Abstract
The Normalized Difference Vegetation Index (NDVI) was used for the analysis of ecological characteristics of the northwestern Black Sea region, which includes significant coastal territories and sea water areas along the northwest coast of the Black Sea. In the present study the following data were used: (1) NDVI and Vegetation Health Index (VHI) spatial distribution data for the period 1997–2008 for southern areas of the Ukraine around the Black Sea (prepared in the USA from NOAA's GVI2 dataset), (2) 1998–2008 NDVI data derived from MODIS (Aqua and Terra) visible channels and SeaWiFS scanners. It is shown that in the integrated land–marine ecosystem in the coastal zone, NDVI can be used as an indicator for hazards such as hypoxia, hydrosulphuric infection and eutrophication.
1. Introduction
Natural disasters in marine and coastal zones such as hypoxia, hydrosulphuric infection, eutrophication and pollution of sea water with industrial and household waste produce an unhealthy water environment. This affects fisheries, tourism, recreation, aquaculture and population health. Control of unhealthy conditions and their consequences for human activities in the coastal zone is a very difficult problem because land and marine ecosystems have not been studied comprehensively as an integrated system. In addition, difficulties in monitoring and predicting unhealthy conditions appear to be due to the absence of high frequency (both spatial and temporal) observations in the area, the absence of models to forecast the indicated natural disasters and impacts and no timely data collection, use and interpretation.
Traditionally, land and marine ecosystems have been studied as independent objects. Land experts have normally masked water areas just as marine ecosystem experts similarly masked the land. Meanwhile, it is a proven fact that marine and land ecosystems are common with physical and biophysical links between them. However, a lack of observations in the coastal zone and no models linking the two ecosystems and their impacts limit investigation and applications in the combined ecosystem.
In recent years, accumulated satellite data together with ground measurements helped to overcome these shortcomings, advancing investigation of the links between land and marine ecosystems and development of an integrated land–marine system in the coastal zone. In the present study, we combine such observations with a modelling approach to investigate physical and biophysical interactions in the coastal zone ecosystems. The area of investigation was the northwestern part of the Black Sea in the southwestern Ukraine. The objectives of this study were: (a) to investigate the interrelation between land and Black Sea ecosystems; (b) correlation analysis of satellite-based marine characteristics with optical-biological parameters of that zone; and (c) to develop a model for forecasting the benthonic hypoxia from the satellite-based marine and land characteristics.
2 Environment and socioeconomics of the studied area
The southwestern part of the Ukraine, where the land borders the Black Sea, was selected as an area of investigation. Land was represented by a 100–200 km zone north of the Black Sea shore and the marine ecosystem was represented by a 60–90 km zone south of the Black Sea shore. These areas were characterized by the following environmental features: continental climate, strong influence of drain from large rivers and intensive human activities contaminating the environment.
The average temperature during January varies in the range of –2 to −7°C and 22 to 30°C during July and the annual precipitation varies in the range 350 to 400 mm; during drought years the precipitation is observed around 150 mm in the steppe zone and 100 mm in the coastal areas of the Azov and Black Seas. A very typical moisture-thermal climatic characteristic is excessive thermal resources to evaporate the available moisture. The deficit reaches up to 400 mm based on the difference between annual precipitation and potential evapotranspiration (Goldsberg 1972). Frequent droughts (every 2–4 years) during warm months are observed in the study area. Moreover, lack or even absence of snow cover during some winters adds to the moisture deficit.
Agriculture in this area is very productive due to the abundance of thermal resources and the best chernozem soils in the world. However, lack of natural water triggers intensive irrigation application, which contributes to the drainage of minerals from the soil into the marine ecosystem. During the past 70 years, intensive agriculture caused considerable exploitation of land in the area (as well as in the entire Ukraine) leading to land degradation, which also contributed to ecosystem changes and contamination of the sea water near the shore.
Two large rivers, the Danube and Dnepr, inject a considerable amount of drainage material (suspended matter, industrial chemicals, agriculture fertilizers and herbicides etc.) into the marine ecosystem. In addition, numerous small rivers also contribute to the contamination of the coastal zone, which is represented by an extensive shelf with depths up to 200 m, the optical-biological and ecological characteristics of which are changing following variations in weather conditions. Considerable population concentration in the area stimulates development of industry. Moreover, the Black Sea coast and warm summers attract large numbers of tourists into the area, which is another factor contributing to the contamination of the coastal zone. Therefore, anthropogenic factors considerably influence the coastal zone (Vinogradov et al. 1992, Orlova et al. 1999, 2007).
3 Data and methods
One of the principle parameters that has been widely used in the past 30 years to characterize land ecosystems and weather conditions is the satellite-based Normalized Difference Vegetation Index (NDVI). This is calculated from satellite reflectances observed in the visible (VIS) and near infrared (NIR) parts of solar spectrum (NDVI = 100(NIR-VIS)/(NIR + VIS)) (Rouse et al. 1973, Richardson and Wiegand 1977, Ricotta and Avena 1999). Several satellite sensors were used to calculate NDVI. First, the Advanced Very High Resolution Radiometer (AVHRR) was used, which measures broad-band reflectance/emission in the VIS (0.4–0.7μm) and NIR (0.7–1.1 μm) wavelengths. The resolutions of these data were: spatial 4 km sampled to 16 km and temporal 7-day composite. Second, MODIS (Aqua and Terra), narrow-band VIS (0.645 μm) and NIR (0.8585 μm) wavelengths at 0.5 km spatial resolution and 2–3 days temporal resolution were used (first level data processing http://ladsweb.nascom.nasa.gov/data/ search.html). These data covered the area of Ukraine with coordinates 44–47.5° N and 28–34.5° E. Third, SeaWiFS data for the same Black Sea area and period with resolution 11 km (third level data processing) and 8-day average values were used to calculate spatial distributions of the chlorophyll-a concentration in the coastal water (http://oceancolor.gsfc.nasa.gov).
Advanced Very High Resolution based VIS and NIR data were pre- and post-launch calibrated before NDVI was calculated. Daily NDVI values were sampled to weekly values using the maximum value composite technique. In addition to NDVI, the daily brightness temperature (BT) from the 10–11μm AVHRR infrared channel (ch4) was retrieved for the day of the week that has the highest NDVI. In order to eliminate high frequency noise (Sun/sensor geometry, limited transparency of the atmosphere, bi-directional reflectance etc.), the 1982–2009 weekly NDVI and BT time series were smoothed using a 5-span sliding median filter together with the least square technique. The processed (low noise) NDVI and BT values were used to calculate the Vegetation Condition Index (VCI) and Temperature Condition Index (TCI) by expressing NDVI and BT as a deviation from the 24-year climatology. Following the low-of-minimum and the low-of-tolerance and the principal of carrying capacity, climatology was presented by the absolute maximum and minimum NDVI (NDVImax, NDVImin) and BT (BTmax, BTmin) calculated from the 24-year NDVI and BT data. Following Kogan (2001) the VCI, TCI and VHI equations are given as.
Moderate Resolution Imaging Spectroradiometer (MODIS) data were pre-processed by soft of Beam VISAT 2.3 and included an extraction of VIS and NIR channels, geolocating each pixel to geographic coordinates. Eight multispectral images of MODIS were processed: for healthy (1999 and 2001 week 18), normal (2004 and 2005 week 30) and stressed (2000, 2001 and 2007 week 30; 2003, week 18) vegetation conditions. To quantify land–sea ecosystem links, cross-sectional land–sea profiles of NDVI were extracted during the 1995–2009 period.
The northwestern Black Sea coastal water is characterized by significant chlorophyll-a concentration and a large amount of dissolved organic chemistry and suspended mineral material. Several algorithms were developed to calculate chlorophyll-a concentration. One of them is based on a correlation between chlorophyll-a concentration (Chl, mg m−3) and colour index, represented by
Figure 1. Correlation between the colour index Iwn (443/555) and chlorophyll-a concentration in the northwestern Black Sea coastal zone (points and approximation line). R is the correlation coefficient.
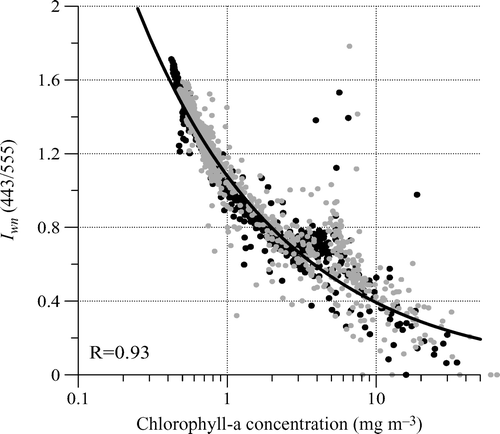
The colour index Iwn (433/555) was used to approximate the depth of visibility in metres of the white disk Zd (measured in metres) (Neymin et al. 1985, Urdenko and Shimerman 1987):
Figure 2. Correlation between NDVI W and (a) chlorophyll-a concentration, Chl = 2509.6(|NDVI W |−1/8574); (b) depth of the white disk visibility (transparency), Zd = 0.0011(|NDVI W |2.2741) and (c) concentration of suspended matter, Cv = 302198 (|NDVI W |−3.0741). Black dots represent Vegetation Health Index (VHI) based healthy vegetation on the land; red dots represent VHI-based vegetation stress; R is the correlation coefficient.
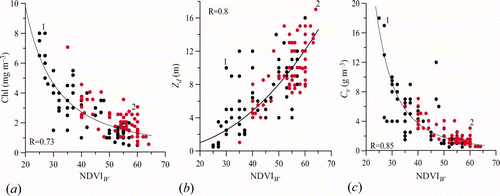
Physical and biological processes in the ‘land–marine’ interactions zone depend on the coastal trapped waves, whose characteristics are defined by the bottom relief. The depth of the coastal zone (h) depends exponentially on the distance to the shore (x) approximated by the following equation.
One of the most adverse phenomena of the marine ecosystem is local hypoxia, which is normally formed in a benthonic layer, a few metres above the seabed. Under the conditions of a high temperature and intensive eutrophication, such layers expand and reach up to about 15 to 20 m from the bottom at a depth of 45–55 m. In the centre of this layer, hydrosulphuric infections are developed, leading to destruction of the benthonic biological communities and large fish mortality. The area of shelf water distribution can be determined from spatial distribution of the WLR for various wavelengths. For example, it is possible to use Lwn data for the 0.67 μm wavelengths as proxy, since it is known that these data are proportional to a concentration of the mineral suspension. This proxy determines the shelf water zone, which is characterized by high turbidity. The NDVI W distribution can also be used as a proxy indicator because it is functionally correlated with chlorophyll-a amount and mineral suspension concentrations. The important advantage of the NDVI W use versus the Lwn (0.67) is its minimal dependence on azimuth and zenith angles of the satellite and Sun, respectively.
Orlova et al. (1999, 2007) have developed a simple statistical hypoxia model (based on long-term experiments) for the northwestern Black Sea shelf, which experiences this phenomenon during the summer:
To understand the links between land ecosystem and long-term Danube and Dnepr river flow, we have used the monthly average data from 1985 to 2006. Danube flow data were received from the Reni hydrological post, which is located in the upper delta. The measurements were aggregated over each month following the Danube hydrometeorological observatory methodology. A similar method was used for the monthly average flow of the Dnepr river measured at the Kahovsky Hydroelectric Power station (Kirilenko et al. 2009).
4 Results and discussion
4.1 Vegetation Health Index (VHI) and chlorophyll-a
The first indication of any links between land and marine ecosystems is examined by comparing land images of the VHI in the land coastal zone with the amount of chlorophyll-a in the water of the northwestern Black Sea. Four cases of VHI were selected: very healthy (1999), normal (2000) and stressed (2001 and 2003). As seen in , when a large land area is covered by moderate (light red) and severe (dark red) vegetation stress (2001 and 2003), normally associated with droughts, the marine area of chlorophyll concentration is much smaller compared to those years with very healthy vegetation (1999, blue colour), which normally occurs when precipitation is above normal. Even in the year 2000, with close to normal VHI (green-yellow), the area is still larger compared to dry years. Larger chlorophyll concentrations in the northwestern Black Sea coastal zone in wet (healthy vegetation) years are associated with much larger drains of contaminants into the marine ecosystem from the Danube and Dnepr rivers. These rivers pollute the marine near the coast each year. But during dry (vegetation stress) years, chlorophyll concentration is much lower.
Figure 3. Advanced Very High Resolution Radiometer (AVHRR) based Vegetation Health Index (VHI) and chlorophyll-a concentration in the northwestern Black Sea for various VHI-based land conditions. In 2001 and 2003, VHI indicated stressed vegetation conditions (red/yellow colour) on the land and the average chlorophyll-a concentrations in the water were 2.51 and 1.61 mg m−3, respectively. In 1999 and 2000, VHI indicated healthy vegetation conditions (blue/green colour) on the land and the average chlorophyll-a concentrations in the water were 3.85 and 3.76 mg m−3, respectively. Available in colour online.
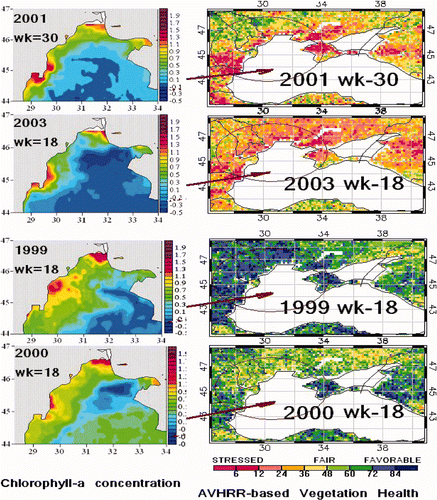
shows a correlation between average chlorophyll concentration in the entire 60–90 km zone (44.7–46.5° N and 29.0–31.5° E) and the average VHI in the 100–150 km land zone for each year during 1998–2008. The corresponding weeks were 18 (mid May) and 30 (end of July) depending on the time when this concentration was derived from the SeaWiFS data. The relationship between these parameters is clearly determined (correlation coefficient 0.77). Low chlorophyll concentration (), is associated with low VHI, which indicates vegetation stress due to dryness. This relationship supports the previously expressed idea about a reduction of contaminants coming from rivers and storm drains into the sea during dry years. It leads to a reduction of the mineral suspension and nutrients in the sea environment and to a corresponding reduction of the chlorophyll-a concentration and biomass of seaweed. The opposite situation develops during years with healthy vegetation, which are associated with above normal rainfall and correspondingly wet conditions. The latter leads to an increase in contaminants from rivers and storm drains, as well as an increase in the quantity of mineral suspensions and nutrients in the sea. All of these cause a growth in seaweed biomass and an increase in chlorophyll-a concentration.
Figure 4. Relationship between average chlorophyll-a concentration in the 60–90 km marine zone of the northwestern Black Sea and land Vegetation Health Index (VHI) in the coastal zone during 1998–2008; VHI = 39.29 ln(Chl) + 12.731 is the approximation of this relationship, R is the correlation coefficient.
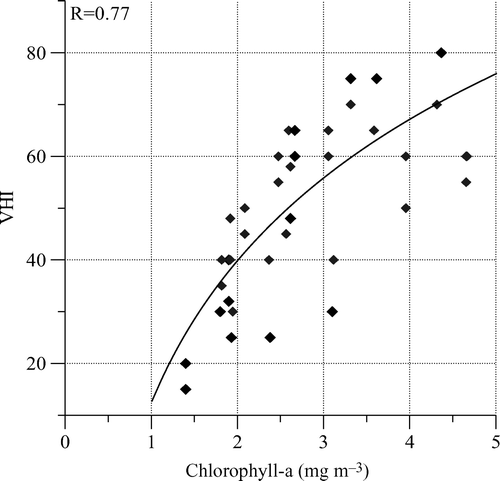
The relationship shown in is supported by the annual analysis of Danube river flow shown in . Variation of the flow (QD) is quite coherent with VHI during spring (mid-May), especially in extreme years (favourable vegetation conditions – 1999, 2001, 2005; stressed conditions – 2003, 2007). Moreover, both QD and VHI variations match well with chlorophyll-a concentration, especially close to the shore zone (60–90 km, channel 2).
Figure 5. Annual variability of river Danube flow (QD), average chlorophyll-a concentration (Chl_1) in the entire northwestern Black Sea (44–47° N and 28.3–34° E), average chlorophyll-a concentration (Chl_2) in the 60–90 km coastal zone (44.7–46.5° N and 29.0–31.5° E) and Vegetation Health Index (VHI) for week 18 (mid-May) during 1997–2007.
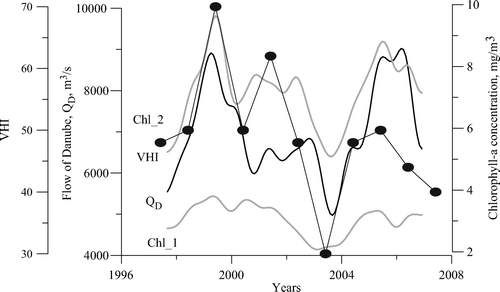
4.2 Interaction between NDVIL and NDVIW
As previously mentioned, NDVI values were calculated along 85 routes on land and sea during stressed and favourable conditions. These results are shown in . The features of the NDVI-based ‘land–marine’ interactions are: (i) sign change transitioning from land to water; (ii) significant correlation (0.634) between NDVI W and NDVI L ; (iii) coherence between land–water NDVI values in the extreme land conditions; (iv) different physical basis of land–water NDVI – NDVI L is a proxy for vegetation health due to healthy-stressed vegetation conditions while NDVI W is a proxy of lesser or lower pollution; (v) prediction ability of satellite-based land vegetation health (conditions) for coastal zone marine pollution. The distance Ld from the coast, for which NDVI W values were calculated, corresponded to a range of the coastal trapped wave distribution, or to the distance of interaction of the land with the marine ecosystems.
Figure 6. Normalized Difference Vegetation Index (NDVI) dynamics over land and marine ecosystems in the near-coastal zone (the coast is at l = 0); black lines represent cases with vegetation stress and grey lines represent cases with healthy vegetation on the land coastal zone.
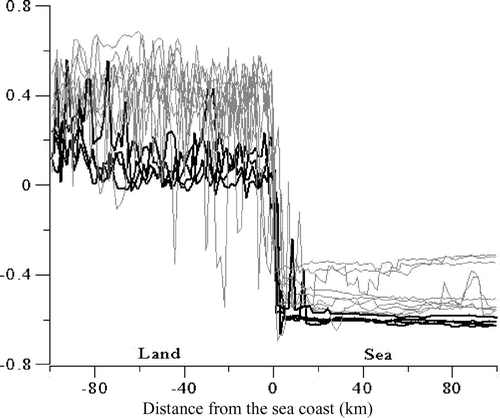
If near-coastal vegetation is stressed, then water NDVI is smaller due to less water turbidity compared to favourable vegetation conditions when water is contaminated by drain materials. During drought-related vegetation stress, the river and storm drains in the sea considerably decrease. This results in a reduction of the mineral suspension and nutrient concentration in the water near the shore and a corresponding reduction in the chlorophyll-a and seaweed biomass: sea water becomes more transparent and homogeneous and NDVI W decreases. The summary diagram in demonstrates the NDVI L and NDVI W relationship. As seen the two groups of point clusters in a low (black dots) and high (grey dots) NDVI values characterizing stressed and favourable conditions, respectively.
4.3 Hypoxia development
Hypoxia (or a ‘dead zone’) is developing in the coastal marine zone due to inadequate oxygen supply. Hypoxia is normally caused by an increase in chemical nutrients (more often nitrogen and phosphorous from leaking agricultural fertilizers into the rivers and from them into the marine coastal zone), which are used by plants and organisms to grow fast and increase their density. This process is followed by an excessive use of oxygen dissolved in water.
shows the dynamics of change in the hypoxia area of the northwestern Black Sea during 1972–2000 (data from Orlova et al., 2007, Shyp_1) and 1998–2008 (calculated from remote sensing data using equation (6), Shyp_2). As seen, the hypoxia area fluctuates considerably from near 0 to 18 km2 during 1972–2000 (Shyp_1). Shyp_2 data are coherent with the earlier measurements fluctuating in the same range. More importantly, this data correlates with the average VHI index in the near coastal zone of the southwestern Ukraine for most of the years. A low hypoxia area is associated with lower VHI, which indicates less favourable vegetation conditions. A near zero hypoxia area was estimated in 2002 and 2003 when VHI was less then 40 for a large area in southwestern Ukraine, indicating the beginning of severe vegetation stress and correspondingly less contaminates coming to the marine ecosystem. A remarkable coincidence is also observed for the three largest hypoxia area years (1999–2001) and VHI, which exceeds 55, indicating very favourable conditions on the land especially in 2001 and 1999, resulting in a considerable drainage of contaminates in the water.
Figure 8. Hypoxia area (left scale, km2) in the northwestern Black Sea from: Shyp_1 based on Orlova et al. (2007) and Shyp_2 based on satellite data (equation (6)) and the average Vegetation Health Index (VHI) (right scale) in the southwestern Ukraine.
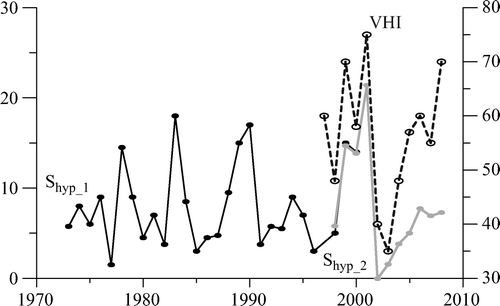
The expression of S hyp = f(VHI) can be used to forecast hypoxia development in the northwestern Black Sea, especially during extreme conditions.
5 Conclusions
-
This paper presented evidence that land and marine ecosystems in the near-coastal zone are closely inter-related: dry conditions on land (100–150 km near the coast) reduce leakage of contaminants into rivers and storm drains and from them into the water in the 60–90 km near-shore zone; the opposite situation occurs in wet years.
-
The AVHRR-based NDVI and VHI are useful indicators characterizing an integrated ‘land–marine’ ecosystem in the coastal zone, especially in the northwestern Black Sea. Since NDVI L and NDVI W correlate with such optic-biological parameters of the water environment, they can be used as the indicators of chlorophyll-a, mineral suspension concentrations and transparency of the upper layer of sea water.
-
There are links between NDVI L and NDVI W , indicating a common environmental tendency on land and in the water: (i) an increase in NDVI indicates healthy vegetation on land and water impurity (due to more mineral suspension and nutrients); (ii) a decrease in NDVI indicates vegetation stress on land (due to drought) and less contaminated coastal zone water (reduction in the transport of suspended matter and a reduction in sea water impurity).
-
One of the unhealthy water environments is hypoxia, which affects fisheries, tourism, recreation, aquaculture and population health. This research indicated that hypoxia in the northwestern Black Sea can be forecast based on AVHRR-derived VHI data.
-
The results linking land and marine ecosystems in the northwestern Black Sea indicates that satellite-based VHI and NDVI methodology can be used in other regions where the river drain appreciably influences the optic-biological structure of the water environment. Examples are the Azov Sea, Chesapeake Bay, Amazon River drain and others.
Acknowledgements
The authors are grateful to the comments made by the two anonymous reviewers.
References
- Goldsberg , I. A. 1972 . Agroclimaticheskii Atlas Mira Moscow-Leningrad ‘Hydrometizdat’
- Kirilenko , N. F. , Kushnir , V. M. and Lemeshko , E. M. 2009 . Influence of a river drain on ecological conditions in northwestern area of Black Sea according to contact measurements and remote sensing data . Geoinformatics , 4 : 23 – 28 .
- Kogan , F. N. 2001 . Operational space technology for global vegetation assessment . Bulletin of the American Meteorological Society , 82 : 1949 – 1964 .
- Kushnir , V. M. and Stanichny , S. V. 2007 . Color index in the Northwestern Black Sea derived from scanner MODIS data . Earth Research from Space , 4 : 62 – 73 .
- Neymin , G. G. , Kushnir , V. M. and Vladimirov , V. L. Spatial distribution, structure and characteristics of “spots” of contrast waters in the Northwestern Tropical Atlantic . Proceedings of a Conference of the Marine Hydrophysical Institute of USSA Academy of Sciences . pp. 12 – 14 . Sevastopol, , Ukraine : Marine Hydrophysical Institute . Sevastopol, Report 5043
- Orlova , I. G. , Belevich , R. R. , Popov , J. I. , Ukrainsky , V. V. and Bondar , S. B. 1999 . Dynamics of the hypoxia processes in benthonic waters in the northwestern shelf of the Black Sea . Oceanology , 39 : 548 – 554 .
- Orlova , I. G. , Pavlenko , N. E. , Ukrainsky , V. V. and Popov , U. I. Condition of eutrophication waters in the northwestern Black Sea as the results of long-term complex monitoring . Proceedings of a Conference on Ecological Safety of Coastal and Shelf Zones and Complex Use of Shelf Resources: EkosiHydrophysics . pp. 32 – 43 . Sevastopol, , Ukraine : Marine Hydrophysical Institute . Sevastopol
- O’Reilly , J. E. , Maritorena , S. , Mitchell , B. G. , Siegel , D. A. , Carder , K. L. , Garver , S. A. , Kahru , M. and McClain , C. 1998 . Ocean color chlorophyll algorithms for SeaWiFS . Journal of Geophysical Research , 103 : 24937 – 24953 .
- Richardson , A. J. and Wiegand , C. L. 1977 . Distinguishing vegetation from soil background information . Photogrammetric Engineering and Remote Sensing , 43 : 1541 – 1552 .
- Ricotta , C. and Avena , G. 1999 . Mapping and monitoring net primary productivity with AVHRR NDVI time-series: statistical equivalence of cumulative vegetation indices . Journal of Photogrammetry and Remote Sensing , 54 : 325 – 331 .
- Rouse , J. W. Jr. , Haas , R. H. , Schell , J. A. and Deering , D. W . 1973 . “ Monitoring the vernal advancement and retrogradation (green wave effect) of natural vegetation ” . 93 – 95 . Remote Sensing Center of the Texas A and M University, College Station . Report RSC 1978-1
- Urdenko , V. A. and Shimerman , G. 1987 . Optical Remote Sensing of the Sea and the Influence of the Atmosphere , Vol. 2 , 197 Berlin-Moscow-Sebastopol : Institute for Space Research, Academy of Sciences of GDR . part 2
- Vinogradov , M. E. , Sapozhnikov , V. V. and Shychina , E. A. 1992 . Ecosystem of the Black Sea , Moskow : Moskow Science .
- Vituk , D. M. 1983 . Weigh Substance and Biogenic Components , Kiev : Naykova Dumka .