectangle four ‘edge neighbours’ and four ‘point neighbours’). The number of offences caused by cyclists was calculated for each hexagon, but only non-null hexagons are displayed in the map in . A single hexagon occupies approx. 0.29 ha. Similarly to the heat map, this method allows to locate clusters.
The parameters and the setting is the advantage of kernel density, which allows us to define the size of an area considered around each point. Grid count is generally one of the simplest methods of point data aggregation and visualization, since the traditional point map is often not very readable. This approach is very sensitive to the definition of grid cell size. There are other ways how to analyse point data and identify hotspots or clusters, but as Chainey and Ratcliffe (Citation2013) noted, the kernel density provides the best results in the description of spatial distribution. One of the dominating cyclists’ offences was cycling in forbidden zones, such as pavements or parks. We understand cyclists cross parks in various directions, so it is problematic to join these offences with specific streets for analysis. We tried to take advantage of streets at least by using street count shown in . Offences which occurred in a considerable distance from streets according to GPS were not considered. Another relatively common method for cluster analysis is Moran’s I statistics calculated primarily with polygons. Using our grid, regardless whether hexagonal or rectangular, we made polygon layer theoretically useable for Moran’s I statistics. In our case, the result would be significantly influenced by arbitrarily established grid cell size; therefore, we consider the result more suitable for analyses within specific boundaries, such as administrative units.
5.2 Results – cyclists’ offences
After the analysis, we combined separate results and tried to find possible cause and a solution for prevention of accidents and offences. As it can be seen in , there are several hot-spots in the centre of Pardubice. The main problem areas are marked with letters that provide reference to offences and solution in . The main idea behind this table is to give an overview of the most common offences, their number and proposed solutions.
demonstrates that the most frequent offences by cyclists are: riding without light, riding on pavement, riding on pedestrian crossing or in an area with prohibited entry for cyclists. For example 17. listopadu street that contains two hot spots (H and I) is a busy road that leads out of the city centre. There are car parks on both sides of the road but no cycle lanes. It is rather a dangerous road for cyclists to use. There are many common road accidents when parked cars return to the road. Therefore, the cyclists use pavements as safer paths. To increase safety, a cycle lane should definitely be built in this street. The hot spot J is located in the park. There are no bicycle lanes in this park and there are only one way roads around the park, which complicates the mobility of cyclists. Therefore, the cyclists use the park as an obvious shortcut. We can sum up that possible solutions for some offences are provided directly during the spatial analysis (hot spots C, G–M). However, finding appropriate spatial solutions can be problematic for other offences (in our case cyclists riding without lights). Although it might help to place appropriate road traffic signs on critical spots, it would be more effective to raise awareness of cyclists to make them ride with the light on.
6. Discussion
The proximity analysis method devised for the use case 1 is of cumulative nature, i.e. that larger buffers always contain all incidents from smaller buffers. This is not necessarily a drawback as we focus on the relative number of incidents, but as an aim for the further research it would be interesting to compare our approach with other proximity analysis methods. As we evaluated the change in relative proportion of crime types, we abstracted the analysed data from differing population densities across the city. With greater amount of input data, the visual examination of line graphs used in our method could be replaced with a regression analysis as it is used by similar buffer-based techniques (changepoint regression in Ratcliffe Citation2012; Poisson regression in Boyd et al. Citation2007). We are aware that the presence of gambling sites is just one of the possible factors that can influence the spatial distribution of incidents. A similar analysis could be made to assess the impact of other sites with potentially aggravating or mitigating effects (e.g. nightclubs or police stations).
In the use case 2, we identified a number of clusters with an increased number of cyclist offences that revealed issues in the public infrastructure, such as missing cycle lanes or underpasses. Maps similar to those in and can be used to back up requests for making the city traffic infrastructure more cycle friendly. That being said we should not forget that the input data are not a perfect abstraction of criminality in the city of Pardubice. Naturally, the events recorded in our dataset are just those reported, but still a substantial part of offences remain hidden to the police. Even though the clusters may seem as sites of the highest concentration of a particular offence, they could also simply represent places of the highest police activity.
With the large number of data points at the municipal scale a need arises for an effective visualization technique to present data to the public. When the raw data (large amount of points) are visualized directly, these data may not be correctly interpreted by laypersons. Both the heat map () and the hexagon method () proved to be apt techniques for this purpose. Such maps can be used by the police for investigation purposes (Wang Citation2012), or to plan the deployment of police forces – the so-called hot-spot policing (Weisburd Citation2005). From hot-spot policing there is just a step further to predictive policing for prevention and detection of emerging hot spots (Uchida Citation2010; McCue Citation2011).
The second reason why we can recommend the above mentioned methods of visualization is the variable position accuracy of the input data. Considering the public GPS devices, the standard accuracy deviation can vary from several centimetres up to several metres (Schenewerk Citation2003). The deviation itself can be in some cases corrected or just outlined through more schematic type of visualization.
Another reason for creating a cluster map is a need for anonymization that is often a prerequisite for publishing results of a crime related analysis as part of community policing. In certain cases, publishing the exact location of a criminal act can have negative side effects on people living in that locality. Anonymization is primarily motivated by ethical principles and legislative restrictions (e.g. publication of personal data about victims of crimes is limited). Anonymization can be achieved through aggregation and transfer to some existing areas (e.g. police districts) or to street segments or to other spatial object (e.g. cross roads, parks, railway stations). Similarly, it is possible to proceed with the data on mobile phone localization (see Řezník, Horáková and Szturc. Citation2015). Other possible methods for anonymization are based on adding positional uncertainty to geographical data, as it is defined and examined by Kubíček, Šašinka, and Stachoň (Citation2014). For example, random numbers (smaller than a predetermined value) can be added to or subtracted from coordinate values.
The methods and results presented in this article are consistent with the results of the project ‘Maps of the Future’ (Hruška et al. Citation2015), which states general recommendations for the use of spatial data in the context of community policing in the Czech Republic According to this project, crime maps should be available to the general public on the Web. It is recommended to use heat maps or grid mapping (using both rectangular and hexagonal grids), when analysing the data directly by the police and other experts. The data aggregated to larger areas, e.g. administrative units, to street lines or quantitative visualization as shown in , are recommended to be used for presentation of results.
7. Conclusions
Two use cases outlined in this article present just a small fraction of a wide range of options for using police open data. None of the above conclusions could have been made without an access to data at a sufficient level of detail. However, in the Czech Republic it is not common for the municipal police to have their data available for open use. The case of the city of Pardubice was a notable exception, and our use cases hope to encourage such practice by other municipal police departments. The police themselves have a limited staff capacity and data analysis is not the task of highest priority for them. Therefore, opening up data for researchers can generate in-depth insight compared to in-house analyses by police specialists. The usability of spatial data is also determined by the compliance with standardized formats and services. The process of integration of the spatial data into the European legislation is described by Řezník (Citation2013).
Furthermore, if the outputs from the analysis are publicly available, the information can reach more people. An informed community can make preventive actions to protect themselves and collaborate effectively with the police forces in line with the principles of community policing. The appropriate level of anonymization of crime incident data is still under discussion and will be influenced by the legislation and the context of use. Different approaches exemplified within our study served as levels of spatial granularity to be used for communication with the public.
Apart from the aforementioned visualization and analyses, time stamped open data can be used within virtual environments to explore a select crime phenomena (Subrahmanian and Dickerson Citation2009; Lin, Chen, and Lu Citation2013; Lin et al. Citation2015). Spatial patterns and correlations with other environmental variables can be simulated and predicted, and the proposed models can be further calibrated using historical data.
Acknowledgements
We would like to thank Róbert Cibula, Pavel Grochal, Josef Chrást and Daniel Vrbík for their collaboration. The authors also thank the two anonymous reviewers for their valuable comments and suggestions, which have been used to improve this article.
Disclosure statement
No potential conflict of interest was reported by the authors.
Additional information
Funding
References
- Barmaki, R. 2010. “Gambling as a Social Problem: On the Social Conditions of Gambling in Canada.“ Journal of Youth Studies 13 (1): 47–64.
- Bernasco, W., and R. Block. 2011. “Robberies in Chicago: A Block-level Analysis of the Influence of Crime Generators, Crime Attractors, and Offender Anchor Points.” Journal of Research in Crime and Delinquency 48: 33–57. doi:10.1177/0022427810384135.
- Boba, R. 2001. “Introductory Guide to Crime Analysis and Mapping.” Report to the Office of Community Oriented Policing Services. 74 p.
- Boyd, S. J., K. M. Armstrong, L. J. Fang, D. R. Medoff, L. B. Dixon, and D. A. Gorelick. 2007. “Use of a “Microecologic Technique” to Study Crime around Substance Abuse Treatment Centers.” Social Science Computer Review 25 (2): 163–173. doi:10.1177/0894439307298928.
- Brantingham, P. L., and P. J. Brantingham. 1984. Patterns in Crime. New York, NY: Macmillan.
- Brantingham, P. L., and P. J. Brantingham. 1995. “Criminality of Place: Crime Generators and Crime Attractors.” European Journal on Criminal Policy and Research 3: 5–26. doi:10.1007/BF02242925.
- Central MeetBike. 2015. “Pardubice v projektu Central MeetBike.“ Accessed December 8, 2015. http://www.cyklodoprava.cz/central-meetbike/partneri-v-cr
- Chainey, S., and J. Ratcliffe. 2013. GIS and Crime Mapping. John Wiley & Sons. 448. ISBN 9781118685198.
- Chainey, S., L. Tompson, and S. Uhlig. 2008. “The Utility of Hotspot Mapping for Predicting Spatial Patterns of Crime.” Security Journal 21: 4–28. doi:10.1057/palgrave.sj.8350066.
- CriminalCheck.com. 2016. “National Sex Offender Database Site.“ Accessed March 3, 2016. http://www.criminalcheck.com/
- Eck, J., S. Chainey, J. Cameron, M. Leitner, and R. E. Wilson. 2008. “Mapping Crime: Understanding Hotspots.” Washington, DC: National Institute of Justice. 79 p.
- Fischer, S. 2001. “A Prevalence Study of Gambling and Problem Gambling in British Adolescents.” Addiction Research & Theory 7 (6): 509–538. doi:10.3109/16066359909004403.
- Fond Otakara Motejla. 2015. “OtevřenáData.cz.” Accessed 10 December 2015 http://www.otevrenadata.cz.
- Grinols, E. L., D. B. Mustard, and D. C. Hunt. 2000. “Casinos, Crime and Community Costs.” Available at SSRN: http://ssrn.com/abstract=233792
- Griswold, M. T., and M. W. Nichols. 2006. “Social Capital and Casino Gambling in U.S. Communities.” Social Indicators Research 77 (3): 369–394. doi:10.1007/s11205-004-6337-7.
- Groff, E. R. 2011. “Exploring ‘Near’: Characterizing the Spatial Extent of Drinking Place Influence on Crime.” Australian & New Zealand Journal of Criminology 44: 156–179. doi:10.1177/0004865811405253.
- Groff, E. R., and B. Lockwood. 2014. “Criminogenic Facilities and Crime across Street Segments in Philadelphia: Uncovering Evidence About the Spatial Extent of Facility Influence.” Journal of Research in Crime and Delinquency 51 (3): 277–314. doi:10.1177/0022427813512494.
- Gundogdu, I. B. 2011. “A New Approach for GIS-Supported Mapping of Traffic Accidents.” Proceedings of the ICE - Transport 164 (2): 87–96. doi:10.1680/tran.2011.164.2.87.
- Hansen, M., and I. Rossow. 2010. “Limited Cash Flow on Slot Machines: Effects of Prohibition of Note Acceptors on Adolescent Gambling Behaviour.” International Journal of Mental Health & Addiction 8 (1): 70–81. doi:10.1007/s11469-009-9196-2.
- Harries, K. 1999. Mapping Crime: Principle and Practice. Washington DC: US Department of Justice.
- Hruška, L., I. Foldynová, I. Šotkovský, L. Zapletalová, T. Václavík, I. Žurovec, R. Fujak, and J. Ševčík. 2015. “Maps of the Future – an Innovative Crime-Prediction-Based Tool to Increase the Effectiveness and Quality of Public Administration Performance in Crime Prevention.” Available at SSRN: http://www.prevencekriminality.cz/evt_file.php?file=1298
- Inspektor, T., I. Ivan, and J. Horák. 2014. “Mapping and Monitoring Unemployment Hot Spots Towards Identification of Socially Excluded Localities: Case Study of Ostrava.” Journal of Maps 10 (1): 35–46. doi:10.1080/17445647.2013.847806.
- Jacques, C., R. Ladouceur, and F. Ferland. 2000. “Impact of Availability on Gambling: A Longitudinal Study.” Canadian Journal of Psychiatry 45 (9): 810–815.
- Kjellén, U. 2000. Prevention of Accidents through Experience Feedback. New York, NY: Taylor & Francis. ISBN 07-484-0925-4.
- Kubíček, P., Č. Šašinka, and Z. Stachoň. 2014. “Selected Cognitive Issues of Positional Uncertainty in Geographical Data.” Geografie, Česká Geografická Společnost 119(1): 67–90. .
- Kumar, N., and C. R. M. Waylor. 2003. “Proximity to Alcohol-serving Establishments and Crime Probabilities in Savannah, Georgia: A Statistical and GIS Analysis.” Southeastern Geographer 43 (1): 125–142. doi:10.1353/sgo.2003.0015.
- Levine, T. 2008. “On Traffic Safety and Law Enforcement.” Geography & Public Safety 2 (1): 1–2.
- Lin, H., M. Batty, S. E. Jørgensen, B. Fu, M. Konečný, A. Voinov, P. Torrens, et al. 2015. “Virtual Environments Begin to Embrace Process-based Geographic Analysis.” Transactions in GIS John Wiley & Sons Ltd 19(4): 493–498. . doi:10.1111/tgis.12167.
- Lin, H., M. Chen, and G. Lu. 2013. “Virtual Geographic Environment: A Workspace for Computer-Aided Geographic Experiments.” Annals of the Association of American Geographers 103: 465–482. doi:10.1080/00045608.2012.689234.
- McCue, C. 2011. “Proactive Policing: Using Geographic Analysis to Fight Crime.” Geography & Public Safety 2 (4): 3–5.
- Ministry of Finance of the Czech Republic. 2014. “Informativní Přehled Povolených Zařízení - Stav K 31. 12. 2014.” Accessed 8 December 2015. http://www.mfcr.cz/cs/soukromy-sektor/regulace/loterie-a-sazkove-hry/prehled-povolenych-zarizeni/2015/informativni-prehled-povolenych-zarizeni-20151
- Office of Community Oriented Policing Services. 2014. Community Policing Defined. ISBN: 978-1-935676-06-5. http://www.cops.usdoj.gov/pdf/vets-to-cops/e030917193-CP-Defined.pdf
- Ratcliffe, J. H. 2011. “HowNear is Near? Quantifying the Spatial Influence of Crime Attractors and Generators.” In Patterns, Prevention, and Geometry of Crime, edited by M. Andreson and B. J. Kinney. Monsey, NY: Criminal Justice Press.
- Ratcliffe, J. H. 2012. “The Spatial Extent of Criminogenic Places: A Changepoint Regression of Violence around Bars.” Geographical Analysis 44 (4): 302–320. doi:10.1111/j.1538-4632.2012.00856.x.
- Rengert, G. F., J. H. Ratcliffe, and S. Chakravorty. 2005. Policing Illegal Drug Markets: Geographic Approaches to Crime Reduction. Monsey, NY: Criminal Justice Press.
- Řezník, T. 2013. “Geografická Informace V Době Směrnice INSPIRE: Nalezení, Získání a Využití Dat Pro Geografický Výzkum [Geographic Information in the Age of the INSPIRE Directive: Discovery, Download and Use for Geographical Research].” Geografie, Praha: Česká Geografická Společnost 118(1): 77–93. .
- Řezník, T., B. Horáková, and R. Szturc. 2015. “Advanced Methods of Cell Phone Localization for Crisis and Emergency Management Applications.” International Journal of Digital Earth Taylor & Francis 8(4): 259–272. . doi:10.1080/17538947.2013.860197.
- Robitaille, E., and P. Herjean. 2008. “An Analysis of the Accessibility of Video Lottery Terminals: The Case of Montreal.” International Journal of Health 7: special section p1–15.
- Roncek, D. W., and D. Faggiani. 1985. “High Schools and Crime: A Replication.“ The Sociological Quarterly 26 (4): 491–505.
- Roncek, D. W., and P. A. Maier. 1991. “Bars, Blocks, and Crime Revisited: Linking the Theory of Routine Activities to the Empiricism of ‘Hot Spots’.” Criminology 29: 725–753. doi:10.1111/j.1745-9125.1991.tb01086.x.
- Schenewerk, M. 2003. “A Brief Review of Basic GPS Orbit Interpolation Strategies.” GPS Solutions 6 (4): 265–267. doi:10.1007/s10291-002-0036-0.
- Scott, D. W. 1988. “A Note on Choice of Bivariate Histogram Bin Shape.” Journal of Official Statistics 4 (1): 47.
- Spolek JURISTIC. 2001. “O možnostech regulovat lokalizaci heren a výherních hracích automatů formou právního předpisu obce.” Accessed 8 December 2015. ISSN 1802-789X. http://spravni.juristic.cz/94133/clanek/j_obce.html
- Subrahmanian, V. S., and J. Dickerson. 2009. “What Can Virtual Worlds and Games Do for National Security?” Science 326: 1201–1202. doi:10.1126/science.1182660.
- Szczyrba, Z., V. Mravčík, D. Fiedor, J. Černý, and I. Smolová. 2015. “Gambling in the Czech Republic.” Addiction 110 (7): 1076–1081. doi:10.1111/add.12884.
- Temelkovski, D., M. Jovanovik, I. Mishkovski, and D. Trajanov. 2012. “Towards Open Data in Macedonia: Crime Map Based on Ministry of Internal Affairs’ Bulletins“. In: The 9th Conference for Informatics and Information Technology (CIIT 2012). Bitola, Macedonia, April 19–22.
- Tompson, L., S. Johnson, M. Ashby, C. Perkins, and P. Edwards. 2015. “UK Open Source Crime Data: Accuracy and Possibilities for Research.” Cartography and Geographic Information Science 42 (2): 97–111. doi:10.1080/15230406.2014.972456.
- Tošovský, M., O. Skalník, M. Hrinko, and D. Jasovská. 2010. Čtení O Community Policing: Soubor Textů, 60. Otevřená společnost, o.p.s. - Centrum ProPolice. Prague. ISBN: 978-80-87110-20-1. http://www.otevrenaspolecnost.cz/download-publication/118
- Uchida, C. D. 2010. A National Discussion of Predictive Policing: Defining Our Terms and Mapping Successful Implementation Strategies. Prepared for the National Institute of Justice, Document No. NCJ, 230404. Los Angeles, CA, November 18–20.
- Wang, F. 2012. “Why Police and Policing Need GIS: An Overview.” Annals of GIS 18 (3): 159–171. doi:10.1080/19475683.2012.691900.
- Weisburd, D. 2005. ““Hot Spots Policing Experiments and Criminal Justice Research: Lessons from the Field.” The Annals of the American Academy of Political and Social Science 599 (1): 220–245. doi:10.1177/0002716205274597.
- Weisburd, D. 2008. Place Based Policing. Washington DC: Police Foundation.
- Weisburd, D., and T. McEwen. 1998. “Crime Mapping and Crime Prevention.” In Crime Mapping and Crime Prevention: Crime Prevention Studies, edited by D. Weisburd and T. McEwen, 1–26. Vol. 8. Monsey, NY: Criminal Justice Press.
- Xie, Z., and J. Yan. 2008. “Kernel Density Estimation of Traffic Accidents in a Network Space.” Computers, Environment and Urban Systems 32 (5): 396–406. doi:10.1016/j.compenvurbsys.2008.05.001.
Appendix
import arcpy
import os
import csv
csvtable = open(env.workspace + ‘\\results.csv’, ‘wb’) # creating a new csv
wr = csv.writer(csvtable)
# initial setting of crime category names
fields = arcpy.ListFields(‘gdb_200 .dbf’)
rows = arcpy.SearchCursor(‘gdb_200 .dbf’) # getting rows of table with most crime categories
events = [‘buffer’] # array for all the column names in new table. index 0 is for ‘Buffer’
for row in rows:
event = row.getValue(fields[1].name)
isthere = event in events
if isthere = = False:
events.append(event.encode(‘utf-8ʹ))
wr.writerow(events) # writing header to csv table
# looping over buffer distance values
for dis in range(5, 200, 5):
gdb = ‘gdb_’ + str(dis) + ‘ .dbf’
fields = arcpy.ListFields(gdb)
rows = arcpy.SearchCursor(gdb)
values = [0] * (len(events) + 1) # new array with 0 values, length is equal to the number of all crime categories
values[0] = dis # setting the buffer distance value
for row in rows:
event = row.getValue(fields[1].name).encode(‘utf-8ʹ)
for e in events:
if e = = event:
index = events.index(e)
values[index] = row.getValue(fields[2].name) # storing the quantity of the particular crime type wr.writerow(values) # writing values from array to our csv