ABSTRACT
This paper combines the principal component analysis (PCA) with a modified normalized difference water index (MNDWI) to improve the accuracy in extracting water bodies from Landsat Thematic Mapper (TM) imagery. To this end, one Landsat TM image of Taiyuan, China, obtained on 23 September 2011 was used to perform PCA and extract MNDWI. The first two principal components of the PCA and extracted MNDWI were combined to compose a false-colour image, which was then used to develop the enhanced water index (EWI). This method was applied to extract water bodies from Landsat TM imagery for both urban areas and surrounding mountain areas of Taiyuan, which resulted in an overall accuracy of 95%. EWI was also compared with other common water indices in extracting water bodies including general water index, MNDWI and new water index. The results show that the proposed method has the highest accuracy and is recommended to extract water bodies using multispectral satellite imagery for urban areas with mountains surrounded.
KEYWORDS:
Introduction
The real-time monitoring of water bodies on the Earth’s surface is an essential work to control surface water pollution and protect ecological environments. Due to the advantages of fast information update and the ability to produce near real-time observations over large geographic areas, remote sensing has been widely applied in investigating and monitoring surface water resources in the past two decades (McFeeters Citation1996; Xu Citation2006; Ji, Zhang, and Wylie Citation2009; Rokni et al. Citation2014). Moreover, if a remote sensor is passively recording the electromagnetic signals, the unobtrusive images can greatly reduce the biases in data collection and improve the accuracy in image processing (Bi, Wang, and Zeng Citation2012).
The first Landsat was launched in 1972, since then its imagery has become an important data resource in environmental remote sensing due to its moderate spatial resolution, multiple spectral bands and continuous observation (Deng Citation2008). Landsat Thematic Mapper (TM), launched in 1984 and retired in 2012, provided nearly 30-year continuous imaging of the Earth. TM collects seven multispectral bands: six bands in 30 m pixel size from blue to mid-infrared regions of the electromagnetic spectrum and one band in 120 m pixel size on thermal-infrared region. The major application of Landsat TM imagery is to detect the land use and land cover changes over time (Jensen Citation2016). As one of the important land covers on the Earth’s surface, open water has been studied by many scholars, and multiple methods have been developed to delineate open waters from lands using Landsat imagery (McFeeters Citation1996; Zhong Citation2007; Feyisa et al. Citation2014; Shridhar, Kulkarni, and Alvarinho Citation2015; Yang et al. Citation2015; Gao et al. Citation2016).
Due to inexpensive implementation and high accuracy, several water indices have been developed in the past to monitor water resources (Jiang et al. Citation2014). Normalized difference water index (NDWI), one of the most popular water indices used in the remote sensing community, was first proposed by McFeeters to identify non-urban surface water associated with wetlands (McFeeters Citation1996). The shortcoming of this index is that it cannot efficiently suppress the signal from built-up lands causing extracted water bodies to be mixed in with built-up lands (Xu Citation2006). Later in 1998 based on Yang and Xu’s finding that the sum of spectral reflectance of water from Band 2 and Band 3 is always greater than the sum of water reflectance from Band 4 and Band 5 in Landsat TM imagery, a general water index (GWI) was proposed to extract water from Landsat data (Yang and Xu Citation1998). The disadvantage of this method is that it needs to repeatedly select thresholds to ensure the optimal water extraction, leading to heavy workload and uncertain results. To avoid this drawback, a modified normalized difference water index (MNDWI) was developed by Xu (Citation2006). The MNDWI is capable of extracting surface water while suppressing errors from built-up lands, vegetation and soil (Xu Citation2006). In 2007, Wang and Yi compared several water indices including NDWI, MNDWI and the normalized difference snow index by extracting waters from a TM imagery of Dongting Lake in northeastern Hunan Province, China and found that Zhong’s (Citation2007) method achieved the highest accuracy in the study (Wang and Yi Citation2007). However, these methods have limitations with regard to shadow elimination and difficulty in extracting small water bodies in areas with both urban and mountain features (Gao et al. Citation2016).
Landsat TM imagery has seven spectral bands, and information in certain bands is redundant, which can reduce the accuracy in water extraction (Zhong and Wang Citation2006). Principal component analysis (PCA), a statistical procedure and spectral enhancement technique widely used in remote sensing, is capable of transforming the multiple correlated bands into a set of linearly uncorrelated bands called principal components (PCs) (Jensen Citation2016). In addition, PCA can reduce the dimensionality of multispectral satellite images and, at the same time, preserve the majority of the information in original imagery (Ou Citation2006). Therefore, in order to accurately extract water bodies for an urban area surrounded by mountains, an enhanced water index (EWI) by combining MNDWI and the first two PCs of PCA from Landsat TM imagery is proposed in this paper. The results indicate that this method can effectively remove shadow effects in water extraction for both mountain and urban areas.
Study area and data
Study area
Taiyuan is the capital city of Shanxi Province in northern China (37.8706° N, 112.5489° E). Taiyuan is surrounded by mountains on its western, northern and eastern sides. The southern side, along with its central plain area, forms a valley like a dustpan from north to south. The main water resources in Taiyuan include the Fen River, the Jian River, the North River, Jinyang Lake, Black Dragon Pool and Yingze Lake. Among them, the Fen River is the second largest tributary of the Yellow River, running from north to south through the whole territory of Taiyuan. The length of the river within the city boundary is about 20 km with approximately 1.7 km2 open water. Jinyang Lake, with 4.8 km2 open water, is the largest artificial lake in northern China.
Data
One Landsat 5 TM image (Path125/Row034) acquired on 23 September 2011 with no cloud cover was used to develop the EWI in this study. The image was projected to a Universal Transverse Mercator (UTM) coordinate system with WGS-84 datum. A typical geometric correction was applied on the original image to eliminate its geometric distortion with a 0.5 pixel root mean square error. Lastly, a vector boundary of Taiyuan city was used to subset the corrected image into the area of interest, as shown in .
Methods
Water indices
The NDWI proposed by McFeeters (Citation1996) uses green band and near-infrared band to maximize the limits of vegetation, so as to outstand the water information. The general equation and the one using Landsat TM imagery to calculate NDWI are shown below:
where Green is the reflectance on green band, corresponding to Band 2 in TM imagery. NIR is the reflectance on near-infrared band, corresponding to Band 4 in TM imagery. The NDWI value ranges from −1 to +1, and zero is set as the threshold to separate water and non-water types. However, this method ignores the interferences from mountains, bare lands and urban shadows, and is unable to completely separate built-up features from water bodies (Xu Citation2006).
Another index, GWI, in water extraction was proposed by Yang and Xu (Citation1998), which calculates the difference between visible bands and infrared bands using the following equation to enhance the water extraction:
where Green and Red are the reflectance on visible region, corresponding to Band 2 and Band 3 in TM imagery, respectively. NIR and MIR are the reflectance on near-infrared and middle-infrared regions, corresponding to Band 4 and Band 5 in TM imagery, respectively. GWI is not a normalized index as NDWI, which makes the determination of the threshold and interpretation of the results difficult.
Xu (Citation2006) proposed a modified NDWI, in which the mid-infrared band (MIR) was used to replace the NIR band in Equation (1).
Like McFeeters’ NDWI, the threshold value for MNDWI was set to zero, but Xu found that a manual adjustment of the threshold could achieve a more accurate result in the water delineation (Ji, Zhang, and Wylie Citation2009).
Ding (Citation2009) developed the new water index (NWI) using four spectral bands from blue to MIR region. In this new index, green band in Equation (1) was replaced by blue band, and NIR band in Equation (1) was replaced by the total reflectance from three infrared bands in Landsat TM imagery. The index is calculated in the following way:
where Blue is the reflectance on blue band, corresponding to Band 1 in TM imagery. MIR1 and MIR2 are two MIRs, corresponding to Band 5 and Band 7 in TM imagery, respectively.
Principal component analysis
PCA is one of the main methods in feature selection (Zhao and Maclean Citation2000; Jia and Chang Citation2006). In the remote sensing field, the usage of PCA lays upon the foundation that bands in multispectral and/or hyperspectral images are strongly correlated and often contain redundant information about the surface objects (Rodarmel and Shan Citation2002). PCA can remove the redundant information in correlated bands, and a linear combination of the original bands accounting for the variation of pixel values in an image can be identified. Taking into account the statistical properties of the multispectral bands, PCA has been widely used to analyse interdependency and correlation between bands in remotely sensed imagery. By applying PCA, the spectral bands in original satellite imagery can be converted into the same number or fewer uncorrelated PCs, and the first 2–3 PCs normally contain more than 95% of the information stored in the original imagery (Yang and Li Citation2013).
presents the eigenvalues and contribution rates of each component after transforming the six spectral bands in TM imagery (without thermal band) into six PCs (PC-1 to PC-6).
Table 1. The eigenvalues and contribution rates of six principal components after PCA on the original TM image.
In , the first two PCs of PC-1 and PC-2 have 98.43% of the cumulative contribution rate, which contains the majority of the information in the original TM imagery with unwanted image noises removed. This can also be illustrated by comparing the spectral reflectance of land features before and after PCA transformation.
presents the spectral characteristics of five typical land surfaces (water, building, vegetation, mountain and shadow) in Taiyuan in the original TM imagery (left) and the first three PCs after PCA transformation (right). In (left), except vegetation, all other land features of water, shadow, building and mountain are difficult to separate spectrally in the original TM imagery due to their similar reflectance. However, after PCA transformation (, right), all five land features have larger spectral separability in PC-1 and PC-2. Water and shadow are close to each other in PC-1 but separated in PC-2; Building and shadow are close in PC-2 but largely separated in PC-1. Considering PC-3 contributes only 1.25% with a very low feature separability, MNDWI was used to replace PC-3 for further analysis in this study.
Construction of EWI
PC-1, PC-2 and MNDWI were used to create a three-layer false-colour image using the layer stack function in ERDAS Imagine. The new image and its spectral profiles for the same five land features in the study area are presented in .
Figure 3. False-colour image with RGB = PC-1, PC-2, MNDWI (left) and the spectral profiles for the five land features (right).
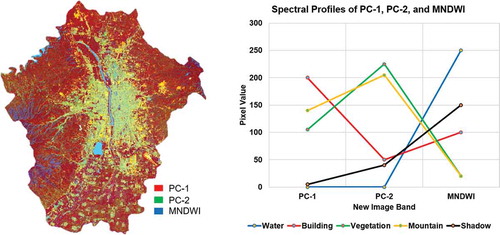
By comparing the spectral profiles of the five typical land features in and , we noticed that the spectral differences of the five land features in MNDWI image (, right) are much larger than those in PC-3 (, right), especially between water and shadow. The reflectance of water and shadow is almost the same in PC-1, but they are quite different in both PC-2 and MNDWI. The slope of the spectral profile of water between PC-1 and PC-2 is almost zero, while the slope of shadow is a positive number. The reflectance of water in PC-1 and PC-2 is the lowest, but it is the highest in MNDWI (, right). Based on the analysis of the spectral characteristics of this false-colour image, we proposed a new EWI, which is calculated in the following way:
where MNDWI is the modified NDWI from Xu (Citation2006), and PC-1 and PC-2 are the first two PCs from PCA.
Results and assessment
To assess the accuracy of proposed water index, water bodies extracted from EWI using Equation (5) were compared with those derived from other water indices including GWI, MNDWI and NWI using Equations (2)–(4) for both urban and surrounding mountain areas of Taiyuan ().
Figure 4. Index images of the study area extracted using different methods: GWI (a), MNDWI (b), NWI (c) and EWI (d).
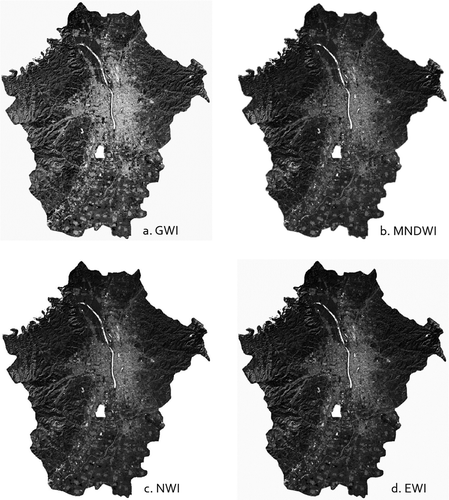
An accuracy assessment on water bodies extracted from four water indices was conducted in ERDAS Imagine using 200 sampling points randomly generated in the study area. Each point was evaluated using Google Earth historical imagery for the results obtained from all methods. Three images are available in 2011 on Google Earth for Taiyuan city, the two images obtained on July 6 and October 24 were combined for this purpose. The results showed that EWI proposed in this paper achieved the highest accuracy with the best visual effect among the four water indices in water extraction in Taiyuan ().
Table 2. Accuracies and visual effects of water bodies extracted from TM image using four water indices.
In , the visual effect refers to the smoothing effect of water boundary, the degree of continuity of river, the existence of a large number of non-water speckles and the effect of water extraction. However, due to the limit of 30 m pixel size in TM imagery, the water bodies with areas less than 900 m2 are not detected in this study.
To further evaluate the effectiveness of shadow removal among four water indices, two small areas including both water and shadow were selected for comparison: one was from downtown Taiyuan and the other from surrounding mountain area. The comparison results are presented in and , respectively. In both figures, the white areas represent extracted water bodies and black areas for lands.
Figure 5. Shadow removal from water bodies (white colour) in four index images for a small urban area in downtown Taiyuan.
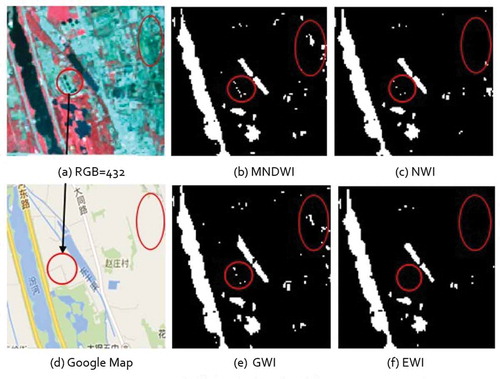
Figure 6. Shadow removal from water bodies (white colour) in four index images for a small mountain area surrounding Taiyuan.
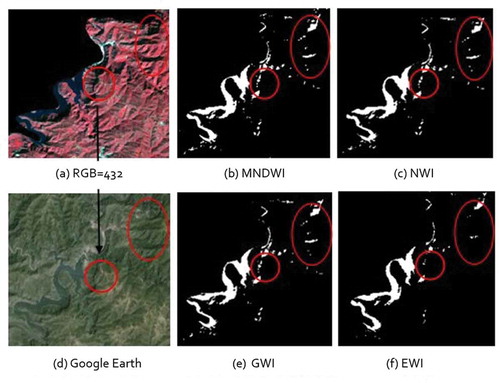
As shown in and , MNDWI, NWI, GWI and EWI are all capable of extracting water bodies from TM imagery with reasonable accuracies. However, misclassification still exists in all four methods, some shadows were mistakenly classified as water bodies in both areas.
shows the results of water bodies extracted using four indices in the urban areas of Taiyuan. It was found that both NWI and EWI are better than MNDWI and GWI in water extraction in urban settings, because some residential areas that were mistakenly classified as water in MNDWI and GWI (in ellipses) were removed in NWI and EWI. However, NWI failed to further remove the urban shadows that were mistakenly classified as water (in circles), while EWI is capable of removing almost all of the residential lands and shadows that were mistakenly classified as water, which is the best in all four methods in extracting water bodies for an urban setting.
shows the results of water bodies extracted using four indices in the surrounding mountain areas of Taiyuan. Circles and ellipses in the map show some mountain shadows that were mistakenly classified as water. Results show that there is almost no difference in the circle and ellipse areas among the three index images of MNDWI, NWI and GWI, but the results obtained from EWI are much better, which removed most shadows in the circle and ellipse areas. The remaining misclassified few shadows in mountains demonstrate the difficulty and limitation in distinguishing water from shadow based solely on spectral analysis.
Conclusions and discussions
This paper presents an EWI to extract water bodies from Landsat TM imagery of Taiyuan, China. By combining the first two PCs of PCA and a modified NDWI, the results demonstrate that the water index we proposed is efficient and accurate in extracting water bodies from TM imagery for an area with both urban features and mountains. This method can reduce the amount of data processing, experimental complexity and running time, and obtain better water-extracted effect and higher accuracy than other methods. In addition, the default threshold of EWI is set to zero to avoid the tedious work of repeatedly selecting a threshold and its inverse influences on the results when a wrong threshold was selected. This leads to a fast and accurate method in water extraction from TM imagery, which is recommended for extracting water bodies from other Landsat multispectral imagery. However, since this index was developed mainly based on the spectral analysis from one Landsat image, it is unable to remove all shadows that have similar spectral characteristics with open water and may not be effective and accurate in extracting water bodies from other remotely sensed images. Therefore, it is strongly suggested always to assess the threshold manually before EWI is applied on a new study site, especially when using other remotely sensed imagery. A small adjustment of the threshold may result in a higher accuracy in water body extraction. Future studies are needed to further improve the separation between mountain shadows and water bodies in TM imagery, as well as to test its adoptability in other places using different remotely sensed imagery.
Disclosure statement
No potential conflict of interest was reported by the authors.
References
- Bi, H., S. Wang, and J. Zeng. 2012. “Comparison and Analysis of Several Common Water Extraction Methods Based on TM Image.” Remote Sensing Information 27 (5): 77–82.
- Deng, K. 2008. Remote Sensing Image Classification Based on Multi-Source Data. Xianyang: Northwest Agriculture and Forestry University Press, Shaanxi Province.
- Ding, F. 2009. “A New Method for Fast Information Extraction of Water Bodies Using Remotely Sensed Data.” Remote Sensing Technology and Application 24 (2): 167–171.
- Feyisa, G. L., H. Meilby, R. Fensholt, and S. R. Proud. 2014. “Automated Water Extraction Index: A New Technique for Surface Water Mapping Using Landsat Imagery.” Remote Sensing of Environment 140: 23–35. doi:10.1016/j.rse.2013.08.029.
- Gao, H., L. Wang, L. Jing, and J. Xu. 2016. “An Effective Modified Water Extraction Method for Landsat-8 OLI Imagery of Mountainous Plateau Regions.” 9th Symposium of the International Society for Digital Earth (ISDE). Vol. 34, 012010. Bristol: IOP Conf. Series: Earth and Environmental Science.
- Jensen, J. R. 2016. Introductory Digital Image Processing: A Remote Sensing Perspective. 4th ed. Upper Saddle River, NJ: Pearson.
- Ji, L., L. Zhang, and B. Wylie. 2009. “Analysis of Dynamic Thresholds for the Normalized Difference Water Index.” Photogrammetric Engineering & Remote Sensing 75 (11): 1307–1317. doi:10.14358/PERS.75.11.1307.
- Jia, K., and Q. Chang. 2006. “Extracting Water Body Information Using Principal Components Analysis.” Journal of Huaiyin Teachers College (Natural Science Edition) 5 (3): 242–245.
- Jiang, H., M. Feng, Y. Zhu, N. Lu, J. Huang, and T. Xiao. 2014. “An Automated Method for Extracting Rivers and Lakes from Landsat Imagery.” Remote Sensing 6: 5067–5089. doi:10.3390/rs6065067.
- McFeeters, S. 1996. “The Use of the Normalized Difference Water Index (NDWI) in the Delineation of Open Water Feature.” International Journal of Remote Sensing 17 (7): 1425–1432. doi:10.1080/01431169608948714.
- Ou, C. 2006. “Research on Principal Component Analysis of Remote Sensing Images Processing through Example.” Geomatics and Spatial Information Technology 29 (5): 56–59.
- Rodarmel, C., and J. Shan. 2002. “Principal Component Analysis for Hyperspectral Image Classification.” Surveying and Land Information Systems 62 (2): 115–122.
- Rokni, K., A. Ahmad, A. Selamat, and S. Hazini. 2014. “Water Feature Extraction and Change Detection Using Multitemporal Landsat Imagery.” Remote Sensing 6: 4173–4189. doi:10.3390/rs6054173.
- Shridhar, D. J., K. Kulkarni, and J. L. Alvarinho. 2015. “A Renew on Extraction of Lakes from Remotely Sensed Optical Satellite Data with a Special Focus on Cryospheric Lakes.” Advances in Remote Sensing 4: 196–213. doi:10.4236/ars.2015.43016.
- Wang, Z., and S. Yi. 2007. “Comparison and Research on the Different Index Models Used in Water Extraction by Remote Sensing.” Science Technology and Engineering 7 (4): 534–537.
- Xu, H. 2006. “Modification of Normalized Difference Water Index (NDWI) to Enhance Open Water Features in Remotely Sensed Imagery.” International Journal of Remote Sensing 27 (14): 3025–3033. doi:10.1080/01431160600589179.
- Yang, C., and M. Xu. 1998. “Discussion on Water Extraction Based on Remote Sensing Information Mechanism.” Geographical Research 7 (Suppl): 86–89.
- Yang, J., and W. Li. 2013. “Feature Selection Methods in Extracting Impervious Surface from Landsat TM Image.” Annals of GIS 10 (4): 253–261. doi:10.1080/19475683.2013.843591.
- Yang, Y., Y. Liu, M. Zhou, S. Zhang, W. Zhan, C. Sun, and Y. Duan. 2015. “Landsat 8 OLI Image Based Terrestrial Water Extraction from Heterogeneous Backgrounds Using a Reflectance Homogenization Approach.” Remote Sensing of Environment 171: 14–32. doi:10.1016/j.rse.2015.10.005.
- Zhao, G., and A. L. Maclean. 2000. “A Comparison of Canonical Discriminant Analysis and Principal Component Analysis for Spectral Transformation.” Photogrammetric Engineering and Remote Sensing 66 (7): 841–847.
- Zhong, C. 2007. “Study of Water Extraction in Wetland Automatically from TM Images.” Water Resources Research 28 (4): 589–595.
- Zhong, J., and R. Wang. 2006. “Multi-Temporal Remote Sensing Images Change Detection Based on PCA.” Journal of Electronics and Information Technology 28 (6): 994–998.