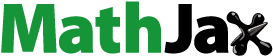
Abstract
Water inrush is a major geological hazard that threatens the safety of karst tunnels. The risk assessment of water inrush is necessary in tunnel engineering. A new model based on intuitionistic fuzzy analysis was proposed to assess the risk of water inrush, which considered the subjective and objective weights of influencing factors. Seven factors were selected to constitute the set of evaluation attributes, namely, formation lithology, unfavourable geological conditions, groundwater level, landform and physiognomy, modified strata inclination, contact zones of dissolvable and insoluble rock, and layer and interlayer fissures. The proposed model was applied in a typical karst tunnel, Xiakou tunnel in China. The sensitivity of the changes in the index weights was analyzed. The results showed that the water inrush risk of section XJK0 + 110 to XJK0 + 060 in Xiakou tunnel was evaluated as grade I (high risk). The risk assessment results were more sensitive to the index weights of landform, formation lithology, and contact zones of dissolvable and insoluble rock than to those of the other indices. Landform was the most sensitive index. The obtained results were identical to the practical findings, and the rationality and feasibility of the model was verified. Intuitionistic fuzzy analysis is a new method for the quantitative risk assessment of water inrush in karst tunnels.
1. Introduction
With the vigorous development of national infrastructure construction, a large number of high-risk deep tunnels have been planned for construction in recent years. These tunnels are characterized by a ‘deep burial depth, long tunnel line, high stress, hydraulic pressure and complex geological structure’. Water inrush is a major geological disaster frequently encountered in the construction of deep tunnels (Bai et al. Citation2006; Jiang et al. Citation2008; Li et al. Citation2015a,Citationb). According to the incomplete statistics, water inrush accounts for approximately 40% of all types of geological disasters.
To date, many scholars have performed a large amount of research on water inrush. According to the different mechanical mechanisms of water inrush (Li et al. Citation2016; Shi et al. Citation2017), the model of water inrush in deeply buried karst tunnels is divided into three categories: water inrush directly caused by excavation, water inrush indirectly caused by excavation, and water inrush behind the excavation face (Li, Citation2009). With the increasing emphasis on the safety of underground engineering, the concept of risk prediction is introduced in tunnel construction and underground engineering (Chen and Huang Citation2005; Huang Citation2006; Wang et al. Citation2009). It has been developed and applied for a series of theories and methods about water inrush forecasting. The evaluation index of water inrush was put forward in tunnels (Li et al. Citation2013a). There are four classical methods for the analysis of water inrush (Kong Citation2011), namely, the Goodman method (Goodman et al. Citation1965), IMS method (McFeat-Smith et al. Citation1985), Heuer and Raymer method (Heuer et al. Citation1995; Raymer et al. 2001) and Heuer Analytical method (Heuer et al. Citation1995, Citation2005). Furthermore, the risk classification system of water inrush and mud inrush was put forward in tunnels, which is combined with a qualitative and quantitative evaluation (Mao et al. Citation2010). An expert evaluation system of water inrush in karst tunnels was established (Han Citation2004; Ye et al. Citation2006; Du et al. Citation2009). The application of the analytic hierarchy process (AHP) has achieved good results in the process of water inrush. The water inrush risk assessment was carried out using attribute recognition theory (Li et al. Citation2013b), efficacy coefficient method, set pair analysis, cloud model, and so on (Wang et al. Citation2014, Citation2016a,b, Citation2017a,Citationb,c, Citation2019).
Water inrush in karst tunnels has been considered as a worldwide problem (Wang Citation2008; Ge Citation2010). Once a disaster happens, the tunnel will be submerged, and the construction will be interrupted. What is worse, water inrush may cause significant casualties and economic losses, and some projects were forced to stop construction or change lines. Therefore, the prediction analysis and safety class evaluation of water inrush in karst tunnels becomes very necessary and urgent in engineering practices.
Many related studies have been performed on the evaluation of water inrush in karst tunnels. However, there are some shortcomings in the above studies. For example, the accuracy of subjective weight methods and the ‘expert judgement system of karst water gushing mainly depend on the decision makers’ practical experience and ability to judge, while the accuracy of the objective weight method depends on the reliability of the data itself. The comprehensive influence of subjective and objective weights on the water inrush of karst tunnels are not considered. Therefore, it is still necessary to further discuss the method of determining the weight of index . In addition, the related sensitivity analysis of the affected indices of water inrush is rarely reported, while many studies have indicated that the index weights have an important effect on the assessment results (Zhao et al. Citation2015).
In view of the shortcomings of current studies, in the present study, the intuitionistic fuzzy model was used on the risk assessment of water inrush, considering the influence of subjective and objective weights. The intuitionistic fuzzy model was applied on the risk assessment of water inrush in the Xiakou tunnel. In addition, the sensitivity of the weight changes in seven main factors influencing water inrush to the risk assessment results of water inrush were analyzed. The proposed model provides a new concept and ideas for the quantitative evaluation of the risk grade of water inrush, which also has a certain practical value for guiding engineering practices.
2. Principle of the intuitionistic fuzzy set
Definition 1 (Gong and Chen Citation2018). Make a nonempty universe , intuitionistic fuzzy sets can be defined as
. Here,
and
are respectively called the membership function and the nonmembership function of
to set A, and meet
,
,
.
Definition 2 (Gong and Chen Citation2018). is intuitionistic fuzzy set of the nonempty universe
, and
is called the intuition coefficient or hesitancy degree of
to set A,
is called the accuracy degree of
to set A,
is called the score function.
If , then
. If
, then
,
;
,
.
3. The technological process of the risk assessment of water inrush based on the intuitionistic fuzzy theory
3.1. The evaluation indices system of water inrush in Karst tunnels
Water inrush is the result of the interaction between tunnel engineering and the water environment of karst tunnels. The water storage capacity of water-bearing strata and karst development are the internal causes of water inrush. Sufficient stored water is a necessary condition of a water inrush incident (Mao et al. Citation2010; Shen et al. Citation2010; Li et al. Citation2011, Citation2013a). Geographic and geomorphic conditions, lithology, rock mass structure, and in situ stress state are regarded as the main factors influencing passageways for water inrush (Zhou et al. Citation2013; Li et al. Citation2013b; Wang et al. Citation2016a, Citation2017, Citation2019). In addition, the depth and location of the tunnel and the excavation method are external causes of water inrush . In this work, seven main influencing factors were selected as evaluation indices, namely, formation lithology, unfavourable geological conditions, groundwater level, landform and physiognomy, modified strata inclination, contact zone of dissolvable and insoluble rocks, and layer and interlayer fissures. The contribution of lithology to water inrush could be determined by rock solubility (t) (Zhou et al. Citation2013) or by the experts’ grading method, while the contributions of unfavourable geological conditions, contact zones of dissolvable and insoluble rock, and layer and interlayer fissures were calculated by the experts’ grading method. Then, the risk assessment of water inrush in karst tunnels could be classified into four grades, namely, grade I (very high), grade II (high), grade III (medium), and grade IV (low), as shown in (Li et al. Citation2011, Citation2013a; Wang et al. Citation2016a).
Table 1. Evaluation indices and risk level standards of water inrush.
3.2. Determination of the attribute set and the scheme set
Assuming that there are m evaluation indices and r risk grades for water inrush in karst tunnels, Y is the attribute set of the water inrush assessment, and W is the grade set of the water inrush assessment. Y and W can be described as follows:
(1)
(1)
3.3. Establishment of the intuitionistic fuzzy set (Wang et al. 2016c)
Assume that there is a universe of a fuzzy set, U, which can be described as following:
(3)
(3)
When y is in the middle of the interval and that is
,
,
;
When y is in the boundary of the interval and that is y = di, the intuitionistic index is the maximum, and the membership degree equals the non-membership degree. Make
,
, then
(4)
(4)
According to the above rules, the membership function uses the typical Gaussian function
(5)
(5)
The non-membership function is transformed from the Gaussian function as
(6)
(6)
The intuition exponential function is
(7)
(7)
In the formula, and
are the parameters of function, are the key to determining the membership degree and non-membership function, can be calculated by the above rules.
(8)
(8)
The mth attribute value of sample P is Ym. The rth grade of scheme set R that Ym corresponds to is the intuitionistic fuzzy number .
3.4. The determination of indices weights
Weights reflect the relative importance of indices in the evaluation process. There are two types of methods to determine the weights (Wang et al. Citation2014, Citation2016b): one is objective methods, such as the Delphi method; the other is subjective methods, including entropy method, principal component analysis, etc. The accuracy of subjective methods is determined by the experiences and judging ability. While the accuracy of objective methods is depend on the reliability of measuring data. In order to accurately calculate the weights of evaluation indices and make sure the effectiveness of the assessment of water inrush risk, an optimal weights method is presented considering both subjective and objective weight information. The Delphi method is used to determine the subjective weight and AHP is used to determine the objective weight.
3.4.1. Determination of the subjective weight (
)
The Delphi method (Wang et al. Citation2016b) is a structured communication technique or method, originally developed as a systematic, interactive forecasting method which relies on a panel of experts. The process of the Delphi method is as follows: (1) select the experts of the relative research field; (2) design forms; (3) seek the opinions of experts; and (4) data processing and determination of the weights. The subjective weights can be calculated as follows,
(11)
(11)
where H is average value of all evaluation values. δ2 is variance, which reflects the degree of decentralization of experts’ opinions, s is the numbers of experts, and ai is the evaluation value of the i-th expert. ai∈[1, 10]. is the subject weight of the i-th evaluation index.
3.4.2. Determination of the objective weight (
)
AHP was presented to determine the objective weights of the evaluation indices. AHP was applied with the following procedure (Saaty 2008; Wang et al. Citation2017c):
An investigated object is selected, and the objective problem was modeled as a hierarchy that contains the decision goal, the alternatives, and the criteria to evaluate the alternatives.
Priorities among the elements of the hierarchy are established through a series of judgments that performs with the pairwise comparisons of the elements.
The obtained judgments are synthesized to yield a set of overall priorities for the hierarchy.
The consistency of the judgments is checked.
A final decision can be gained according to the above processes.
3.4.3. Determination of the comprehensive weight
Combing the subjective weight and the objective weight, the comprehensive weight of each index, , can be calculated as follows:
(14)
(14)
3.5. Risk grade determination of water inrush
Each method has its own assessment criterion. For the intuitionistic fuzzy theory, the maximum subordination principle is selected as its assessment criterion. According to the principle, the maximum intuitionistic fuzzy number corresponds to the risk level of water inrush, which can be obtained as follows:
(15)
(15)
4. Project applications
4.1. Project overview
The Xiakou tunnel (Li et al. Citation2013a; Wang et al. Citation2016a) of the Yichang-Badong expressway is located in Xiakou Town, Xingshan County, Hubei Province, China. The tunnel is a one-way section with two separate lanes. Xiakou tunnel is a deep and extra-long tunnel, with a length of 6.45 km and a maximum overburden thickness of 1500 m.
The mountain in the tunnel construction area comprises carbonate rock intercalated with clastic rock of the Cambrian period and Triassic period. Carbonate rock belongs to soluble rock. The geological conditions and karst hydrologic conditions are extremely complex, with karst depressions at the surface, karst tunnels and sinkholes. Karst develops from ZK106 + 400 to ZK108 + 900 in the left tunnel and from YK106 + 400 to YK108 + 850 in the right tunnel. The engineering geological profile of Xiakou tunnel is shown in . The formation lithology of the inclined shaft from XJK0 + 110 to XJK0 + 060 mainly includes Permian and oolitic limestone intercalated with impure zebra limestone.
Figure 2. Engineering geological profile of Xiakou tunnel (Li et al. Citation2013a).
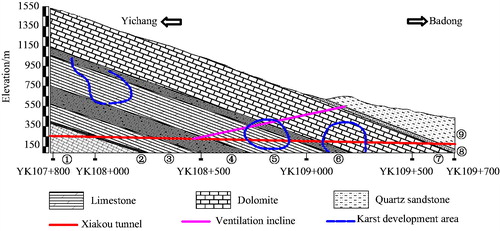
To ulteriorly test the validity and applicability of the presented intuitionistic fuzzy set model, XJK0 + 110 to XJK0 + 060 in the Xiakou tunnel was selected and evaluated with respect to water inrush.
4.2. The analysis of the risk of water inrush in Xiakou tunnel
The standards of quantitative classification () for the assessment indices of water inrush in the tunnel and the sample data of the inclined shaft section of Xiakou tunnel XJK0 + 110 to XJK0 + 060 are used to test the validity and applicability of the presented model. Because the sample data is the interval value, the average value of the interval is used for the convenience of calculation, which is shown in .
Table 2. Average values of evaluation indices.
For Xiakou tunnel from XJK0 + 060 to XJK0 + 110, the intuitionistic fuzzy numbers of the grades of risk assessment on water inrush can be calculated: A1= −0.049, A2= −0.450, A3= −0.761, A4= −0.617. According to the size relationship among the intuitionistic fuzzy numbers, the ordering of all the grades of water inrush can be obtained: A1>A2>A4>A3. Therefore, the risk grade of the water inrush of section XJK0 + 110 to XJK0 + 060 of the inclined shaft of Xiakou tunnel is grade I, which is high risk.
Moreover, intuitionistic fuzzy set theory can be used to analyze the sensitivity of the changes in the index weights. It is shown that among these seven main influential factors, the fuzzy weights of formation lithology (X1), landform and physiognomy (X4), and contact zones of dissolvable and insoluble rock (X6) are smaller than 1, which indicates that the results of the risk assessment of water inrush are sensitive to the determination of these weights. The fuzzy weight of landform and physiognomy (X4) is the lowest, and it is the most sensitive index for the assessment results of water inrush, so its weight value needs to be carefully determined. The fuzziness of four assessment index weights, namely, unfavourable geological conditions (X2), groundwater level (X3), modified strata inclination (X5), and layer and interlayer fissures (X7) are 1, and the changes in their index weights exert relatively smaller influences on the assessment results. The analysis of the fuzziness of index weights shows that the fuzziness of all index weights displays irregularity, and it does not correlate to the size of the index weights.
The on-site actual data show that the two blast holes on the left side of XJK0 + 101 at the tunnel face were gushing water, and the jet distance was approximately 4 m on 7 August 2011. The measurement results showed that the estimated water inflow was approximately 64 m3/h (Li et al. Citation2013a). From February to March 2012, rock fractures developed in section XJK0 + 087 to XJK0 + 067 of the inclined well. In addition, silt filled in the fractures, and the water seepage was approximately 20 m3/h. At 10:20 am, 22 March 2012, the primary support of the sidewalls of section XJK0 + 095 to XJK0 + 090 was broken, causing water inrush. Many water injection points appeared at the left side of the primary support of the section under construction XJK0 + 090 to XJK0 + 080. At 10:30 pm, the primary support of the short sidewall of section XJK0 + 098 to XJK0 + 097 was totally broken and the water burst. Moreover, according to the previous results (Wang et al. Citation2016a), the risk level was also found to be Level I based on the cloud model ().
Figure 3. Water inrush from tunnel face at K0 + 101 in Xiakou tunnel (Li et al. Citation2013a).
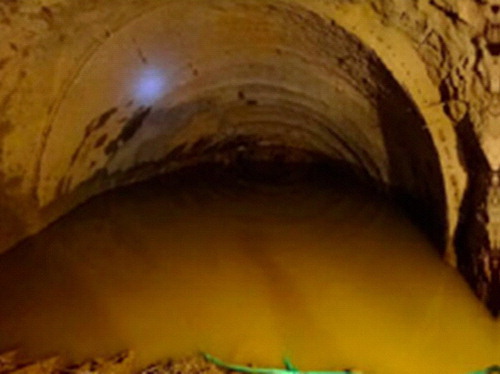
The actual situation of water inrush on site and the evaluation result of the cloud model (Wang et al. Citation2016a) are identical with the assessment results of the intuitionistic fuzzy set, so the rationality and feasibility of the model is verified. The intuitionistic fuzzy set provides a new analytical method and ideas for the quantitative evaluation of risk grades of water inrush in tunnel construction.
5. Conclusions
Water inrush is one of the most common geological disasters and seriously threatens the safety and economy of underground engineering and construction. Therefore, it is very necessary to study the risk prediction of water inrush in tunnels. In this work, the intuitionistic fuzzy model is applied to the field of water inrush, which comprehensively considers the influence of the subjective and objective weight, avoids the limitation of the subjective weight and the objective weight separately, and carries out the fuzzy analysis of the main factors innovatively. The method has a certain reference value for the quantitative evaluation of the water inrush risk level and engineering practices.
Seven main factors of water inrush were selected to construct the intuitionistic fuzzy set of the assessment, namely, formation lithology, unfavourable geological conditions, groundwater level, landform and physiognomy, modified strata inclination, the contact zones of dissolvable and insoluble rock, and layer and interlayer fissures. Comprehensively considering the influence of the subjective and objective weights, the intuitionistic fuzzy evaluation model was established, and the rationality and feasibility of the model was verified by comparing it with the actual water inrush cases and the cloud model.
In this work, the fuzzy weights of the seven main factors affecting water inrush in karst tunnels were analyzed according to the sample data of section XJK0 + 110 to XJK0 + 060 in Xiakou tunnel. The results show that the determination of index weights for formation lithology (X1), landform and physiognomy (X4), and contact zones of dissolvable and insoluble rock (X6) are more sensitive for the results of the risk assessment of water inrush, in which landform and physiognomy is the most sensitive index, while the fuzziness values of the four assessment indices of unfavourable geological conditions (X2), groundwater level (X3), modified strata inclination (X5), and layer and interlayer fissures (X7) are 1, and the changes in index weights have less influence on the results of the assessment. Moreover, the result of the fuzzy analysis of index weight also shows that the fuzzy weight of each index is not regular, which has no correlation to the weight of the index.
Nomenclature | ||
= | The calculation parameter | |
= | The calculation parameter | |
δ2 | = | Variance |
= | The subjective weight of each index | |
= | The objective weight of each index | |
= | The comprehensive weight of each index | |
ai | = | The evaluation value of the i-th expert |
Ar | = | The intuitionistic fuzzy number |
= | The calculation parameter | |
= | The calculation parameter | |
H | = | Average value of all evaluation values |
m | = | Evaluation indices of water inrush |
r | = | Risk grades for water inrush |
s | = | The numbers of experts |
Y | = | The attribute set of the water inrush assessment |
W | = | The grade set of the water inrush assessment |
Disclosure statement
No potential conflict of interest was reported by the authors.
Additional information
Funding
References
- Bai MZ, Xu ZY, Gong LJ. 2006. Study on geological hazards in tunnel construction in complex karst area. Chin J Safety. 16:114–118.
- Chen L, Huang HW. 2005. Risk analysis of rock tunnel engineering. Chin J Rock M Eng. 24:110–115.
- Du YC, Han XR, Li ZL. 2009. Professional evaluating system for karst tunnel gushing based on AHP and its application. Carsol Sin. 28:281–287.
- Ge YH. 2010. Study on risk assessment and early warning mechanism of water inrush in karst tunnel. Jinan: Shandong University.
- Gong F, Chen XM. 2018. Study on the evaluation of ecological livable city in anhui based on intuitionistic fuzzy theory. J Chongqing Technol Business Univ. ( Nat Sci Ed). 35(4): 30–36.
- Goodman R, Moye D, Schalkwyk A, Javendel I. 1965. Ground-water inflow during tunnel drivingEng. Geol Bull IAEG. 2:39–56.
- Han XR. 2004. Karst water bursting in tunnel and expert judging system. Carsol Sin. 23:213–218.
- Heuer R. 1995. Estimating rock tunnel water inflow. Proceedings of Rapid Excavation and Tunnelling Conference. p. 41–60.
- Heuer R. 2005. Estimating rock tunnel water inflow—II. Proceedings of Rapid Excavation and Tunnelling Conference. p. 394–407.
- Huang HW. 2006. State-of- the-art of the research on risk management in construction of tunnel and underground works. Chin J under S Eng. 1:13–20.
- Jiang FX, Ye GX, Wang CW, Zhang DY, Guan YQ. 2008. Application of high-precision microseismic monitoring techniqueto water inrush monitoring in coal mine. Chin J Rock Mech Eng. 27:1932–1938.
- Kong WK. 2011. Water ingress assessment for rock tunnels: a tool for risk planning. Rock Mech Rock Eng. 44(6):755–765.
- Li W. 2009. Mechanism and model analysis of water inrush in highway tunnel construction in Karst Mountain Area. J Guizhou Univ. 26:125–128.
- Li LP, Li SC, Chen J, Li JL, Xu ZH, Shi SS. 2011. Construction license mechanism and its application based on karst water inrush risk evaluation. Chin J Rock Mech Eng. 30:1345–1355.
- Li SC, Liu B, Nie L, Liu Z, Tian M, Wang S, Su X, Guo Q. 2015a. Detecting and monitoring of water inrush in tunnels and coalmines using direct current resistivity method: a review. J Rock Mech Geotech Eng. 7(4):469–478.
- Li LP, Tu WF, Shi SS, Chen JX, Zhang YH. 2016. Mechanism of water inrush in tunnel construction in karst area. Geomat Nat Haz Risk. 7(Suppl. 1):35–46.
- Li SC, Zhou ZQ, Li LP, Shi SS, Xu ZH. 2013a. Risk evaluation theory and method of water inrush in karst tunnels and its applications. Chin J Rock Mech Eng. 32:1858–1867.
- Li SC, Zhou ZQ, Li LP, Xu ZH, Zhang QQ, Shi SS. 2013b. Risk assessment of water inrush in karst tunnels based on attribute synthetic evaluation system. TunnUndergr Space Technol. 38:50–58.
- Li LP, Zhou ZQ, Li SC, Xue YG, Xu ZH, Shi SS. 2015b. An attribute synthetic evaluation system for risk assessment of floor water in rush in coal mines. Mine Water Environ. 34(3):288–294.
- Mao BY, Xu M, Jiang LW. 2010. Preliminary study on risk assessment of water and mud inrush in karst tunnel. Carsologica Sinica. 29:183–189.
- McFeat-Smith I, Turner VD, Bracegirdle DR. 1985. Bracegirdle tunnelling conditions in Hong Kong. Hong Kong Engineer. 13:13–25.
- Raymer JH. 2001. Predicting groundwater inflow into hard-rock tunnels: estimating the high-end of the permeability distribution. Proceedings of Rapid Excavation and Tunnelling Conference. p. 1027–1038
- Saaty TL. 2008. Decision making for leaders: the analytic hierarchy process for decisions in a complex world. Pittsburgh, Pennsylvania: RWS Publications. ISBN 0-9620317-8-X.
- Shen XM, Liu PL, Wang JF. 2010. Evaluation of water inrush risks of karst tunnel with analysis hierarchy process. J. Rail Eng Soc. 12:56–63.
- Shi SS, Bu L, Li SC, Xiong ZM, Xie XK, Li LP, Zhou ZQ, Xu ZH, Ma D. 2017. Application of comprehensive prediction method of water inrush hazards induced by unfavourable geological body in high risk karst tunnel: a case study. Geomat Nat Haz Risk. 8(2):1407–1423.
- Wang D. 2008. Prediction of water inflow and its impact on construction of a karst tunnel in Shaoguan section of Wuhan Guangzhou line. Chengdu: Southwest Jiao Tong University.
- Wang YC, Geng F, Yang SQ, Jing HW, Meng B. 2019. Numerical simulation of particle migration from crushed sandstones during groundwater inrush. J. Hazard Mater. 362:327–335.
- Wang Y, Huang HW, Xue YD. 2009. Risk analysis of collapse for tunnels constructed by drill and blast method. J. Shenyang Jianzhu Univ Nat Sci. 25:23–27.
- Wang Y, Jing H, Han L, Yu L, Zhang Q. 2014. Risk analysis on swell–shrink capacity of expansive soils with efficacy coefficient method and entropy coefficient method. Appl Clay Sci. 99:275–281.
- Wang YC, Jing HW, Su HJ. 2017b. Effect of a fault fracture zone on the stability of tunnel-surrounding rock. Int J Geomech. 17:1–20.
- Wang Y, Jing H, Yu L, Su H, Luo N. 2017a. Set pair analysis for risk assessment of water inrush in karst tunnels. Bull Eng Geol Environ. 76(3):1199–1207.
- Wang YN, Lei YJ, Wang Y. 2016c. Intuitionistic fuzzy time series model based on intuitionistic fuzzy reasoning. Syst Eng Electron. 6:1332–1338.
- Wang Y, Yin X, Geng F, Jing H, Su H, Liu R. 2017c. Risk assessment of water inrush in karst tunnels based on the efficacy coefficient method. Pol J Environ Stud. 26(4):1765–1775.
- Wang YC, Yin X, Jing HW. 2016a. A novel cloud model for risk analysis of water inrush in karst tunnels. Environ Earth Sci. 75:1–13.
- Wang YC, Zhao N, Jing HW. 2016b. A novel model of the ideal point method coupled with objective and subjective weighting method for evaluation of surrounding rock stability. Math Probl Eng. 4:1–9.
- Ye ZH, Han XR, Zhang GM. 2006. Application of expert’s judging system in predicting karst water bursting in Zhujiayan tunnel. Carsologica Sinica. 25:139–145.
- Zhao M, Ren RR, Qiu WH. 2015. Experts’ weights method and computational experiment analysis based on intuitionistic fuzzy entropy measures. Contr Decis. 7:1233–1238.
- Zhou ZQ, Li SC, Li LP, Shi SS, Song SG, Wang K. 2013. Attribute recognition model and its application of fatalness assessment of water inrush in karst tunnels. Rock Soil Mech. 34:818–826.