Abstract
Himalaya, being an active orogen, is continuously undergoing tectonic activities that pose threats of land stability on the mountains. A large number of mass movement occurrences have been observed in a part of the Tons river valley area. Remote sensing and geographical information system (GIS) based techniques have been used to derive and analyse various parameters and attributes pertinent to the landslide hazards. The GIS based analysis in the present work, incorporating the fuzzy membership values from the existing landslides, has been used for spatial modelling of the parameter maps including geology, slope amount, slope aspect, weathering, erosion, drainage influence, tectonic influence and landuse/land cover for landslide hazard zonation. The model has been used to spatially classify the study area into zones of very high, high, moderate, low and very low landslide hazard zones. Eighty-five percent of the active landslides have been observed to occur in very high and high hazard zones.
1. Introduction
The Himalaya, being an active fold mountain, is still undergoing tectonic upliftment (Jade Citation2004) causing lots of rapid changes in the landscape due to mass movement activity. Landslides cause heavy damage to property, infrastructure and lives in mountainous regions every year. Although the phenomenon of landslides is controlled by a variety of geological and geomorphological factors, the triggering factors however, may be earthquakes and heavy rainfall in the region (Glade et al. Citation2000, NRSA Citation2000). It is not easy to control rainfall or earthquakes, therefore in order to minimize the damage, it is necessary to identify and map potential landslide hazard areas. Such a map, known as a landslide hazard zonation (LHZ) map (Anbalagan Citation1992) can be used for substantially reducing the risk from the potential landslide by adopting appropriate management measures (Mathew et al. Citation2007a, Citationb). The reliability of such landslide susceptibility maps will be controlled by the quality of data and the methodology or the model used in preparing the maps (Ayalew and Yamagishi Citation2005). Geographical information system (GIS) and remote sensing techniques are now being widely used for LHZ maps. The present work envisages the remote sensing and GIS based landslide hazard susceptibility analysis in a part of Tons river valley, NW Himalaya, bordering the Uttarakhand and Himachal Pradesh states of India.
2 Study area
The area under investigation () forms a part of Lesser Garhwal Himalaya covering the autochthonous zone and Krol nappe tectonic divisions of Auden (Citation1937). The regional geology of these tectonic zones has been studied by many workers for example, Gansser (Citation1964), Rupke (Citation1974), Srikantia and Bhargava (Citation1974), Srivastava and Lakhera (2007). The area undertaken for present study falls between latitudes 30°36′29″ N and 30°44′12″ N and between longitudes 77°42′01″ E and 77°47′58″ E covering 135.4 km2 across the Tons Thrust (). The landslide hazard susceptibility mapping carries a significance because the area not only provides an alternative route to the world famous tourist place and pilgrimage Yamunotri, but a new hydroelectric power project is also expected to be built on Tons river within the study area. Therefore fast developmental activities in this region are very much expected. The present work is aimed at identifying the safer grounds within the study area in terms of different degrees of landslide hazard vulnerability.
Figure 1. (a) Simplified geological map of Himalaya after Gansser (1964). MCT, Main Central Thrust; MBT, Main Boundary Thrust; HFT, Himalayan Frontal Thrust. (b) Geological map of the study area after Srivastava and Lakhera (2007) presented in a 3-dimensional perspective view from the south. Available in colour online.
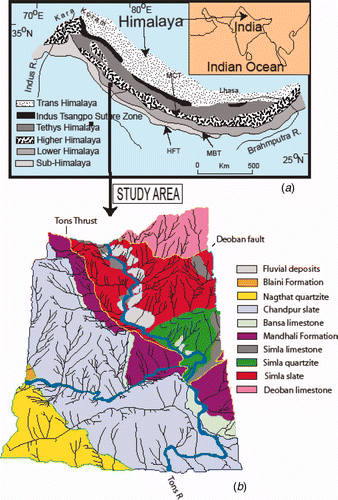
3 Geomatics of landslide hazard susceptibility zonation
Landslide hazard zonation refers to the division of a land surface into homogeneous areas or domains and their ranking according to the degree of actual/potential hazard caused by mass movement (Varnes Citation1984). The methods available for preparing LHZ maps can be divided into two categories: qualitative and quantitative (Ayalew and Yamagishi Citation2005). Qualitative methods involve the process of ranking the area into different zones of hazard based on expert opinions. Many researchers have applied qualitative methods successfully in their specific areas of study using the parameters of geomorphology (Keinholz Citation1978, Rupke et al. Citation1988), the blind weighting method (Gee Citation1992), remote sensing data (Chung and Fabbri Citation1999, Sarkar and Kanungo Citation2004, Lee Citation2005, Nichole et al. 2006, Pradhan et al. Citation2006), weighted landslide hazard mapping (Pachauri and Pant Citation1992), the parameter-weighting method (Gupta et al. Citation1999, Saha et al. Citation2002), the analytic hierarchy process (Saaty Citation1980, NRSA Citation2001) and multi-criteria analysis (Abella and van Westen Citation2008) for preparing landslide hazard zonation maps. Qualitative or heuristic methods are usually applicable for regional studies (Guzetti et al. Citation1999, Soeters and van Westen 1996, Ayalew et al. Citation2004, Ruff and Czurda Citation2008). Quantitative methods for landslide susceptibility mapping include statistical process that may include the information value method (Yin and Yan Citation1988, Lee and Pradhan Citation2006), the multivariate statistical method (Carrara et al. Citation1995, Chung et al. Citation1995, Lee et al. Citation2002), the certainty factor function (Binaghi et al. Citation1998), logistic regression analysis (Johnson Citation1998, Arora et al. 2004, Lee et al. Citation2004, Lee Citation2005, Chang et al. Citation2007, Lee and Pradhan Citation2007, Das et al. Citation2010), the weights of evidence model (Mathew et al. Citation2007a), an artificial neural network approach (Lee et al. Citation2003, Ermini et al. Citation2005, Melchiorre et al. Citation2008, Pradhan and Lee Citation2009), discriminant analysis (Carrara et al. Citation1991, Citation2003, Baeza and Corominas Citation2001), the likelihood ratio (Chung and Fabbri Citation2003, Fabbri et al. Citation2003, Lee Citation2004), the landslide susceptibility index (Lee and Jones Citation2004) and binary logistic regression analysis (Mathew et al. Citation2007b). The role of GIS in mass movement studies in respect of theory and methods has been discussed by Alexander (Citation2008). Studies by Gorsevski et al. (Citation2003, Citation2005), Kanungo et al. (Citation2006, Citation2009), Chung and Fabbri (Citation2008) and Pradhan et al. (Citation2009) utilized the fuzzy set model to generate a landslide hazard prediction map.
3.1 The input parameters
The techniques of remote sensing in association with ground verification, wherever possible, is used to map the spatial distributions of various causative factors such as geology, structures, landuse, weathering and erosion in relation to their probable role in the landslide phenomenon in the study area. In addition, various other pertinent parameter maps such as slope, aspect, drainage influence buffer and tectonic influence buffer maps have been generated in the present work using a GIS. The data that have been used in the study include those from different satellites (SPOT-1, HRV-2; Landsat-5, TM; IRS-1B, LISS-II; IRS 1C, LISS-III; and IRS-1C PAN) from different dates ranging between 1987 and 2008, aerial photographs (black and white aerial photographs of approximately 1: 60,000 scale with 80% overlap) and Survey of India toposheets (no. 53F/10 and 53 F/14 on 1: 50,000 scale). Eight parameter maps mentioned above were rasterized at 20 m grid for GIS analysis. The study area thus had a total of 338,520 numbers of raster grids and the total number of grids that the active landslides occupied was 4129. The parameters that are used for the GIS analysis for LHZ of the area are discussed.
3.1.1 Geology
The study area mainly comprises of Precambrian rocks of the Deoban, Simla, and Jaunsar Groups and Blaini Formation of Krol Group, besides the recent fluvial deposits. The Deoban Group overlies the autochthonous Simla Group of rocks along the Deoban Fault in the northeastern sector of the study area while the Krol nappe rocks tectonically overlie the Simla autochthonous rocks along the Tons Thrust ((b)). The geological map is presented in the form of a 3-dimensional perspective view from the south in (b).
3.1.2 Slope
The slope amount is an important parameter for the phenomenon of landslide. It is so important that the landslides are often designated as slope failure. The relief of the study area varies between 580 to 2530 m from mean sea level and accounts for the significant slope variation in the area. The slope maps have been prepared from Survey of India toposheets using 40 m contour intervals. A digital elevation model (DEM) was generated and accordingly the slope map was prepared by classifying the area into six slope classes, given in (a). The maximum density of the active landslide pixels was obtained where the slope of the ground was between 40° and 50°. The still steeper slopes have shown much less landslide activity, perhaps because they expose the bedrocks and any deposition of loose material or soil does not easily take place over them on account of steepness.
3.1.3 Aspect
Slope aspects in the Himalayan terrain also play an equally important role in the landslide phenomenon. The southern aspects of the slope are most vulnerable because of scant vegetation on account of low retained soil moisture. The northern aspect, on the other hand, retains soil moisture because of less exposure to sun rays. Hence taller trees may grow that tend to stabilize the northern slope. The distribution of different slope aspects into eight categories is given in (b). The south facing slope in the study area contains the maximum density of active landslide pixels whereas the minimum is obtained for the north facing slopes, as expected. The scant vegetation on the south and adjacent facing slopes accounts for more landslides being triggered. Lack of vegetation renders dryness to the slope material and hence reduces its cohesive strength.
3.1.4 Weathering
Weathering is the process of decomposition or loosening of the rocks, thus creating the material available for mass movement. Only a small portion of the area exposes the fresh outcrop and the majority of the portion is covered by weathered material that may occur in the form of residual or colluvial deposits of differing depths. For the assessment of depth of weathering, the 3-dimensional images from the stereo-pair aerial photographs were of much help. The road cuttings on the hill slopes were examined and verified in the field. The tonal characters of the verified zones were used to extract the related information from other places through supervised classification of IRS 1C PAN and SPOT data. Four attribute classes, namely deeply weathered (> 60 m), moderately weathered (30–60 m), shallow weathered (1–30 m) and fresh (<1 m)) were assigned on the basis of a tentative depth of weathered zone ((c)). The moderately weathered zone has the highest landslide density while the deeply weathered zone has the lowest because most of the zones falling under this category have been stabilized and occupy either agricultural sites or old/ dormant landslide zones in the study area.
3.1.5 Erosion
The streams and drainage are the main agents for erosion in the study area besides the seasonal ice caps on the hill tops. The neotectonic activities in the area bring about the deep gully erosion on the slopes, while the perennial streams are responsible for toe erosion. Thus the state of erosion is different in different parts of the area. The different zones in the area have been assessed into five erosional classes ((a)) namely very low erosion, low erosion, moderate erosion, high erosion and very high erosion, subjectively, based on the remote sensing data study supported by field investigation. It has been observed that the maximum density of active landslide pixels is present in the very high erosion class.
Figure 3. (a) Erosion map, (b) drainage influence map created by a geographical information system (GIS) using the buffer at an interval of 50 m from the stream and (c) tectonic influence map of the study area created by GIS using the buffer at an interval of 50 m from the already mapped tectonic planes such as lineaments, faults. Available in colour online.
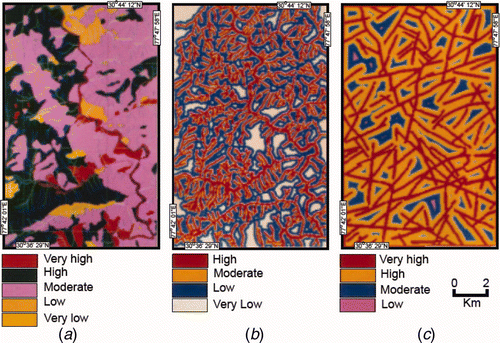
3.1.6 Drainage influence
The drainage map of the study area was prepared with the help of Survey of India toposheets. The drainage of the study area is shown along with the distribution of landslides in (b). At higher altitude levels on a slope, even the lower order drainage during the rainy season may adversely affect the slope stability, while at lower altitudes the drainage may cause toe erosion. The influence of drainage may differ at different places depending upon the distance of the place from the actual stream flow. Therefore, in the present work three buffer zones at a successive interval of 50 m on either side of the stream were created by GIS for all of the present drainage. The high drainage influence zone was considered as a 50 m zone on either side of the stream. Similarly, the zones of moderate, low and very low drainage influence were considered as having the distance on either side of the stream as 50–100 m, 100–150 m and > 150 m, respectively ((b)). It has been observed that the highest density of landslide is observed in the moderate influence zone.
3.1.7 Tectonic influence
The Lesser Himalayan rocks have suffered five phases of deformation (Dubey Citation2004). These deformational phases have brought about the development of many pervasive or non-pervasive (Turner and Weiss Citation1963) planes of weakness such as foliation, axial planes of the folds, faults, thrusts, joints and lineaments on varying scales of local and regional dimensions. Some of these planes of weakness, such as Tons Thrust and Deoban Fault () are well recognized and have regional dimensions. However, numerous local planes of weakness recognized from the satellite images as lineaments (O'Leary et al. Citation1976) have substantial effects on the mass movement or slope failure. Presence of numerous old and new landslides and uplifted terraces () suggests that the area is undergoing neotectonic activities. Therefore, mapping of the tectonic planes acquires prime importance. The landslide activities are expected more in zones that fall in the vicinity of these planes and less away from them. In the present work therefore, such tectonic influence zones have been recognized and categorized into four classes depending upon their distance from the surface trace of the tectonic plane (). The very high tectonic influence zone was considered to be the 50 m zone on either side of the trace of a tectonic plane. Similarly the zones of high, moderate and low tectonic influence were considered as having distances on either side of the tectonic planes as 50–100 m, 100–150 m and > 150 m, respectively. The highest density of landslide is observed in the very high tectonic influence zone.
3.1.8 Landuse/land cover
The land use or land cover has its own positive or negative influence on the slope instability. The vegetation cover, for example, will try to stabilize the slope while the improper land use practices may adversely affect the slope stability. The unsafe landuse practices due to anthropogenic activities may lead to landsliding, even in the otherwise safe areas. Many times the unsafe slopes have been suitably modified for cultivation practices in the area. The different land use/land cover classes have been derived from visual interpretation of false colour composite (FCC) images (). reveals that the areas that are devoid of vegetation (barren land) pose maximum threats to landslides and thus the highest number of active landslide pixels and pixel density have been observed in the barren land. The settlement areas have zero density of active landslide pixels because people try to settle in a safe zone or modify the area to a safe level. Often in the present study area, the settlements are over old stabilized landslide zones as they provide cultivable lands. Anthropogenic activities, such as construction of roads and modifying slopes, sometimes remain unrecorded in toposheets. Moreover, mass movement of smaller dimensions than the spatial resolution of the satellite sensor may be misregistered in satellite images. Therefore, because of the flaws mentioned above, the spatial analyses are liable to be affected by some inaccuracies.
3.2 Crossing of thematic maps with the landslide occurrence map
The study area incorporates various types of slope failure and mass movement, including rotational, translational, rock fall, creep and flow categories of Varnes (Citation1978), Cruden and Varnes (1996) and Hungr et al. (Citation2001). In the present study however, the mapping has been carried out by classifying the landslides as active landslide, creep and old landslide (). From the total 135.4 km2 study area, 1.65 km2 is covered under active landslide zones. A landslide has been categorized as old in the present work if its debris has a growth of tall trees over it or good soil has developed over it for agricultural activities. Active landslides, on the other hand, exhibit little vegetation, fresh appearance with a characteristic light blue tone on the FCC. The leaning and/or bending characters of the majority of the tree trunks in an area help to identify a creep zone. The creep zones were identified primarily on the basis of field observation.
The pattern of distribution of these landslides () reveals that most of the old or active landslide activities are located near and parallel to the Tons Thrust and Tons river. This is a remarkable feature that makes a connection of these landslide activities to the phenomenon of thrusting or thrust movement. Thirteen uplifted terraces along the Tons river at the northern side of the Tons thrust () have been observed that exhibit an elevation up to 120 m from the present course of the river. The thickness of the incised bedrock indicates neotectonic uplift (Srivastava and Misra Citation2008) and these fluvial terraces preserve a composite record of regional variation in climate, tectonics and surface processing of sediments (Srivastava et al. Citation2008). The asymmetric nature of the majority of these terraces and their bedrock examinations in the study area suggest that the terraces are uplifted along the pre-existing faults, which have been reactivated perhaps due to neotectonic activities prevalent in the area. The ongoing neotectonism is expected to act as an accelerating factor for landslide activity. The activity is expected to be the most preferred in zones that have the weakest slope stability strengths. Therefore in the present study, an attempt has been made to identify the landslide hazard vulnerable zones based on GIS analysis incorporating the information on the existing landslides in the region.
The main objective of the data integration or model is to combine spatial data from different sources together to describe and analyse interactions in order to make predictions with models. These models may be based (a) entirely on the statistical data that is data driven, a quantitative approach or (b) entirely expert knowledge based that is a qualitative or a knowledge-driven approach in the absence or non-availability of sufficient data. A third category may combine the data-driven and knowledge-driven approaches. In the present work the studies could generate sufficient data from the landslide occurrences in different parameters and their attributes through remote sensing techniques and field work. Therefore, it was decided to use the data-driven approach using bivariate or information value techniques discussed by Yin and Yan (Citation1988) at first, followed by fuzzy based techniques. A flow chart of the methodology adopted in the present study is given in . Yin and Yan (Citation1988), using a probabilistic approach, have shown a way of calculating weights for individual classes of a thematic layer, by assigning an information value, which takes the form
Figure 5. Flow chart depicting methodology for geographical information system (GIS) based landslide hazard zonation.
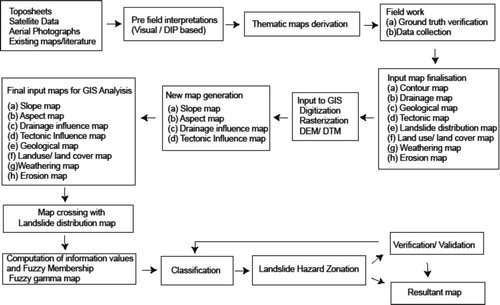
From probability consideration this is equivalent to:
The total information value in a grid unit P is given by the equation
The larger the values of Ip , the greater the risk to the grid P. Parameters having negative information values show negative correlation with landslide events. The information obtained from the known landslide occurrence values were treated with fuzzy logic, which is discussed in the following section.
3.3 Fuzzy membership value for hazard susceptibility zonation
In traditional Boolean set theory an object is completely either in the set (degree of membership is 1), or it is not in the set at all (degree of membership is 0). However, in a fuzzy set, the degree to which an object is a member of a fuzzy set can acquire any value between 0 and 1, rather than being strictly 0 or 1 as in a traditional set. With respect to a given dataset (a thematic map), a membership can be assigned to each map category according to its direct/indirect relationship with the landslide phenomenon. Thus, in the present consideration, a fuzzy set can be interpreted as a set A consisting of all pixels (p∈A) where the proposition of a pixel contained landslide prone area is likely to be true. The membership function μ: A[0,1] represents ‘grades of membership’ or degrees of compatibility of each member (here each pixel value) with the set of landslide prone area. This membership function is also interpreted as a ‘possibility function’ (Zadeh Citation1978) of p, where the proposition is likely to be true.
The fuzzy membership values in the present analysis have been derived from the information values because it was observed for most of the thematic units that information values support the field evidence. These values reflect the spatial statistical relationship and are objectively obtained from simple statistical measures. At the first step all the thematic classes of individual maps have been considered an independent piece of information and their information values were rescaled to fuzzy membership values from 0 to 1. A selection of the lowest and highest information values was most critical because the highest and lowest need to be transferred to 1 and 0, respectively and the original information value 0 should obtain a fuzzy membership value of 0.5, indicating highest uncertainty. Some of the attributes of different thematic parameters contained zero active landslide pixels, so their pixel density in a strict sense will be zero. However, the zero density value has been modified to 0.00001 in order to avoid complete negligence of the concerned attribute in LHZ analysis.
Finally, fuzzy membership values for all thematic units were obtained simply by stretching the values defining the lower and higher limits to fuzzy ranges of 0 and 1. Some of the membership values are changed suitably on the basis of field observations, such as Deoban limestone and Blaini Formations, to represent the possibility of having landslides in these areas. Values of very low influence zone class of drainage were changed to 0.005 as other causative factors are favourable. The final fuzzy membership used (under GIS) for different themes in the present study is shown in . Thus, factor maps were converted to fuzzy evidence maps by using suitable membership values (partly derived from original data) by the process known as fuzzification, which is a data driven-cum-knowledge driven approach and allows expert knowledge regarding the field conditions and their relative importance to be used.
Figure 6. Bar diagrams depicting the thematic map-parameters used for landslide hazard zonation and fuzzy membership values of their attributes. The attribute classes are provided with ‘number of active land slide pixels’/‘total number of pixels in that class’.
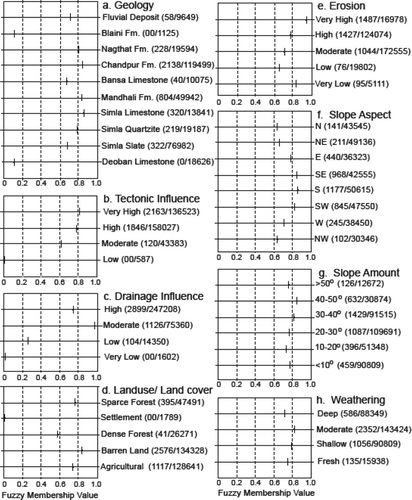
3.3.1 Landslide hazard zonation using fuzzy gamma
Various thematic data layers, represented by respective fuzzy membership values (), may now be combined using the standing rules of fuzzy algebra. Zimmermann (Citation1985) and An et al. (Citation1991) discussed five such operators that can be useful for combining exploration datasets, namely Fuzzy AND, Fuzzy OR, Fuzzy Algebraic Product (FAP), Fuzzy Algebraic Sum (FAS) and Fuzzy Gamma Operator. These operators were tried in the present study for landslide hazard zonation mapping in a GIS. During FAP and FAS operations it was noticed that the average fuzzy resultant values were very low and high, respectively. This may be because eight different thematic maps were integrated and each map represented a value between these two extreme values. The fuzzy gamma operator allows a judicious choice of gamma that can produce an output that ensures a flexible compromise between the ‘increasing’ trend of FAS and the ‘decreasing’ effect of FAP (Bonham-Carter Citation1994). The fuzzy gamma operation (at γ = 0.9) in landslide hazard zonation in the Kakan catchment area in southwest Iran was applied by Tangestani (Citation2004) and γ = 1 by Kanungo et al. (Citation2009) in the Darjeeling area of eastern Himalaya. Champatiray et al. (Citation2007) also successfully used the fuzzy gamma operation for landslide hazard assessment in the Uttarkashi region of the Himalaya. In the present work, therefore, the Fuzzy Gamma Operator was experimented with, changing the gamma (γ) values in the range of 0 and 1 using the following formula:
In the present study, as stated earlier, different gamma values were considered, however, the γ value 0.8 produced the most satisfying gamma map, which is later used for LHZ mapping into five hazard classes. For the purpose of classification the entire range of fuzzy gamma values of the map were considered as a column of 0 to 100%, to be sliced into five category zones. The bottom and top 10% of this column were considered as the ‘very low hazard’ and ‘very high hazard’ classes, respectively. The rest of the column was further sliced at 60% and 80% levels to obtain ‘low’ (10–60%), ‘moderate’ (60–80%) and ‘high’ (80–90%) hazard classes. Now the fuzzy gamma map is in five different class intervals, having respective values of <0.751622, 0.751622–0.796610, 0.796610–0.801486, 0.801486–0.803855 and >0.803855. These class intervals were selected as they produced the most satisfactory resultant map as per field verifications by the authors in selected parts of the study areas. The resultant landslide hazard zonation map is given in .
4 Discussion and conclusion
The present study derived the fuzzy membership values of known landslide occurrences into eight different parameters, as discussed above. There are 46 numbers of attribute classes all together that were treated with the existing active landslide information. Therefore, the resultant map has been analysed for its validation of the spatial location of different hazard classes by calculating the percentage of active landslides falling in the different hazard classes of the resultant map. For this purpose, a cluster of active landslide (including creep) pixels, which are interconnected, were considered as a single number of active landslides, regardless of the number of active landslide pixels in the cluster. Thus, the locations of 56 numbers of active landslides present in the area were superimposed over the resultant LHZ map (). The active landslide location of the area may fall over any one, or more than one, of the hazard classes of the resultant LHZ map. It is taken as an assumption that a landslide, when originated in a very high hazard zone, will affect the nearby lower categories of the hazard zone too. The affected zone of the other LHZ classes in the map however, will depend upon the actual sizes of the active landslide zone and the affected hazard class on the LHZ map. Therefore, only the highest category of the LHZ map that an active landslide touches is considered in counting for the validation purpose. Plotting of the 56 active landslides and their analysis in this way is presented in the form of a bar diagram in , which reveals that the highest percentage (64.4%) of active landslides is triggered in the ‘very high hazard zone’. The figure goes up as high as 84% if the high hazard class (19.6%) is also added. This may be considered as a good validation of the LHZ map as an outcome of GIS analysis. The occurrence of landslides in moderate, low and very low hazard zones (10.7%, 3.5% and 1.8%, respectively) indicates the possible effects of anthropogenic activities.
Figure 8. Bar diagram depicting the percentage of active landslides in different landslide hazard classes from .
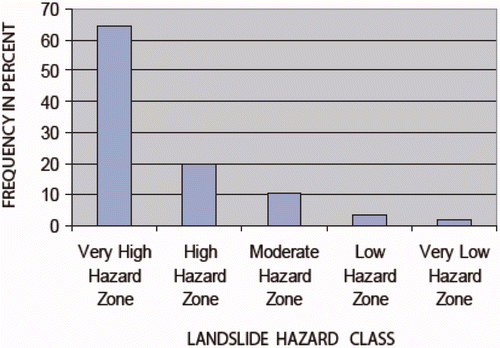
The ongoing convergence and collision of the Indian plate with the Eurasian plate has kept Himalaya as the youngest mountain of the world that is still rising. According to Burbank (Citation2005), the northernmost Lesser Himalaya may have been undergoing deformation since the last 6 million years, suggesting the Lesser Himalayan region is one of the most neotectonically active zones. Frequent seismicity (Singh et al. Citation1996, Citation1999, Dash et al. Citation2000, Pant and Paul Citation2007) in the form of major earthquakes (Uttarkashi earthquake 1991, Chamoli earthquake 1999) and landslides (at Malpa 1998, Nanchani 2009 in Pithoragarh district, at Varunavrat 2003 in Uttarkashi district of Uttarakahand state) substantiate for the active tectonics in the Lesser Garhwal Himalayan region (Pandey Citation2006). One of the most hazardous implications of neotectonic activities is landslides (Shroder and Bishop Citation1998). The zones occurring in the vicinity of the Tons thrust have been the most affected as suggested by the distribution of the majority of old and new landslides in the vicinity of the Tons thrust (, ). The pre-existing transverse and longitudinal faults provide an easy site of displacement by reactivation. Neotectonic activity is an important kinematic force but not the sole factor for the mass wasting phenomenon. In the present study therefore, due emphasis is given to landslide hazard zonation, utilizing existing information on active landslides. The eight highest landslide favouring factors, which have been recognized in the present study, are the Simla Limestone geological unit from the geological map, the 40° to 50° slope from the slope amount map, the southern slope aspect from the slope aspect map, the moderately weathered zone from the weathering map, the very high erosion zone from the erosion map, the moderate drainage influence zone from the drainage influence map, the very high tectonic influence zone from the tectonic influence map and barren land from the land use/land cover map ().
The utility of a LHZ map is expected to plan and control the disastrous effects associated with landslides during the developmental process of a region. Though the expectation would be to provide information about the spatial and temporal probability, type, magnitude, velocity run-out distances etc. of the mass movement, it is difficult to produce such detailed LHZ maps and in most of the cases only relative indicators such as high, medium or low are given regarding the hazard (van Westen Citation1993). A geomatics based landslide hazard zonation map is presented that has been validated with existing information of the active landslides in the area. The present work recommends the ‘very low’ and ‘low’ landslide hazard susceptibility areas from the GIS output map () for any sustainable development in this region.
Acknowledgements
This work has been carried out at the Department of Geology, Banaras Hindu University and the Indian Institute of Remote Sensing, Dehradun, India. The support provided under DST project (ESS/16/242/2005/Kameng/02) is thankfully acknowledged. The authors would like to thank Mr R.K. Chandra and Mr S. Gautam for their help. The authors also thank two anonymous reviewers for their comments and suggestions on the earlier version of the manuscript.
References
- Abella , E. A.C. and Van Westen , C. J. 2008 . Qualitative landslide susceptibility assessment by multicriteria analysis: a case study from San Antonio del Sur, uantánamo, Cuba . Geomorphology , 94 : 453 – 466 .
- Alexander , D. E. 2008 . A brief survey of GIS in mass-movement studies, with reflections on theory and methods . Geomorphology , 94 : 261 – 267 .
- An , P. , Moon , W. M. and Renez , A. 1991 . Application of fuzzy set theory for integration of geological, geophysical and remote sensing data . Canadian Journal of Exploration Geophysics , 27 : 1 – 11 .
- Anbalagan , R. 1992 . Landslide hazard evaluation and zonation mapping in mountainous terrain . Engineering Geology , 32 : 269 – 277 .
- Arora , M. K. , Dasgupta , A. S. and Gupta , R. P. 2004 . An artificial neural network approach for landslide hazard zonation in the Bhagirathi (Ganga) valley, Himalayas . International Journal of Remote Sensing , 25 : 559 – 572 .
- Auden , J. B. 1937 . Structure of the Himalaya in Garhwal . Records of the Geological Survey of India , 71 : 407 – 433 .
- Ayalew , L. , Yamagishi , H. and Ugawa , N. 2004 . Landslide susceptibility mapping using GIS-based weighted linear combination, the case in Tsugawa area of Agano River, Niigata Prefecture, Japan . Landslides , 1 : 73 – 82 .
- Ayalew , L. and Yamagishi , H. 2005 . The application of GIS-based logistic regression for landslide susceptibility mapping in Kakuda-Yahiko Mountains, Central Japan . Geomorphology , 65 : 15 – 31 .
- Baeza , C. and Corominas , J. 2001 . Assessment of shallow landslide susceptibility by means of multivariate statistical techniques . Earth Surface Processes and Landforms , 26 : 1251 – 1263 .
- Binaghi , E. , Luzi , L. , Madella , P. , Pergalani , F. and Rampini , A. 1998 . Slope instability zonation: a comparison between certainty factor and fuzzy Dempster–Shafer approaches . Natural Hazards , 17 : 77 – 97 .
- Bonham-Carter , G. F. 1994 . Geographical Information System for Geoscientists: Modelling with GIS , 292 – 302 . New York : Pergamon Press .
- Burbank , D. W. 2005 . Cracking the Himalaya . Nature , 434 : 963 – 964 .
- Carrara , A. , Cardinali , M. , Detti , R. , Guzzetti , F. , Pasqui , V. and Reichenbach , P. 1991 . GIS techniques and statistical models in evaluating landslide hazard . Earth Surface Processes and Landforms , 16 : 427 – 445 .
- Carrara , A. , Cardinali , M. , Guzzetti , F. and Reichenbach , P. 1995 . “ GIS technology in mapping landslide hazard ” . In Geographical Information System in Assessing Natural Hazard , Edited by: Carrara , A. and Guzzetti , F. 135 – 175 . Dordrecht : Klummer-Nijholf .
- Carrara , A. , Crosta , G. and Frattini , P. 2003 . Geomorphological and historical data in assessing landslide hazard . Earth Surface Processes and Landforms , 28 : 1125 – 1142 .
- Champatiray , P. K. , Dimri , S. , Lakhera , R. C. and Sati , S. 2007 . Fuzzy based method for landslide hazard assessment in active seismic zone of Himalaya . Landslides , 4 : 101 – 111 .
- Chang , K. , Chiang , S. and Hsu , M. 2007 . Modeling typhoon- and earthquake-induced landslides in a mountainous watershed using logistic regression . Geomorphology , 89 : 335 – 347 .
- Chung , C. F. , Fabbri , A. G. and van Westen , C. J. 1995 . “ Multivariate regression analysis for landslide hazard zonation ” . In Geographical information system in assessing natural hazard , Edited by: Carrara , A. and Guzzetti , F. 107 – 134 . Dordrecht : Kluwer Academic Press .
- Chung , C. J.F. and Fabbri , A. G. 1999 . Probabilistic prediction models for landslide hazard mapping . Photogrammetric Engineering and Remote Sensing , 65 : 1389 – 1399 .
- Chung , C. J.F. and Fabbri , A. G. 2003 . Validation of spatial prediction models for landslide hazard mapping . Natural Hazards , 30 : 451 – 472 .
- Chung , C. J. and Fabbri , A. G. 2008 . Predicting landslides for risk analysis – spatial models tested by a cross-validation technique . Geomorphology , 94 : 438 – 452 .
- Cruden , D. M. and Varnes , D. J. 1996 . “ Landslide types and processes ” . In Landslides: Investigation and Mitigation , Edited by: Turner , A. K. and Shuster , R. L. 36 – 75 . Washington, DC : Transportation Research Board, National Research Council . Special Report, 247
- Das , I. , Sahoo , S. , Van Westen , C. , Stein , A. and Hack , R. 2010 . Landslide susceptibility assessment using logistic regression and its comparison with a rock mass classification system, along a road section in the northern Himalayas (India) . Geomorphology , 114 : 627 – 637 .
- Dash , P. , Singh , R. P. and Voss , F. 2000 . Anomalous stress patterns in Chamoli Region observed from IRS-1b data . Current Science , 78 : 1066 – 1070 .
- Dubey , A. K. 2004 . Structural evolution of the Himalaya: field studies, experimental models and implications for seismicity . Himalayan Geology , 25 : 33 – 50 .
- Ermini , L. , Catni , F. and Casagli , N. 2005 . Artificial neural networks applied to landslide susceptibility assessment . Geomorphology , 66 : 327 – 343 .
- Fabbri , A. G. , Chung , C. J. , Cendrero , C. and Remondo , J. 2003 . Is prediction of future landslides possible with a GIS? . Natural Hazards , 30 : 487 – 503 .
- Gansser , A. 1964 . Geology of the Himalaya , London : Interscience .
- Gee , M. D. Classification of landslide hazard zonation methods and a test of predictive capability . Proceedings of the 6th International Symposium on Landslides . Christchurch. Vol. 2 , pp. 947 – 952 .
- Glade , T. , Crozier , M. and Smith , P. 2000 . Applying probability determination to refine landslide triggering rainfall threshold using an empirical “Antecedent Daily Rainfall Model” . Pure and Applied Geophysics , 157 : 1059 – 1079 .
- Gorsevski , P. V. , Gessler , P. E. and Jankowski , P. 2003 . Integrating a fuzzy k -means classification and a Bayesian approach for spatial prediction of landslide hazard . Journal of Geographical Systems , 5 : 223 – 251 .
- Gorsevski , P. V. , Jankowski , P. and Gessler , P. E. 2005 . Spatial prediction of landslide hazard using fuzzy k-means and Dempster–Shafer theory . Transactions in GIS , 9 : 455 – 474 .
- Gupta , R. P. , Saha , A. K. , Arora , M. K. and Kumar , A. 1999 . Landslide hazard zonation in parts of Bhagirathi valley, Garhwal Himalaya, using integrated remote sensing – GIS . Himalayan Geology , 20 : 71 – 85 .
- Guzetti , F. , Carrara , A. , Cardinali , M. and Reichenbach , P. 1999 . Landslide hazard evaluation: a review of current techniques and their application in a multi-scale study, Central Italy . Geomorphology , 31 : 181 – 216 .
- Hungr , O. , Evans , S. G. , Bovis , M. and Hutchinson , J. N. 2001 . Review of the classification of landslides of the flow type . Environmental and Engineering Geoscience , VII : 221 – 238 .
- Jade , S. 2004 . Estimates of plate velocity and crustal deformation in the Indian subcontinent using GPS geodesy . Current Science , 86 : 1443 – 1448 .
- Johnson , D. E. 1998 . Applied Multivariate Methods for Data Analysis , Behmont : Duxbury Press .
- Kanungo , D. P. , Arora , M. K. , Sarkar , S. and Gupta , R. P. 2006 . A comparative study of conventional, ANN black box, fuzzy and combined neural and fuzzy weighting procedures for landslide susceptibility zonation in Darjeeling Himalayas . Engineering Geology , 85 : 347 – 366 .
- Kanungo , D. P. , Arora , M. K. , Sarkar , S. and Gupta , R. P. 2009 . A fuzzy set based approach for integration of thematic maps for landslide susceptibility zonation . Georisk , 3 : 30 – 43 .
- Keinholz , H. 1978 . Maps of geomorphology and natural hazards of Grindelwald, Switzerland, scale 1:10000 . Arctic and Alpine Research , 10 : 169 – 184 .
- Lee , E. M. and Jones , D. K.C. 2004 . Landslide Risk Assessment , London : Thomas Telford .
- Lee , S. 2004 . Application of likelihood ratio and logistic regression models to landslide susceptibility mapping using GIS . Environmental Management , 34 : 223 – 232 .
- Lee , S. 2005 . Application of logistic regression model and its validation for landslide susceptibility mapping using GIS and remote sensing data . International Journal of Remote Sensing , 26 : 1477 – 1491 .
- Lee , S. and Pradhan , B. 2006 . Probabilistic landslide hazards and risk mapping on Penang Island, Malaysia . Journal of Earth System Science , 115 : 661 – 672 .
- Lee , S. and Pradhan , B. 2007 . Landslide hazard mapping at Selangor, Malaysia using frequency ratio and logistic regression models . Landslides , 4 : 33 – 41 .
- Lee , S. , Chwae , U. and Min , K. 2002 . Landslide susceptibility mapping by correlation between topography and geological structure: the Janghung area, Korea . Geomorphology , 46 : 149 – 162 .
- Lee , S. , Ryu , J. H. , Min , K. and Won , J. S. 2003 . Landslide susceptibility analysis using GIS and artificial neural network . Earth Surface Processes and Landforms , 28 : 1361 – 1376 .
- Lee , S. , Ryu , J. H. , Won , J. S. and Park , H. J. 2004 . Determination and application of the weights for landslide susceptibility mapping using an artificial neural network . Engineering Geology , 71 : 289 – 302 .
- Mathew , J. , Jha , V. K. and Rawat , G. S. 2007a . Weights of evidence modelling for landslide hazard zonation mapping in part of Bhagirathi valley, Uttarakhand . Current Science , 92 : 628 – 638 .
- Mathew , J. , Jha , V. K. and Rawat , G. S. 2007b . Application of binary logistic regression analysis and its validation for landslide susceptibility mapping in part of Garhwal Himalaya, India . International Journal of Remote Sensing , 28 : 2257 – 2275 .
- Melchiorre , C. , Matteucci , M. , Azzoni , A. and Zanchi , A. 2008 . Artificial neural networks and cluster analysis in landslide susceptibility zonation . Geomorphology , 94 : 379 – 400 .
- Nichol , J. E. , Shaker , A. and Wong , M. S. 2006 . Application of high-resolution stereo satellite images to detailed landslide hazard assessment . Geomorphology , 76 : 68 – 75 .
- NRSA . 2000 . Methodology Manual for Landslide Hazard Zonation Mapping , Hyderabad, , India : Technical document, National Remote Sensing Agency, Department of Space .
- NRSA . 2001 . Landslide hazard zonation mapping along the corridors of the pilgrimage routes in Uttaranchal Himalaya , Hyderabad, , India : Technical document, NRSA, Department of Space .
- O'Leary , D. W. , Friedman , J. D. and Pohn , H. A. 1976 . Lineament, linear and lineation: some proposed new standards for old terms . Geological Society of America Bulletin , 87 : 1463 – 1469 .
- Pachauri , A. K. and Pant , M. 1992 . Landslide hazard mapping based on geological attributes . Engineering Geology , 32 : 81 – 100 .
- Pandey , R. K. 2006 . Landslide problem in Uttaranchal, India: issues and challenges . Disaster Prevention and Management , 15 : 247 – 255 .
- Pant , C. C. and Paul , A. 2007 . Recent trends in seismicity of Uttaranchal . Journal of the Geological Society of India , 70 : 619 – 626 .
- Pradhan , B. and Lee , S. 2009 . Landslide risk analysis using artificial neural network model focussing on different training sites . International Journal of Physical Sciences , 4 : 1 – 15 .
- Pradhan , B. , Singh , R. P. and Buchroithner , M. F. 2006 . Estimation of stress and its use in evaluation of landslide prone regions using remote sensing data . Advances in Space Research , 37 : 698 – 709 .
- Pradhan , B. , Lee , S. and Buchroithner , M. F. 2009 . Use of geospatial data and fuzzy algebraic operators to landslide-hazard mapping . Applied Geomatics , 1 : 3 – 15 .
- Ruff , M. and Czurda , K. 2008 . Landslide susceptibility analysis with a heuristic approach in the Eastern Alps (Vorarlberg, Austria) . Geomorphology , 94 : 314 – 324 .
- Rupke , J. 1974 . Stratigraphy and structural evolution of the Kumaon Lesser Himalaya . Sedimentary Geology , 11 : 81 – 265 .
- Rupke , J. , Cammeraat , E. , Seijmonsbergen , A. C. and Van Westen , C. J. 1988 . Engineering geomorphology of the Widentobel catchment, Apenzell and Sankt Gallen, Switzerland: a geomorphological inventory system applied to geotechnical appraisal of slope stability . Engineering Geology , 26 : 33 – 68 .
- Saaty , T. L. 1980 . The Analytical Hierarchy Process , New York : McGraw Hill .
- Saha , A. K. , Gupta , R. P. and Arora , M. K. 2002 . GIS-based landslide hazard zonation in Bhagirathi (Ganga) valley, Himalayas . International Journal of Remote Sensing , 23 : 357 – 369 .
- Sarkar , S. and Kanungo , D. P. 2004 . An integrated approach for landslide susceptibility mapping using remote sensing and GIS . Photogrammetry Engineering and Remote Sensing , 70 : 617 – 625 .
- Shroder , J. F. JR. and Bishop , M. P. 1998 . Mass movement in the Himalaya: new insight and research directions . Geomorphology , 26 : 13 – 35 .
- Singh , R. P. , Aman , A. and Prasad , Y. J.J. 1996 . Attenuation relations for strong seismic ground motion in the Himalayan region . Pure and Applied Geophysics , 147 : 161 – 180 .
- Singh , R. P. , Singh , U. K. , Aman , A. and Prasad , Y. J.J. 1999 . “ Attenuation relation for the Himalayan region and seismic hazards at Tehri Dam ” . In Proceeding of the Golden Jubilee Seminar on Exploration Geophysics in India 497 – 500. . Special Publication no. 49 (Calcutta: Geological Survey of India)
- Soeters , R. and Van Westen , C. J. 1996 . “ Slope instability recognition, analysis and zonation ” . In Landslides: Investigation and Mitigation , Edited by: Turner , K. A. and Schuster , R. L. 129 – 177 . Washington, DC : National Academy Press . Transportation Research Board National Research Council, Special Report no. 247
- Srikantia , S. V. and Bhargava , O. N. 1974 . The ‘Jaunsar’ problem in the Himalaya, a critical analysis and elucidation . Journal of the Geological Society of India , 4 : 115 – 138 .
- Srivastava , P. and Misra , D. K. 2008 . Morphosedimentary records of active tectonics at the Kameng river exit, NE Himalaya . Geomorphology , 96 : 187 – 198 .
- Srivastava , P. , Tripathi , J. K. , Islam , R. and Jaiswal , M. K. 2008 . Fashion and phases of late Pleistocene aggradation and incision in the Alaknanda river valley, western Himalaya, India . Quaternary Research , 70 : 68 – 80 .
- Srivastava , V. and Lakhera , R. C. 2007 . “ Geology, structure and tectonics of the Kwanu-Shillai area, Uttaranchal- Himachal Pradesh, using remote sensing techniques ” . In Himalaya (Geological Aspects) , Edited by: Saklani , P. S. Vol. 5 , 229 – 264 . New Delhi : Satish Serial .
- Tangestani , M. H. 2004 . Landslide susceptibility mapping using the fuzzy gamma approach in a GIS, Kakan catchment area, southwest Iran . Australian Journal of Earth Sciences , 51 : 439 – 450 .
- Turner , F. J. and Weiss , L. E. 1963 . Structural Analysis of Metamorphic Tectonites , New York : McGraw Hill .
- Van Westen , C. J. 1993 . Application of Geographic Information Systems to Landslide Hazard Zonation , Vol. 15 , Enschede : ITC .
- Varnes , D. J. 1978 . “ Slope movement types and processes ” . In Landslides, Analysis and Control , Edited by: Schuster , R. L. and Krizek , R. J. 11 – 33 . Washington D.C.: National Academy of Sciences . Transportation Research Board Special Report Number 176
- Varnes , D. J. 1984 . Landslide hazard zonation: a review of principles and practice . Natural Hazards , 3 : 1 – 63 . (Paris: UNESCO)
- Yin , K. L. and Yan , T. Z. 1988 . “ Statistical prediction model for slope instability of metamorphosed rocks ” . In Proceedings of the 5th International Symposium on Landslides , Vol. 2 , 1269 – 1272 . Lausanne, Rotterdam : Elsevier .
- Zadeh , L. A. 1978 . Fuzzy sets as a basis for a theory of possibility . Fuzzy Sets and Systems , 1 : 3 – 28 .
- Zimmermann , H. J. 1985 . Fuzzy Set Theory and its Applications , Dordrecht : Klummer-Nijholf .